Prisoner Classification Study, CDCR, 2011
Download original document:
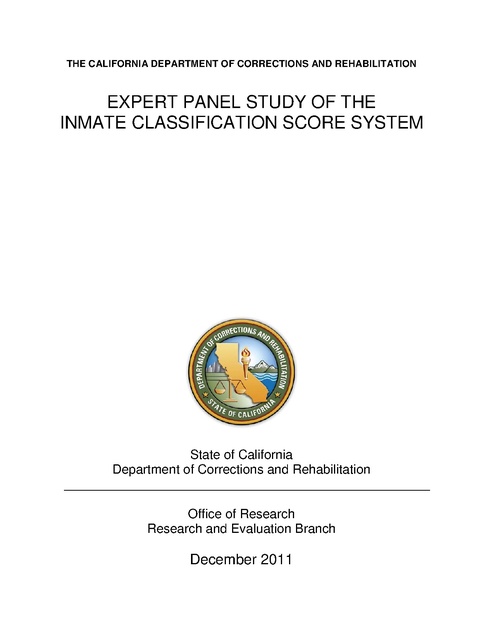
Document text
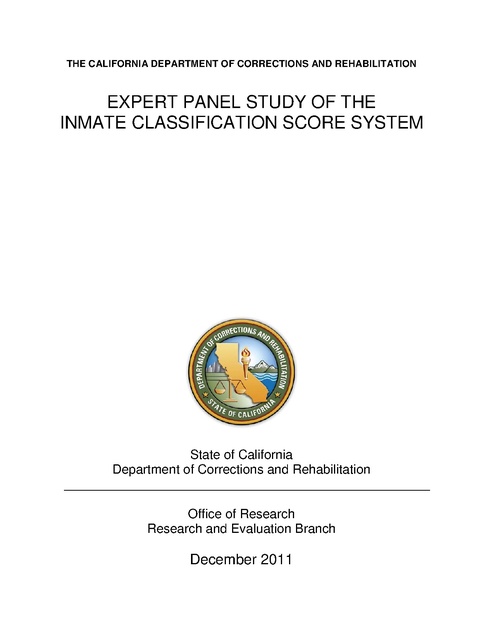
Document text
This text is machine-read, and may contain errors. Check the original document to verify accuracy.
THE CALIFORNIA DEPARTMENT OF CORRECTIONS AND REHABILITATION EXPERT PANEL STUDY OF THE INMATE CLASSIFICATION SCORE SYSTEM State of California Department of Corrections and Rehabilitation ___________________________________________________ Office of Research Research and Evaluation Branch December 2011 Distributed by the: CDCR Research and Evaluation Branch 1515 S Street., Suite 208-S, Sacramento, CA 95811 (916) 322-2919 http://www.cdcr.ca.gov/Reports_Research/index.html December 19, 2011 Matt Cate Secretary, California Department of Corrections and Rehabilitation 1515 S Street Sacramento, CA 95814 Dear Mr. Cate: Over the past 15 months, our group has served as the Expert Panel to the CDCR effort to evaluate the inmate classification system. Expert Panel members were drawn from academia and represent individuals with research, methodological and statistical skills necessary to assist and review the efforts of CDCR staff and their consultants. Throughout the course of the project, the Expert Panel has assisted the Department in the development and review of the study design, evaluated analyses and results, and provided comments on draft and final reports. Our goal has been to bring the best available methods and data to bear to key questions related to potential classification changes. The findings and recommendations presented in the report are supported by all members of the Expert Panel. We thank the many members of the CDCR who assisted the Expert Panel in our work by providing extensive documentation on the classification process, gathering and abstracting data, and answering our many questions. We hope the findings of the report will prove helpful to the Department classification process. Sincerely, _________________________ David Farabee, Ph.D. University of California, Los Angeles _________________________ Ryken Grattet, Ph.D. University of California, Davis Matt Cate _________________________ Richard McCleary, Ph.D. University of California, Irvine _________________________ Steven Raphael, Ph.D. University of California, Berkeley ________________________ Susan Turner, Ph.D. University of California, Irvine Page 2 December 19, 2011 Table of Contents Members of the Expert Panel ................................................................................................. i Department of Corrections and Rehabilitation Representatives ............................................ ii Glossary of Acronyms .......................................................................................................... iii Executive Summary ..............................................................................................................1 THE INMATE CLASSIFICATION SCORE SYSTEM STUDY ................................................ 3 Introduction ...........................................................................................................................3 History of the CDCR Inmate Classification Score System (ICSS).......................................... 3 Current ICSS Design.............................................................................................................4 Major Project Goals and Research Questions of Study ......................................................... 4 Project Methodology .............................................................................................................5 Classification Study Team ............................................................................................. 5 Prison Housing Design Tours ........................................................................................ 5 Classification Study Team Meetings .............................................................................. 6 Expert Panel Data Analysis Plan ...........................................................................................6 Data Sources ........................................................................................................................8 Study Findings ......................................................................................................................9 Expert Panel Recommendations ......................................................................................... 13 Supplementary Materials: Misclassification and Other States’ Current Strategies for Reducing Inmate Population ............................................................................................... 13 Suggestions for Future Research ........................................................................................ 13 Appendix A Overview of the CDCR Inmate Classification Process...................................... 15 Appendix B Overview of the CDCR Inmate Classification Procedures ................................ 19 Appendix C CDC 839 Classification Score Sheet ................................................................ 22 Appendix D CDC 840 Reclassification Score Sheet ............................................................ 23 Appendix E Expert Panel Data Analysis Plan March 2011 .................................................. 24 Appendix F Successful CDCR Escapes: 1999 to 2010 ...................................................... 32 Appendix G Variable List for the Classification Score and Close Custody Designation Datasets ...........................................................................................................33 Appendix H Data Analysis and Reporting Project Plan........................................................ 35 Appendix I Summary of Findings from the Regression-Discontinuity Analysis of Inmate Behavioral Outcomes ....................................................................................... 39 Appendix J Statistical Methods and Summarized Results for Gap, Matching and Longitudinal Analyses .......................................................................................................... 74 Appendix K CDCR Inmate Classification Score System Study Crosswalk......................... 115 Appendix L Cohort Descriptives ........................................................................................ 120 Inmate Classification Score System Study Appendix M Literature Review on Escape Risk Factors ................................................... 122 Appendix N Literature Review on Misclassification .......................................................... 135 Inmate Classification Score System Study Members of the Expert Panel __________________________________________________________________________________________________________________ Ryken Grattet is Professor of Sociology at the University of California, Davis. He writes about punishment and criminal law, focusing on the development and enforcement of hate crime law and the California prison and parole system. His research on the policing of hate crime in California led directly to several provisions of Senate Bill 1234, the California Omnibus Hate Crime Act of 2004. His research on the California parole system contributed to changes in the way parole violations are handled in the state. In 2005-6, he took a leave from his teaching and research duties at UC Davis to serve as the first Assistant Secretary of Research in the new California Department of Corrections and Rehabilitation. His research has been awarded the Law & Society Association 2001 Article Prize; the Society for the Study of Social Problems, Crime and Delinquency 2002 Award for Outstanding Scholarship; and the American Sociological Association, Sociology of Law Section 2007 Distinguished Article Award. In recognition of his public service contributions, Professor Grattet was awarded the Pacific Sociological Association’s 2006 Distinguished Practice Award and the UC Davis 2010 Distinguished Scholarly Public Service Award. David Farabee is Professor of Psychiatry and Biobehavioral Sciences at the University of California, Los Angeles and a principal investigator at the Integrated Substance Abuse Programs (ISAP). He has published in the areas of substance abuse, adult and juvenile crime, HIV/AIDS, and offender treatment, was co-editor of the books Treatment of Drug Offenders (2002; New York: Springer) and Treating Addicted Offenders: A Continuum of Effective Practices, Volumes I and II (2004, 2007; New York: Civic Research Institute), author of Rethinking Rehabilitation: Why Can’t We Reform Our Criminals? (2005; Washington, D.C.: AEI Press), and is co-editor of the Offender Programs Report. Richard McCleary is Professor of Social Ecology at the University of California, Irvine where he teaches courses in criminology and statistics. Dr. McCleary's research interests include the evaluation of corrections and supervision programs. Along with Jan Chaiken and Michael Maltz, Dr. McCleary recently completed an evaluation of CDCR population projection models. Dr. McCleary has been a consultant to the U.S. Bureau of Prisons and to more than a dozen state corrections departments. Susan Turner is a Professor in the Department of Criminology, Law and Society at the University of California's Irvine campus. She also serves as Director of the Center for Evidence-Based Corrections, and is a board member of the newly created California Rehabilitation Oversight Board (C-ROB). She received her Ph.D. in Social Psychology from the University of North Carolina at Chapel Hill. She has lead a variety of research projects, including studies on racial disparity, field experiments of private sector alternatives for serious juvenile offenders, work release, day fines and a 14-site evaluation of intensive supervision probation. Dr. Turner's areas of expertise include the design and implementation of randomized field experiments and research collaborations with state and local justice agencies. Dr. Turner has conducted a number of evaluations of drug courts, including a nationwide implementation study. Her article, "A Decade of Drug Treatment Court Research" (2002) appeared in Substance Use and Misuse, summarizing over 10 years of drug court research conducted while she was at RAND Corporation. Dr. Turner is a member of the American Society of Criminology, the American Probation and Parole Association, and is a Fellow of the Academy of Experimental Criminology. Steve Raphael is a Professor of Public Policy at the University of California, Berkeley. Dr. Raphael received his Ph.D. in economics from UC Berkeley in 1996. His primary fields of concentration are labor and urban economics. Dr. Raphael has authored several research projects investigating the relationship between racial segregation in housing markets and the relative employment prospects of African-Americans. Dr. Raphael has also written theoretical and empirical papers on the economics of discrimination, the role of access to transportation in determining employment outcomes, the relationship between unemployment and crime, the role of peer influences on youth behavior, the effect of trade unions on wage structures, and homelessness. i Inmate Classification Score System Study Department of Corrections and Rehabilitation Representatives __________________________________________________________________________________________________________________ Executive Office Classification Services Unit Matthew L. Cate Secretary Tanya Rothchild Chief Martin Hoshino Undersecretary Elizabeth DeSilva Correctional Counselor III Terri McDonald Undersecretary (A) Jeff Lynch Associate Warden Lee E. Seale Director Brian Moak Classification and Parole Representative Office of Research Jim Short Correctional Counselor III Jay Atkinson Deputy Director (A) Brenda Grealish Research Manager III Kevin Grassel Research Program Specialist II Jean Leonard Research Program Specialist II ii Inmate Classification Score System Study Glossary of Acronyms CC CCF CCR CDCR CQAU CSR CSU DAI DRB DDPS FTB FY ICC ICSS ISRS LWOP MEPD MSF OBIS OISB OR PG PV RC RD REB RTC RVR SHU UCB UCC UCD UCI UCLA WG Correctional Counselor Community Correctional Facility California Code of Regulations California Department of Corrections and Rehabilitation Classification Quality Assurance Unit Classification Staff Representative Classification Services Unit Division of Adult Institutions Departmental Review Board Distributed Data Processing System Franchise Tax Board Fiscal Year Institution Classification Committee Inmate Classification Score System Institution Staff Recommendation Summary Life Without Parole Minimum Earliest Parole Date Minimum Support Facility Offender Based Information System Offender Information Services Branch Office of Research Privilege Group Parole Violator Reception Center Regression Discontinuity Design Research and Evaluation Branch Return to Custody Rules Violation Report, also known as a CDC 115 Security Housing Unit University of California, Berkeley Unit Classification Committee University of California, Davis University of California, Irvine University of California, Los Angeles Work Group iii Inmate Classification Score System Study Executive Summary The California Department of Corrections and Rehabilitation (CDCR) uses an inmate classification system to ensure that inmates are properly housed and supervised. The proper housing and supervision of inmates serves goals that are paramount to the correctional setting: protecting staff and inmates from in-prison