Ban the Box, Criminal Records and Statistical Discrimination, Michigan Law, 2016
Download original document:
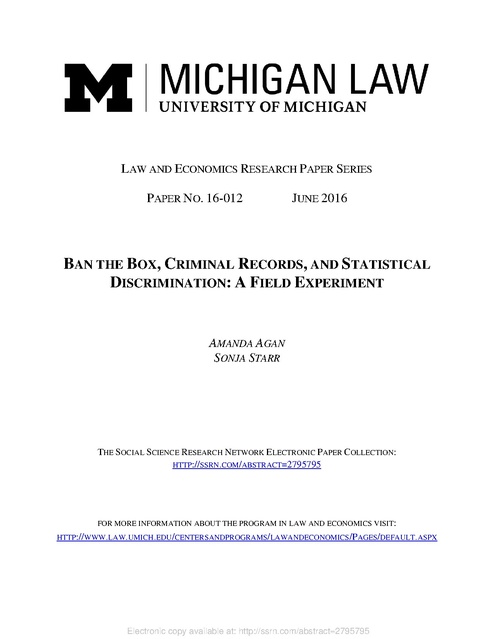
Document text
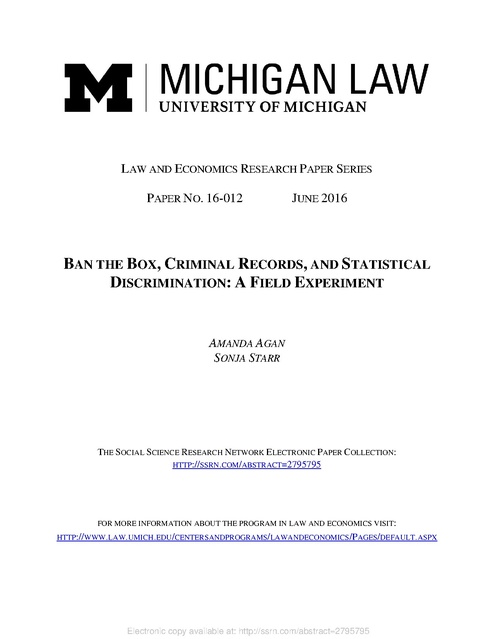
Document text
This text is machine-read, and may contain errors. Check the original document to verify accuracy.
LAW AND ECONOMICS RESEARCH PAPER SERIES PAPER NO. 16-012 JUNE 2016 BAN THE BOX, CRIMINAL RECORDS, AND STATISTICAL DISCRIMINATION: A FIELD EXPERIMENT AMANDA AGAN SONJA STARR THE SOCIAL SCIENCE RESEARCH NETWORK ELECTRONIC PAPER COLLECTION: HTTP://SSRN.COM/ABSTRACT=2795795 FOR MORE INFORMATION ABOUT THE PROGRAM IN LAW AND ECONOMICS VISIT: HTTP://WWW.LAW.UMICH.EDU/CENTERSANDPROGRAMS/LAWANDECONOMICS/PAGES/DEFAULT.ASPX Electronic copy available at: http://ssrn.com/abstract=2795795 Ban the Box, Criminal Records, and Statistical Discrimination: A Field Experiment Amanda Agan and Sonja Starr1 June 14, 2016 ABSTRACT “Ban-the-Box” (BTB) policies restrict employers from asking about applicants’ criminal histories on job applications and are often presented as a means of reducing unemployment among black men, who disproportionately have criminal records. However, withholding information about criminal records could risk encouraging statistical discrimination: employers may make assumptions about criminality based on the applicant’s race. To investigate this possibility as well as the effects of race and criminal records on employer callback rates, we sent approximately 15,000 fictitious online job applications to employers in New Jersey and New York City, in waves before and after each jurisdiction’s adoption of BTB policies. Our causal effect estimates are based on a triple-differences design, which exploits the fact that many businesses’ applications did not ask about records even before BTB and were thus unaffected by the law. Our results confirm that criminal records are a major barrier to employment, but they also support the concern that BTB policies encourage statistical discrimination on the basis of race. Overall, white applicants received 23% more callbacks than similar black applicants (38% more in New Jersey; 6% more in New York City; we also find that the white advantage is much larger in whiter neighborhoods). Employers that ask about criminal records are 62% more likely to call back an applicant if he has no record (45% in New Jersey; 78% in New York City)—an effect that BTB compliance necessarily eliminates. However, we find that the race gap in callbacks grows dramatically at the BTB-affected companies after the policy goes into effect. Before BTB, white applicants to BTB-affected employers received about 7% more callbacks than similar black applicants, but BTB increases this gap to 45%. 1 Princeton University and University of Michigan, respectively. The authors gratefully acknowledge generous funding from the Princeton University Industrial Relations Section, the University of Michigan Empirical Legal Studies Center, and the University of Michigan Office of Research, without which this study could not have taken place. We thank Will Dobbie, Henry Farber, Alan Krueger, Steven Levitt, Alex Mas, Emily Owens, Alex Tabarrok, David Weisbach, Crystal Yang and seminar participants at Princeton University, Rutgers University, the University of Chicago, the University of Michigan, UCLA, the University of Pennsylvania, the University of Toronto, the University of Virginia, the University of Notre Dame, the Society of Labor Economists Annual Meeting, and the American Law and Economics Association Annual Meeting for helpful comments. Finally, we thank every member of our large team of research assistants for their hard work and care, especially head RAs Louisa Eberle, Reid Murdoch, Emma Ward, and Drew Pappas, and our ArcGIS experts Linfeng Li and Grady Bridges. Electronic copy available at: http://ssrn.com/abstract=2795795 AGAN & STARR, BAN THE BOX , CRIMINAL RECORDS, AND STATISTICAL DISCRIMINATION 1. Introduction In an effort to reduce barriers to employment for people with criminal records, more than 100 jurisdictions and 23 states have passed “Ban-the-Box” (BTB) policies (Rodriguez and Avery 2016). Although the details vary, these policies all prohibit employers from asking about criminal history on the initial job application and in job interviews; employers may still conduct criminal background checks, but only at or near the end of the employment process. Most BTB policies apply to public employers only, but seven states (including New Jersey) and a number of cities (including New York City) have now also extended these restrictions to private employers. These laws seek to increase employment opportunities for people with criminal records. They are often also presented as a strategy for reducing unemployment among black men, who in recent years have faced unemployment rates approximately double the national average (Bureau of Labor Statistics 2015).2 The theory underlying this strategy is straightforward: black men are more likely to have criminal convictions than other groups (Shannon et al. 2011), and having a criminal record is a substantial barrier to employment (Pager 2003; Holzer, Raphael, and Stoll 2006; Holzer 2007; Pager, Western, & Bonikowski 2009). Thus, a policy that increases the employment of people with records should disproportionately help minority men. This effort could have unintended consequences, however. In the absence of individual information about which applicants have criminal convictions, employers might statistically discriminate against applicants with characteristics correlated with criminal records, such as race. In this scenario, applicants with no criminal records who belong to groups with higher conviction rates, such as young black males, would be adversely affected by BTB policies. While some observational research provides support for this theory (see, for example, Finlay 2009; Freeman 2008; Holzer, Raphael, and Stoll 2006), it has never been tested experimentally. Moreover, whether statistical discrimination will occur in the context of BTB (which merely delays employer access to criminal convictions, rather than precluding it entirely) has never been tested at all. We investigate the effects of BTB laws via a field experiment. We submitted nearly 15,000 fictitious online job applications to entry-level positions before and after BTB laws went into effect 2 See for example Minnesota Department of Human Rights (2015): “The Ban the Box law can mitigate disparate impact based on race and national origin in the job applicant pool, and is one tool to help reduce these inequalities.” New York City’s public Ban the Box law was passed as part of the Young Men’s Initiative, an initiative designed to address disparities faced by young Black and Latino men (City of New York 2016). Civil rights organizations are also major supporters of Ban the Box movements (NAACP 2014, Color of Change 2015). 2 Electronic copy available at: http://ssrn.com/abstract=2795795 AGAN & STARR, BAN THE BOX , CRIMINAL RECORDS, AND STATISTICAL DISCRIMINATION in New Jersey (March 1, 2015) and New York City (October 27, 2015). We sent these applications in pairs matched on race (black and white), which was our primary variable of interest. We also randomly varied whether our applicants had a felony conviction as well as two other characteristics that could also potentially signal criminal history to employers: whether the applicant has a GED, and whether the applicant has a one-year employment gap.3 Our study explores several key questions. First, we investigate whether employer callback rates vary by race and by felony conviction status, and whether there is an interaction between these effects. Second, we estimate how the availability of information about job applicants’ criminal records changes the racial gap in callback rates. Many employers, even absent BTB, choose not to ask about criminal convictions on employment applications, so we are able to draw cross-sectional comparisons between askers and non-askers in the pre-BTB period, as well as pre- and postcomparisons for the same employers before and after BTB. Our estimates of BTB’s effects exploit this cross-sectional and temporal variation in a triple-differences design. We estimate post-BTB changes in racial disparity after differencing out changes over the same time period among similar companies whose applications were unaffected by BTB. We also estimate the effects of having a GED and of a one-year employment gap. Finally, we assessed whether racial discrimination patterns vary based on the racial composition of the neighborhood employers are located in. Our experiment supports several key findings. First, white applicants overall received about 23% more callbacks compared to similar black applicants (a statistically significant difference of about 2.5 percentage points over a baseline of 10.6%, averaged across periods and criminal record statuses). Second, among employers that asked about criminal convictions in the pre-period, the effect of having a felony conviction is also significant and large: applicants without a felony conviction are 62% (5.2 percentage points over a baseline of 8.4%) more likely to be called back than those with a conviction, averaged across races. Third, in contrast to prior research (Pager 2003; Pager, Western, and Bonikowski 2009), we find no significant interaction between the effects of race and felony convictions. Fourth, although one might have expected that a GED (versus a high school diploma) or a 1-year gap in employment might have been disfavored or used by employers as a proxy for a criminal record, neither characteristic significantly affects callback rates. 3 We use “criminal record” and “felony conviction” interchangeably here; our experimental design varies whether employers have a felony conviction. Employers that ask about records on initial job applications overwhelmingly limit their questions to convictions (not arrests), and most limit them to felony convictions specifically. 3 AGAN & STARR, BAN THE BOX , CRIMINAL RECORDS, AND STATISTICAL DISCRIMINATION Our estimates of BTB’s effects on callback rates imply that BTB substantially increases racial disparities in employer callbacks. We find that BTB expands the black-white gap by about 4 percentage points, multiplying the gap at affected businesses by a factor of about six. In our main specification, before BTB, white applicants to BTB-affected employers received 7% more callbacks than similar black applicants, but after BTB this gap grew to 45%. This increase in racial inequality in callback rates could come from a combination of two sources. First, there could be a reduction in callbacks to black applicants with no criminal record, i.e. employers statistically discriminate against black applicants when they cannot see information about criminal history. In addition, there could be an increase in callback rates to white applicants with criminal records if employers statistically generalize that white applicants do not have records. Our results suggest some support for both of these mechanisms. Both explanations for the increasing gap involve forms of statistical discrimination, and provide reason to question the idea that BTB will reduce racial disparity in employment. When our results are broken down by jurisdiction, some interesting differences emerge. The overall effects of having a criminal record are larger in New York City than in New Jersey, where people without records receive 78% more callbacks (versus 45% in New Jersey). On the other hand, the main effects of race are much larger in New Jersey, where white applicants are 38% more likely to receive a callback (vs. a not statistically significant 6% in New York City). Further analysis suggests that this difference may be partly, but not mostly, explained by the city’s greater racial diversity. Businesses in whiter neighborhoods much more strongly favor white applicants, but even accounting for these differences, New York’s race gap in callback rates is considerably smaller. Meanwhile, the effects of BTB are fairly similar in both jurisdictions—favoring white applicants relative to black applicants—albeit operating on different pre-BTB baselines. This study makes several distinct contributions to the literature. First, this is the first empirical study of BTB’s statistical discrimination effects,4 and we hope it will inform ongoing legislative debates about BTB throughout the country. Second, removing information about criminal history on job applications allows us to use field-experimental methodology to contribute to the literature on statistical discrimination in employment, which has not generally used such methods.5 Although 4 One of the authors is currently carrying out observational research on BTB’s effects on public employers, detailed further below (Starr 2015). 5 See List (2004) for an experimental approach to statistical discrimination in another context, sports card trading. 4 AGAN & STARR, BAN THE BOX , CRIMINAL RECORDS, AND STATISTICAL DISCRIMINATION our study is not a pure experiment (a key variable, whether the application asks about records, is not manipulated), our ability to perfectly observe and randomize all of our fictional applicants’ characteristics allows us to avoid many of the most likely threats to causal inference that affect purely observational research, and leaves us better equipped than are purely observational researchers to tease out the mechanisms underlying the effects we observe. Third, our assessment of geographic differences adds another dimension to the experimental literature on racial discrimination in employment; to our knowledge, no prior auditing study has assessed how differences in employer behavior vary based on neighborhood racial composition. Finally, we make a methodological contribution to the literature on auditing, which has for decades been a central tool for empirical research on discrimination in employment, housing, lending, and other areas. To our knowledge, this is the first study to use auditing to assess the effects of a policy, rather than to obtain a static picture of discrimination patterns. Because researchers cannot randomize the application of the policy itself, using auditing to assess policies requires combining the field-experimental approach with additional methods of causal inference— in this case, differences-in-differences analysis. We believe that combining auditing with quasiexperimental analysis of policy changes enriches the study of discrimination. 2. Background and Literature Review 2.1 Ban-the-Box Policies and their Motivations The “box” referred to in “Ban the Box” (and hereinafter in this paper) is the question on a job application form asking whether the applicant has been convicted of a crime – which is often accompanied by yes and no checkboxes. While BTB policies vary, all of them ban employers from asking such questions on application forms. The policies typically also bar employers from asking about records during an initial job interview. They do not, however, permanently bar them from performing criminal records checks. Instead, employers must delay these checks until a later stage in the hiring process: in New Jersey, that stage is anytime after the first interview, and in New York City it is after a conditional job offer is made. Some BTB laws also substantively restrict the role that criminal records can play in employers’ ultimate decisions (roughly paralleling existing federal anti-discrimination guidelines), but New Jersey’s and New York’s do not.6 6 New Jersey’s law affects only the “initial employment application process” (N.J. P.L. 2014, Ch. 32). Meanwhile, New York already had, long before the beginning of this study, a substantive restriction requiring employers to consider 5 AGAN & STARR, BAN THE BOX , CRIMINAL RECORDS, AND STATISTICAL DISCRIMINATION BTB is often presented as an important tool for reducing racial disparity in employment, and especially for improving access to employment for black men (Pinard 2014, Southern Coalition for Social Justice 2013, Clarke 2012, and Community Catalyst 2013). Black unemployment levels are generally about twice those of whites (DeSilver 2013), so expanding black male employment is a priority for many policymakers and civil rights advocates (see, for example, NAACP 2014). This argument for BTB proceeds in several steps. First, black individuals are much more likely to have criminal records than are other groups. Brame et al (2014) find that by age 23, 49% of black men have experienced an arrest versus 38% of white men; Shannon et al. (2011) estimate that 25% of the U.S. black population has a felony conviction, compared with only 6% of the non-black population. Second, having a criminal record, especially a felony conviction, is a substantial barrier to employment (Holzer, Raphael, and Stoll 2006; Pager 2003; see Holzer 2007 for a review of studies). One can expect this employment hurdle to have a disparate impact on black men because they are more likely to have records.7 Finally, advocates argue that BTB will effectively improve access to employment for people with records. This step in the reasoning may not be so obvious, since BTB only delays rather than prevents employer access to criminal records. But BTB’s motivations are premised on a psychological claim: “Rejection is harder once a personal relationship has been formed” (Love 2011). The goal is to stop employers from making the premature judgment to throw out everyone with a record, and instead to encourage more nuanced consideration, which is believed to be more likely if employers have already met with the candidate (Pinard 2010). In short, the objective is to enable candidates with records to get their foot in the door. 2.2 The Potential for Statistical Discrimination There is, however, a plausible counterargument to the view that BTB will improve black male employment prospects. Economists have frequently suggested that in the absence of specific information about individuals (or where obtaining such information is costly), employers and other whether a conviction is job-relevant; this restriction is unchanged by BTB. N.Y. Correction Law Sec. 752. In any event, employers in all U.S. jurisdictions are subject to similar substantive restrictions at the federal level. The Equal Employment Opportunity Commission has for decades interpreted the Civil Rights Act of 1964 to bar employers from blanket bans on persons with criminal records, to avoid racially disparate impacts. According to EEOC, employers must consider “the nature and gravity of the offense or conduct; the time that has passed since the offense, conduct, and/or completion of the sentence; and the nature of the job sought” (EEOC 2012). 7 This is why EEOC interprets race discrimination law to constrain employers’ treatment of criminal records (EEOC 2012). 6 AGAN & STARR, BAN THE BOX , CRIMINAL RECORDS, AND STATISTICAL DISCRIMINATION decision-makers are more likely to rely on statistical generalizations about groups (Phelps 1972; Arrow 1973; Aigner and Cain 1977; Fang and Moro 2011). In our context, this theory implies that if employers cannot ascertain at the outset which applicants have criminal records, they may use observable characteristics such as race to infer the probability an applicant has a criminal history, and this may trigger discriminatory treatment (Finlay 2009; Freeman 2008; Holzer, Raphael, and Stoll 2006). Thus, for example, young black men without criminal records could be hurt by BTB if employers assume that they are likely to have a record, based on assumptions about young black men generally. Of course, BTB does not permanently bar employers from obtaining record information, which could reduce the incentive to rely on demographic proxies. Still, employers may want to avoid the costs associated with interviewing and making tentative offers to candidates that they fear will ultimately be disqualified after the background check, especially if those search costs are high. The premise of the theory of statistical discrimination relies on the idea that the unobservable information is costly to obtain, not necessarily inaccessible (Phelps 1972; see also Stoll (2009) for an argument that BTB might trigger statistical discrimination). If BTB does trigger statistical discrimination against black men, it would subvert the policy objective of expanding their access to employment. Moreover, although statistical discrimination on the basis of race is sometimes defended as rational (if employers’ generalizations are accurate), it is plainly unlawful in the employment context. This prohibition reflects a policy judgment disfavoring racial generalizations and favoring expansion of workplace opportunities for historically excluded groups. Title VII of the Civil Rights Act of 1964 prohibits hiring discrimination on the basis of race as well as gender, and does not permit otherwise-illegal treatment to be based on statistical generalizations about groups, even if there is empirical support for the generalization.8 But these restrictions are famously difficult to enforce, and the fact that statistical discrimination would be an unlawful response to BTB does not mean it is impossible, or even unlikely. No prior study has yet assessed the potential statistical discrimination effect of BTB, although one of this study’s authors is currently conducting a parallel observational study focusing 8 For example, in City of Los Angeles Department of Water and Power v Manhart, 435 U.S. 702 (1978), the Supreme Court held that an employer could not rely, in formulating terms of a pension plan, on the well-founded actuarial prediction that women live longer. 7 AGAN & STARR, BAN THE BOX , CRIMINAL RECORDS, AND STATISTICAL DISCRIMINATION on public employers.9 Outside the BTB context, several observational studies have suggested that lack of employer access to criminal records may encourage statistical discrimination (Bushway 2004; Holzer, Raphael, and Stoll 2006; Stoll 2006; and Finlay 2014). Holzer, Raphael, and Stoll (2006) and Stoll (2009) use survey data from establishments in four cities to show that employers who perform criminal records checks are more likely to hire African-Americans; the researchers interpret this finding as evidence of statistical discrimination. Bushway (2004) studies cross-state variation in accessibility of criminal records databases and finds that states with greater accessibility have smaller race gaps in employment. Finlay (2014) exploits temporal variation in states’ expansion of Internet criminal records databases and uses individual longitudinal data that includes criminal history; he finds that blacks without records have better employment outcomes under open records policies. However, Finlay (2014) also finds that the net employment effect of open records on young black men appears to be negative, suggesting that the benefits of open records to nonoffenders within that group may be outweighed by harms to offenders. Statistical discrimination has also been studied in contexts other than criminal records. For example, Wozniak (2015), relying on a similar theory, shows that legislation that allows drugtesting increases black employment, with the largest increases among low-skill black men. Autor and Scarborough (2008) find that a retail chain’s adoption of a pre-employment personality test did not hurt black employment success even though black candidates had lower scores; they interpret this as evidence that employers were statistically discriminating before they used the test. Clifford and Shoag (2016) show that bans on the use of credit checks by employers reduce black employment and employment of young people. 2.3 Auditing Research “Auditing” or “audit” studies are field experiments in which researchers randomly vary the characteristics of interest about a person with whom a subject interacts (for example, a job applicant). While some audit studies use actors for in-person communications, many use written or online communications (such as resumes and cover letters) in which the “person” in question does 9 Starr (2015, unpublished draft on file with author) uses the Current Population Survey and American Community Survey, exploiting temporal variation in the dates of cities’ and states’ adoption of BTB. Preliminary results using the CPS show a substantial increase in racial disparity in rates of being employed by local governments, but the analysis of the ACS shows no significant change. Both datasets have some limitations that might explain the differences, but it is not clear whether one or the other result is “right” (Starr 2015). In addition, we are also aware of a forthcoming working paper by Doleac and Hansen (2016) that will study the effects of BTB laws using CPS data; however, the draft was not available at the time of this posting. 8 AGAN & STARR, BAN THE BOX , CRIMINAL RECORDS, AND STATISTICAL DISCRIMINATION not exist, so researchers can directly manipulate characteristics of interest. Such designs have been used to test employment discrimination on the basis of characteristics such as race, gender, length of unemployment spell, age, and type of postsecondary education (Neumark 1996; Bertrand and Mullainathan 2004; Lahey 2008; Oreopoulos 2011; Kroft, Lange, and Notowidigdo 2013; Deming et al. 2014; Farber et al 2015; Neumark et al 2015. In-person audits have been used by Pager (2003) and Pager, Western, and Bonikowski (2009) to explore the effects of criminal records on employment outcomes and its interaction with race, finding that criminal records have a heightened adverse effect on black applicants. For a review of auditing methods, see Riach and Rich (2002). Auditing can provide a stronger basis for causal inference than observational methods, because only the variables of interest are varied. Additionally, compared to lab experiments, audit studies provide stronger external validity, since they test real employer reactions. Despite its prominent role in discrimination research, auditing has to our knowledge never been used to study the effects of a policy on discrimination. Instead, it has been used to obtain a onetime snapshot of discrimination in a particular decision process. In our view, auditing holds considerable untapped potential as a tool of policy analysis, and we hope to demonstrate that potential. The principal challenge in auditing for policy analysis is that it is no longer a pure experiment. Applicant characteristics are randomized, but the policy variable is determined by nature, not by the researchers, and its applicability may be correlated with unobserved confounding variables (such as seasonal variations). Obtaining causal identification in this context requires combining the field-experimental method with another econometric method to filter out these potential confounds. We do so using triple-differences analysis. Because this approach involves estimating three-way interactions, it requires a larger sample than most auditing studies require, making it relatively resource intensive. However, it is otherwise quite straightforward. 3. Experimental Design We submitted online job applications on behalf of fictitious job applicants to low-skill, entry-level job openings both before and after BTB went into effect in New Jersey and New York City. New Jersey’s version of BTB, the “Opportunity to Compete Act”, was passed on August 11, 2014 and became effective March 1, 2015. We submitted applications in New Jersey in the preBTB period between January 31 and February 28, 2015 and in the post-BTB period between May 4 and June 12, 2015. New York City’s BTB law went into effect on October 27, 2015. We submitted 9 AGAN & STARR, BAN THE BOX , CRIMINAL RECORDS, AND STATISTICAL DISCRIMINATION applications in New York City between June 10 and August 30, 2015 (the pre-BTB period) and between November 30, 2015 and March 31, 2016 (the post-BTB period). 3.1 Choosing Employers and Job Postings Our subjects were exclusively private, for-profit employers. We principally targeted chain businesses because such businesses are likely to have online job applications and to be subject to the NJ BTB policy, which exempts employers with fewer than 15 employees. We rely on two main sources for locating job openings. First, we searched snagajob.com and indeed.com, two large online job boards; snagajob.com focuses specifically on hourly employment. Second, with certain exceptions, we also directly searched the employment websites of chain businesses meeting certain size criteria in certain industries: restaurants, department stores, home centers, grocery and convenience stores, pharmacies, miscellaneous retail, service stations, and hotels/motels.10 We hired a large team of University of Michigan student research assistants to search for jobs using these methods, apply to them, and record information about the job applications. We directed them to look for jobs that were suitable for candidates with limited work experience, no post-secondary education, and no specialized skills. Such jobs are predominantly non-supervisory team-member jobs at fast food and other restaurants, grocery and convenience stores, and other retail establishments. We focus on these sectors because they almost universally use job applications (particularly online applications) rather than resumes as an initial screen of job applicants; employers that do not use applications do not have a “box” that can be banned. In addition, these sorts of jobs are likely to attract applicants with criminal records, who disproportionately tend to have relatively little work experience or post-secondary education. 10 In New Jersey, we applied to businesses with at least 30 locations and 300 employees in the state. In New York City, we applied to chains with at least 20 locations in the city, plus smaller chains if we had also applied to them in New Jersey. Employers that did not use online job applications were excluded, although the vast majority of chains meeting those size criteria do use them, as well as virtually all employers that advertise postings on Snagajob or Indeed. We also excluded a few chains due to extremely arduous online application processes (e.g., those that took our RAs more than an hour to complete). We excluded employers targeting an overwhelmingly female clientele, such as cosmetics companies. Finally, some employers required full SSNs on job applications. For ethical reasons, we wanted to avoid using potentially real SSNs, and thus assigned our applicants invalid SSNs (beginning with 9xx or 666). Some employers we initially tried to apply to had systems that automatically detected these invalid SSNs, and we excluded those businesses from further applications. It is possible that setting up such a system could be correlated with special interest in criminal records, such that excluding this pool means that our estimates of the effect of a criminal record will be lower than they would otherwise be. However, within the pool we did apply to, there was no correlation between whether employers asked for an SSN at all and whether they asked about criminal records. 10 AGAN & STARR, BAN THE BOX , CRIMINAL RECORDS, AND STATISTICAL DISCRIMINATION 3.2 Applicant Profiles Our fictitious applicants are all male and approximately 21 to 22 years old.11 We created applicant profiles that included answers to a wide range of questions that employers could potentially ask, using the Resume Randomizer program created by Lahey and Beasley (2009). Our research assistants then filled out the applications based on those profiles. Each applicant profile included a name, a phone number, an address, an employment history, a unique email address, two references with phone numbers, information on high school diploma or GED receipt, a felony conviction status and information about the criminal charge, a formatted resume, and answers to many other routine application questions concerning job requirements, availability, and pay sought (minimum wage).12 The profiles were created in pairs, each consisting of one black and one white applicant. These pairs were assigned to the same store in the same time period. Our applicants were all similar on all but our randomly assigned treatment dimensions. In addition to race, those dimensions are: (1) Has felony criminal conviction or not a. (Conditional on conviction): convicted of property crime or drug crime (2) Has 1-year employment gap versus a 0- to 2-month gap (referred to as “no gap” below) (3) GED or High School Diploma These characteristics were randomized with equal (50%) probability. In addition to race, we chose to vary the employment gap and high school diploma status because they are also characteristics that hiring managers might perceive as correlated with criminal history.13 Race is indicated via the name of the applicant, as discussed further in Section 3.3 below. The crimes our applicants were 11 Due to legal restrictions on age discrimination, age and high school graduation year are rarely requested on job applications, so age can only loosely be inferred by the length of work history. 12 It was not possible for the applicant profiles to anticipate every question asked on the applications of all of the businesses to which we applied, especially as many applications require an extensive online personality or skills assessments. For this reason, we relied on the RAs’ judgment, but provided detailed training about what employers would likely ask and what they are generally looking for; we are confident that our RAs were capable of filling out these assessments in a satisfactory manner that would “clear the bar” and allow the applicant to be considered. 13 As of 2005, 13.6% of GEDs were issued in state and federal Prisons (Heckman and LaFontaine 2010). The relationship between GED, race, and criminal records is further addressed in the Discussion. The one-year employment gap is meant to signal potential time spent incarcerated or dealing with the criminal justice process. That an applicant may have a felony conviction and no employment gap is not implausible: of individuals charged with felonies in state courts, 62% are not detained before trial; 27% of those convicted receive no incarceration, and of those incarcerated 48% receive sentences of 1-3 months (Reaves 2013). In addition, the felonies we chose were relatively minor. 11 AGAN & STARR, BAN THE BOX , CRIMINAL RECORDS, AND STATISTICAL DISCRIMINATION convicted of were relatively minor felonies – either property crimes (e.g., shoplifting, receiving stolen property, theft) or drug crimes (e.g., controlled substances possession). We chose 40 geographically distributed cities/towns in New Jersey and 44 neighborhoods throughout New York City’s boroughs to serve as “centers” where the applicants’ addresses would be located; each center then served as a base for application to nearby employers.14 All applicant addresses were in racially diverse, lower- to-middle-class neighborhoods. Other job applicant characteristics such as work history, address within center, high school name or GED program, and names of references were designed to have similar connotations, although they were randomly varied among a set of similar options (e.g., different high schools with similar demographic and academic profiles; employment history at different fast food restaurants) and forced to differ within pairs so as to disguise the similarity of the applications. Each applicant received a unique email account with the address format randomly varied. Phone numbers were assigned at the center/race/crime level and thus shared by multiple applicants, but in a way that almost entirely avoided using the same number more than once within any chain. For more details on profile contents and applicant characteristics, see Appendix A1. 3.3. Indicating Applicant Race Race is a central characteristic of interest in our study, and we signal race by the name of the applicant.15 To identify racially distinctive names, we used birth certificate data for babies born between 1989 and 1996 from the New Jersey Department of Health (NJDOH), which encompasses the cohort that would include our applicants. We then chose a set of first and last names that were racially distinctive (meeting threshold requirements for the percentages of babies given that name who were black or non-Hispanic white) and common (meeting threshold requirements for the total number of babies born with that name and race).16 Each applicant was then assigned a random first 14 This assignment method differed somewhat from New Jersey to New York City, due to differing geographic concerns. In New Jersey, we assigned each municipality in the state to its nearest center. For example, applicants from Princeton, NJ (one of our centers) applied to jobs in Princeton as well as in the nearby towns of East Windsor, Hightstown, Monmouth Junction, Plainsboro, Princeton Junction, and Skillman. These towns are all within 15 miles of Princeton. In New York City, because distances are much smaller generally, we prioritized distributing chain locations across centers (so that no chain received too many applications from the same neighborhood) and minimized distance within equal-distribution constraints, rather than in absolute terms. 15 This is a common strategy in auditing studies (Bertrand and Mullainathan 2004). 16 Because blacks are a much smaller fraction of the population, these thresholds varied by race: the minimum percentages were 80% for white first names, 85% for white last names, and 70% for black first and last names, while the minimum frequencies were 450 for white first names, 150 for white last names, 150 for black first names, and 100 for black last names. The white first names we used averaged 84% non-Hispanic white and 5% black, and the white last 12 AGAN & STARR, BAN THE BOX , CRIMINAL RECORDS, AND STATISTICAL DISCRIMINATION name and random last name from the appropriate list. We expect that the combination of racially distinctive first and last names will produce a very strong racial signal: according to the birth certificate data, 96% of persons with first and last names on our “black” list are black, and 91% of persons with first and last names on our “white” list are white. A list of the names we used is provided in Appendix A2. One critique of using racially distinctive names to signify race in audit studies is that such names could also signal socioeconomic status, which employers may also believe to be correlated with productivity (Fryer and Levitt 2004). We note first that our applications provided a great deal of concrete SES-related information to employers, including complete work histories, education, current neighborhood, high school location, and wage sought. Employers thus hardly need to rely on names to draw SES inferences—whereas no other application characteristics signaled race, because those characteristics were randomized and were designed to be race-neutral. Nevertheless, to mitigate this concern we used only names falling below the socioeconomic median for whites (as measured by maternal education recorded on the birth certificate, the best available indicator), reducing the implied-SES gap between our white and black names. 17 In addition, because the names we chose were common, we avoided any perceived socioeconomic connotations that may be associated with the choice of unusual names or spellings. Although some SES gap remains, it is very similar to the overall SES gap between black and white citizens—that is, choosing distinctive names did not amplify the gap.18 Distinctively white or black names do not point to an individual being a high- or low-SES outlier within their race; in fact, such names are very common. In our birth certificate sample, 47% of black children have a racially distinct first name and 36% have a racially distinct last name (as we define distinctiveness, see footnote 17), while 35% of white children have a racially distinct first name and 65% have a racially distinct last names averaged 90% non-Hispanic white and 3% black. The black first names we used averaged 88% black and 3% non-Hispanic white; the black last names averaged 77% black and 17% non-Hispanic white. We eliminated a few first names that either were not distinctively male or that had strong associations with Islam or Judaism, so as to avoid confounding the effects of race with those of perceived gender or religion. A heavily overlapping name list would have been chosen had we classified names in the manner of Bertrand and Mullainathan (2004) or Fryer and Levitt (2004). 17 It was not possible to create a list of racially distinct names that are completely balanced on SES indicia, because virtually every distinctively white name averages higher than virtually every distinctively black name, due to socioeconomic stratification by race. 18 According to the birth certificate data, persons with first and last names that were both on our “black” lists had an average maternal education level that was nearly identical to the overall black average; persons with first and last names that were both on our “white” lists had nearly the same average maternal education as the overall white average. 13 AGAN & STARR, BAN THE BOX , CRIMINAL RECORDS, AND STATISTICAL DISCRIMINATION name. Thus, to the extent employers make assumptions about SES based on racially distinctive names, these are assumptions that would affect a large fraction of real-world job applicants. 