misconduct, protecting the public from inmate escapes, and safeguarding opportunities for inmates to benefit from rehabilitative programming. All three goals serve public safety by promoting institutional order and inmate rehabilitation. California’s prison system presents a multitude of housing and supervision options to achieve these goals. Housing types range from camps to open dormitories to cells. Some housing is protected by a low-security perimeter, some secured by an electrified fence. Some areas have armed coverage, others do not. Within those different types of housing, inmate supervision levels may vary as well, with some inmates more closely monitored than others. A successful inmate classification system utilizes this spectrum of choices to ensure an appropriate balance between liberty and security. Currently, CDCR uses a classification process that is based on two overlapping systems: the inmate’s placement score and the inmate’s Custody Designation. The placement score is determined by the Inmate Classification Score System (ICSS), which is further broken down into two parts – the preliminary score and any applicable Mandatory Minimums. The preliminary score predicts risk for institutional misconduct using several variables related to an inmate’s background and prior incarceration behavior. Additional Mandatory Minimum scores are then applied to inmates incarcerated for certain violent or sex crimes, crimes of public notoriety, or crimes carrying life sentences. Mandatory minimums restrict the housing level to which these inmates can be assigned. The final classification placement score is the maximum of either the preliminary or the Mandatory Minimum score. Final classification scores determine the institution or housing level in which an inmate will be placed by producing four levels of scores that correspond to four institutional housing security levels. Custody Designations attempt to mitigate an inmate’s risk for escape and threat to the community if escaped. They determine the level of in-prison supervision that inmates receive and also present a further opportunity for restricting program access and the housing levels to which certain inmates can be assigned. The purpose of this study was to evaluate CDCR’s classification system. The study aims to assist CDCR in best identifying factors that justify restrictions on liberty while avoiding factors that could lead to unwarranted impingements on inmate rehabilitation. Analyses focused on male offenders since the research design relied on the delineation between particular housing levels that are not applicable to female offenders. CDCR determined that the best strategy for carrying out the study was to work with outside correctional experts and statisticians. An “Expert Panel” was created, comprised of scholars with experience in studying correctional issues. To assist in conducting its analyses, the department contracted with the University of California (UC), Davis and UC Berkeley for statistical services. California Department of Corrections and Rehabilitation Office of Research/Research and Evaluation Branch Page 1 December 19, 2011 Inmate Classification Score System Study The major findings from this study are as follows: 1) There are no natural “breaks” in preliminary classification scores that indicate sharp changes in inmate behavior across housing levels, though the likelihood of behavioral infractions increases with preliminary score. 2) Mandatory Minimum scores appear to “trap” many well-behaving inmates into higher housing levels. Inmates crowded above the classification score cut points due to the Mandatory Minimum scores are relatively well behaved. This better behavior is explained entirely by age and the lower average preliminary scores of these inmates. In other words, age and the preliminary classification score provide a better predictor of behavior for those “trapped” at a specific placement classification score than does the actual placement classification score determined by binding mandatory minimums. 3) There is little evidence that housing inmates with preliminary scores slightly above the Classification Score Level I/II, Level II/III, and Level III/IV thresholds suppresses institutional misconduct. Furthermore, there is evidence of a criminogenic effect for inmates who have classification scores just above the Classification Score Level III/IV threshold who are placed in Level IV housing. 4) There are few escapes, particularly in institutions with electric fences. The risk of inmate escapes from facilities with electrified fences is nearly zero. Based on these findings, the Expert Panel developed the following recommendations: 1) Decisions to move inmates into lower housing levels should be guided by the safety risks those inmates pose to other inmates, staff, and the public. Estimates of risk should be grounded in the preliminary classification score and should not be overridden by CDCR Mandatory Minimum factors. Older inmates could also be given priority in downward housing placements. 2) Inmates with preliminary and placement scores at the threshold (or classification score cut points) of each housing level can be moved to lower levels with the expectation that it will not lead to increases in individual or overall rates of serious misconduct within levels. 3) CDCR should not use Custody Designation as a proxy for the risk of inmate misconduct. The custody classification system was not designed for this purpose and does not capture meaningful dimensions of an inmate’s likelihood of bad behavior. Downward movements in custody should be based upon preliminary classification score. 4) Moreover, Custody Designations may no longer be justified as a mechanism to reduce the likelihood of escape. CDCR should consider removing the use of Custody Designation as markers for escape risks. Changes to current policy need to be monitored. The Expert Panel advocates the use of random assignment as the best way to determine the impact. If that is not possible, quasiexperimental methods could provide some evidence of impact, although not as conclusive. Monitoring will require more extensive data collection on specifics of timing, location and the nature of violations than is currently collected in automated systems. California Department of Corrections and Rehabilitation Office of Research/Research and Evaluation Branch Page 2 December 19, 2011 Inmate Classification System Study THE INMATE CLASSIFICATION SCORE SYSTEM STUDY Introduction The California Department of Corrections and Rehabilitation (CDCR) uses an Inmate Classification Score System (ICSS) to ensure that inmates are properly housed and supervised. The ICSS is based on several variables, including inmate social factors and history of incarceration behavior. This system produces four levels of scores that correspond to four institutional housing security levels. A preliminary classification score predicts risk for institutional misconduct using several variables related to an inmate’s background and prior incarceration behavior. Additional Mandatory Minimum scores are applied to inmates who have case factors that require they be housed no lower than a designated housing security level (e.g., convictions for certain violent or sex crimes, public notoriety or life sentences). The final classification placement score is either the preliminary or the Mandatory Minimum score, whichever is higher. While classification scores determine the institution and housing level in which an inmate will be placed, Custody Designations further determine the amount of supervision an inmate will receive once he is placed in an institution. The purpose of this study is to evaluate the ICSS to assist CDCR in best identifying factors that justify restrictions on liberty while avoiding factors that could lead to unwarranted impingements on inmate rehabilitation. History of the CDCR Inmate Classification Score System (ICSS) California was the first state in the country to develop a standardized prison inmate classification system 1. The initial classification score system implemented by CDCR in the early 1980s relied on a consensus of opinion rather than on empirical evidence. Since that time, the CDCR Inmate Classification Score System (ICSS) has evolved based on periodic validation studies designed to improve the association between classification scores and institutional misconduct. The CDCR ICSS was first validated in the mid-1980s. The most recent validation study was conducted in 1997 by Dr. Richard Berk, University of California, Los Angeles (UCLA). As a result of the 1997 UCLA study, CDCR implemented a pilot study to evaluate the variables used to calculate initial classification scores. Based on the pilot study findings, several changes were made to the scoring practices used with inmates at their classifications. Two of these changes involved the development and application of Mandatory Minimum Scores to prevent institutional misconduct and refinement of Close Custody Designations to restrict particular inmates from having opportunities and from escaping. In 2000, CDCR implemented the more stringent Close Custody regulations, which dictate the degree of personal supervision inmates require. In 2002, CDCR implemented an updated ICSS, consistent with the 1997 UCLA research, which added Mandatory Minimum Placement Scores to systematize the administrative overrides that were prevalent in the prior ICSS. These Mandatory Minimum scores, which were not assessed in terms of their 1 Austin, J. & Hardyman, P. Objective Prison Classification: a Guide for Correctional Agencies. (2004). California Department of Corrections and Rehabilitation Office of Research/Research and Evaluation Branch Page 3 December 19, 2011 Inmate Classification System Study ability to predict institutional misconduct, applied to specific classes of inmates [e.g., Life Without the Possibility of Parole (LWOP)], ensuring they would never be housed lower than a designated level. The net result of the changes in the Close Custody regulations and the introduction of Mandatory Minimum scores led to an increased need for celled housing. Current ICSS Design The current CDCR ICSS still uses calculated scores, referred to as preliminary classification scores, and Mandatory Minimum scores. The preliminary classification score, validated by Berk (1997), predicts risk for institutional misconduct and is based on several variables related to an inmate’s background and prior incarceration behavior. Mandatory Minimum scores are then applied to restrict the housing levels of particular inmates who are considered to be threats to staff and other inmates. Final classification scores are either based on the preliminary or the Mandatory Minimum score, whichever is higher. This final score is referred to as the placement classification score. Close Custody Designations are then added, where applicable, to restrict inmate movement throughout the facilities to prevent escape and to limit the threat to communities if an escape occurs. An inmate’s classification score will fall into one of four ranges corresponding to the four institution housing and security levels; greater scores equating to greater security. A score of 0-18 is Level I, 19-27 is Level II, 28-51 is Level III, and 52+ is Level IV. Each institution is assigned a housing level based on physical construction. There are six Custody Designations used in general population housing settings: Close A, Close B, Medium A, Medium B, Minimum A, and Minimum B. Custody is assigned to denote the level of supervision the inmate requires within the institution with greater supervision at the higher custody levels. Close custody inmates require direct and constant staff supervision while minimum custody inmates may work in the community with little staff supervision. The final component to the ICSS is the Administrative Determinants, which are conditions that allow for alternative placement based upon specific case factors that may result in overrides that are inconsistent with calculated classification scores. An inmate with one or more Administrative Determinants may be housed in an institution with a housing and custody level that is inconsistent with his placement score. More detailed information about the inmate classification process is located in Appendix A. An overview of the CDCR ICSS procedures is located in Appendix B. The associated classification scoring forms that support these ICSS processes and procedures are in Appendices C and D. Major Project Goals and Research Questions of Study The major goals of this study are to investigate the possibility of adjusting upward the cut points between the four classification score levels, as well as potentially modifying the Mandatory Minimum scores and Close Custody Designations to ensure that the policies for determining security needs are based on empirical support. While Administrative Determinants are used as variables in some of the analyses, they are not in and of themselves a focus of the study. Research questions regarding classification scores include whether there are any “tipping points,” or particular scores above or below the cut points, at which there are noticeable differences in institutional misconduct. These tipping points might enable classification scores to be adjusted upward to allow more inmates to be housed in less secure housing, if California Department of Corrections and Rehabilitation Office of Research/Research and Evaluation Branch Page 4 December 19, 2011 Inmate Classification System Study appropriate. Mandatory Minimum scores are also studied to determine