3.4 The Job Application Process Each RA was randomly assigned one or more of our geographic centers in which to search for jobs via the above-described methods, and applied for those jobs using profiles from that center; the profile order within and between pairs was random. While submitting the job application, they filled out a spreadsheet that indicated, among other things, which profile was used, the date and time of the submission, the name of the chain being applied to, the name of the position, address of the location, and whether the application asked about criminal history. With some time lag, a second application was submitted to each store. Most applicant profiles (approximately 59%) were sent to only one business. However, we sometimes used the same profile pairs to apply to multiple nearby locations of the same chain, as real-world applicants might do; our criteria for grouping the applications in this way differed between New Jersey and New York City, producing more grouping in New Jersey.19 The post-BTB application procedure was essentially the same, except that we began with the chains that we had already identified and applied to in the pre-period. Each specific store that we applied to at least once in the pre-period was assigned a new pair of profiles. The RAs were assigned to submit applications to these stores in an order that was designed to make the length of time between members of each pair roughly mirror what occurred in the pre-period. Stores thus received up to four applications total, one pair in each period. It was sometimes not possible to send a complete set of four applications to an establishment. The primary reason for this was that the store was hiring in one period but not the other. In addition, a few RA assignments were not completed before BTB’s effective date, leaving some applications unsent; this especially occurred in the New Jersey pre-period, our first wave of applications, which had to be completed relatively quickly. In New Jersey, we filled in these gaps 19 In New Jersey, we were concerned that the same hiring managers might cover multiple locations of chains and might become suspicious upon noticing groups of applicants coming within a short time from the same nearby town. Accordingly, we used the same applicant profiles for all locations that were assigned to a given center. In New York, our concerns were different: the centers are not towns and likely appear less distinctive to managers, and we had more available time before BTB’s effective date, so we were able to space out the timing of our applications. Thus, in New York we chose to increase power by sending each application to only one location, except for the largest five chains (in which we sent each applications to up to two or three stores). We forced addresses and phone numbers to differ within chains, such that chains would not receive multiple applications from the same ones. 14 AGAN & STARR, BAN THE BOX , CRIMINAL RECORDS, AND STATISTICAL DISCRIMINATION in the post-period whenever possible, and identified some new opportunities on snagajob.com. In New York City, our pre-period wave represented a quite comprehensive search, so we limited the post-period wave to the same locations that we had sent at least one application to in the pre-period; there was some attrition due to unavailable jobs in the post-period. As a result, while the pre-and post-period samples are almost identical in size, the percentage of applications that are from New York City was higher in the pre-period (60% versus 52%), and moreover, the composition of chains and stores is not identical across periods. We address these concerns below. 3.5 Measuring Outcomes The main outcome of interest is whether an application receives a voicemail or email from an employer requesting that the applicant contact them or requesting an interview. We refer to this outcome as a callback (although it includes emails). For some alternative specifications, we focus on responses that specifically requested an interview. However, this outcome variable is subject to measurement error because employer messages often do not specifically mention an interview even if they are seeking to interview the applicant. Thus, our preferred specification uses the callback as the outcome. Phone calls and emails were tracked for eight weeks from the application date. In New Jersey, our pre-BTB data collection ended on April 25, 2015 (for the last applications sent);20 our post-BTB data collection ended on August 6, 2015.21 In New York City, our pre-BTB data collection ended on October 26, 2015, and our post-BTB data collection ended on May 26, 2016. 4. Summary Statistics and Main Effects of Applicant Characteristics on Employer Callbacks We submitted a total of 15,220 applications, of which 14,640 are included in our analysis sample.22 These include 6,401 applications in New Jersey and 8,239 in New York City. The 20 Note that although this is considerably after BTB went into effect, all of the applications were submitted before it went into effect, which meant that the applications did contain the criminal records question (except for businesses that voluntarily omitted the question even prior to BTB). Because our outcome of interest is the employer response to the initial application (not subsequent stages of employer decision-making, such as ultimate hiring decisions), consideration of these applicants should therefore not be affected by BTB. 21 RAs posing as the applicants responded to employer messages by leaving brief messages thanking them but stating that the applicant was no longer available. We had no further communications with the businesses and, per IRB constraints, did not collect any information about the individuals we interacted with. 22 The remaining 580 observations (3.8% of those we sent) were dropped for several reasons. First, when an entire chain was applied to only in the pre-period or only in the post-period, we had no way to code whether the application had the criminal record “box” in the other period, so the treatment variable could not be coded. Second, some stores had inconsistencies within one or both rounds as to whether the box was present. The most common reason for these inconsistencies was early precompliance with BTB (which in both jurisdictions was announced several months before it went into effect), occurring before we sent the second application but after the first. Another reason was RA mistakes in interpreting the job application form—usually answering the criminal history 15 AGAN & STARR, BAN THE BOX , CRIMINAL RECORDS, AND STATISTICAL DISCRIMINATION summary statistics and results presented in the tables and figures below combine both jurisdictions; in Appendix A3, we replicate several of the tables and figures for New Jersey and New York separately. The applications were sent to 4,292 stores (that is, establishments) in 296 chains. We begin with summary statistics and then analyze the main effects of our randomly varied characteristics on employer callbacks. 4.1 Summary Statistics Summary statistics are presented in Table 1a, by period and overall. As expected, approximately 50% of our applications had each of our randomized characteristics of interest. However, the prevalence of our other variable of interest—whether the application asked about criminal records—was determined by nature (that is, by the chains), not by randomization. Among our pre-period applications, 36.6% had a required criminal record question (the “box”). In the postperiod, 3.6% still had the box (“noncompliers”), leaving approximately 33% of the sample as “treated” observations: employers that had the box before BTB, but not after. Overall, 1,715 applications received callbacks, a rate of 11.7% overall. This rate was slightly higher in the post-period (12.5% vs. 10.9%), and lower in NYC than in NJ (9.4% vs 14.7%; see Appendix Tables A4 and A5). Among the callbacks, about 55% specifically mentioned an interview. The overall callback rate for white applicants was 12.9%, and 10.5% for black applicants. In both periods, callback rates were much more similar across the other randomized characteristics (GED/H.S. diploma and employment gap). Although the race gaps appear fairly similar across time periods (2.1 percentage points in the pre-period and 2.8 percentage points in the post-period), they represent averages that do not differentiate treated and untreated observations, and mask large changes occurring at treated stores, as discussed below. 4.2. Effects of Applicant Characteristics on Callback Rates We begin by assessing the underlying employment patterns that BTB is principally designed to address. How much of an effect does having a criminal record have on employer callback rates? question even when they were not required to because they missed a disclaimer telling New Jersey or New York City applicants not to answer the question. In either event, when the two observations from the same store and round were in conflict, we discarded the observation that was an outlier from the overall chain norm. The effect was to drop RAmistake observations, and in the precompliance cases, to drop the later, non-box observation. Third, we also dropped some businesses (about 1% of the sample) that appeared, mysteriously but presumably due to an administrative mistake, to add the box after BTB, and therefore could not be coded as 0 or 1 on the Treated variable. We add these back in in a robustness check below, with the coding of -1. 16 AGAN & STARR, BAN THE BOX , CRIMINAL RECORDS, AND STATISTICAL DISCRIMINATION How much does this vary by race? Table 1a did not show a breakdown of callback rates by criminal record status, because criminal record is unobserved by employers for 63% of our applications even in the pre-period, making that breakdown not very informative for the full sample. Instead, we show separate summary statistics in Table 1b limited only to pre-BTB period observations where the application had the box. Among companies with the box, callback rates are about 60% higher for applicants without criminal records (about 5.1 percentage points, over a base rate of about 8.5%). Applicants with drug convictions had similar callback rates to those with property crime convictions—perhaps surprisingly, as one might have expected employers to be particularly concerned about potential employee theft. However, all the crimes we used were of similar legal severity—relatively low-level felonies. As Table 1b further shows, for employers with the box in the pre-period, the callback rate advantage for applicants without records is slightly larger for white applicants (5.7 percentage points, or 69% higher than the base rate of 8.3%) than for black applicants (4.5 percentage points, or 52% higher than the base rate of 8.6%). Overall, when employers ask about records, we see essentially no race gap in callback rates: the white average is 11.1% and the black average is 10.9%. Figure 1 puts those numbers into perspective by comparing them to the callback rates for white and black applicants to employers without the box in the pre-period. Among these employers, white applicants have a 3.1-percentage-point (or 33%) callback rate advantage (12.5% vs. 9.4%; p<0.001). The overall callback rates at both groups of employers are essentially identical (11%), but the separation between white and black applicants is seen only at the employers who do not ask about criminal records. This is suggestive evidence for the statistical discrimination theory, although other differences between these employers could potentially underlie these cross-sectional differences; the triple-differences results below provides a stronger basis for causal inference. Table 2 provides multivariate regression estimates of the main effects of race, record, GED status, and employment gap on callback rates. These estimates closely parallel what we see in the summary statistics, which is not surprising given that all the applicant characteristics were distributed randomly. All the results shown in Table 2 are for both periods combined (unlike Table 1b and Figure 1, which were for the pre-period only), but the regression results look similar if only the pre-period observations are used. Columns 1 and 2 show the results of regressions run in the full sample of 14,640 cases. They differ in that the Column 2 regression adds chain fixed effects (with the smallest chains grouped by business category) and center fixed effects, which make little 17 AGAN & STARR, BAN THE BOX , CRIMINAL RECORDS, AND STATISTICAL DISCRIMINATION difference. Both imply that white applicants are on average about 2.4 percentage points more likely to receive a callback from an employer, which corresponds to a statistically significant 23% increase in callbacks over the 10.5% black baseline (p<0.001). Note that the estimated criminal record effect in these regressions (about 1.5 percentage points) substantially understates the magnitude of the real criminal record effect, because in four-fifths of the sample, criminal record was not actually conveyed to the employer. Columns 3 and 4 parallel the regression in Column 2, but they are limited to observations without and with the box, respectively. (Although the time periods remain combined, the Column 4 regression’s observations are almost entirely from the pre-period, since only 3.6% of businesses retained the box after BTB.) The criminal record variable is removed from the non-box Column 3 regression because no criminal record information was conveyed. The advantage to white applicants appears only in the non-box sample, in which it is about three percentage points (Col. 3); there is no race gap at stores with the box (Col. 4). Column 4 also shows a statistically significant 5.2-percentage-point criminal record effect in the box sample (p<0.001). This represents a 63% higher callback rate for persons without records, compared to the 8.2% baseline for persons with records in this sample. Column 5, which is also limited to observations with the box, shows that this effect is similar for property crimes and drug crimes. Finally, Column 6 adds an interaction of the race and criminal record variables, within the box sample only. The negative criminal record effect is 1.5 percentage points larger for white applicants—among applicants without criminal records, whites have a slightly higher callback rate, but among applicants with criminal records, they have a slightly lower callback rate. This interaction is not statistically significant, but its sign is nonetheless interesting given that earlier, smaller auditing studies (Pager 2003; Pager, Western, and Bonikowski 2009) had found a strong interaction in the opposite direction. In every specification and sample, having a one-year employment gap and obtaining a GED rather than a high school diploma have little effect on employer responses. Point estimates for both are close to zero, and the GED coefficient varies in sign across the specifications and samples. 4.3 Alternative Specifications and Samples: Race and Criminal Record Effect In Table 3A, we show the race effect from several alternative specifications and samples. All combine “box” and “non-box” observations from both time periods, and all include chain and 18 AGAN & STARR, BAN THE BOX , CRIMINAL RECORDS, AND STATISTICAL DISCRIMINATION center fixed effects. They are variants on the Table 2, Column 2 main effects regression, the “white” coefficient of which is reproduced in Column 1 of Table 3 for comparison purposes. In Column 2, we use interview request as the dependent variable rather than callback, which identifies observations in which a voicemail or email specifically mentioned an interview. Although the effect appears superficially smaller (1.4 percentage points), it is actually very slightly larger as a percentage of the (lower) black baseline rate: whites receive 24% more messages specifically mentioning interviews than blacks do (and 23% more callbacks). In Column 3, we alter the company fixed effect. The main specification grouped chains with fewer than 3 locations (or 12 observations) according to business type (such as fast food restaurants or clothing stores). Column 3 shows that the estimate is robust to using an ungrouped company fixed effect. In Columns 4 and 5, we show the race effect separately estimated for the New Jersey and New York City subsamples, respectively. Here we see a dramatic difference: the “white” effect is far larger in New Jersey (4.5 percentage points versus 0.7 percentage points), and is statistically insignificant in New York City. The overall callback rate is considerably higher in New Jersey (14.7% compared to 9.4%), but not nearly enough so to explain this difference: in New York City, whites receive about 8% more callbacks than equivalent black applicants, while in New Jersey they receive about 37% more. In the Appendix A3 and A4, we reproduce in full Table 2 and the other main tables and figures for New Jersey and New York separately, and we discuss the geographic differences further below. In Table 3B, we show an analogous of alternative analyses of the main effect of having a criminal record within the box sample, paralleling the estimate from Table 2, Column 4, which is reproduced in Column 1 of Table 3B. As with the “white” effect, the criminal record effect appears smaller in percentage-point terms when interview request is used as the outcome (Table 3B, Col. 2), but this effect is actually larger in relative terms. Applicants without records receive 67% more messages specifically mentioning interviews, and 61% more callbacks overall. Column 3 shows that the effect estimate is essentially unchanged by substituting the ungrouped company fixed effects. Finally, Columns 4 and 5 show that the criminal record effect is just slightly larger in percentage-point terms in New York City than in New Jersey—but in light of the city’s lower callback rate, it is much larger in relative terms. Applicants without records receive 45% more callbacks than those with records in New Jersey; in New York City, applicants without records receive 78% more callbacks. 19 AGAN & STARR, BAN THE BOX , CRIMINAL RECORDS, AND STATISTICAL DISCRIMINATION Note that clustering in all regressions is on the chain, for reasons discussed further in Section 5.5 below. Standard errors on the race and criminal record effect estimates are not substantially affected by clustering on the store or the geographic center instead (p<0.001 in all specifications). 