if they contribute any value in predicting institutional misconduct. The Close Custody Designation research questions address potential reductions in time an inmate must be closely supervised. If this time could be reduced, inmates could more quickly move from celled to dormitory housing. Close Custody Designation criteria used to prevent escapes are also studied. The research questions, along with the answers to each question, are presented in Appendix K, entitled “CDCR Inmate Classification Score System Study Crosswalk.” Project Methodology Classification Study Team Expert Panel The CDCR Office of Research (OR) determined that the best strategy for addressing the research questions was to have CDCR staff work with outside correctional experts and statisticians. Accordingly, an “Expert Panel” was created, comprised of academicians with experience in studying correctional issues. The panel members included: David Farabee, Ph.D., University of California, Los Angeles Ryken Grattet, Ph.D., University of California, Davis Richard McCleary, Ph.D., University of California, Irvine Steven Raphael, Ph.D., University of California, Berkeley Susan Turner, Ph.D., University of California, Irvine Statisticians To assist in conducting the statistical analyses the department contracted with the University of California, Davis (UC Davis) for the services of the UC Davis Statistics Department. The OR also executed a no-cost Memorandum of Understanding (MOU) with the University of California, Berkeley (UC Berkeley), for the services of a graduate student who worked directly under the close supervision of one of the Expert Panel members, Dr. Raphael. CDCR Classification Services Unit and Office of Research Representatives The CDCR Classification Services Unit (CSU) and OR worked collaboratively on all aspects of this study, including the development of the research questions for this study, and coordination of the introduction and background materials, prison tours and all meetings associated with the project. Prison Housing Design Tours In order for Classification Study Team members to understand the different types of prison housing that underlie this study, the first meeting was held on September 14, 2010, at California State Prison, Solano. Team members toured 270 design housing units and observed institutional classification committees at work (see Appendix B for a description of the institutional classification committees). A second meeting was held at California State Prison, Sacramento, on October 6, 2010, during which team members toured 180 design housing units. California Department of Corrections and Rehabilitation Office of Research/Research and Evaluation Branch Page 5 December 19, 2011 Inmate Classification System Study Classification Study Team Meetings The prison housing design tours were followed by a series of conference calls in November and December 2010, during which the study design was discussed. On January 14, 2011, the Classification Study Team convened in person at CDCR Headquarters in Sacramento. Decisions were made on the study design. CDCR Secretary Matthew Cate attended the meeting and discussed the importance of this study with the Expert Panel members. From April through November 2011, the Classification Study Team held weekly conference calls to identify data sources/elements, troubleshoot issues/concerns, review preliminary findings and monitor the overall progress of the project. Expert Panel Data Analysis Plan The Expert Panel drafted a Data Analysis Plan that identified and guided the analyses performed to address the research questions. Although the preference of the Expert Panel was to address the research questions using a randomized experiment, this method is not always feasible. Not only are there time and resource constraints due to the court-ordered mandates and departmental reduction efforts, but the safety-compromising implications associated with the potential for error in correctional settings often does not allow for experimental research. With this in mind, the Expert Panel opted for a quasi-experimental study design to evaluate the current ICSS for potential changes that could be made using existing CDCR administrative datasets, acknowledging that a future research recommendation might be to address data gaps with experimental methodologies. Female offenders were not examined for this study since the research design relied on the delineation between the housing Levels I-IV, which are only applicable to male offenders. Once drafted, input regarding the Data Analysis Plan was sought from a correctional classification system subject matter expert from the Association of State Correctional Administrators (ASCA). This feedback was incorporated into the final Data Analysis Plan, where possible. Completed in March 2011, the final Data Analysis Plan is found in Appendix E. The analytical strategies for the classification score and Close Custody Designation research questions are as follows: Classification Scores The Expert Panel sought to address two broad questions regarding the classification scores. One question is, “does the preliminary score predict the behavior of inmates whose placement scores are constrained by the Mandatory Minimum scores?” Another is, “do inmates with large differences between their preliminary and placement scores behave better than individuals with small differences between their preliminary and placement scores?” If so, then individuals with large differences could be considered better candidates for moving to lower levels than individuals with small differences between preliminary and placement scores. If there is no difference, then moving individuals with Mandatory Minimums based upon their preliminary classification score would not be advisable since such inmates would not be expected to behave differently than inmates with the same placement classification score but a higher preliminary classification score. The Expert Panel referred to this study design as a “Gap Analysis.” California Department of Corrections and Rehabilitation Office of Research/Research and Evaluation Branch Page 6 December 19, 2011 Inmate Classification System Study Two Gap Analyses are used to explore the preliminary scores of inmates who have similar placement scores due to the Mandatory Minimums in order to determine whether or not actual differences exist in their propensity to engage in institutional misconduct (also referred to as Rules Violation Reports, or “RVRs”). One uses the difference between the preliminary and placement classification scores to predict misconduct. A follow-up analysis examines each particular Mandatory Minimum criterion (e.g., LWOP, Lifer) in order to see if particular criteria indicate elevated or lowered risk of violation. Furthermore, a Matching Analysis compares inmates who have similar backgrounds, with the exception that some have a Mandatory Minimum, to see if differences exist in their propensity to engage in institutional misconduct (i.e., for a group of similar inmates, do those with Mandatory Minimum scores behave better or worse than those who do not). Both the Gap and Matching Analyses model the likelihood an inmate has an RVR (using logistic regression models), as well as an inmate’s count of RVRs during the review period (using Poisson regression models). Separate models are run to isolate results for A-F violations, A-D violations and A violations (violation types range from A through F, with A being the most serious violation type and F being the least serious). Additional statistical methods are employed to account for other relevant factors, such as the fact that inmates could have multiple reviews over time and that there are varying lengths of time between classification score reviews. The second question posed by the Expert Panel is, “would increasing the cut points at the thresholds separating the levels increase misconduct?” This is addressed through the application of a Regression Discontinuity (RD) design analysis. Specifically, the Expert Panel hypothesized that two factors may lead to an impact of security on the likelihood of a behavioral infraction: a suppression effect (tighter restrictions may suppress behavioral problems) and a peer effect (associating with poorly behaving inmates in higher security levels may result in increases in institutional misconduct for inmates who might otherwise behave better if placed in a lower security level). The RD analysis serves as an empirical approach to observe the net of these two effects. Analyses are performed to examine discontinuities at each of the classification score cut points in relation to RVRs. Multiple models examine both preliminary and placement scores in relation to: 1) inmate housing level crossing into the review period and 2) inmate housing level after the reclassification hearing at the end of the review period (statistically adjusting the model based on an estimate of the amount of time inmates spent in the housing level endorsed by the classification review). Further analyses isolate results for any violations, A violations, B/C/D violations and E/F violations. Collectively, the results from these analyses serve to triangulate around the classification score research questions. Close Custody Designations Since the Close Custody Designation research questions address both the impact the designations have on escape risk and risk for institutional misconduct, different methodological approaches were used. These methodologies include: CDCR Successful Escape Reports; manual file reviews; literature reviews; regression, Matching and Gap Analyses. California Department of Corrections and Rehabilitation Office of Research/Research and Evaluation Branch Page 7 December 19, 2011 Inmate Classification System Study Data Sources Internal meetings were held between CDCR Classification Services Unit and CDCR Office of Research staff to identify appropriate and relevant data sources to support the study. After careful consideration, the following databases were used to compile the datasets for the statisticians. Offender Based Information System (OBIS) OBIS maintains offender demographic, sentencing and parole revocation data, location where the offender is serving his sentence, length of sentence and time served, information on holds, warrants or detainers, and DNA collection information. OBIS data are used in the study to identify inmates’ locations and length of sentences. Inmate Classification Score System (ICSS) Information from paper-format classification sheets are entered into the ICSS. This system provides statistical reports and is used for quality control of the manual calculation process. ICSS data are used in this study to identify components of classification scores, including mandatory minimums, points added due to misconduct, and points subtracted due to good behavior. Distributed Data Processing System (DDPS) DDPS contains information on inmates’ housing location within an institution, classification level; job assignment; trust account activity; restitution collection; visiting activity; medical, mental health, and developmental disability identification; and canteen activity. These data are used to manage inmates locally, but are also sent to CDCR headquarters for system-wide management. DDPS data are used in this study to identify prison and housing locations, and medical, mental health or developmental disability status. Data Quality The Offender Information Services Branch (OISB) is CDCR’s primary provider of summary statistical information about inmates and parolees. It is also responsible for coordinating the timely, accurate, and consistent coding and entry of data, including classification score data. OISB staff perform data integrity and quality control functions for OBIS. Within OISB, the Classification Quality Assurance Unit (CQAU) is responsible for managing the paper-based process that captures data from the classification scoring sheets of the ICSS, the CDC Forms 839, 840 and 841. 2 Working Datasets Five datasets were produced by CDCR OR staff to support this study. One is a classification score dataset that includes male felons (non-death row) who were admitted to CDCR prior to June 30, 2009, had a reclassification review with a review period beginning date that occurred on or after July 1, 2008, and who had served at least one consecutive 2 Documentation regarding the limitations of the CDCR ICSS data are reported in the Office of Research Data Evaluation and Recommendation (ORDER) Project, Final Version 1.2. (2008). Estrada Consulting Inc. California Department of Corrections and Rehabilitation Office of Research/Research and Evaluation Branch Page 8 December 19, 2011 Inmate Classification System Study year in prison (records with inaccurate or missing dates were omitted). The remaining four datasets were created to address the Close Custody Designation questions pertaining to four sentence types: those sentenced to 15 to 50 years, 50+ years, life and multiple life. Each of these longitudinal data files includes classification data collected since November 1998 for all inmates in Level I-IV housing on June 30, 2011. The primary variables compiled in these datasets are the number and type of RVRs, preliminary classification scores, Mandatory Minimum classification scores, respective housing levels, and demographic characteristics (e.g., criminal offense, age). The complete table of variables is in Appendix G. Study Findings The Expert Panel’s Data Analysis Plan lays out the strategies for conducting the statistical analyses. CDCR OR staff performed the basic descriptive analyses. The UC Davis and UC Berkeley statisticians performed the more complex RD, Gap, Matching and longitudinal analyses. Specific responsibilities for the Classification Study Team members are located in the “Data Analysis and Reporting Project Plan” (Appendix H). Below is a brief description of the study cohort, followed by the results from the statistical analyses for the classification score and Close Custody Designation research questions. Detailed documentation of the RD analyses, entitled “Summary of Findings from the Regression-Discontinuity Analysis of Inmate Behavioral Outcomes,” may be found in Appendix I. Detailed documentation of the Gap and Matching Analyses may be found in Appendix J, entitled “Statistical Methods and Summarized Results for the Gap, Matching, and Longitudinal Analyses.” A crosswalk between the research questions, the strategies to address each of the research questions as outlined in the Data Analysis Plan, and the findings for each of the research questions is presented in Appendix K, entitled “CDCR Inmate Classification Score System Study Crosswalk.” Description of Sample Appendix L provides the demographic characteristics of the classification study cohort, which represent 98,355 reclassification score reviews. As mentioned above, this study focused solely on males as there are no housing data (Level I-IV) available for females. Nearly forty percent of those were for inmates in Level III housing. Just over 25 percent were in Level IV housing and just fewer than 25 percent were in Level II housing. Only 6.1 percent were in Level I housing. The oldest inmates (average age of 43.5 years) were housed in Level II and the youngest (average age of 36.0 years) were in Level IV housing. Race/ethnicity categories were fairly evenly distributed between the four housing levels. Hispanic inmates made up the largest percentage of inmates in Levels II – IV, while Black/African American inmates made up the largest percentage of inmates in Level I. Over 75 percent of the inmates in Levels II – IV had a crime against a person as their commitment offense. Nearly 30 percent of Level I inmates were committed for property, drug, and crimes against a person. The highest number of those required to register as a sex offender were in Level III (8,977); however, Level II had the highest proportion (26.5 percent). Most inmates designated as CCCMS or EOP were housed in Levels III and IV. In Level III, nearly 27 percent of inmates had one of these mental health designations, while just over 32 percent were designated so in Level IV. In Level IV, nearly 99 percent of the inmates had ever been committed for a serious and/or violent offense while just under 76 percent of the inmates housed in Level I had ever been committed for serious and/or violent offenses. California Department of Corrections and Rehabilitation Office of Research/Research and Evaluation Branch Page 9 December 19, 2011 Inmate Classification System Study Level II inmates had the lowest proportion of inmate reclassification score reviews where a serious disciplinary violation occurred (16.1 percent). Levels I and IV had the highest proportion of inmates with serious disciplinary violations (30.5 percent and 30.0 percent respectively). However, the majority of the Level IV violations were of the more serious variety (A1, A2, B, C, D), while the vast majority of the Level I violations were of the least serious variety (E, F). Looking at the relationship between an inmate’s preliminary score and placement score (after Mandatory Minimums are factored in) we see that nearly 66 percent of the inmates with a preliminary score that fall within Level I were elevated to Level II due to their Mandatory Minimum. Another 7 percent were elevated to Level III and 3.4 percent were elevated to Level IV. For inmates with a preliminary score that falls within level II, 3.7 percent had a Mandatory Minimum that placed them in Level III and nearly 2 percent had one that placed them in Level IV. For inmates with a preliminary score in Level III, 2.6 percent had a Mandatory Minimum that placed them in Level IV. With respect to administrative overrides, 30.1 percent of inmates with a Level I placement score were actually housed in Level II. Nearly 42 percent of the inmates with a Level II placement score were housed in Level III and only 12.7 percent of the inmates with a Level III placement score were housed in Level IV. Classification Scores The strongest findings from this study resulted from the examination of the classification scores. The results from the analyses performed to address the classification score research questions provide additional support for the work performed by Berk (1997) on the preliminary classification score in that, of the routinely captured administrative data within the CDCR, it is the best predictor of institutional misconduct. The primary classification score research questions asked if there are any natural “tipping points” or particular scores that indicate distinct increases in RVRs along the continuum of classification scores, and further questioned if cut points could be adjusted upward to allow inmates to move to lower housing levels without compromising institutional and public safety. Statistical analyses reveal that there are no “tipping points” and that there is a positive correlation between preliminary classification scores and RVRs, i.e., as preliminary scores increase so do RVRs (see Appendix I, pp. 47-48). Based on this information, classification score ranges could modestly be adjusted upward to include more inmates in the lower levels without serious compromise to institutional safety or public safety. There is little evidence of a suppression effect at each of the current classification score cut points (i.e., 19, 28, and 52). Housing inmates who are just above the cut points (or threshold) in the respective higher housing level does not suppress their institutional misconduct. There is evidence of a criminogenic effect for inmates who have classification scores just above the Classification Score Level III/IV threshold who are placed in Level IV housing. These inmates have worse behavioral outcomes than those who have scores just below the threshold and are placed in Level III housing (see Appendix I, pp. 48-51). Thus, it appears that modest adjustments may be made to the current classification score cut points without jeopardizing institutional or public safety. In fact, adjusting the Level III/IV cut point could be considered a proactive measure toward preventing institutional misconduct. Furthermore, the placement score (determined by the Mandatory Minimum factors) houses well-behaved inmates in higher levels of security than is necessary relative to in-custody behavior. Inmates placed in higher housing levels due to Mandatory Minimum scores pose less risk of engaging in institutional misconduct than individuals who are placed in a higher California Department of Corrections and Rehabilitation Office of Research/Research and Evaluation Branch Page 10 December 19, 2011 Inmate Classification System Study level determined by their preliminary score. Therefore, it is optimal to determine the appropriate housing level for inmates based on their preliminary classification score (see Appendix I, pp. 52-54). Additional classification score research questions asked if housing level restrictions may be lowered for two particular Mandatory Minimum scores: Mandatory Minimum score “B” (Life Without Parole) and Mandatory Minimum score “C” [CCR 3375.2 (a)(7), Life Inmate (multiple/execution style murders; escapes)]. The research question pertaining to Mandatory Minimum Score “B” also calls for investigation into the type of housing design that may be allowed (180 versus 270 housing design). Since data for 270 housing design are not captured electronically, the analyses performed do not directly address the impact of housing design on institutional misconduct. Analyses of the specific reasons for the Mandatory Minimum show that inmates with “B” (LWOP) and “C” (CCR 3375.2, Lifer) designations engage in less institutional misconduct than other inmates who do not have a Mandatory Minimum score (see Appendix J, pp. 9395). Again, among the variables considered in these analyses, the preliminary score was found to be a better predictor of institutional misconduct, particularly when compared to the placement score. However, these analyses should be viewed with some caution as the sample is restricted to inmates who have placement scores near housing level thresholds and may not capture all important relationships and variables which may affect RVRs. Close Custody Designations Since the original purpose for the Close Custody Designations is to restrict inmate access around institutions to prevent escape, it is logical that any statistical analyses for this study be performed on escape data. However, since few inmates escape from Level II through Level IV institutions with electric fences, it is not possible to model the impact that changes in Close Custody Designations may have on inmate escapes. Because of this issue, the Expert Panel relied on existing CDCR escape reports to address research questions about escapes. In particular, the Expert Panel examined frequency counts of successful escapes that occurred from 1999 to 2010 for each CDCR institution within the various housing levels, focusing on Levels II-IV since these levels are protected by an electric fence at most CDCR institutions (Appendix F). To supplement this information, CSU staff performed manual case reviews of successful escapes by inmates assigned to Level II through Level IV housing. They found that virtually all escapes were from settings outside of Level II through Level IV institutions 3. Such settings include reception centers, being out to court, and being out on medical leave, all of which are irrelevant to this study. Collectively, this information demonstrates that there is a near-zero escape probability for inmates who are housed behind electric fences, thus resulting in the finding that the Close Custody Designations are unnecessary for preventing escapes. 3 Between 1999 and 2010 there were 18 reported escapes of level II-IV inmates. Over that span of time the annual Average Daily Population of level II-IV inmates ranged from a low of 127,600 to a high of 138,670. However, only two of the 18 inmates (both Level II) actually escaped from behind the walls of a CDCR institution. California Department of Corrections and Rehabilitation Office of Research/Research and Evaluation Branch Page 11 December 19, 2011 Inmate Classification System Study Over time, the Close Custody Designations have evolved to serve as yet another tool to supervise inmates who engage in institutional misconduct. As such, several of the Close Custody Designation research questions focus on their relationship to institutional misconduct rather than escape. Although the Close Custody data available for statistical modeling is limited by the lack of comparison groups (current CDCR policies dictate that all inmates who meet certain Close Custody Designation requirements must be supervised at a designated custody level), the Expert Panel attempted to address these research questions through a series of exploratory analyses on the Close Custody Designations as they relate to RVRs. Analyses of the classification score data, including the longitudinal data, were used to produce and test multiple statistical models. The results from these various models are mostly ambiguous and uncertain (Appendices K and L provide documentation of these inconclusive results). Some analyses reveal that decreasing custody levels appear to increase RVRs; however, these findings hinge on inmates who are already engaging in institutional misconduct. As demonstrated by the classification score analyses, the preliminary score is the best known predictor for institutional misconduct. Therefore, the benefit of the Close Custody Designations is proportional to inmate preliminary scores. The higher the preliminary score, the greater the need for Close Custody. Conversely, the lower the preliminary score, the less the need for Close Custody. Based on the data used for these analyses, a majority of the inmates meeting the Close Custody Designation criteria who are not engaging in institutional misconduct are being closely supervised despite the fact that their preliminary classification score provides no indication that such supervision is warranted. After having an opportunity to examine the relationship between Close Custody Designations and institutional misconduct, the Expert Panel concluded that the existing administrative data are inadequate and inappropriate for addressing the research questions; a randomized experiment is necessary. Furthermore, the Expert Panel questioned the added value of repurposing the Close Custody Designations to address institutional misconduct since there are already other tools in place to do so (e.g., preliminary classification score, Administrative Determinants). Conclusions The main findings from this study may be summed up as follows: 1) There are no natural “breaks” in preliminary classification scores that indicate sharp changes in inmate behavior across housing levels, though the likelihood of behavioral infractions increases with preliminary score. 