4.4 Further Investigation of Geographic Differences in Callback Rates by Race The difference in the White effect between the New Jersey and New York City subsamples, shown in Table 3A, is quite striking, and motivates further analysis. One plausible explanation is that New York City is more racially diverse than New Jersey. Per Census data, it has a larger black population share (22%, vs. 15% in New Jersey), a smaller non-Hispanic white population share (32%, vs. 57% in New Jersey), and larger populations of other ethnicities, especially Hispanic (29%, vs. 19% in New Jersey) and Asian (14%, vs. 9% in New Jersey). New Jersey is itself a fairly diverse state, and its racial composition far more closely tracks the country as a whole, so if racial composition explains the differences in observed disparities, the New Jersey results might be more representative of broader patterns. In Table 4, we directly test whether local racial composition at a more localized level—the census block group of the business address23—influences the White effect, and whether this in turn can explain the different patterns in New York City and New Jersey. The racial composition of the neighborhood population could potentially influence employer racial discrimination in various ways. Employers could seek to appeal to local customers’ own-group preference, or perhaps to pick applicants who “fit in” based on the racial composition of current staff. Hiring managers could themselves be of different races in different neighborhoods, and this might influence their perceptions of applicants. We lack data on managers’ or staff members’ race, so we cannot differentiate these mechanisms, but we can test their cumulative effect. The regressions in Table 4 add various interactions to the main-effects regression. The center fixed effects are omitted because other geographic variables are included instead. The other variables from Table 2, Column 2 are all included in the regressions, although only the coefficients on the White variable, the geographic variables, and their interactions are shown in the table. Before incorporating the racial composition data, Column 1 of Table 4 first shows that the White x 23 When job postings were not specific to a location with an identifiable address, we used the averages for the city or town instead in New Jersey or the zip code or borough (depending on the detail given in the posting) if in New York City. 20 AGAN & STARR, BAN THE BOX , CRIMINAL RECORDS, AND STATISTICAL DISCRIMINATION NJ interaction is significant (p<0.001) and large (3.8 percentage points), while the estimated White effect in New York is only an insignificant 0.7 percentage points, consistent with the split-sample results above. It also shows that overall callback rates for New Jersey, as noted above, were higher. Column 2 drops the NJ variables, and instead includes the non-Hispanic white population share of the census block group where the store is located, and interacts that share with White. (The racial composition variables are labeled Store CBG %White and Store CBG %Black in the tables to reflect this precise definition, but for simplicity in this text, we refer to them as PercentWhite and PercentBlack.) The interaction effect is very strong, indicating that employers in whiter neighborhoods are much more likely to discriminate based on race. Its coefficient (4.9 percentage points, p<0.01) represents the increased advantage of white applicants when one goes from an entirely nonwhite neighborhood to an entirely white neighborhood (both of which are found in our sample). The true effect, of course, may be nonlinear. Note that white neighborhoods have higher callback rates as well: the main effect of PercentWhite is 3.4 percentage points (p<0.01). Column 3 shows an analogous analysis of the effects of the black population share (PercentBlack). Its interaction with White is even larger (6 percent, p<0.001). This regression suggests that in entirely nonblack neighborhoods the White effect is large and positive (3.2 percentage points, p<0.001), while in entirely black neighborhoods, the White effect is about the same size but negative (about -2.8 percentage points). Of course, these effects do not in practice offset one another in the overall employment market, because (given the lower black population share), there are many more white (and nonblack) neighborhoods than there are black neighborhoods. The median employer neighborhood in our sample is 5% black, and only 8% of employer neighborhoods are more than half black. In Columns 4 and 5, we add back the NJ and White x NJ terms to the regressions from Columns 2 and 3 respectively, to assess whether racial composition differences can explain the White x NJ interaction. For the most part, they do not—and nor does the NJ effect explain away the racial composition effect. In each of the combined regressions, the White x NJ interaction is almost as large as it was in Column 1 (3.3 and 3.6 percentage points, respectively. In Column 4, the PercentWhite x White interaction is 3.3 percentage points, and in Column 5 the PercentBlack x White interaction is -4.9 percentage points. Column 6 shows that the White x NJ interaction persists when both sets of racial composition interactions are added to the regression. It appears that the PercentWhite*White interaction disappears--however, because PercentBlack and PercentWhite are 21 AGAN & STARR, BAN THE BOX , CRIMINAL RECORDS, AND STATISTICAL DISCRIMINATION strongly collinear, the distinct effect of each (and their interactions) may be difficult to estimate meaningfully when both are included. The effect of local racial composition on racial discrimination patterns is important in its own right, and has not been investigated by prior auditing studies. It suggests that at least one of the mechanisms described above is at play—all forms of own-group preference. Still, it does not appear to explain most of the difference between New York and New Jersey. This is likely because, as it turns out, the racial compositions of employer neighborhoods in our New Jersey and New York samples are much less different from one another than one might have expected based on the jurisdictions’ overall demographics. For example, the median percent black for both jurisdictions is 5% (far lower than either jurisdiction’s black population share) although the mean differs (16% for New York, 11% for New Jersey). Employers in both jurisdictions, especially New York City, appear to be very disproportionately concentrated in whiter (and less black) neighborhoods. Note that we test these effects only at the census block group level, but the city’s overall greater diversity might nonetheless influence racial discrimination patterns, even if employers are not located in especially diverse neighborhoods—for example, existing staff and managers need not be drawn from the immediate neighborhood. 5. Effects of Ban-the-Box on Racial Discrimination In this section we turn to our policy-effects analysis: what is the causal effect of BTB on racial discrimination in employer callbacks? In order to answer this question we combine our field experiment with a difference-in-difference-in-differences strategy. This strategy exploits the two sources of variation in employer knowledge about criminal records before the callback: crosssectional variation in the pre-period between applications with the box and those without, and timeseries variation caused by the law change which required companies that asked about criminal records to stop doing so. 5.1 Difference-in-Difference-in-Differences Estimation Strategy One problem with comparing callback rates in two different time periods is that seasonal variation, other state- or city-level policy changes, and general economic trends could all effect callback rates in different periods, differences unrelated to the BTB policy itself. To account for this possibility, we employ a difference-in-differences-in-differences approach. This method exploits the fact that not all employers ask about criminal records even in the pre-BTB period 22 AGAN & STARR, BAN THE BOX , CRIMINAL RECORDS, AND STATISTICAL DISCRIMINATION (indeed, the majority do not). We treat such stores as a control group, comparing whether changes in the effects of race after BTB goes into effect differ between stores that have the box in the preperiod and those that do not. This will “difference out” effects of seasonal variation or other temporal differences unrelated to BTB, leaving us with an estimate of the causal effect of the BTB policy on employer callback difference by race or other characteristics of interest. Similarly, purely cross-sectional comparisons between employers with and without the box could be confounded by unobserved differences between those employers unrelated to the presence of the box. But the triple-differences analysis will difference out those unrelated differences as well, so long as they are time-invariant over the period in question. This method implies the following general difference-in-difference-in-differences estimating equation: 𝑐𝑎𝑙𝑙𝑏𝑎𝑐𝑘 = 𝛼 + 𝛽! 𝑊ℎ𝑖𝑡𝑒 + 𝛽! 𝑃𝑜𝑠𝑡 + 𝛽! 𝑇𝑟𝑒𝑎𝑡𝑒𝑑 + 𝛽! 𝑊ℎ𝑖𝑡𝑒 𝑥 𝑃𝑜𝑠𝑡 + 𝛽! 𝑊ℎ𝑖𝑡𝑒 𝑥 𝑇𝑟𝑒𝑎𝑡𝑒𝑑 + 𝛽! 𝑃𝑜𝑠𝑡 𝑥 𝑇𝑟𝑒𝑎𝑡𝑒𝑑 (1) + 𝛽! 𝑇𝑟𝑒𝑎𝑡𝑒𝑑 𝑥 𝑊ℎ𝑖𝑡𝑒 𝑥 𝑃𝑜𝑠𝑡 + 𝜖 𝑃𝑜𝑠𝑡 is an indicator for the post-BTB period, 𝑐𝑎𝑙𝑙𝑏𝑎𝑐𝑘 is an indicator for whether the applicant received a positive-response callback from the employer, 𝑇𝑟𝑒𝑎𝑡𝑒𝑑 is an indicator for whether the criminal record question on the store’s job application form changed after BTB. Treated is coded at the individual store level. Observations from a given store are coded as not treated (𝑇𝑟𝑒𝑎𝑡𝑒𝑑 = 0) if the store never had “the box,” and also in the rarer case of stores that had the box and failed to remove it after BTB. Observations are coded as treated if the store had the box but removed it after BTB.24 In most specifications, we also add a vector of control variables that accounts for the possibility of random imbalances in other applicant or application characteristics (GED, employment gap, criminal record, and geographic center). In Equation (1) above, the main effect of interest is the triple-difference coefficient, 𝛽! , which tells us how the employer callback gap for whites versus blacks changes differentially after BTB for treated versus non-treated stores. A positive coefficient implies that BTB favors white 24 The sample used for this analysis is slightly smaller than the sample for the main-effects analysis above because we dropped a small number of observations—about 1% of the sample—for which Treated could not be coded as either 0 or 1, because the chain moved from not having the box to having it after BTB (the opposite of the expected direction of change, seemingly due to administrative mistakes). 23 AGAN & STARR, BAN THE BOX , CRIMINAL RECORDS, AND STATISTICAL DISCRIMINATION applicants relative to black applicants, that is, that treated employers become relatively more likely to call back white applicants after the box is removed. An additional issue is that we did not apply to exactly the same set of stores or chains in the pre- and post-period—as discussed above, it was not always possible to send all four intended applications to each store. If the employers that we applied to in the post-period happened to have different patterns of discrimination from those in the pre-period (in a way that differed across treated and untreated employers), we could mistakenly interpret a compositional effect as an effect of BTB. We have two approaches for addressing these compositional differences across periods. First, in some specifications, we substitute interacted chain fixed effects instead of some of the “Treated” terms in the equation above, as follows: ! 𝑐𝑎𝑙𝑙𝑏𝑎𝑐𝑘 = 𝛼 + 𝛽! 𝑊ℎ𝑖𝑡𝑒 + 𝛽! 𝑃𝑜𝑠𝑡 + 𝛽!! 𝐶ℎ𝑎𝑖𝑛! + 𝛽! 𝑊ℎ𝑖𝑡𝑒 𝑥 𝑃𝑜𝑠𝑡 !!! ! + 𝑊ℎ𝑖𝑡𝑒 𝑥 ! 𝛽!! 𝐶ℎ𝑎𝑖𝑛! + 𝑃𝑜𝑠𝑡 𝑥 !!! (2) 𝛽!! 𝐶ℎ𝑎𝑖𝑛! !!! + 𝛽! 𝑇𝑟𝑒𝑎𝑡𝑒𝑑 𝑥 𝑊ℎ𝑖𝑡𝑒 𝑥 𝑃𝑜𝑠𝑡 + 𝜖 where 𝑖 indexes chains, and 𝐶ℎ𝑎𝑖𝑛! represents a series of dummy variables for the chains in our sample.25 Because “treated” status occasionally varies between stores (usually because some chains give franchisees a choice of application platforms, or because a chain’s BTB compliance differed between New Jersey and New York City), we assign separate 𝐶ℎ𝑎𝑖𝑛 fixed effects to treated and untreated subsets of such chains. The result is that the 𝐶ℎ𝑎𝑖𝑛 fixed effects perfectly parallel the Treated variable: Treated status follows directly from the 𝐶ℎ𝑎𝑖𝑛. The equation above substitutes the main effect of 𝑇𝑟𝑒𝑎𝑡𝑒𝑑 with 𝐶ℎ𝑎𝑖𝑛 fixed effects, and likewise substitutes 𝑇𝑟𝑒𝑎𝑡𝑒𝑑 𝑥 𝑊ℎ𝑖𝑡𝑒 𝑥 𝑃𝑜𝑠𝑡 with parallel sets of interacted fixed effects. However, it keeps the main effect of interest, the triple-differences estimate, in its easier-to-interpret form of 𝑇𝑟𝑒𝑎𝑡𝑒𝑑 𝑥 𝑊ℎ𝑖𝑡𝑒 𝑥 𝑃𝑜𝑠𝑡. This term represents the average change in racial disparity due to BTB: in effect, a weighted average of what the coefficients would be if 𝑊ℎ𝑖𝑡𝑒 and 𝑃𝑜𝑠𝑡 were instead triply interacted with 𝐶ℎ𝑎𝑖𝑛, completing the substitution. 25 The smallest chains (fewer than three locations or 12 total observations) are combined into industry-category groups; these chains represent about 9% of the sample. Original coding is used in a robustness check below. 24 AGAN & STARR, BAN THE BOX , CRIMINAL RECORDS, AND STATISTICAL DISCRIMINATION The chain-fixed-effects specifications account for differences in composition across periods by chain, but not by individual store (or by the geographic distribution of stores). Moreover, they do not provide easy-to-interpret coefficients on the main effects of White, Treated, and Post or their two-way interactions. We thus also offer a simpler approach for confronting the compositional differences: we conduct the analysis within the subset of stores to which we did send exactly four applications: one white/black pair in each period. Fortunately, we were usually able to do so, and so this “perfect quad” sample contains 11,118 observations, or 76% of our full sample. When using the perfect quad sample, the concerns about different distributions across chains, stores, or jurisdictions disappear (and no controls for these variables are necessary), because the sample is perfectly balanced between the pre- and post-periods. The simple triple-differences analysis can thus be used, and all the coefficients are easy to interpret; the disadvantage is some loss of power. In any of these analyses, identification of 𝛽! as a causal effect relies on the assumption that, absent BTB, trends in employer callback differences by race would have been the same for treated and untreated stores (stores that had the box in the pre-period and those that did not). Unfortunately, our data are not long enough to compare pre-period trends. However, we believe the assumption is plausible. For a vast majority of stores in our sample (even those that are franchised), the job applications are standardized nationally at the chain level, with built-in variations accommodating local differences in BTB laws.26 Thus, the decision to include or not include the box on the application is made at the chain level, whereas callback decisions are made at the individual store level by store managers, or in some chains by local managers who supervise a small subset of locations. In that sense, whether a store has the box should be exogenous to the decision-makers we are studying. Moreover, there is no qualitative reason to believe that these chains differ in any way that would affect hiring trends in a racially disparate way. After all, to pose a threat to identification, hiring differences would have to be racially disparate in a way that differs over the time between our pre- and post-period applications (about four months on average). Note that not having the box does not generally reflect lack of interest in criminal records; chains with and 26 To comply with BTB laws, applications that normally have the “box” will usually ask a question similar to “Are you applying in Rhode Island, Hawaii, Massachusetts, California, or Minnesota?” If one clicks “yes,” the criminal conviction question will not appear. Alternatively, the conviction question will be preceded by instructions telling the applicant not to answer if applying in certain jurisdictions. So the treatment we are studying generally takes the form of the national chain adding New Jersey or New York City to these lists of BTB jurisdictions on the applications. 25 AGAN & STARR, BAN THE BOX , CRIMINAL RECORDS, AND STATISTICAL DISCRIMINATION without the box, before and after BTB, routinely do back-end background checks (and their applications usually warn applicants of this fact). 5.2 Temporal Differences in Racial Disparity at Treated Stores We start descriptively with Figure 2, which compares pre- and post-BTB call back rates among treated employers—that is, those that had the box in the pre-period but then removed it to comply with BTB.27 Just as with Figure 1 (the cross-sectional comparison), Figure 2 (the temporal comparison) suggests that when companies don’t see applicants’ criminal records, they are more likely to discriminate based on race. In this sample, in the pre-period, white applicants both with and without records have a slightly higher callback rate than equivalent black applicants do: for applicants without records, the white and black rates are 13.8% and 12.7% respectively, and for those with records, the white and black rates are 8.8% and 8.4%, respectively (Figure 2). Averaging these subgroups together, the overall pre-period callback rates in this sample were 11.3% for whites and 10.5% for blacks. However, in the post-period, this quintuples in size, and white applicants receive 36% more callbacks than blacks do: the white callback rate is 15.0%, and the black callback rate is 11%. This figure does not, however, take into account potential seasonal or temporal variation between the pre- and post-period. The difference-in-difference-in-differences results below will “difference out” temporal variation in racial discrimination among employers whose applications never had the box and thus were unaffected by BTB, as discussed above. As we will see, this differencing out only strengthens the implication that BTB encourages racial discrimination. 5.3 Differences-in-Differences-in-Differences: Raw Percentages Before showing regression estimates, we start with raw percentage differences. Table 5 summarizes the changes in callback rates by race for treated and untreated stores before and after BTB went into effect. Each cell in Table 5 is itself a difference: the callback rate for black applicants minus the callback rate for white applicants. The “treated” column replicates what we already saw in Figure 2: at treated stores, the “white” advantage grew by 3.2 percentage points (from 0.7 percentage point to 4.0 percentage points) after BTB. The “not treated” column shows what happened at the same time at other stores whose applications were unaffected by BTB (mostly 27 The figure looks very similar if done only within the “perfect quad” sample. 26 AGAN & STARR, BAN THE BOX , CRIMINAL RECORDS, AND STATISTICAL DISCRIMINATION because they did not have the box to begin with). At these stores, the “white” advantage declined very slightly, from 2.7 percentage points to 2.2 percentage points. When we further difference out the temporal differences in racial differences at untreated stores, we get a difference-in-differences-in differences figure of 3.7 percentage points. That is, the black-white gap grew by 3.7 percentage points more at the treated stores after BTB, relative to the untreated stores. This is a large increase, given that baseline callback rates are low; the average callback rate for black applicants in this sample is 10%. Below the line, we show a the tripledifferences calculation for treated and untreated observations in the perfect quad sample which is balanced on the chains and stores we applied to in the pre- and post-period 4.2 percentage points, a similarly large effect. 