2) Mandatory Minimum scores appear to “trap” many well-behaving inmates into higher housing levels. Inmates crowded above the classification score cut points due to the Mandatory Minimum scores are relatively well behaved. This better behavior is explained entirely by age and the lower average preliminary scores of these inmates. In other words, age and the preliminary classification score provide a better predictor of behavior for those “trapped” at a specific placement classification score than does the actual placement classification score determined by binding mandatory minimums. California Department of Corrections and Rehabilitation Office of Research/Research and Evaluation Branch Page 12 December 19, 2011 Inmate Classification System Study 3) There is little evidence that housing inmates with preliminary scores slightly above the Classification Score Level I/II, Level II/III, and Level III/IV thresholds suppresses institutional misconduct. Furthermore, there is evidence of a criminogenic effect for inmates who have classification scores just above the Classification Score Level III/IV threshold who are placed in Level IV housing. 4) There are few escapes, particularly in institutions with electric fences. The risk of inmate escapes from facilities with electrified fences is nearly zero. Expert Panel Recommendations The Expert Panel developed four recommendations based on the study findings: 1) Decisions to move inmates into lower housing levels should be guided by the safety risks those inmates pose to other inmates, staff, and the public. Estimates of risk should be grounded in the preliminary classification score and should not be overridden by CDCR Mandatory Minimum factors. Older inmates could also be given priority in downward housing placements. 2) Inmates with preliminary and placement scores at the threshold (or classification score cut points) of each housing level can be moved to lower levels with the expectation that it will not lead to increases in individual or overall rates of serious misconduct within levels. 3) CDCR should not use Custody Designation as a proxy for the risk of inmate misconduct. The custody classification system was not designed for this purpose and does not capture meaningful dimensions of an inmate’s likelihood of bad behavior. Downward movements in custody should be based upon preliminary classification score. 4) Moreover, Custody Designations may no longer be justified as a mechanism to reduce the likelihood of escape. CDCR should consider removing the use of Custody Designation as markers for escape risks. Supplementary Materials: Misclassification and Other States’ Current Strategies for Reducing Inmate Population As a supplement to this study, CDCR Executive staff requested a literature review of factors related to misclassification, as well as a review of measures other states are taking to reduce their inmate population to address overcrowding (Appendix N). Despite the fact that the research is somewhat limited, it is clear that misclassification is a serious problem. Although underclassification can be a problem, it appears to be relatively insignificant compared to the repercussions of overclassification. In particular, some studies have demonstrated that inmates who are overclassified may learn new criminal behaviors through interaction with more experienced criminals. As mentioned above, this finding is also evident in this classification study as inmates housed in Level IV institutions were found to have worse behavioral outcomes than inmates who are in Level III housing. Suggestions for Future Research It is the contention of the Expert Panel that changes to current policy need to be monitored and members collectively advocate the use of an experiment as the best way to determine the impact. If that is not possible, members assert that quasi-experimental methods could California Department of Corrections and Rehabilitation Office of Research/Research and Evaluation Branch Page 13 December 19, 2011 Inmate Classification System Study provide some evidence of impact, although not as conclusive. Monitoring will require more extensive data collection than is currently collected in automated systems (e.g., timing, location, and nature of RVR). In addition to monitoring system changes that result from the findings of this study, the Expert Panel offered the following suggestions for future study: Preliminary Classification Score Two suggestions are related to the preliminary score. One is to further examine the importance of age in the calculation of the preliminary classification scores since this study resulted in a hypothesis that age may be underweighted in the final score. Another is a revalidation study, which would investigate whether a new classification system might perform better, especially given the expected nature of the inmate population changes due to realignment. Close Custody Designations Further research could be pursued to assess the true value of the Close Custody Designations in predicting institutional misconduct (i.e., do the Close Custody Designations provide additional value that is over and above the predictive power of the preliminary classification scores). Administrative Determinants Additionally, the use and predictive power of Administrative Determinants could be examined. Descriptive statistics show that administrative overrides place a substantial percentage of inmates in housing levels higher than placement scores dictate. The question to be addressed is similar to the previous question: Do Administrative Determinants provide additional value that is over and above the predictive power of the preliminary score? Cost-Benefit Study Some form of cost-benefit analyses could be conducted for randomized experiments to determine not only the impact of RVRs, but also to identify the costs associated with proposed changes. Randomized designs could be used to answer questions related to moving certain groups to lower housing levels. To answer the question about whether inmates with placement scores just above the thresholds could be safely moved down, inmates with placement scores several points above the threshold could be randomly assigned to either remain in the higher housing level or moved one level lower. To determine whether celled or dorm housing has an independent effect on RVRs, a study could focus on inmates near the II/III threshold and randomly assign into cell or dorm housing, within level II or level III. A third randomized design might consider different age groups and randomly assign age cohorts to different housing levels. Key to all randomized designs is assuring comparable inmates are in the different study groups, as well as carefully measuring behaviors using methods that may be more sensitive than current databases containing RVRs, and following all inmates for at least a period of one year in order to gauge the impact of the different treatments. California Department of Corrections and Rehabilitation Office of Research/Research and Evaluation Branch Page 14 December 19, 2011 Inmate Classification System Study Appendix A Overview of the CDCR Inmate Classification Process The current CDCR inmate classification process has three components: the Inmate Classification Score System (ICSS), Custody Designations and Administrative Determinants. Although these factors are used to classify both male and female inmates, female inmates are housed in institutions irrespective of their classification score and Custody Designations. Classification Scores 4 When inmates are first received by CDCR, their classification process begins by establishing a Preliminary Classification Score using a point system based on the following factors: - Age at first arrest Age at reception by CDCR Current term of incarceration Street gang/disruptive group Prior incarceration(s) (including juvenile, federal, other states) Prior incarceration behavior o Favorable prior behavior o Unfavorable prior behavior In addition, inmates with certain case factors will receive a Mandatory Minimum Score that requires he be housed no lower than a specific security level. The Mandatory Minimum Score was instituted to minimize unnecessary security level overrides. Mandatory Minimum Scores are given for the reasons shown in Table 1, below. Table 1. Reasons for Mandatory Minimum Score Reason Condemned Life without possibility of parole CCR 3375.2 (a)(7) Life inmate (multiple/execution style murders; escapes) History of escape Warrants “R” Suffix (sex crimes) Violence exclusion Public interest case Other life sentence Score 52 52 28 19 19 19 19 19 The final classification placement score is either the preliminary or the mandatory minimum score, whichever is highest. Currently there are four ranges of classification scores and four levels of institutions, which are reflected in Table 2, below.: 5 4 CCR Title 15. Crime Prevention and Corrections, Section 3375.3. CDC Classification Score Sheet, CDC Form 839, Calculation. 5 CCR Title 15. Crime Prevention and Corrections, Section 3375.1. Inmate Placement and Section 3377. Facility Security Levels. California Department of Corrections and Rehabilitation Office of Research/Research and Evaluation Branch Page 15 December 19, 2011 Inmate Classification System Study Table 2. Institutional Security Levels Level Points Institutional Design I 0 – 18 Open dormitory facilities and camps and low security perimeter. II 19 – 27 Open dormitories with a secure perimeter, which may include armed coverage. III 28 - 51 Housing units with cells adjacent to exterior walls and secure Perimeter with armed coverage. IV 52+ Housing units or cell block housing with cells nonadjacent to exterior walls and secure perimeter with internal and external armed coverage. The inmate’s preliminary score continues to be computed to the absolute of zero (0), below which there is no computation. It is one source used to determine in what level prison a male inmate will serve his sentence. An inmate’s placement score is the primary determining factor for the institution in which he will serve his sentence. Changes in a placement score may cause an inmate to be transferred to a different level institution. Custody Designations 6 In addition to a classification score, an inmate receives a Custody Designation which determines the level of supervision he will receive once he is in the institution. It may also impact the jobs or programs to which he may be assigned. The purpose of the Custody Designation is to determine supervision control levels based upon problematic behavior or an individual’s potential for escape and threat to the community if an escape occurs. The designation is primarily based on the following factors, although other reasons may be considered: - 6 The inmate’s total term, sentence, or remaining time-to serve The inmate’s escape history Receipt of an active law enforcement felony hold An inmate who is considered to be High Notoriety or is designated as a Public Interest Case Identification of a management concern A finding of guilt for a serious, felony level, Rules Violation Report (RVR) CCR Title 15. Crime Prevention and Corrections, Section 3377.1. Inmate Custody Designations. California Department of Corrections and Rehabilitation Office of Research/Research and Evaluation Branch Page 16 December 19, 2011 Inmate Classification System Study Table 3. Custody Designations Custody Designation Close A Housing Type Cells within Level III and Level IV facilities in housing units located within an established facility security perimeter. Close B Housing is in cells within designated institutions in housing units located within an established facility security perimeter. Medium A Housing is in cells or dormitories within the facility security perimeter. Medium B Housing is in cells or dormitories within the facility security perimeter. Minimum A Housing is in cells or dormitories within the facility security perimeter. Level of Supervision Required Custody staff supervision is required to be direct and constant. Custody staff provide direct and constant supervision at all times. The work supervisor is required to provide direct and constant supervision during the inmates’ assigned work hours. Custody and/or work supervisor supervision is frequent and direct. Custody staff provide frequent and direct supervision inside the facility security perimeter and direct and constant supervision outside the facility security perimeter. Staff supervision consists of at least hourly observation if assigned outside the facility security perimeter. Sufficient staff supervision of the inmate shall be provided to ensure the inmate is present if assigned inside the facility security perimeter. California Department of Corrections and Rehabilitation Office of Research/Research and Evaluation Branch Assignment and Activity Conditions Program assignments and activities are only scheduled within the hours of 6:00 a.m. to 6:00 p.m. unless hours are extended by the Warden to no later than 8:00 p.m. when it is determined that visibility is not compromised within the facility security perimeter. Assignments and activities are only scheduled within the hours of 6:00 a.m. to 8:00 p.m. in areas located within the facility security perimeter, including beyond the work change area in a designated Level II, Level III or Level IV institution. Inmates may participate in designated work program assignments until 10:00 p.m. when the work program is in an assigned housing unit located within the facility security perimeter. Inmates may participate in limited evening activities after 8:00 p.m. until the general evening lockup and count when the limited activity is in a designated housing unit located within the facility security perimeter. Assignments and activities are within the facility security perimeter. Assignments and activities are within the facility security perimeter. Inmates may be given daytime assignments outside the facility security perimeter but must remain on facility grounds. Assignments and activities may be inside or outside the facility security perimeter. Page 17 December 19, 2011 Inmate Classification System Study Minimum B Housing may be in cells or dormitories on facility grounds, in a camp, in a Minimum Support Facility (MSF) or in a community based facility such as a Community Correctional Facility (CCF). Sufficient staff supervision of the inmate shall be provided to ensure the inmate is present. Assignments and activities may be inside or outside the facility security perimeter. Administrative Determinants 7 The third component is Administrative Determinants. These are conditions that allow for alternative placement based upon specific case factors that may identify overriding factors inconsistent with the normal scoring process. For example, an inmate who is eligible for an identified security level who has medical or mental health needs that can be better provided at an institution with a different security level, or an inmate who is an active gang member would not be housed in an institution where he would be in danger from members of an opposing gang, although his classification score qualifies him to be sent there. An inmate with one or more Administrative Determinants may be housed in a facility with a security level which is not consistent with the inmate’s placement score. This component of the classification system was originally outside of the scope of this study. Because Administrative Determinants were found to have an impact on Close Custody and housing placement, a few major determinants were included as variables in the data analyses. 