5.4 Triple-Differences Regressions Table 6 shows regression-adjusted triple-differences estimates across several specifications and samples. The effect of principal interest is on the top line, Post x Treated x White. Across specification, the estimates are economically large and significant (ranging from 3.6 to 4.1 percentage points, which amounts to a multifold increase in the underlying race gap). Our estimates here are somewhat less precise than the main-effects estimates discussed in Section 4, because triple-differences analyses demand much larger samples than analyses of main effects or even twoway interactions do in order to provide equivalent statistical power to estimate effects of a given size. Even so, all of these estimates are statistically significant (p<0.05), with p-values generally around 0.04. All of our regression estimates are quite similar to the basic difference-in-differencein-differences analysis in Table 5, which is unsurprising given that the applicant characteristics are randomized. Columns 1 and 2 show the simple triple-differences regression with the Treated, Post, and White variables interacted (per Equation 1), with and without controls for the other randomized applicant characteristics (GED, employment gap, and criminal record) as well as center fixed effects. Adding these controls increases the triple-differences coefficient slightly, from 3.7 to 4.1 percentage points. This analysis does not, however, account for the above-discussed differences in composition of the sample across time periods. We begin to address these in Column 3, which parallels Column 2 but substitutes interacted chain fixed effects for the Treated variable and its twoway interactions (per Equation 2). This analysis accounts for differences in the representation of the various chains in the pre- and post-period, and the main effect of interest declines slightly, to 3.6 27 AGAN & STARR, BAN THE BOX , CRIMINAL RECORDS, AND STATISTICAL DISCRIMINATION percentage points. It bears noting that the White, Post, and Post*White estimates do not have a meaningful interpretation in this regression because the total effects of those variables are diffused among the interacted fixed effects. Column 4 then further account for differences in the individual stores represented in the preand post-period samples by limiting the analysis to the “perfect quad” sample. In this sample chains and centers are perfectly balanced across time periods and race, so there is no reason to include the chain or center fixed effects. Accordingly, we can use the simple triple differences specification, retaining from Column 2 only the controls for GED status, criminal record, and employment gap, since these might have randomly been slightly imbalanced even among the “perfect quads.” The effect estimate remains similar: 4 percentage points. In this sample, the estimated race gap at the treated stores goes from 0.7 percentage points before BTB to 4.7 points after, after differencing out changes at untreated stores. Again, to put this estimate in perspective, one must compare it to the baseline callback rate: other things equal, whites receive 6.7% more callbacks than similar black candidates do when employers are able to observe criminal records, but they receive about 45.2% more callbacks than similar black candidates when employers cannot observe records. In short, these analyses provide evidence that BTB increases racial discrimination in employer callbacks. Prior to the adoption of BTB, racial disparities are somewhat larger among the stores that do not have the box. After BTB, that difference flips. The growth in the “white” effect after BTB appears to multiply the race gap at affected stores by a factor of between five and seven; this factor varies slightly across specifications and samples, mainly because of variations in the small estimated pre-BTB race gap. In Appendix A5, we recreate the above analysis substituting GED or employment gap for White to explore whether employer responses to these characteristics, which are also correlated with a criminal record, change after BTB. The triple differences coefficients for both GED and employment gap are not significant. For the employment gap, however, the point estimates are nontrivial (around 2.5 percentage points; Table A5.2), albeit imprecise, and their signs go in the anticipated direction that statistical discrimination theory would predict: the negative effect of the employment gap increases when employers lose criminal record information. In the GED analysis, the point estimates are also negative but smaller, and very close to zero in the full-sample fixedeffect analysis (Table A5.1, Col. 3). So we cannot characterize this as even suggestive evidence of statistical discrimination on the basis of the GED. 28 AGAN & STARR, BAN THE BOX , CRIMINAL RECORDS, AND STATISTICAL DISCRIMINATION 5.5 Alternative Specifications and Samples: Effects of BTB Our results are quite robust to alternate specifications. Table 7, Panels A and B, shows robustness checks and alternative samples corresponding to our estimates for the full sample and the “perfect quad” sample respectively. Only the triple-differences coefficient is shown. We base these variations on what we consider the main specifications for each sample, which are found in Columns 3 and 4 of Table 6. For the full sample, because of our concern about compositional differences between periods, we prefer the specification that includes the interacted chain fixed effects, and use that as the basis for the robustness checks. The triple-differences coefficient from Table 6, Column 3 is accordingly reproduced in Column 1 of Table 7A for comparison purposes. Meanwhile, the robustness checks for the “perfect quad” sample are based on the Table 5, Column 4 specification, and its triple-differences coefficient is reproduced in Column 1 of Table 7B. Columns 1 through 6 of both panels parallel one another, while Columns 7 and 8 of Panel A show additional checks that are not relevant to the “perfect quad” sample. Note at the outset that the coefficients and p-values are fairly similar for all variants except for columns 5 and 6 of each panel, which show results for New Jersey and New York City separately and are much less precise. In a few of the other specifications the p-values are above 0.05, but barely, representing only a small loss of precision or slightly reduced effect size; all pvalues are between 0.04 and 0.06, other than in the NJ-only and NYC-only regressions. Column 2 in both panels replaces the callback outcome variable with the interview variable. In percentage point terms, the estimate becomes slightly smaller (but still significant) in the full sample, and is essentially unchanged in the “perfect quad” sample. Again, however, the recorded “interview” rate was much lower (6.3% overall in the full sample, versus a “callback” rate of 11.7%)—so the effect on “interview” rates was actually quite a bit more dramatic in relative terms. That said, because we suspect that the vast majority of callbacks were in fact seeking interviews (even if they did not specifically say so), we consider the callback variable the better measure. Columns 3 and 4 in both panels alter subjective choices that we made about whether to exclude certain problematic observations. In Column 3, we add back in a group that we excluded from the main triple-differences analyses: “reverse complier” stores that had no box before BTB, but mysteriously (apparently due to administrative mistakes) added it after BTB. “Treated” cannot 29 AGAN & STARR, BAN THE BOX , CRIMINAL RECORDS, AND STATISTICAL DISCRIMINATION be coded as 0 or 1 for these observations, but here we code it as -1, reflecting the reversal of the usual treatment direction.28 The effect size is slightly smaller in both samples and the p-value is slightly above 0.05 in the perfect-quad specification. In Column 4, we exclude a small number of observations or quads (about 0.4% of each sample) in which an RA made a mistake and answered a “box” question that she was not required to answer, or vice versa.29 Excluding them leaves both samples’ estimates virtually unchanged, though the perfect quad sample p-value again rises slightly above 0.05. Columns 5 and 6 in both panels divide the sample between New Jersey and New York City, respectively. The large reduction in sample size renders these analyses underpowered for the purpose of estimating triple differences, and thus these estimates are quite imprecise. The New Jersey point estimate is larger in percentage-point terms, but not much so in relative terms, once one accounts for New Jersey’s substantially higher callback rate (14.9% in the full sample, versus 9.4% in New York City). As a proportion of the respective samples’ callback rates, New Jersey’s fullsample point estimate is only slightly higher than New York’s, and New Jersey’s “perfect quad” point estimate is slightly lower than New York’s. In any event, because of their imprecision, one ought not to give much interpretive weight to the jurisdictional differences in the point estimates (whereas the jurisdictional differences in the main effects of race, discussed above, are clear). In Panel A, Columns 7 and 8 show two additional variants on the full sample analysis that alter the chain fixed effects and their interactions. In the main sample, the smallest chains (with under 12 observations total, or three stores) had been grouped based on business-type category (such as fast food restaurants or clothing stores). Column 7 instead uses individual chain fixed effects regardless of company size. Column 8, meanwhile, divides the chain fixed effects into New York and New Jersey subsets of each chain. Both changes add a large number of fixed-effect indicators to each regression and reduce precision slightly, but the point estimates remain similar. Clustering in all regressions shown in the tables is on the chain, because whole chains are likely susceptible to serially correlated shocks. We observed quite different callback rates by chain, 28 Note that the relationship between treatment and the passage of time is inverted for these observations, making this specification diverge from a standard triple-differences analysis. This is the main reason we excluded them. 29 The main sample had already dropped RA-error cases when they created inconsistencies in the coding of the treatment variable within stores, but kept them when the same error was made consistently within the store; we then coded the treatment variable according to how the RA interpreted the application, since that tracked the information about criminal records that the RA provided or did not provide to the employer. 30 AGAN & STARR, BAN THE BOX , CRIMINAL RECORDS, AND STATISTICAL DISCRIMINATION as well as some chains that had distinct increases or reductions in callback rates or in job-posting availability in one or more of the four time periods in which we sent applications. The chain also encompasses the smaller units according to which the applications we sent were grouped. That is, we sometimes sent the same set of four applications to multiple locations of the same chain (especially in New Jersey, where we did so for all locations within the same center), but never to different chains. If one clusters on the geographic center instead (another dimension along which one could anticipate possible correlated shocks), the p-values for our main specifications are slightly higher in the full sample (0.054) and slightly lower in the perfect quad sample (0.024), and if one clusters on the individual store (ignoring correlations between chains), they are slightly higher in both samples (0.05 and 0.06, respectively). 6. Discussion and Conclusion Our results support BTB’s basic premise: when employers ask about them, criminal records pose an obstacle to employment. However, our findings also provide evidence of a serious apparent unintended consequence of BTB: increased racial discrimination against black men. These findings suggest a difficult dilemma for policymakers. Here, we discuss their limitations and implications further, as well as those of our results on the main effects of race. 6.1 BTB and the Effect of Criminal Records The key premise of BTB is that when employers ask about criminal records, people with records will have a much harder time getting their foot in the door. Although this seems intuitive, it can be difficult to quantify with observational research—but our field experiment provides very clear evidence of the serious obstacle to employment that criminal records pose. Applicants without records received 61% more callbacks than identical applicants without records did when employers had the box. And this is despite two facts that may have mitigated this effect. First, our applicants with records had minor records (a single conviction of a nonviolent drug or property crime, more than two years prior, with no incarceration history). Second, we applied mainly to positions that one might expect, in general, to be comparatively welcoming to people with records—for example, crew member jobs in restaurants. The practical effect of the criminal-record penalty might be offset to some degree by the fact that most employers in the sectors we studied do not have the criminal-records box even absent BTB. However, even when employers do not have the box on their applications, they are free 31 AGAN & STARR, BAN THE BOX , CRIMINAL RECORDS, AND STATISTICAL DISCRIMINATION (absent BTB) to ask about records at an interview and to check records at any time; even with BTB, they are free to do so later in the application process. So if employers disfavor people with records, this effect may be present to some degree at later stages of the process even among non-BTB employers—stages our study does not assess. For BTB’s advocates, the good news in our findings is that employers comply with it, and thus BTB effectively eliminates criminal-record effects on employer callback rates for identical applicants. Fewer than 5% of employers retained the box in the post-period, a few months after BTB’s effective date. This means that for our applicants with records, BTB worked: those records were never conveyed to employers before the callback decision was made. Note, however, that we were unable to study the effect of BTB (or of criminal record or race) on actually getting a job, only initial employer responses. Perhaps BTB might not change employment rates after all, if firms are reluctant to hire applicants with a record even after they “get their foot in the door” (for a similar point on discrimination against the long-term unemployed, see Jarosch and Pilossoph (2015)). Still, while this is a substantial limitation, BTB is meant precisely to impact the initial stage of the hiring process, and so it is an important question whether doors do, indeed, open—and whether BTB brings about unintended consequences at the same initial stage. 6.2 Main Effects of Race Our results also confirm a clear advantage of white applicants, who receive 23% more callbacks compared to otherwise identical black applicants. This finding is consistent with those of nearly all prior auditing studies, so it should not surprise readers, although it is useful to confirm it in a newer sample and a setting (online job applications) which has hardly been studied but is central to the modern job market. Our estimate of white applicants’ advantage is somewhat less dramatic than most prior auditing studies have found, but as with the criminal record, our setting is one in which lesser race effects might have been expected. Online applications involve no personal interactions (and indeed may be initially narrowed down by software before a hiring manager ever sees them), and our applications gave no racial signals other than the name. Moreover, the job categories to which we applied are ones in which young black men are relatively well represented; one might expect black applicants to face lesser hurdles there than in fields where they would be a smaller minority. 32 AGAN & STARR, BAN THE BOX , CRIMINAL RECORDS, AND STATISTICAL DISCRIMINATION This apparent racial discrimination could reflect a number of specific mechanisms: (1) statistical discrimination based on expectations concerning criminality (for companies that do not have the box, or in the post-BTB period); (2) statistical discrimination based on expectations concerning other productivity-related factors; (3) attempts to appeal to the discriminatory tastes of a customer base; and (4) pure taste-based discrimination unrelated to job performance expectations. A critique of auditing studies has been that they usually do not allow researchers to distinguish taste-based and statistical mechanisms of discrimination (Neumark 2011; Heckman and Siegelman 1993). Our research design offers some traction on this question, in that it helps to disentangle the first mechanism from the others, but we cannot disentangle the other three mechanisms. However, all four of these mechanisms amount to illegal racial discrimination, and all four conflict with the policy objective of expanding black male unemployment. Regardless of the specific causal pathway, then, our findings should be troubling to many policymakers, and are a reminder of the very substantial persistence of racial discrimination in hiring despite its legal prohibition. Given the prior literature, one surprise in our analysis is that the main effect of race does not pervade all segments of our sample. The advantage of white applicants is quite small when employers have the box, and it is quite small overall in New York City. Among employers with the box in New York City, the black callback rate was actually higher (10.2% versus 8.4% for whites, though this difference is not statistically significant). Moreover, our findings demonstrating a strong interaction of applicant race and neighborhood racial composition also indicate that racial discrimination is less prevalent (or may even be reversed in direction) in neighborhoods that are less white—although it also suggests larger degrees of racial discrimination in whiter neighborhoods. All of this variation suggests that racial discrimination in hiring, while prevalent, is not ubiquitous and may be avoidable—although we cannot yet fully explain why New York City is more successful than New Jersey in avoiding it, as demographic differences do not entirely explain the difference. 6.3. Effects of BTB on Racial Discrimination BTB appears to substantially increase racial discrimination against black men—indeed, by more than a factor of six in our main specifications. At BTB-affected employers, white applicants went from being 7% more likely to receive a callback than similar black applicants to being 45% more likely. This consequence is clearly unintended, as BTB is often presented as a strategy for increasing access to employment for black men. 33 AGAN & STARR, BAN THE BOX , CRIMINAL RECORDS, AND STATISTICAL DISCRIMINATION We believe that the randomized experimental design, in combination with the tripledifferences analysis, provides a strong basis for interpreting our estimates as causal effects of BTB. The randomization means that we avoid most of the potential interpretive challenges that observational researchers encounter: our black and white applicants to all business types in all locations and periods have the same qualifications and characteristics. Any remaining threats to identification would have to come from unobserved differences that (1) affect applicants to treated and untreated businesses differently (2) in ways that differ by race and (3) this difference must differ across time periods as well. Although it is of course possible that (independent of BTB) some such difference might exist, there is no obvious candidate for what it might be. This is especially so because the time period between the pre- and post-periods is short—the two groups of quite similar businesses are unlikely to have greatly diverged from one another in their racial discrimination patterns in just a few months—and because we see approximately the same triple-differences effect in New Jersey and New York City, even though the pre- and post-periods in those two jurisdictions were seasonally nearly opposite to one another.30 We note that there are at least two plausible mechanisms that would explain this result. The first is statistical discrimination against black men: although black men with records could be helped by BTB, this effect could be swamped by negative effects for black men without records because absent the information employers treat them as if they have a high probability of having a record (Finlay (2014) concluded similarly in his research about the availability of online criminal records). Indeed, given that we gave our applicants fairly minor criminal records, it is even possible that some of our black candidates with records would have been better off revealing them (so that a more serious record was not assumed). A second mechanism focuses on BTB’s benefits for white applicants. Perhaps for some subset of employers, either black race or a criminal record are enough to push marginal candidates out of consideration. Such employers would be expected to treat white applicants with records more favorably after BTB, but their treatment of black applicants with records would not change, because black applicants without records already were not getting callbacks. The mechanism for these employers’ racial discrimination need not primarily relate to expectations about criminal records—it could be based on the other reasons identified above: pure prejudice with no statistical 30 In New Jersey we went from winter to late spring/early summer; in New York we went from summer to winter. 