7 CCR Title 15. Crime Prevention and Corrections, Section 3375.2. Administrative Determinants. California Department of Corrections and Rehabilitation Office of Research/Research and Evaluation Branch Page 18 December 19, 2011 Inmate Classification System Study Appendix B Overview of the CDCR Inmate Classification Procedures Initial CDCR Inmate Classification When an individual is sentenced to prison, he will first go to a Reception Center (RC) located in a prison. 8 There are currently nine CDCR men’s institutions that have a RC. Upon arrival, a new inmate receives brief physical and mental health evaluations and an evaluation for any safety concerns, such as gang activity. In addition, the inmate’s legal documents are reviewed by a case records analyst and then sent to the Inmate Case Records Office (Records) for processing. Records staff assemble a Central File (C-File) for each inmate. New commitments receive new CDCR numbers. Parole Violators (PV) keep their existing CDCR number and their C-File is ordered from the appropriate Parole Records office. It can take three to six weeks for the complete assembly and processing of C-File, depending upon the inmate and the Records staff workload. During his time in the RC, the inmate receives further in-depth evaluations for physical, mental, dental and education needs. Documentation of these evaluations is added to the C-File. After a C-File is created and the evaluations are completed, a Correctional Counselor I (CC I) will review all documentation and complete a CDC 839, 9 Classification Score Sheet (Appendix C) for inmates who are new to prison. A CDC 841, Readmission Score Sheet, is completed if the inmate is a PV who returned to prison with a new term or a PV-Returned to Custody (RTC). The CCI then completes an Institution Staff Recommendation Summary (ISRS) or a CDC-816 (Readmission Summary), which suggests appropriate housing options for the inmate. The ISRS is reviewed by a Supervising Correctional Counselor II (CC II) and provided to a Classification Staff Representative who will endorse the inmate to the prison where he will serve his sentence. Ideally the total process time is 30 days from RC arrival to transfer, but complex case factors, staff shortages and overcrowding in living units can prolong the process time. In addition to the placement score a number of other factors are considered when making the final placement decision. These include the inmate’s preferences, the county of last legal residence, mental health or physical disability needs, program needs and available bed space. Endorsement and transfer from the RC usually results in arrival at a General Population (GP) institution for long term housing. An inmate is assigned to housing based on his endorsement and bed space availability. He is then assigned to a CCI, who will review the C-File and prepare for the Initial-Unit Classification Committee (UCC). The UCC is supposed to occur within 14 days of the inmate’s arrival. At this classification hearing, the inmate’s custody level, Work Group (which determines his credit-earning status) and Privilege Group (WG/PG), assignment to a waiting list if indicated, and any visiting restrictions are established. 8 There is one exception: male inmates who are sentenced to death are sent directly to the Condemned housing at San Quentin State Prison. 9 Forms continued to be referred to as CDC forms, although the department is now CDCR. California Department of Corrections and Rehabilitation Office of Research/Research and Evaluation Branch Page 19 December 19, 2011 Inmate Classification System Study Subsequent CDCR Inmate Classification Procedures Inmates are reclassified at least once annually. They may be reclassified after six months, whenever case factors indicate a change in endorsement, or when program or treatment needs require it. The form used is the CDC 840 Reclassification Score Sheet (Appendix D). There are different levels of classification committees. The first and second level committees are held within an institution. The highest level committee is held in CDCR headquarters. The committees’ responsibilities are as follows: • The Unit Classification Committee (UCC) conducts the majority of hearings dealing with routine matters of program or housing assignments. It is chaired by a Facility Captain or his or her designee. • The Institution Classification Committee (ICC) considers cases referred by a UCC when an inmate must be placed in Administrative Segregation or security housing. This committee is chaired by the Warden or his or her designee. • The Departmental Review Board (DRB) considers cases referred by ICCs to resolve an ICC difference of opinion, transfer of an inmate to a Federal or other state system, Meritorious Time Reduction cases, or any other case that is unusually complex. The DRB represents the CDCR Secretary and is comprised of various executive staff in CDCR headquarters. Housing An inmate’s classification determines the type of housing in which he will be placed. Level I or II inmates may be housed in open dormitory settings. Level III and IV inmates are placed in 180 degree or 270 celled housing units. The number of degrees refers to view from a central elevated control booth. The “180-degree” design is a configuration of the cellblocks (housing units). The cellblocks are partitioned into three separate, self-contained sections, forming a half circle (180 degrees). The partitioning of sections, blocks, and facilities ensures maximum control of movement and quick isolation of disruptive incidents, thereby ensuring effective overall management of inmates. In addition to open dormitories and cell units there are the following special housing units: • Security Housing Unit (SHU): the most secure area within a Level IV prison designed to provide maximum coverage. These are designed to house inmates that cannot be housed with the general population of inmates. This includes inmates that are validated prison gang members or gang leaders. SHU terms can vary in length. • Administrative Segregation (ASU): similar in design to a SHU, ASU houses inmates for up to 30 days, or longer with approval from a Classification Staff Representative (CSR). Inmates are placed into ASU to resolve issues that concern the safety of the inmate, the safety of others, or jeopardize the security of the institution. ASU may also house inmates as Disciplinary Detention for up to ten days as a disposition resulting from a guilty finding on a serious RVR. • Reception Center (RC): provides short term housing to process, classify and evaluate incoming inmates. • Condemned (Cond): Holds inmates with death sentences. California Department of Corrections and Rehabilitation Office of Research/Research and Evaluation Branch Page 20 December 19, 2011 Inmate Classification System Study Lethal Electrified Fences (LEF) Beginning in 1993, CDCR added LEFs to the perimeters of its prisons. All new prisons with a security classification level of II and above require the installation of an LEF. Today 27 institutions are surrounded by LEFs, which serve as lethal barriers to assist in the prevention of escapes. California Department of Corrections and Rehabilitation Office of Research/Research and Evaluation Branch Page 21 December 19, 2011 Inmate Classification System Study Appendix C CDC 839 Classification Score Sheet California Department of Corrections and Rehabilitation Office of Research/Research and Evaluation Branch Page 22 December 19, 2011 Inmate Classification System Study Appendix D CDC 840 Reclassification Score Sheet California Department of Corrections and Rehabilitation Office of Research/Research and Evaluation Branch Page 23 December 19, 2011 Inmate Classification System Study Appendix E Expert Panel Data Analysis Plan March 2011 As outlined in the project Blueprint, the major goal of this study is to determine whether the CDCR classification system may be modified without jeopardizing institutional security or public safety. The Expert Panel members developed this data analysis plan to guide the Office of Adult Research and the statistical consultant in performing analyses that will inform CDCR management in the revision of its classification practices. The proposed research design developed by the Expert Panel addresses four major questions: • What is the nature of the problem? • Does an inmate’s preliminary score add to our ability to predict incidents over and above placement score? • What is the estimated effect on violations of moving up cut-points? • Do close Custody Designations serve to reduce escape and violation risk? We note that the strongest method for answering many of these questions would involve an experimental research design in which inmates are randomly assigned to different housing or close custody levels and we would record information on subsequent violations. We do not have such a design. Our analyses are based on analysis of existing data, controlling for factors that may cloud our ability to determine the actual impacts of housing and custody levels. We caution that our conclusions must be interpreted in this light. For each question, we pose the main and secondary questions and outline proposed analysis plans for each question. We note that when we discuss violations, our analyses will consider multiple definitions if possible: 1) any violation; 2) any “serious” violation (i.e., AF); 3) any “violent” violations; 4) the number of violations and 5) the number of serious violations, and 6) the number of “violent” violations. We note that our analyses of the Blueprint questions related to custody are dependent upon the ability to tie violations to the level of custody (close, minimum, medium) the inmate was in when the violation was recorded. What is the nature of the problem? This first question addresses the current state of housing, placement and violations. How is the current population distributed across housing levels in the system and how does that distribution compare with design capacity? Which housing levels are over- and which are under-capacity? Analysis Plan Analysis is descriptive. Tables/graphs should be constructed to: • Present changes over the last 15 years in the distribution of inmates by level, and custody levels. • Illustrate the “impactedness” of all housing levels California Department of Corrections and Rehabilitation Office of Research/Research and Evaluation Branch Page 24 December 19, 2011 Inmate Classification System Study How does the existing classification process drive housing level? What is the relationship between preliminary score, placement score, and housing level? How are inmates sorted into housing levels in ways that conform to and depart from preliminary and placement scores? Analysis Plan Analysis is descriptive. Construct tables that show: • Preliminary ScorePlacement Score Housing Level for each range of Preliminary Scores (L1, L2, L3, L4). • Upward moves (mandatory minimums) for Preliminary ScorePlacement Score • Show upward and downward (administrative overrides) for Placement ScoreHousing Level How are incidents distributed by Preliminary Score, Placement Score, and Housing Level? Analysis Plan • Construct Spline graphs for Preliminary and Placement Scores broken down by Housing Level and by seriousness of the RVR. Use AIC to make this choice. Does an inmate’s preliminary score add to our ability to predict incidents over and above placement score? Do preliminary scores have predictive power in explaining the behavior of those whose placement is constrained by mandatory minimum scores? According to data provided by CDCR, the distribution of placement scores reveals bunching right above the cut-points for levels II, III, and IV. Specifically, of the 56,614 inmates in the analysis sample, 28 percent have placement scores of 19, 4.5 percent have placement scores of 28, while roughly 3.1 percent have placement scores of 52. These masses at the cutoffs exceed the mass of inmates with scores just below and just above. In conjunction with the fact that we don’t observe such bunching in the preliminary scores, these patterns suggest that the policy of assigning mandatory minimums is shifting the distribution of security designations to the right. The first approach is to focus on the behavior of inmates with placements scores of 19, 28, and 52. There are sufficient observations at each of these levels to do a simple analysis of the predictors of behavioral problems among these groups. Analysis Plan A. Matching Analysis The first analysis will match individuals with similar backgrounds but who differ in terms of whether they have a mandatory minimum. Matches will be based upon preliminary score, race/ethnicity, mental status, etc. using observed categories in the data. Matches are seldom perfect and will require as much individual level data California Department of Corrections and Rehabilitation Office of Research/Research and Evaluation Branch Page 25 December 19, 2011 Inmate Classification System Study on each inmate as we can obtain, but it will help us in our attempt to use multiple approaches to address the questions. We will determine whether “similar” inmates, except for the mandatory minimums are different on our outcomes of interest. As supplementary analyses, we will examine CCR3375, 2(a) (7) and LWOP inmates. B. “Gap-Analysis” Using Regression The Gap Analysis will use a simple set of regressions to determine whether, among individuals with similar placement scores due to imposed mandatory minimums, those with lower preliminary scores have a lower incidence of violation relative to those with higher preliminary scores. There are two ways we recommend exploring this. One is to estimate a model where the dependent variable is having committed a violation and the key explanatory variable is the difference between the preliminary and placement score. The difference effect (or lack thereof) would provide a global test of whether some of the inmates given a mandatory minimum are less risky than others. A second approach would be to create dummy variables for each of the specific reasons for mandatory minimums. CDCR uses eight official reasons: condemned, LWOP, Life, History of Escape, "R" suffix (sex crimes), violence exclusion, public interest case, and other life sentence. Controlling for preliminary score the effect of each dummy variable would tell us whether an individual given mandatory minimum has a higher incidence of 115s relative to individuals with comparable preliminary scores but who does not have a mandatory minimum. These regressions can be seen as validation tests for the mandatory minimum exceptions. The dependent variable in these analyses is a count, which skewed and nonnegative, and which suggests a Poisson or negative binomial model. Such models are relatively easy to interpret for a lay audience and variable effects can be expressed in terms of effects on the predicted probability of incidents; however, we might also consider dichotomizing the dependent variable to contrast those who have no incidents and those who have one or more, and use model a logit, probit, or linear probability model. The analyses should be undertaken for all incidents and then subdivided by violent incidents and the seriousness scale (A/B, C/D, E/F). We will need to determine what makes the best sense for the outcomes – whether to use categories such as “D or worse” or narrower categories based on specific types of violations. Decisions will be informed by the data. Analyses will be done within housing level. As supplementary analyses, we will examine CCR3375, 2(a) (7) and LWOP inmates. The main caveat of these analyses is that they will be flawed by our inability to assess the amplification or suppression of inmate behavior that may occur in the different housing levels and types. Nonetheless, put together with the other evidence we assemble below it may contribute to a fuller understanding of the likelihood of moving inmates who are subject to mandatory minimums into lower housing levels. California Department of Corrections and Rehabilitation Office of Research/Research and Evaluation Branch Page 26 December 19, 2011 Inmate Classification System Study What is the estimated effect on violations of moving up cut-points? The preliminary score is intended to provide a measure of the risk that an inmate poses to an institution in terms of their behavior while incarcerated. Presumably, higher preliminary scores should predict worse behavior. If we are willing to assume that whatever the relationship between the risk of bad behavior and the score (linear, quadratic, or governed by a higher-order polynomial), this underlying relationship is continuous, then we can estimate the impact of being in different security levels by testing for discontinuities in the violation-score relationship at key break points. In our group discussions, we have identified two factors that may lead to an impact of security level on the likelihood of a behavioral infraction. First, the tighter restrictions and more circumscribed liberties in higher security levels may suppress behavioral problems. For the sake of argument, let’s label this a suppression effect. On the other hand, one’s peers in higher security levels may be relatively bad influences, may create social situations conducive to behavioral problems etc. We can label this a peer effect. What we can observe empirically is the net of these two effects. Since they are of opposite sign (or I would guess that is the case), any significant impact of security level designation on the likelihood of committing a behavioral infraction would reflect one of these effects dominating the other. Analysis Plan The assignment process of the CDCR in conjunction with a regression-discontinuity empirical model can be used to estimate these net effects. We can illustrate the method with an example. Suppose we restrict the sample to those inmates housed in levels I or II facilities. Define the variable P as an inmate’s preliminary score and the variable P19 as a dummy variable indicating a preliminary score of 19 or higher (which should place the inmate in a level II facility). Furthermore, define the variable LII as being housed in a level II facility. The regression discontinuity model basically estimates the following two equations: S115 i = α 0 + α 1 Pi + α 2 Pi + βP19 i + δ 1 Pi P19 i + δ 2 Pi P19 i + ε i 2 2 LII i = λ0 + λ1 Pi + λ 2 Pi + κP19 i + γ 1 Pi P19 i + γ 2 Pi P19 i + η i 2 2 The coefficient β is an estimate of how someone with a preliminary score of 18 would behave if they were Arbitrarily assigned a score of 19 (and everything that entails in terms of the probability of being housed at a level II institution). The coefficientĸ measures the effect of going from level 18 to level 19 on the likelihood of being assigned to a level II facility. The ratio of one to the other ( β / κ ) provides an estimate of what would happen to behavior if we moved a person on the margin from level I to level II or vice versa. We will then redo this analysis for inmates housed in levels II and III and inmates house in levels III and IV. Note, in order for this to work there needs to be some discrete increase in assignment to higher levels as the preliminary score crosses these thresholds. Looking over some of the tabulations performed by CDCR, there does seem to be such an impact, especially between levels II and III. Of course, the breaks aren’t as sharp as they would be if it were not for the mandatory minimums ratcheting up the California Department of Corrections and Rehabilitation Office of Research/Research and Evaluation Branch Page 27 December 19, 2011 Inmate Classification System Study placement scores. We need to determine which of the six outcomes would be used in this analysis –this determination will be made based on distributions of the different outcomes in the data. Using a quadratic trend on either side of the break does indeed assume that the relationship between behavior and the preliminary score is smooth. We could also try higher-order polynomials; use more non-parametric techniques (with various levels of smoothing) on either side of the discontinuity and also look specifically within the neighborhood of the break. The latter might be problematic if the sample size is very small, but we will try it and see what happens. Regarding interpretation of the coefficient, the trend terms are involved in calculating the change as we pass through the discontinuity. We can and will calculate effect sizes in this manner. The way it is written above effectively identifies the impact if one were to extrapolate the trend on either side of the discontinuity beyond the break. Both ways are commonly used in applied social science research and we will try both. Regarding measuring the effect of different security levels, we actually are not conditioning the RDD models on housing level. We are simply conditioning on nonlinear functions of the preliminary score with a permit structural break. Ultimately, these estimates will be equivalent to two-stage least squares estimates of security level on behavior, where the discontinuous increase in moving levels at the threshold is used as an instrument for level of housing. Do close Custody Designations serve to reduce escape risk and violations? Custody Level and Escape Risk Analyses of the Custody Designations on escapes may be limited. This is because we expect to see few escapes, particularly with the advent of electric fences which appear to have dramatically reduced the occurrence of escapes. Nevertheless, as part of a comprehensive review of classification procedures, data should be collected to specify this trend and to describe the primary “drivers” that determine how Custody Designations are made. From a policy standpoint, both of these goals can be achieved largely through the use of descriptive statistics. Analysis Plan A. Describe Escapes Documenting escape trends would involve reporting the number of escape events by year for the past 10 years (or earlier, if possible). To control for the growth in the CDCR inmate population over time, a chart should be prepared plotting both the raw numbers of escapes by year, with a separate trend line based on the number of escapes per 1,000 inmates (i.e., rates of escape). These data should be limited to inmates escaping from secure facilities, not walk-aways from minimum support facilities (although a separate plot of walk-aways might also be of interest). If possible, escapes should be differentiated by facility level, as well as type of custody, housing type, and whether the facility has electrified fences. Preliminary data show such a small number of escapes that we will not be able to perform analyses which attempt to describe those inmates who are at better or worse risk for escape (however, we propose on analysis based on sentence length below). California Department of Corrections and Rehabilitation Office of Research/Research and Evaluation Branch Page 28 December 19, 2011 Inmate Classification System Study To determine whether there is a relationship between sentence length and escape, we can calculate the mean sentence length (as well as other descriptive statistics) for those who escape. We can also determine whether the escapes are due to any particular sentence type (e.g., life, 3-striker). Due to the low number of escapes, we cannot determine whether there is a suitable range (time remaining to serve) that can be identified to allow for adjustment and identify risk. B. Document Custody Rules and Regulations Assessing the principal drivers of Custody Designations will require a review of the policies and procedures governing this practice. According to CCR Title 15. Crime Prevention and Corrections, Section 3377.1, inmates are assigned designations based (primarily) on the following factors: • • • • • • The inmate’s total term, sentence, or remaining time-to serve The inmate’s escape history Identification of a management concern Receipt of an active law enforcement felony hold A finding of guilt for a serious Rules Violation Report (RVR) An inmate who is considered to be High Notoriety or is designated as a Public Interest Case This descriptive analysis will require a compilation of the reasons using Title 15 as a source. C. Which Close Custody Designations are Used Most Frequently? This would start with a descriptive analysis in which a table would be created that shows a breakdown of close Custody Designations by the factors leading to that designation. Assuming that these reasons have been relatively stable over time, a historical plot would not be necessary. Rather, a simple bar chart could be prepared based on aggregate data from the past 5 years. This simple, descriptive analysis would show which of the factors listed above account for the largest share of close-Custody Designations. Custody Level and Violations This analysis will examine the relationship between custody level and violations using crosstabs and regression analyses. These analyses will primarily try and address the “suppression” effect of being in close custody, as opposed to minimum and medium security. The variables we may be able to examine in custody analyses include demographic characteristics (age, ethnicity), sentence length, preliminary score, placement score, housing level, and custody level. Analysis Plan Multiple analyses will be conducted. Perform crosstabs/means, by housing level, that show violations (using outcome measures for violations) for subgroups