34 AGAN & STARR, BAN THE BOX , CRIMINAL RECORDS, AND STATISTICAL DISCRIMINATION basis, appeals to a discriminatory customer base, or perhaps statistical discrimination on the basis of some other factor besides criminal record. This theory suggests that BTB could allow white applicants with records, in essence, to take advantage of the racial advantage that other white candidates have. It is a statistical discrimination theory as well, insofar as it requires employers to assume that white applicants likely do not have criminal records. But it suggests a more complicated story, implying that other mechanisms of discrimination may also play a role. These mechanisms are not mutually exclusive, and our results suggest that both likely contribute. At BTB-affected employers, after differencing out trends at unaffected employers, black applicants see their callback rates fall by two percentage points after BTB, while white applicants see theirs rise by two percentage points. These estimates are suggestive that both mechanisms are at work, although we lack the statistical power to disentangle them completely. (To truly tease out these pathways, we would need to add a fourth difference to our triple-differences analysis—that is, whether applicants have a record—which would require an enormous sample to do precisely.) And in any event, regardless of which explanation primarily drives our result, both suggest that BTB may not do the job that many of its advocates are hoping it will do: expanding access to employment for black men. One alternative causal theory is that BTB might affect treated businesses’ applicant pools, by encouraging more applicants with records to apply. If this is so, then even though our fictional applicants are the same in both periods, their competition is not, potentially affecting callback rates. But to explain our triple-differences estimates, changes in the competition have to affect our black and white applicants differently—and it is not obvious why this would be the case. If the mechanism involves statistical discrimination based on assumptions about records, then it is simply a variant on the theories we have already proposed. Indeed, whatever employers’ reasoning, if the theory is that BTB causes changes in the applicant pool that somehow cause employers to treat black applicants more adversely than identical whites, then it does not threaten our causal inference that BTB increases racial discrimination—it simply provides another mechanism by which it might do so. A variant of this concern is that BTB might affect untreated businesses’ applicant pools in some way (presumably reducing the number of applicants with records, as they apply to treated businesses instead) that leads them to increase callbacks of black applicants relative to whites. This possibility is more of a threat to causal identification because it would mean the control is not really 35 AGAN & STARR, BAN THE BOX , CRIMINAL RECORDS, AND STATISTICAL DISCRIMINATION untreated. But changes to the untreated employers’ applicant pool are likely to be relatively subtle, because for many (probably most) applicants there is no necessary tradeoff between applying to treated and untreated businesses. In addition, given that the untreated employers lack the box both before and after BTB (and after BTB cannot ask about records even at interviews), it seems that many would be unlikely to notice changes in the percentage of their applicants with records, especially if those changes are not drastic. Employers would have to notice or anticipate such a change, and update their race-specific expectations and decision-making accordingly, very quickly in order to affect our results; our post-period applications were sent an average of less than three months after BTB’s effective date. Moreover, again, the change in competition would have to affect our black and white applicants differently, and it is not clear that it would. Nor is there empirical reason to suspect that it does: the estimates in Table 5 strongly suggest that the tripledifferences effect is being driven by an increase in racial disparity among treated employers, not a reduction among untreated employers. In any event, the effect of BTB on applicant pools (of either set of employer) may well be mitigated if applicants do not know what employers have the box before they are nearly done with the application (the box usually appears as one of the last screens). Some applicants with records might well gain such information before applying, but we suspect that this knowledge is at least not ubiquitous, in part due to the challenges we faced finding it. Despite considerable effort, we were unable to find resources listing employers with and without the box prior to conducting our resource-intensive data collection, and we were ourselves surprised to learn what a large share of employers did not have it. Applicants would also have to know about BTB, as well as its effective date (actual passage of BTB in both jurisdictions came months earlier, before our pre-period). There is a more direct way in which BTB might affect untreated employers, however: we identified employers as untreated based on their job applications, but BTB also governs the interview. So it is possible that it could encourage even untreated employers to statistically discriminate as well: knowing that they cannot ask records questions in the interview might make them less likely to interview candidates that they think might have records. However, if anything this possibility should mean our triple-differences estimate is downward biased, because BTB encourages statistical discrimination at both sets of employers, while we are measuring only the difference. In addition, in New Jersey employers are permitted to do background checks immediately after the interview (and even in New York City, where a conditional job offer must be 36 AGAN & STARR, BAN THE BOX , CRIMINAL RECORDS, AND STATISTICAL DISCRIMINATION made, this could potentially occur in quite short sequence), so this concern for subsequent delay seems relatively minor—it is not a dramatic difference to find out about a record shortly after the interview rather than during it, since the time spent on the interview would already have been invested. We therefore think the best explanation for the triple-differences estimate is that BTB encourages statistical discrimination against black applicants and/or in favor of white applicants. Although such discrimination is illegal and against public policy, one could still be interested in asking: is it rational, in the sense of reflecting accurate expectations by employers about who is likely to have a criminal record? Or are employers relying on inaccurate stereotypes about black criminality? It is difficult to assess the rationality of employer decisions because there is much we do not know: for example, the costs to employers of interviewing an applicant who turns out to have a disqualifying criminal record, and on the other hand the costs of inadvertently failing to interview a candidate (due to assumptions about his record) who would have been the best choice. That said, there is good reason to believe that employers are relying on assumptions that exaggerate real-world racial differences in conviction rates. It is difficult to find useful statistics on the percent of specific populations with felony convictions – the National Longitudinal Survey of Youth 1997 (NLSY97) offers one data source, albeit with a fairly small sample size for this purpose. An initial point is that although absolute black/white differences in felony conviction rates are large (Shannon et al. 2011), they are much smaller once one conditions on other applicant characteristics that employers can observe. Indeed, this is so even once one simply limits the pool to young men with relatively limited education. Our calculations from the NLSY97 show that amongst men between the ages of 18 and 25 without any higher education degrees, 29.4% of black men had a criminal conviction between the ages of 18 and 25, whereas 24.7% of white men did. Our black and white applicants are identical on a range of other characteristics as well—work history, neighborhood, and so forth—which one would expect to narrow the gap further. And yet employers who are provided with a great deal of individualized information about our applicants appear to nonetheless be giving considerable weight to race as a predictor of criminality. One possibility is that employers engage in statistical discrimination in a far less nuanced way than rational-choice economic theory would predict—they may rely on a general impression that black rates of involvement with the criminal justice system are higher in absolute terms, without any specific sense of whether these differences persist after conditioning on the relevant set 37 AGAN & STARR, BAN THE BOX , CRIMINAL RECORDS, AND STATISTICAL DISCRIMINATION of observed characteristics. It would not be surprising if employers made assumptions about black applicants’ likely criminality, even if those assumptions are not well founded in fact. Lab experiments on implicit biases have consistently found that most Americans make such assumptions subconsciously (see, for example, Eberhardt 2004; Nosek et al. 2007), and such mechanisms may not involve an accurate comparison of conditional probabilities. Further support for this theory comes from the contrast with our results on the GED versus high school diploma distinction; we did not find that BTB significantly increased the weight employers placed on that distinction. Nor, indeed, do employers place significant weight on this variable at all, even at non-box stores. And yet having a GED in lieu of a diploma is actually a much stronger predictor of criminal convictions than race is, conditional on the same observables. In the NYLS97, among young men with no college degrees, 43% of those with a GED have a conviction by age 25, whereas only 18% of those with a high school diploma have one. This contrast suggests that whatever employers’ cost-benefit calculus about interviewing people with records, they must either be irrationally overweighting race as a signal, underweighting education, or both. Employers also give no apparent weight (before or after BTB) to year-long employment gaps, despite the possibility that this might be associated with arrest or incarceration (or might otherwise signal that the applicant is a less appealing job prospect). 6.4. Policy Implications BTB may open doors to some applicants with records, but this gain comes at the expense of another group that faces serious employment challenges: black men. BTB is often presented as a way of increasing black male employment, but most black men do not have criminal convictions, and BTB risks harming black men without records by preventing them from signaling that fact to employers. This is a serious unintended consequence, but it is not necessarily dispositive as to BTB’s merits. Policymakers will have to evaluate how to weigh this risk versus BTB’s potential benefits, and also to consider whether there are strategies that could simultaneously be pursued that might successfully mitigate this disadvantage. Even if one simply wishes to evaluate BTB’s race-related effects (setting aside other policy concerns), the picture is somewhat complex. While in our sample BTB’s apparent effect on the race gap was fairly dramatic, an important unanswered question is how large an effect this phenomenon will have on real world job applicants. One limitation of auditing studies generally is that they do not directly provide estimates of changes in actual markets (Heckman 1998). In the real world, 38 AGAN & STARR, BAN THE BOX , CRIMINAL RECORDS, AND STATISTICAL DISCRIMINATION applicants are not divided 50/50 between identical black male and white male candidates (and no other groups), with 50% of each group having a record. Our study suggests that BTB should be expected to substantially help applicants with records, at least at the initial callback stage, and in the real world black men have records at higher rates. This point means that even if BTB increases racial discrimination by employers, it does not necessarily follow that it will increase racial disparity in employment on balance. It could simultaneously be true that BTB helps black men with records (by eliminating record-based discrimination in callbacks), while hurting black men without records (by increasing racial discrimination), and the net effect on black male employment would depend on the size of each effect and the size of the respective groups they affect. And this calculus may vary as BTB is applied to different markets and places—employers’ treatment of both race and criminal records may vary considerably, as our comparisons of New Jersey and New York City illustrate. That said, some back-of-the-envelope calculations suggest that at least in contexts similar to the one we studied, the net effect may be to enlarge the black-white employment gap. Consider again 25-year-old men without college degrees: per the NLSY97, the black and white conviction rates are 29.4% and 24.7%, respectively. Suppose all such men were subject to changes in employer callback rates paralleling the pattern in Figure 2 (the raw pre- to post-period changes at treated employers)—a pattern that actually slightly understates the growth in racial discrimination that our triple-differences regression analyses found. Callback rates increased by 2.6 percentage points for black men with records, and declined by 1.7 percentage points for black men without records. Meanwhile, for white men with records, callback rates increased by 7.2 percentage points, and for white men without records they actually rose also, by 1.2 percentage points. (Callback rates increased at all stores in this period—an effect differenced out in the triple-differences analysis—so this preponderance of gains does not tell us anything about BTB’s effects. The relative rates are the focus of this calculation.) Applying these changes to the real-world distribution of records among young men without college degrees implies that overall black callback rates would fall by 0.4 points, while overall white callback rates would rise by 2.8 points— a net rise of 3.2 percentage points in the black-white callback rate gap (more than a quarter of the overall callback rate for the sample). This example suggests that even after offsetting the effect of eliminating criminal-recordbased discrimination, the increase in racial disparity due to BTB could be considerable. In addition 39 AGAN & STARR, BAN THE BOX , CRIMINAL RECORDS, AND STATISTICAL DISCRIMINATION to the differential effects on white and black applicants without records, part of the reason for this is that it is white applicants with records who appear to benefit more substantially from BTB than black applicants with records do.31 Of course, a full analysis of real world effects would have to account for the fact that white and black men are not the only groups competing for jobs. We chose to focus on white and black men only because further subdividing the sample would have presented challenges in terms of statistical power. But women and men of other racial groups could be affected, and such effects could be avenues of future research. Moreover, while auditing studies point to a mechanism, observational studies can help to further explore how that mechanism plays out given the actual distribution of candidates. Policymakers might also consider whether there are other interventions that BTB could be combined with to reduce its adverse effects on black candidates. Race-based statistical discrimination in hiring is unlawful, and if the hiring discrimination laws were effectively enforced or operated as an effective deterrent, BTB could not have this unintended consequence. This, to be sure, is easier said than done, but the intuition behind BTB perhaps suggests one plausible innovation: asking employers to blind themselves to names in addition to records. The racial-disparity implications are not the only policy consideration surrounding BTB and whether our results imply that the policy is unsuccessful depends, of course, on what policymakers seek to maximize. To the extent that advocates and policymakers hoped this BTB would reduce racial inequality in employment opportunities, it appears to be doing quite the opposite. However, policymakers might reasonably endorse it on the ground that people with records are a group in acute need of a leg up, regardless of race. If jobs discourage crime, society may also have a special interest in providing that help for public safety reasons. Our study does not seek to inform every aspect of the policy debate surrounding BTB, but we do find that as a racial-disparity-reduction strategy, it appears to have unintended consequences. One complicating factor is that not every applicant in the real world has a racially distinctive name (only about half do), perhaps reducing the relative impact of the racial-discrimination effect in comparison to the record-discrimination effect. However, this point may be offset by the fact that real-world applicants may also have other signals of likely race on their job applications, such as their neighborhood of residence or high school; our fictional applications included no such signals, as everything was randomized among a set of fairly race-neutral options. 31 40 AGAN & STARR, BAN THE BOX , CRIMINAL RECORDS, AND STATISTICAL DISCRIMINATION References Aigner, D.J. and Glen G. Cain. (1977). “Statistical Theories of Discrimination in Labor Markets”, Industrial and Labor Relations Review 30. Autor, D.H. and D. Scarborough. (2008). “Does Job Testing Harm Minority Workers? Evidence from Retail Establishments,” The Quarterly Journal of Economics 123(1): 219-277. Bertrand, M. and S. Mullainathan. (2004). “Are Emily and Greg More Employable Than Lakisha and Jamal? A Field Experiment on Labor Market Discrimination”, American Economic Review 94(4): 991-1013 Brame, R., S.D. Bushway, R. Paternoster and M. G. Turner. (2014). “Demographic Patterns of Cumulative Arrest Prevalence by Ages 18 and 23,” Crime & Delinquency 60(3): 471-486. Bushway, S. (2004). “Labor Market Effects of Permitting Employer Access to Criminal History Records,” Journal of Contemporary Criminal Justice. Special Issue on Economics and Crime 20: 276-291. City of New York (Jan 2016), “Young http://www.nyc.gov/html/ymi/html/justice/justice.shtml#ban Men’s Initiative: Justice” Clarke, H. (December 20, 2012). “Protecting the Rights of Convicted Criminals: Ban the Box Act of 2012” Washington Post. Clifford, R. and Shoag, D. (2016). “No More Credit Score: Employer Credit Check Bans and Signal Substitution” Unpublished Manuscript Color of Change (November 2, 2015). “Civil Rights Group Responds to the ‘Ban the Box’ Executive Order” http://colorofchange.org/press/releases/2015/11/2/civil-rights-group-respondsban-box-executive-orde/ Community Catalyst. (December 2, 2013). Banning the Box in Minnesota—and across the United States, http://www.communitycatalyst.org/blog/banning-the-box-in-minnesota-and-across-theunited-states#.UuG1__Yo46U. Deming, D., N. Yuchtman, A. Abulafi, C. Goldin and L. Katz (September 2014). “The Value of Postsecondary Credentials in the Labor Market: An Experimental Study,” NBER WP #20528 DeSilver, D. (August 21, 2013). “Black Unemployment Rate Is Consistently Twice That of Whites”, Pew Research Center, http://www.pewresearch.org/fact-tank/2013/08/21/through-goodtimes-and-bad-black-unemployment-is-consistently-double-that-of-whites/ Equal Employment Opportunity Commission (EEOC). (2012). EEOC Enforcement Guidance 915.002. Consideration of Arrest and Conviction Records under Title VII of the Civil Rights Act of 1964. Eberhardt, J.L. et al. 2004. “Seeing Black: Race, Crime, and Visual Processing,” Journal of Personality and Social Psychology 87:876. 41 AGAN & STARR, BAN THE BOX , CRIMINAL RECORDS, AND STATISTICAL DISCRIMINATION Fang, H. and A. Moro. (2011). “Theories of Statistical Discrimination and Affirmative Action: A Survey” in Handbooks in Economics: Social Economics eds J. Benhabib, M. Jackson, and A. Bisin Farber, H., D. Silverman, and T. von Wachter. (2015, Sept 17) “Factors Determining Callbacks to Job Applications by the Unemployed: An Audit Study” Unpublished Manuscript. Accessed at: http://www.irs.princeton.edu/sites/irs/files/event/uploads/audit_hf09.pdf Finlay, K. (2009). “Effect of Employer Access to Criminal History Data on the Labor Market Outcomes of Ex-Offenders and Non-Offenders”, in Studies of Labor Market Intermediation, 89 (David H. Autor, ed.). Finlay, K. (2014). “Stigma in the Labor Market”, Unpublished Manuscript Freeman, R. (2008). “ Incarceration, Criminal Background Checks, and Employment in a Low(er) Crime Society,” Criminology & Public Policy 7: 405-412 Fryer, R.G. Jr and S.D. Levitt (2004). “The Causes and Consequences of Distinctly Black Names”, The Quarterly Journal of Economics 119(3): 767-805 Heckman, J.J. (1998) “Detecting Discrimination.” Journal of Economic Perspectives 12(2):101-116 Heckman, J.J. and P.A. LaFontaine. (2010). “The American High School Graduation Rate: Trends and Levels.” Review of Economics and Statistics 92(2): 244-262. Heckman, J., and P. Siegelman (1993). “The Urban Institute Audit Studies: Their Methods and Findings,” in ed. M. Fix and R. Struyk, Clear and Convincing Evidence: Measurement of Discrimination in America, 187-258. Holzer, H.J. (2007). “Collateral Costs: The Effects of Incarceration on the Employment and Earning of Young Workers” IZA Discussion Paper No. 3118 Holzer, H.J., S. Raphael and M.A. Stoll (2006). “Perceived Criminality, Criminal Background Checks, and the Racial Hiring Practices of Employers,” Journal of Law and Economics 49:451. Jarosch, G. and L. Pilossoph (2016). “Statistical Discrimination and Duration Dependence in the Job Finding Rate” Unpublished Manuscript Kroft, K., F. Lange and M. Notowidigdo (2013). “Duration Dependence and Labor Market Conditions: Evidence from a Field Experiment,” Quarterly Journal of Economics 128(3): 11231167 Lahey, J. (2008). “Age, Women, and Hiring: An Experimental Study,” Journal of Human Resources 43(1): 30-56. Lahey, J. and R. Beasley (2009). “Computerizing Audit Studies,” Journal of Economic Behavior and Organization 70(3): 508-514 List, J. (2004). “The Nature and Extent of Discrimination in the Marketplace: Evidence from the Field,” The Quarterly Journal of Economics 119(1): 48-89 Love, M. (2011). “Paying Their Debt to Society: Forgiveness, Redemption, and the Uniform Collateral Consequences of Conviction Act,” Howard Law Journal 54(3): 753-793 Minnesota Department of Human Rights (2015), “Ban The Box: Overview for Private Employers” http://mn.gov/mdhr/employers/banbox_overview_privemp.html Last Accessed Jan 19, 2016. 42 AGAN & STARR, BAN THE BOX , CRIMINAL RECORDS, AND STATISTICAL DISCRIMINATION NAACP (2014, Jan). Our Accomplishments, http://www.naacp.org/pages/2106. Neumark, D. (1996), “Sex Discrimination in Restaurant Hiring: An Audit Study”, The Quarterly Journal of Economics 111(3): 915-941. Neumark, D. (2011) “Detecting Discrimination in Audit and Correspondence Studies”, The Journal of Human Resources 47(4): 1128-1157 Neumark, D., I. Burn, and P. Button (2015) “Is it Harder for Older Workers to Find Jobs? New and Improved Evidence from a Field Experiment” NBER WP 21669 Nosek, B.A. et al. (2007) “Pervasiveness and Correlates of Implicit Attitudes and Stereotypes,” European Review of Social Psychology 2007:1. Oreopoulos, P. (2011). “Why Do Skilled Immigrants Struggle in the Labor Market? A Field Experiment with Thirteen Thousand Resumes,” American Economic Journal: Economic Policy 3(4): 148-71. Pager, D. (March 2003). “The Mark of a Criminal Record,” American Journal of Sociology 108(5): 937-975. Pager, D., B. Western, & B. Bonikowski. (2009). “Discrimination in a Low-Wage Labor Market,” American Sociological Review, 74:777-799. Phelps, Edmund S. (1972). “The Statistical Theory of Racism and Sexism”, American Economic Review. 62:659. Pinard, M. (January 7, 2014). “Ban the Box in Baltimore,” Baltimore Sun. Pinard, M. (2010). Collateral Consequences of Criminal Convictions: Confronting Issues of Race and Dignity, 85 N.Y.U. L. Rev. 457. Reaves, B. (December 2013). “Felony Defendants in Large Urban Counties, 2009 – Statistical Tables” US Department of Justice Bureau of Justice Statistics Report NCJ 243777 Riach, P.A. and J. Rich (2002). “Field Experiments in Discrimination in the Market Place”, The Economic Journal 112(483): F480-F518 Rodriguez, M and B. Avery. (April 2016). “Ban The Box: U.S. Cities, Counties, and States Adopt Fair-Chance Policies to Advance Employment Opportunities for People with Past Convictions”. National Employment Law Project Guide Accessed June 9, 2016: http://www.nelp.org/publication/ban-the-box-fair-chance-hiring-state-and-local-guide/ Shannon, S., C. Uggen, M. Thompson, J. Schnittker, and M. Massoglia (2011). “Growth in the U.S. Ex-Felon and Ex-Prisoner Population, 1948-2010” Unpublished Manuscript Southern Coalition for Social Justice. (2013). Ban the Box Community Initiative Guide, http://www.southerncoalition.org/program-areas/criminal-justice/ban-the-box-communityinitiative-guide/. Starr, S. (2015). “Do Ban the Box Laws Reduce Employment Barriers for Black Men?” Unpublished Manuscript. Stoll, Michael A. (2009). Ex-Offenders, Criminal Background Checks, and Racial Consequences in the Labor Market, 1 Univ. of Chicago Legal Forum 381 (2009). Wozniak, A. (2015, July). “Discrimination and the Effects of Drug Testing on Black Employment”. The Review of Economics and Statistics 97(3): 548-566 43 AGAN & STARR, BAN THE BOX , CRIMINAL RECORDS, AND STATISTICAL DISCRIMINATION .15 Figure 1: Callback Rates by Race, Crime, and Box: Pre-Period Applications Only 0.140 0.131 0.094 0.086 0.083 0 .05 Callback Rate .1 0.125 Box No Box Box No Box Black White Crime No Crime Notes: This figure compares callback rates within the pre-period before Ban the Box goes into effect, comparing applications with the box (application which ask about criminal records) and those without (applications that do not ask about criminal records). A callback is a personalized phone call or e-mail to the applicant requesting follow-up contact or an interview. Figure 2: Callback Rates by Race, Criminal Record, and Period: Treated Only .15 0.150 0.138 0.127 0.088 0.084 0 .05 Callback Rate .1 0.110 Pre Post Pre Black Post White Crime No Crime 44 AGAN & STARR, BAN THE BOX , CRIMINAL RECORDS, AND STATISTICAL DISCRIMINATION Notes: This figure compares callback rates within treated companies, i.e. those companies that asked the criminal record question in the pre-period, before and after Ban the Box goes into effect. A callback is a personalized phone call or e-mail to the applicant requesting follow-up contact or an interview. Table 1a: Means of Applicant and Application Characteristics and Callback Rates by Period Pre-Period Post-Period Combined Characteristics: White Crime GED Employment Gap Application has Box 0.502 0.497 0.498 0.492 0.366 0.497 0.513 0.502 0.504 0.036 0.500 0.505 0.500 0.498 0.199 Results: Callback Rate Interview Req 0.109 0.060 0.125 0.067 0.117 0.063 Callback Rate by Chars: Black White GED HSD Emp Gap No Emp Gap Observations 0.099 0.120 0.106 0.113 0.110 0.109 7246 0.111 0.139 0.127 0.122 0.126 0.124 7394 0.105 0.129 0.117 0.118 0.118 0.116 14640 Notes: Callback implies application received a personalized positive response from the employer (either via phone or e-mail). Interview request means the positive response specifically mentioned an interview. Application has box means that the application asked about criminal records. Employment (emp) gap is a 1113 month employment gap in work history, no emp gap is a 0-2 month gap. Table 1b: Callback Rates by Crime Status for Stores with the Box in the Pre-Period Callback Rate Callback Black Callback White Observations No Crime Crime Property Drug Combined 0.136 0.131 0.140 1319 0.085 0.086 0.083 1336 0.084 0.091 0.077 703 0.085 0.081 0.089 633 0.110 0.109 0.111 2655 Notes: Sample restricted to pre-period applications where the application asked about criminal records. Callback implies application received a personalized positive response from the employer. 45 AGAN & STARR, BAN THE BOX , CRIMINAL RECORDS, AND STATISTICAL DISCRIMINATION Table 2: Effects of Applicant Characteristics on Callback Rates (1) 0.0244*** (0.0057) (2) 0.0239*** (0.0054) Crime -0.0161*** (0.0053) -0.0136** (0.0054) GED -0.0014 (0.0052) -0.0041 (0.0048) -0.0076 (0.0056) 0.0096 (0.0134) 0.0097 (0.0132) 0.0097 (0.0134) Emp. Gap 0.0012 (0.0048) 0.0017 (0.0046) 0.0005 (0.0050) 0.0103 (0.0101) 0.0104 (0.0100) 0.0102 (0.0101) White Pre-Period (3) 0.0297*** (0.0070) (4) -0.0010 (0.0093) (5) -0.0012 (0.0093) -0.0520*** (0.0121) -0.0444*** (0.0134) -0.0149 (0.0096) Drug Crime -0.0501*** (0.0133) Property Crime -0.0536*** (0.0143) White x Crime Constant Observations Sample Chain FE Center FE (6) 0.0065 (0.0149) -0.0149 (0.0171) 0.1132*** (0.0156) 14640 All No No -0.0069 (0.0261) 14640 All Yes Yes 0.0016 (0.0291) 11722 Non-Box Yes Yes -0.0134 (0.0538) 2918 Box Yes Yes -0.0133 (0.0539) 2918 Box Yes Yes -0.0184 (0.0537) 2918 Box Yes Yes Notes: Dependent variable is whether the application received a callback. Standard errors clustered on company in parentheses. The non-box sample includes only applications that did not ask about criminal history; the box sample includes only those applications that asked about criminal records. Company and center fixed effects are included in Columns (2) – (6) as indicated. White is as compared to black applicants, crime is as compared to no-crime, GED is as compared to a HS Diploma and Emp. Gap is a 11-13 month gap in work history as compared to a 0-2 month gap. 46 AGAN & STARR, BAN THE BOX , CRIMINAL RECORDS, AND STATISTICAL DISCRIMINATION Table 3A: Robustness Checks on Main Effect of White Observations Specification (1) 0.0239*** (0.0054) 14640 Main (2) 0.0136*** (0.0045) 14640 Interview Sample All All White (3) 0.0242*** (0.0054) 14640 Ungroup Chain FE All (4) 0.0454*** (0.0097) 6401 Main (5) 0.0073 (0.0050) 8239 Main NJ-All NYC-All Notes: Dependent variable is whether the application received a callback. Standard errors clustered on company in parentheses. Column (1) reproduces the White coefficient from Column 2 of Table 2, and the remaining columns show the White coefficient from different specifications. Column (2) uses interview as the dependent variable rather callback. Column (3) uses ungrouped chain FE rather than grouped. Columns (4) and (5) separate the sample in the NJ sample and the NYC sample. Table 3B: Robustness Checks on Main Effect of Crime in the Box Sample Only Observations Specification (1) -0.0520*** (0.0121) 2918 Main (2) -0.0353*** (0.0062) 2918 Interview Sample All All Crime (3) -0.0522*** (0.0123) 2918 Ungroup Chain FE All (4) -0.0535** (0.0220) 1156 Main (5) -0.0513*** (0.0160) 1762 Main NJ-All NYC-All Notes: All regressions are conditional on the application having the box. Dependent variable is whether the application received a callback. Standard errors clustered on company in parentheses. Column (1) reproduces the Crime coefficient from Column 4 of Table 2, and the remaining columns show the Crime coefficient from different specifications. Column (2) uses interview as the dependent variable rather callback. Column (3) uses ungrouped chain FE rather than grouped. Columns (4) and (5) separate the sample in the NJ sample and the NYC sample. 47 AGAN & STARR, BAN THE BOX , CRIMINAL RECORDS, AND STATISTICAL DISCRIMINATION Table 4: Local Racial Composition and the Impact of Race on Callback Rates (1) (2) (3) (4) (5) (6) White 0.00717 (0.00495) -0.00603 (0.00844) 0.0322*** (0.00664) -0.0108 (0.00856) 0.0153*** (0.00589) 0.00994 (0.0164) White x NJ 0.0380*** (0.0106) 0.0335*** (0.0103) 0.0350*** (0.0104) 0.0345*** (0.0103) NJ 0.0109 (0.0172) 0.00589 (0.0171) 0.00982 (0.0175) 0.00531 (0.0168) Store CBG %White x White Store CBG %White 0.0489*** 0.0326** 0.00770 (0.0170) (0.0164) (0.0248) 0.0342*** (0.0124) 0.0334*** (0.0111) 0.0471*** (0.0171) Store CBG %Black x White Store CBG %Black Constant -0.00246 (0.00976) 14640 All Yes No Yes -0.0173* (0.00889) 14634 All Yes No Yes -0.0597*** -0.0485*** -0.0425* (0.0154) (0.0148) (0.0229) -0.0175 (0.0146) -0.0161 (0.0156) 0.0233 (0.0233) -0.000223 (0.0107) 14635 All Yes No Yes -0.0325* (0.0177) 14634 All Yes No Yes 0.00675 (0.00588) 14635 All Yes No Yes -0.0213* (0.0113) 14634 All Yes No Yes Observations Sample Chain FE Center FE Other Controls Notes: Standard errors in parenthesis clustered on chain. Dependent variable is whether the application received a callback. All columns include controls for GED, employment gap, criminal record, and preperiod. Center or company FE included as indicated. Store CBG %White(Black) is the %White (Black) in the Census Block Group that the individual store is located (or sometimes in the town/city/borough if the address was not specified). 48 AGAN & STARR, BAN THE BOX , CRIMINAL RECORDS, AND STATISTICAL DISCRIMINATION Table 5: Average Black-White Response Rate Differences by Race and Treated, Before and After BTB Goes into Effect in NJ Black - White Callback Rate, Pre Black - White Callback Rate, Post Diff Diff, Perfect Quad Sample Treated -0.008 -0.040 0.032 0.038 Not Treated -0.027 -0.022 -0.005 -0.004 Diff 0.019 -0.018 0.037 0.042 Notes: Each cell is a black-white response rate differential, measured in percentage points. The last line restricts analysis to only those stores in the “perfect quad” sample, that is, stores for which we sent two applications in the pre- and two in the post. The two outlined cells represent the raw difference-in-differences in-differences in the full sample and the perfect quad sample. 49 AGAN & STARR, BAN THE BOX , CRIMINAL RECORDS, AND STATISTICAL DISCRIMINATION Table 6: Effects of Ban the Box on Racial Discrimination, Triple Difference Specification (1) Post x Treated x White 0.0371** (0.0180) (2) 0.0409** (0.0184) (3) 0.0358** (0.0180) (4) 0.0399** (0.0200) Post x White -0.00530 (0.0125) -0.00627 (0.0123) -0.00618 (0.0128) -0.00236 (0.0136) Post x Treated -0.0102 (0.0177) -0.0115 (0.0177) -0.0198 (0.0214) White x Treated -0.0187 (0.0140) -0.0213 (0.0140) -0.0175 (0.0146) Treated 0.00893 (0.0262) 0.00954 (0.0239) 0.0167 (0.0276) White 0.0268** (0.0108) 0.0281*** (0.0107) 0.106 (0.130) 0.0247** (0.0116) Post 0.0153 (0.0131) 0.0127 (0.0137) 0.340** (0.140) 0.0163 (0.0158) Crime -0.0155*** (0.00544) -0.0152*** (0.00548) -0.0174*** (0.00666) GED -0.00261 (0.00514) -0.00567 (0.00492) -0.00307 (0.00656) Employment Gap 0.000232 (0.00466) 0.00131 (0.00456) 0.00366 (0.00577) 0.108*** (0.0267) 14640 0.027 No No No Yes All -0.0101 (0.0256) 14640 0.193 Yes Yes Yes Yes All 0.0986*** (0.0216) 11188 0.003 No No No Yes Quad Constant Observations R2 Chain FE Post x Chain FE White x Chain FE Center FE Sample 0.0962*** (0.0199) 14640 0.002 No No No Yes All Notes: Standard errors in parenthesis clustered on chain. Dependent variable is whether the application received a callback. The Quad sample indicates the “perfect quad” sample of 11,118 observations where we sent exactly 4 applications, one white/black pair in each period. Fixed effects can include, chain, post x chain, white x chain, or center, and are included as indicated. 50 AGAN & STARR, BAN THE BOX , CRIMINAL RECORDS, AND STATISTICAL DISCRIMINATION Table 7A: Robustness Checks: Triple Difference Specification (1) Post x Treated 0.0358** x White (0.018) Observations 14640 R2 0.193 Specification Main (2) 0.0326** Sample All All (3) 0.0328** (0.016) 14640 0.171 (0.017) 14816 0.197 Interview Main (4) 0.0361** (5) 0.0464 (6) 0.0266 (7) 0.0349* (8) 0.0348* (0.018) 14581 0.191 Main (0.037) 6401 0.216 Main (0.020) 8239 0.228 Main NJ NYC (0.018) 14640 0.236 Ungroup Chain All (0.018) 14640 0.226 Chain x NJ FE All Add Rev Drop Compliers RA Errors Notes: Standard errors clustered on chain in parenthesis. Dependent variable is whether the application received a positive call back, except in column (2) where it is whether the application received a specific request for an interview. All regressions include controls for, crime, GED, emp. gap, and fixed effects for center, chain, chain x white and chain x post. Column (1) recreates Table 6 Column (3). The remaining columns are each different modifications of this specification. Column (2) uses interview as the dependent variable, Column (3) adds in the reverse compliers, Column (4) drops instances where RA erred and answered a box question they weren’t required to answer or did not answer one they should have, Column (5) is restricted to only NJ, Column (6) is only NYC, Column (7) uses individual chain fixed effects regardless of size, and Column (8) divides chain fixed effects into NJ and NYC. Table 7B: Robustness Checks: Triple Difference Specification in Perfect Quad Sample (1) Post x Treated 0.0399** x White (0.020) Observations 11188 2 R 0.003 Specification Main Sample Quad (2) 0.0394** (3) 0.0351* (4) 0.0387* (5) 0.0500 (6) 0.0335 (0.020) 11188 0.004 Interview Quad (0.019) 11324 0.003 Main Quad + Rev. Compliers (0.020) 11128 0.003 Main Quad-Drop RA Errors (0.040) 4376 0.007 Main Quad NJ (0.021) 6812 0.003 Main Quad NYC Notes: Observations restricted to the “perfect quad” sample of 11,118 observations where we sent exactly 4 applications, one white/black pair in each period. Standard errors clustered on chain in parenthesis. Dependent variable is whether the application received a positive call back, except in column (2) where it is whether the application received a specific request for an interview. All regressions include controls for center FE, crime, GED, emp. gap. Panel A Column (1) recreates Table 6 Column (4). The remaining columns are each different modifications of this specification. Column (2) uses interview as the dependent variable, Column (3) adds in the reverse compliers, Column (4) drops instances where RA erred and answered a box question they weren’t required to answer or did not answer one they should have, Column (5) is restricted to only NJ, Column (6) is only NYC. 51 AGAN & STARR, BAN THE BOX , CRIMINAL RECORDS, AND STATISTICAL DISCRIMINATION Appendix A1. Applicant Profile Details Applicant profiles consist of all information that our RAs might need in order to fill out a given job application. In addition to the characteristics we randomly varied, many other types of information were necessary to include such as previous job titles and descriptions, home addresses, names of high schools, references, and e-mail addresses. We wanted to keep these additional characteristics as similar as possible while still introducing slight (random) variation so as not to arouse employer suspicion. (1) Work history: All job applicants have about 3.5 years of work experience: about 2 years as crew members at fast-food chains or convenience stores and about 1.5 years in manual labor jobs such as home improvement, landscaping, or moving. The fast-food chains or convenience stores were real companies that we were not applying to. Each applicant was randomly assigned a company from that list of fast-food chains or convenience stores. They were given crew member or team member positions and assigned relatively generic job duties meant to imply they held basic entry-level cashier-type positions at the establishments. The manual labor jobs were randomly assigned to be in landscaping, paving, moving, home improvement, or lawn care and were not given real company names. Company names were made up but based on names standard to the industries involved (e.g., A1 Best Landscaping, [Reference Last Name] Contracting LLC, or Newark Home Improvement Inc.). Applicants were similarly assigned generic job duties meant to imply entry-level, unskilled crew-member or assistant positions in the fictitious companies. All applicants are unemployed at the time of the job application, having ended their most recent job 2 or 3 months before the application is submitted. Descriptions of previous job duties and reasons for leaving jobs varied slightly. Applicants with employment gaps have 11 to 13 months of unemployment between the two jobs; those without employment gaps have only 0- to 2-month gaps. (2) Address and center city: Because it is likely that employers would be concerned about employees being able to travel to work, we wanted applicants to live near the jobs they 52 AGAN & STARR, BAN THE BOX , CRIMINAL RECORDS, AND STATISTICAL DISCRIMINATION apply to. As described in the text, to achieve that, we chose 40 geographically distributed cities or towns in New Jersey and 44 in New York City to serve as centers where the applicants’ addresses would be located; each center then served as the base for applications to jobs located nearby. To choose the centers, we first narrowed down the entire list of New Jersey cities and towns as well as community districts in New York City to those that were at least 6% black, were at least 20% white, and had median annual incomes less than $100,000. We then used an optimization tool in the ArcGIS software package to select among those possibilities the 40 centers that would minimize distance to jobs; in New Jersey this was based on the distribution of postings then found (in January 2015) on snagajob.com, and in New York City it was based on the locations of employers that we located in a BusinessUSA database. In New Jersey, we assigned every municipality in the state to its nearest center, excluding only a few small towns that were more than 20 miles from any center. In New York City, we minimized distances subject to a constraint of equal distribution of chains across centers—for example, all chains with 44 or fewer locations were distributed such that no more than one location was assigned to each center, while a chain with 45 to 88 locations would be distributed with one to two locations per center, and so forth. Within each center, eight qualifying addresses were located within census blocks that were at least 10% black and 20% white and that had a median annual income less than $100,000. All addresses came from different streets, and Google Street View was consulted to ensure that the choices were appropriate residential or mixed-use blocks and that they did not notably differ from one another. Addresses were then slightly changed so as not to represent real addresses, and they were then randomly assigned to applicants. (3) High school or GED program: For diploma earners, high schools for the New Jersey study were chosen to be in New Jersey cities or towns at least 30 miles away from the center to reduce the probability that the high school could send any unobservable signals to the employer. High schools for the New York City study were divided equally between New Jersey and upstate New York schools, since similar geographic separation could not be achieved within the city. The high schools used were all at least 10% black, are at least 20% white, have at least 25,000 people, and do not have median incomes more than 53 AGAN & STARR, BAN THE BOX , CRIMINAL RECORDS, AND STATISTICAL DISCRIMINATION $100,000. In addition, the high schools do not have median test scores above the 90th or below the 10th percentile in the state. Applicants with GEDs were randomly assigned descriptions and names of New Jersey or New York GED training programs. (4) References: Two fictitious references with phone numbers were created, representing the applicant’s supervisors for each of two previous jobs. To complement and strengthen the racial signal provided by our applicant names, the previous supervisor from the manual labor job was also given a racially distinctive name suggesting the same race as the applicant. The previous supervisor of the retail or restaurant job was given a race-neutral name. However, no employers ever called the phone numbers that we purchased and provided for the references, suggesting that little attention was likely paid to them. (5) Phone number: Each applicant was assigned a phone number based on center, race, criminal history, and time period. (Thus, each center has at least four potential phone numbers during each phase of the study; in New York City, because we were sending a larger number of distinct applications per center, we bought two numbers for each combination of characteristics and varied them randomly.) The result of that division is that no store received two applications using the same phone number. That method also helps us identify which application a voice mail belongs to, because hiring managers would not always leave all pertinent information on the voice mail. The information left, combined with the phone number being called, was sufficient to uniquely assign responses to applications. We purchased these phone numbers from www.callfire.com, which enabled us to create voicemails for our applicants using one of several available robotic voices. The wording and voice on the outgoing voice mail greeting were randomized across several options and designed to sound like a generic cell phone voice mail greeting for someone who has not recorded a personalized one. (6) E-mail address: A unique e-mail address was created for each applicant, with the format randomly varied. All e-mail addresses were created with the same domain, and the format always included the applicant’s first and last names but could also include numbers, a middle initial, periods, or underscores so as to differentiate the format across applicants to the same store. 54 AGAN & STARR, BAN THE BOX , CRIMINAL RECORDS, AND STATISTICAL DISCRIMINATION (7) Criminal record: Applicants with felony convictions were randomly assigned either a property crime or a drug crime. Within those two categories, several potential crimes were chosen—all of them meant to imply similar levels of seriousness. In addition, many applications with the box ask the applicant to “Please explain.” For that, specific language was given as part of the profiles, with sentences randomly generated to indicate when the crime occurred, a potential expression of remorse, and a potential expression of desire to discuss the matter further in person. Each of the profiles were randomly generated using the Resume Randomizer program of Lahey and Beasley (2009). Applicant pairs were always of opposite race, and were otherwise created so that the details of the aforementioned characteristics were randomly varied among the pair. For example, both members of the pair could have high school diplomas, but never from the same high school or the same town; no two applicants in the same pair had the same address; none worked for the exact same former employers; if both had a criminal record, it did not involve the same criminal charge, and so forth. For examples of profiles, which are several pages in length, please e-mail the authors. 55 AGAN & STARR, BAN THE BOX , CRIMINAL RECORDS, AND STATISTICAL DISCRIMINATION A2. Names Used Table A2.1: White and Black Names Used for Applicants White Names Black Names First %White Last %White First %Black Last %Black SCOTT 88.87 WEBER 94.37 TYREE 97.94 PIERRE 97.78 THOMAS 86.92 ESPOSITO 93.30 TERRELL 96.23 WASHINGTON 90.28 CODY 86.71 SCHMIDT 92.63 DAQUAN 96.04 ALSTON 88.96 RYAN 85.37 BRENNAN 92.45 JAQUAN 95.03 BYRD 85.50 NICHOLAS 84.99 MEYER 92.27 DARNELL 93.43 INGRAM 78.63 DYLAN 84.70 KANE 91.75 JAMAL 91.36 JACKSON 76.32 MATTHEW 83.97 HOFFMAN 91.38 MARQUIS 91.36 BANKS 75.68 JACOB 83.37 RYAN 89.98 JERMAINE 89.45 FIELDS 74.83 KYLE 82.93 WAGNER 89.96 DENZEL 89.27 BRYANT 74.49 TYLER 82.82 HANSEN 89.60 DWAYNE 88.89 WILLIAMS 74.22 SEAN 82.41 SNYDER 88.84 REGINALD 88.41 SIMMONS 72.45 DOUGLAS 81.93 ROMANO 88.84 TYRONE 86.75 CHARLES 72.33 SHANE 81.11 O'NEILL 88.72 MALCOLM 86.06 HAWKINS 70.81 JOHN 80.36 RUSSO 88.67 DARRYL 84.78 ROBINSON 70.70 STEPHEN 80.12 FOX 86.43 TERRANCE 84.12 JENKINS 70.50 SWEENEY 86.03 MAURICE 82.47 FRANKLIN 70.45 SULLIVAN 85.08 ISAIAH 74.06 JOSEPH 70.42 ELIJAH 72.35 Notes: The %race columns indicate the percentage of babies born in NJ between 1989 and 1996 with that first or last name that were of that race (i.e. 88.87% of babies with the first name Scott are White). 56 AGAN & STARR, BAN THE BOX , CRIMINAL RECORDS, AND STATISTICAL DISCRIMINATION A3. Analysis Tables for NJ Only This appendix recreates Figures 1 and 2 as well as Table 1a and 1b, Table 2 and 5 for only NJ only. 0.188 0.180 0.139 0.125 0.118 0.108 0 .05 Callback Rate .1 .15 .2 Figure A3.1: Callback Rates by Race, Crime, and Box: Pre-Period NJ Applications Only Box No Box Box Black No Box White Crime No Crime Notes: Limited to only NJ applications. This figure compares callback rates within the pre-period before Ban the Box goes into effect, comparing applications with the box (application which ask about criminal records) and those without (applications that do not ask about criminal records). A callback is a personalized phone call or e-mail to the applicant requesting follow-up contact or an interview. 57 AGAN & STARR, BAN THE BOX , CRIMINAL RECORDS, AND STATISTICAL DISCRIMINATION Figure A3.2: Callback Rates by Race, Criminal Record, and Period: NJ Treated Only 0.136 0.129 0.201 0.122 0.109 0 .05 Callback Rate .1 .15 .2 0.193 Pre Post Pre Black Post White Crime No Crime Notes: Limited to only NJ applications. This figure compares callback rates within treated companies, i.e. those companies that asked the criminal record question in the pre-period, before and after Ban the Box goes into effect. A callback is a personalized phone call or e-mail to the applicant requesting follow-up contact or an interview. 58 AGAN & STARR, BAN THE BOX , CRIMINAL RECORDS, AND STATISTICAL DISCRIMINATION Table A3.1a: Means of Applicant and Application Characteristics and Callback Rates by Period, NJ Only Pre-Period Post-Period Combined Characteristics: White Crime GED Employment Gap Application has Box 0.507 0.498 0.506 0.503 0.362 0.495 0.504 0.513 0.504 0.034 0.500 0.501 0.510 0.504 0.181 Results: Callback Rate Interview Req 0.147 0.081 0.146 0.076 0.147 0.078 0.125 0.170 0.139 0.156 0.145 0.150 2864 0.124 0.170 0.143 0.150 0.149 0.144 3537 0.124 0.170 0.142 0.152 0.147 0.146 6401 Callback Rate Chars: Black White GED HSD Emp Gap No Emp Gap Observations by Notes: Sample limited to NJ applications. Callback implies application received a personalized positive response from the employer (either via phone or e-mail). Interview request means the positive response specifically mentioned an interview. Application has box means that the application asked about criminal records. Employment (emp) gap is a 11-13 month employment gap in work history, no emp gap is a 0-2 month gap. Table A3.1b: Callback Rates by Crime Status for Stores with the Box in the Pre-Period, NJ Only Callback Rate Callback Black Callback White Observations No Crime Crime Property Drug Combined 0.164 0.139 0.188 507 0.113 0.108 0.118 530 0.102 0.087 0.118 293 0.127 0.139 0.118 237 0.138 0.124 0.151 1037 Notes: Sample restricted to pre-period applications in NJ where the application asked about criminal records. Callback implies application received a personalized positive response from the employer. 59 AGAN & STARR, BAN THE BOX , CRIMINAL RECORDS, AND STATISTICAL DISCRIMINATION Table A3.2: Effects of Applicant Characteristics on Callback Rates NJ ONLY White (1) (2) 0.0466*** 0.0454*** (0.0100) (0.0097) (3) (4) 0.0500*** 0.0260 (0.0116) (0.0213) (5) 0.0251 (0.0210) Crime -0.0157** (0.0070) -0.0153** (0.0071) GED -0.0120 (0.0089) -0.0161** (0.0078) -0.0210** (0.0087) -0.0026 (0.0285) -0.0016 (0.0281) -0.0000 (0.0273) Employment Gap 0.0008 0.0011 0.0024 -0.0065 -0.0057 -0.0062 (0.0073) (0.0071) (0.0080) (0.0123) (0.0123) (0.0125) Pre-Period -0.0535** (0.0220) (6) 0.0515 (0.0360) -0.0280 (0.0326) -0.0034 (0.0138) Drug Crime -0.0423 (0.0305) Property Crime -0.0626** (0.0250) White x Crime Constant Observations Sample Chain FE Center FE -0.0499 (0.0368) 0.1372*** (0.0192) 6401 All No No 0.0392 (0.0380) 6401 All Yes Yes 0.0333 (0.0368) 5245 Non-Box Yes Yes 0.0137 (0.0958) 1156 Box Yes Yes 0.0128 (0.0971) 1156 Box Yes Yes 0.0021 (0.1002) 1156 Box Yes Yes Notes: This table recreates Table 2 for NJ only. Dependent variable is whether the application received a callback. Standard errors clustered on company in parentheses. The non-box sample includes only applications that did not ask about criminal history; the box sample includes only those applications that asked about criminal records. Chain and center fixed effects are included in Columns (2) – (6) as indicated. White is as compared to black applicants, crime is as compared to no-crime, GED is as compared to a HS Diploma and Emp. Gap is a 11-13 month gap in work history as compared to a 0-2 month gap. 60 AGAN & STARR, BAN THE BOX , CRIMINAL RECORDS, AND STATISTICAL DISCRIMINATION Table A3.3: Effects of Ban the Box on Racial Discrimination, Triple Difference Specification NJ ONLY (1) Post x Treated x White 0.0523 (0.0380) (2) 0.0587 (0.0381) (3) 0.0464 (0.0371) (4) 0.0500 (0.0395) Post x White -0.0158 (0.0234) -0.0184 (0.0232) -0.0106 (0.0227) 0.00152 (0.0289) Post x Treated 0.0113 (0.0280) 0.00765 (0.0273) 0.00413 (0.0373) White x Treated -0.0144 (0.0307) -0.0195 (0.0307) -0.00442 (0.0314) Treated -0.00383 (0.0335) -0.00290 (0.0325) 0.00344 (0.0396) White 0.0498** (0.0206) 0.0536** (0.0205) 0.0188 (0.0348) 0.0405* (0.0204) Post -0.00447 (0.0214) -0.000530 (0.0213) 1.019*** (0.0348) -0.00828 (0.0286) Crime -0.0158** (0.00678) -0.0151** (0.00709) -0.0165** (0.00788) GED -0.0126 (0.00846) -0.0174** (0.00758) -0.0133 (0.0123) Employment Gap 0.00108 (0.00718) 0.00146 (0.00667) 0.00544 (0.0100) 0.183*** (0.0478) 6401 0.031 No No No Yes All 0.0489 (0.0360) 6401 0.216 Yes Yes Yes Yes All 0.138*** (0.0354) 4376 0.007 No No No Yes Quad Constant Observations R2 Chain FE Post x Chain FE White x Chain FE Center FE Sample 0.126*** (0.0277) 6401 0.005 No No No Yes All Notes: This table recreates Table 5 for NJ only. Standard errors in parenthesis clustered on chain. Dependent variable is whether the application received a callback. The Quad sample indicates the “perfect quad” sample of observations where we sent exactly 4 applications, one white/black pair in each period. Fixed effects can include, chain, post x chain, white x chain, or center, and are included as indicated. 61 AGAN & STARR, BAN THE BOX , CRIMINAL RECORDS, AND STATISTICAL DISCRIMINATION A4. Analysis Tables for NYC Only This appendix recreates Figures 1 and 2 as well as Table 1a and 1b, Table 2 and 5 for only NJ only. .15 Figure A3.1: Callback Rates by Race, Crime, and Box: Pre-Period NYC Applications Only 0.126 0.088 0.073 0.073 0.058 0 .05 Callback Rate .1 0.111 Box No Box Box Black No Box White Crime No Crime Notes: Limited to only NYC applications. This figure compares callback rates within the pre-period before Ban the Box goes into effect, comparing applications with the box (application which ask about criminal records) and those without (applications that do not ask about criminal records). A callback is a personalized phone call or e-mail to the applicant requesting follow-up contact or an interview. 62 AGAN & STARR, BAN THE BOX , CRIMINAL RECORDS, AND STATISTICAL DISCRIMINATION .15 Figure A3.2: Callback Rates by Race, Criminal Record, and Period: NYC Treated Only 0.121 0.108 0.094 0.067 0.063 0 .05 Callback Rate .1 0.102 Pre Post Pre Black Post White Crime No Crime Notes: Limited to only NYC applications. This figure compares callback rates within treated companies, i.e. those companies that asked the criminal record question in the pre-period, before and after Ban the Box goes into effect. A callback is a personalized phone call or e-mail to the applicant requesting follow-up contact or an interview. 63 AGAN & STARR, BAN THE BOX , CRIMINAL RECORDS, AND STATISTICAL DISCRIMINATION Table A3.1a: Means of Applicant and Application Characteristics and Callback Rates by Period, NYC Only Pre-Period Post-Period Combined Characteristics: White Crime GED Employment Gap Application has Box 0.500 0.496 0.492 0.485 0.369 0.499 0.521 0.492 0.505 0.037 0.499 0.508 0.492 0.494 0.214 Results: Callback Rate Interview Req 0.085 0.046 0.105 0.059 0.094 0.052 0.083 0.087 0.083 0.086 0.086 0.084 4382 0.099 0.110 0.112 0.098 0.104 0.105 3857 0.090 0.098 0.097 0.092 0.095 0.094 8239 Callback Rate Chars: Black White GED HSD Emp Gap No Emp Gap Observations by Notes: Sample limited to NYC applications. Callback implies application received a personalized positive response from the employer (either via phone or e-mail). Interview request means the positive response specifically mentioned an interview. Application has box means that the application asked about criminal records. Employment (emp) gap is a 11-13 month employment gap in work history, no emp gap is a 0-2 month gap. Table A3.1b: Callback Rates by Crime Status for Stores with the Box in the Pre-Period, NYC Only Callback Rate Callback Black Callback White Observations No Crime Crime Property Drug Combined 0.118 0.126 0.111 812 0.066 0.073 0.058 806 0.071 0.093 0.046 410 0.061 0.052 0.069 396 0.092 0.099 0.085 1618 Notes: Sample restricted to pre-period applications in NYC where the application asked about criminal records. Callback implies application received a personalized positive response from the employer. 64 AGAN & STARR, BAN THE BOX , CRIMINAL RECORDS, AND STATISTICAL DISCRIMINATION Table A4.2: Effects of Applicant Characteristics on Callback Rates: NYC Only (1) 0.0073 (0.0050) (2) 0.0073 (0.0050) Crime -0.0168** (0.0082) -0.0137* (0.0079) GED 0.0049 (0.0064) 0.0044 (0.0059) 0.0018 (0.0066) 0.0141 (0.0105) 0.0136 (0.0104) 0.0143 (0.0104) Employment Gap 0.0007 0.0012 -0.0033 0.0213* 0.0214* 0.0215* (0.0057) (0.0055) (0.0055) (0.0117) (0.0118) (0.0117) White Pre-Period (3) 0.0139** (0.0060) (4) -0.0182** (0.0087) (5) -0.0179** (0.0087) -0.0513*** (0.0160) -0.0571*** (0.0184) -0.0238 (0.0169) Drug Crime -0.0577*** (0.0170) Property Crime -0.0453*** (0.0164) White x Crime Constant Observations Sample Chain FE Center FE (6) -0.0239 (0.0146) 0.0115 (0.0192) 0.0961*** (0.0176) 8239 All No No 0.0192 (0.0242) 8239 All Yes Yes 0.0168 (0.0277) 6477 Non-Box Yes Yes 0.0296 (0.0575) 1762 Box Yes Yes 0.0293 (0.0572) 1762 Box Yes Yes 0.0329 (0.0567) 1762 Box Yes Yes Notes: This table recreates Table 2 for NYC only. Dependent variable is whether the application received a callback. Standard errors clustered on company in parentheses. The non-box sample includes only applications that did not ask about criminal history; the box sample includes only those applications that asked about criminal records. Chain and center fixed effects are included in Columns (2) – (6) as indicated. White is as compared to black applicants, crime is as compared to no-crime, GED is as compared to a HS Diploma and Emp. Gap is a 11-13 month gap in work history as compared to a 0-2 month gap. 65 AGAN & STARR, BAN THE BOX , CRIMINAL RECORDS, AND STATISTICAL DISCRIMINATION Table A4.3: Effects of BTB on Racial Discrimination, Triple Difference Analysis: NYC ONLY (1) Post x Treated x White 0.0267 (0.0196) (2) 0.0275 (0.0198) (3) 0.0266 (0.0203) (4) 0.0335 (0.0212) Post x White -0.00191 (0.0112) -0.00165 (0.0112) -0.00402 (0.0113) -0.00380 (0.0103) Post x Treated -0.0253 (0.0344) -0.0268 (0.0342) -0.0353 (0.0360) White x Treated -0.0229* (0.0119) -0.0228* (0.0118) -0.0244* (0.0134) Treated 0.0174 (0.0289) 0.0173 (0.0283) 0.0265 (0.0292) White 0.0116 (0.00839) 0.0112 (0.00832) 0.240*** (0.0542) 0.0139 (0.0103) Post 0.0250 (0.0220) 0.0259 (0.0220) 0.192*** (0.0162) 0.0320 (0.0231) Crime -0.0166** (0.00831) -0.0143* (0.00810) -0.0175* (0.00971) GED 0.00485 (0.00628) 0.00276 (0.00622) 0.00136 (0.00737) Employment Gap -0.000253 (0.00560) 0.000362 (0.00561) 0.00234 (0.00607) 0.108*** (0.0274) 8239 0.011 No No No Yes All 0.000804 (0.0254) 8239 0.228 Yes Yes Yes Yes All 0.0739*** (0.0177) 6812 0.003 No No No Yes Quad Constant Observations R2 Chain FE Post x Chain FE White x Chain FE Center FE Sample 0.0769*** (0.0186) 8239 0.002 No No No Yes All Notes: This table recreates Table 5 for NYC only. Standard errors in parenthesis clustered on chain. Dependent variable is whether the application received a callback. The Quad sample indicates the “perfect quad” sample of observations where we sent exactly 4 applications, one white/black pair in each period. Fixed effects can include, chain, post x chain, white x chain, or center, and are included as indicated. 66 AGAN & STARR, BAN THE BOX , CRIMINAL RECORDS, AND STATISTICAL DISCRIMINATION A5. Triple Differences with GED and Emp Gap Table A5.1: Effects of Ban the Box on GED vs High School Diploma, Triple Differences (1) -0.0108 (0.0183) (2) -0.0103 (0.0192) (3) -0.00360 (0.0190) (4) -0.0123 (0.0255) Post x GED 0.0155 (0.00971) 0.00981 (0.00962) 0.00186 (0.00969) 0.0218* (0.0127) Post x Treated 0.0137 (0.0223) 0.0142 (0.0233) 0.00619 (0.0300) Treated x GED 0.0186 (0.0155) 0.0203 (0.0150) 0.0213 (0.0219) Treated -0.00967 (0.0278) -0.0114 (0.0263) -0.00269 (0.0294) GED -0.0131 (0.00868) -0.0124 (0.00824) 0.410*** (0.131) -0.0189* (0.0110) Post 0.00478 (0.0132) 0.00469 (0.0137) 0.476*** (0.176) 0.00421 (0.0157) Crime -0.0153*** (0.00546) -0.0143*** (0.00549) -0.0174** (0.00673) Employment Gap 0.000270 (0.00466) 0.00176 (0.00475) 0.00361 (0.00583) White 0.0248*** (0.00572) 0.0236*** (0.00549) 0.0243*** (0.00613) 0.115*** (0.0280) 14640 0.027 No No No Yes All -0.0214 (0.0270) 14640 0.196 Yes Yes Yes Yes All 0.107*** (0.0244) 11188 0.003 No No No Yes Quad Post x Treated x GED Constant Observations R2 Chain FE Post x Chain FE GED x Chain FE Center FE Sample 0.116*** (0.0237) 14640 0.001 No No No Yes All Notes: This table recreates Table 5, substituting GED for White. Standard errors in parenthesis clustered on chain. Dependent variable is whether the application received a callback. The Quad sample indicates the “perfect quad” sample of observations where we sent exactly 4 applications, one white/black pair in each period. Fixed effects can include, chain, post x chain, white x chain, or center, and are included as indicated. 67 AGAN & STARR, BAN THE BOX , CRIMINAL RECORDS, AND STATISTICAL DISCRIMINATION Table A5.2: Effects of Ban the Box on Emp Gap vs No Emp Gap, Triple Differences (1) -0.0248 (0.0204) (2) -0.0262 (0.0194) (3) -0.0221 (0.0200) (4) -0.0267 (0.0231) Post x Emp Gap 0.00996 (0.0132) 0.0116 (0.0129) 0.00907 (0.0121) 0.0150 (0.0137) Post x Treated 0.0205 (0.0179) 0.0220 (0.0179) 0.0134 (0.0223) Treated x Emp Gap 0.0180 (0.0148) 0.0197 (0.0142) 0.0129 (0.0150) Treated -0.00920 (0.0297) -0.0109 (0.0274) 0.00167 (0.0300) Employment Gap -0.00549 (0.00969) -0.00775 (0.00941) 0.586*** (0.103) -0.00377 (0.00995) Post 0.00756 (0.0150) 0.00383 (0.0154) 0.633*** (0.154) 0.00764 (0.0171) Crime -0.0154*** (0.00541) -0.0150*** (0.00556) -0.0173** (0.00667) GED -0.00247 (0.00521) -0.00537 (0.00491) -0.00303 (0.00663) White 0.0247*** (0.00569) 0.0235*** (0.00529) 0.0243*** (0.00605) 0.113*** (0.0277) 14640 0.026 No No No Yes All -0.0133 (0.0251) 14640 0.194 Yes Yes Yes Yes All 0.102*** (0.0231) 11188 0.003 No No No Yes Quad Post x Treated x Emp Gap Constant Observations R2 Chain FE Post x Chain FE Emp Gap x Chain FE Center FE Sample 0.112*** (0.0233) 14640 0.001 No No No Yes All Notes: This table recreates Table 5, substituting Emp Gap for White. Standard errors in parenthesis clustered on chain. Dependent variable is whether the application received a callback. The Quad sample indicates the “perfect quad” sample of observations where we sent exactly 4 applications, one white/black pair in each period. Fixed effects can include, chain, post x chain, white x chain, or center, and are included as indicated. 68