defined by preliminary score, placement score and custody level. California Department of Corrections and Rehabilitation Office of Research/Research and Evaluation Branch Page 29 December 19, 2011 Inmate Classification System Study Regress violations as a function of placement score + preliminary score + close custody [plus covariates such as age, ethnicity, and sentence length] for the violation outcomes. Regressions should be done separately by housing level. We will examine multicollinearity among variables, particularly placement and preliminary score. We will determine whether differences in outcomes are due to individual characteristics or close custody. We will try to determine if there are certain types of offender characteristics associated with better outcomes from the regression analyses. Matching can also be done here; matching on inmate characteristics except for close custody status. Supplemental regression analyses would be conducted (in the same spirit as for the “gap” analysis) in order to assess which of the reasons used for Custody Designation are associated with greater or fewer violations. However, in this analysis, it is not clear a priori which reasons affect which Custody Designations. We will need to determine which reasons affect which designations in order to conduct this analysis. If we can, we will create dummies for the reasons and use them in regressions in place of the custody status. To determine whether changes in close custody time can be reduced, we propose a three-step strategy: 1) first determine how many are in each group (in Blueprint custody question #1); 2) if there are enough inmates, determine if there is a relationship between time to MERP and violation using regression with individual level covariates; 3) if there is a relationship, identify inmates at the lowest risk. To determine whether Minimum A and Minimum B custody can be combined into one custody level, the first step would be to gather current information on the numbers of inmates in the two different custody levels. If there were enough in Minimum A (Kevin Grassel’s data show several hundred), we can predict RVRs as a function of background inmate variables and Min A vs. Min B status. How many close custody inmates can be safely removed to lower custody levels? In the Blueprint, several questions are asked about how many current inmates can be moved as a result of analyses undertaken. This applies to Blueprint Custody questions 4, 5). In general, answers to these questions would require additional data on current inmate populations – not the sample we are working with. In addition, it would require a determination made in conjunction with CDCR staff about what constitutes “safely” moved. For example, if one were willing to tolerate 5% increase in violations, the number that could be “safely” moved would be less than if one were willing to tolerate 20%. We could also do different scenarios of risk tolerance, but would require guidance from CDCR on what are reasonable parameters. Miscellaneous Blueprint questions related to custody The Blueprint asks several questions that may be best answered with literature reviews. For example, in order to determine whether the current regulatory Close Custody accurately identify escape risk based on evidence based practices or to identify criteria that could be used to change an inmate’s custody classification, a literature review would be appropriate. This might be conducted by AOR staff. California Department of Corrections and Rehabilitation Office of Research/Research and Evaluation Branch Page 30 December 19, 2011 Inmate Classification System Study In order to determine whether electrified fences have reduced the number of escapes, we would require data on escapes and whether institutions had electrified fences at the time of the escape (Kevin has sent data on this). This is a descriptive analysis since the numbers of escapes are so low. To determine whether data are available that identifies age and physical impairment as factors that would allow for reduced custody, we would need to ask OAR if these data are captured in automated files that can be used. We can address age as a risk factor in analyses described above that identify individual level factors associated with violations. California Department of Corrections and Rehabilitation Office of Research/Research and Evaluation Branch Page 31 December 19, 2011 Inmate Classification System Study Appendix F Successful CDCR Escapes: 1999 to 2010 1999 2000 2001 2002 2003 2004 2005 2006 2007 2008 2009 2010 Yearly Avg Camps CA Corr Center Sierra Cons Cente CA Men's Colony Total 7 8 0 15 4 7 0 11 3 1 1 5 3 3 0 6 0 5 1 6 3 6 0 9 2 11 0 13 6 5 0 11 3 1 0 4 6 4 0 10 3 12 0 15 4 5 0 9 9.50 Level I CA Corr Center Wasco Chuckawalla Pelican Bay RJ Donovan Sierra Cons Cente CA Medical Facility Centinela High Desert North Kern Salinas CA Men's Colony Folsom CA Corr Institution Calipatria CA Inst for Men Pleasant Valley San Quentin Mule Creek Deuel Corr Training Facil Ironwood Corcoran CSP-LA CSP-SAC Total 0 0 0 0 0 0 0 0 1 0 0 0 0 0 0 0 0 1 1 0 0 0 1 0 0 4 0 1 0 0 2 1 0 0 0 0 0 0 3 0 1 0 0 1 0 0 1 1 0 0 0 11 0 0 0 1 1 0 0 1 0 1 0 0 1 0 0 0 1 1 0 1 0 0 0 0 0 8 0 0 0 0 1 0 0 0 0 0 0 0 2 0 0 0 0 0 2 1 0 0 0 2 1 9 0 2 2 0 0 1 0 0 1 0 1 0 0 0 1 1 0 0 0 0 0 0 0 0 0 9 0 2 0 0 0 0 2 5 0 0 0 0 0 0 0 0 0 0 0 0 0 0 0 0 0 9 0 0 1 1 2 0 0 0 0 0 0 0 0 0 0 0 0 0 0 0 0 0 0 0 0 4 1 0 0 0 0 0 1 2 1 1 2 0 0 0 0 0 0 0 0 0 0 0 0 0 0 8 1 0 0 0 1 0 0 0 0 0 0 1 1 0 0 0 0 0 0 0 0 0 0 0 0 4 0 0 2 0 1 1 2 0 0 0 0 0 0 1 0 0 0 0 0 0 0 0 0 0 1 8 1 0 0 0 2 0 0 0 0 0 0 1 0 0 0 0 0 0 0 0 0 0 0 0 0 4 0 0 0 0 0 0 0 0 0 0 0 0 1 0 0 0 0 0 0 0 0 0 1 0 1 3 6.75 Level II Pitchess San Quentin Avenal Folsom CA Rehab Center Total 2 0 0 0 0 2 0 1 0 0 1 2 0 0 0 0 0 0 0 0 0 0 0 0 0 0 1 0 0 1 0 0 0 0 1 1 0 1 0 0 0 1 0 0 1 0 0 1 0 0 0 0 0 0 0 0 0 0 0 0 0 0 1 0 2 3 0 0 0 1 0 1 1.00 Level III Ironwood CA Inst for Men Wasco Deuel RJ Donovan Total 1 0 0 0 0 1 0 0 0 0 0 0 0 0 0 0 0 0 0 0 0 0 0 0 0 0 0 0 0 0 0 1 0 0 0 1 0 0 1 0 0 1 0 0 0 0 0 0 0 0 0 0 0 0 0 0 0 1 1 2 0 0 0 0 0 0 0 0 1 0 0 1 0.50 Level IV 0 0 0 0 0 0 0 0 0 0 0 0 0.00 Other Deuel I/II Salinas I/II Kern Valley I/II Pleasant Valley I/II SATF-CORC Total 0 0 0 0 0 0 0 0 0 0 0 0 0 0 0 0 0 0 0 0 0 0 0 0 0 0 0 0 0 0 0 0 0 0 0 0 0 0 0 0 0 0 1 0 0 0 0 1 0 2 0 0 0 2 0 0 2 0 1 3 0 0 0 0 0 0 0 0 0 1 0 1 0.58 Grand Total 22 24 13 15 16 20 19 21 10 23 22 15 18.33 California Department of Corrections and Rehabilitation Office of Research/Research and Evaluation Branch Page 32 December 19, 2011 Inmate Classification System Study Appendix G Variable List for the Classification Score and Close Custody Designation Datasets Variable A1A2_Viol adjust840 age BCD_Viol C1 C2 C3 C4 C5 C6 C7 C8 C9 CLVL contmin840 count_RVR csrcep840 csrina840 csrinb840 DDPS det1 det1_840 det2 det2_840 det3 det3_840 det4 det4_840 det5 det5_840 DLVL DPP dppdate EF_Viol EOPDate Ethnicity FACILITY Firstarrest HCAT HCLV HPAS ID IN_DATE L4_180_840 L4_180R840 lastd840 Length_Stay Type Char Num Num Char Num Num Num Num Num Num Num Num Num Char Num Num Char Char Char Char Char Char Char Char Char Char Char Char Char Char Char Char Num Char Num Char Char Char Char Char Char Num Num Char Char Num Num Description Has an A-1/A-2 Violation (Y/N) Additional adjustments (+/-) to score Age at REV BEG date Has an B,C, or D Violation (Y/N) Number of A-1/A-2 Violations Number of B,C, or D Violations Number of E or F Violations Number of 'battery on non-prisoner' enhancements Number of 'battery on prisoner' enhancements Number of 'drug distribution' enhancements Number of 'deadly weapon' enhancements Number of 'inciting a disturbance' enhancements Number of 'cause serious injury' enhancements custody level points subtracted for Continuous Minimum Custody Total number of RVRs Reason for administrative or irregular placement Endorsed Institution (location they should move to) Endorsed level/program (level/program they should move to) DDPS data available on REV BEG date (Y/N) if = *, then corresponding Administrative Determinant Code is removed Administrative Determinant Code if = *, then corresponding Administrative Determinant Code is removed Administrative Determinant Code if = *, then corresponding Administrative Determinant Code is removed Administrative Determinant Code if = *, then corresponding Administrative Determinant Code is removed Administrative Determinant Code if = *, then corresponding Administrative Determinant Code is removed Administrative Determinant Code DDP level (developmental disability) (via DDPS) Disability Placement Program Code Date of DPP Code Designation Has an E or F Violation (Y/N) Date Designated EOP Race/Ethnic Group Facility name Age at time of first arrest ever (Categorical) Housing category (Cell/Dorm) Housing level (Housing Level: I, II, III, IV) Housing program assignment Pseudo Identification Number TERM GROUP START DATE Level IV 180 Degree Design Status Reason Code for Level IV 180 Degree Design Date of previous 840 review Number of days served as of REV BEG date California Department of Corrections and Rehabilitation Office of Research/Research and Evaluation Branch Page 33 December 19, 2011 Inmate Classification System Study Variable List for the Classification Score and Close Custody Designation Datasets (continued) Variable MHcode netchange Type Char Num NEW_840_CALC nodisp840 now1_840 now2_840 OFFSGRP old_840 Old_Placement OUT_DATE PRELDATE PRELDATE_TYPE rdate840 revbeg revend reversed Risk_Level RVR SCORE840_CALC scoref840 scorem840 SCOREM840_CALC SEN_TYPE sent_Length Sex SexReg_Date SexReg_Flag Streetgang Stays Sum_RVRs Num Num Char Char Num Num Num Num Num Char Num Num Num Char Num Char Num Char Num Num Char Num Char Num Char Char Num Char SV voc840 Char Num Description Mental Health Status Net change in preliminary score during review period from old preliminary score New Preliminary Score (Computer Calculated) points subtracted for No Serious Disciplinary incidents Institution at time of review Program/Level at time of review Commitment Offense Group Old Preliminary Score Old Placement Score TERM GROUP END DATE Projected Release Date Source of Projected Release Date Date 840 review form was completed Start of review period End of review period Revend and revbeg dates were switched (Y/N) CSRA Risk Score Has an RVR (Y/N) New Placement Score (Computer Calculated) Reason code for Mandatory Minimum (A through H) MANDATORY MINIMUM SCORE (Paper) New Mandatory Minimum (Computer Calculated) SENTENCE TYPE (D,2,3,L,R,W) - via OBIS Length of Sentence in Months Gender Date Sex Reg Flag was Assigned Required to Register as a Sex Offender (Y/N) Street gang member at time of incarceration (YES/NO) Number of incarcerations at CDCR ever RVRs Received (None, One, Multiple) Has been incarcerated at CDCR for a serious and/or violent offense EVER points subtracted for average (or above) performance in programming California Department of Corrections and Rehabilitation Office of Research/Research and Evaluation Branch Page 34 December 19, 2011 Inmate Classification System Study Appendix H Data Analysis and Reporting Project Plan July 2011 Background An overview of this project and its scope are well-summarized in the “Inmate Classification Study, Project Data Analysis” and the “crosswalk” that were prepared by the Expert Panel, which lists questions to be addressed and the proposed analyses for each question. One of the original strategies to approach this project was to train California Department of Corrections and Rehabilitation (CDCR), Office of Research, Adult Research Branch (REB), staff to perform these statistical analyses under the direction of the project’s University of California, Davis (UCD), Statistical consultant. However, since that plan was envisioned, the project has encountered delays (related both to the contracting process and to the dataset development) that have resulted in a need to change this strategy. Rather than training REB staff as the analyses are performed, the Statistical consultant is first going to work independently to perform these analyses and, once the analyses are finalized, will then train REB staff on the steps that were taken to perform the analyses. Thus, the analyses will not be a product of the training sessions, but rather will be used to guide a subsequent training portion of the project. Furthermore, some analyses that would have initially been delegated to the UCD Statistical consultant will now be directed to the University of California, Berkeley (UCB), graduate student who will work under the close supervision of Expert Panel member, Dr. Raphael. The “Inmate Classification Study, Project Data Analysis” plan and the “crosswalk” are subject to modification because data that would enable the planned analyses are not always available in the anticipated form, or because some additional data have been acquired that enable questions to be addressed in more sophisticated ways. While the intent of this document is to reflect current thinking about the questions and the approaches to be used, it should be recognized that even this project plan may change as the study progresses. Project Steps Step One: Creation of Data Sets for Subsequent Analysis REB staff will create the working data sets to be used in the analysis phase. They will also maintain any associated code used to manipulate the data in standardized ways. Two primary data sets will be created and used for these analyses. The first is a Classification Score System data set that includes data collected on and reported from the CDCR 840 Reclassification Score Sheet for annual classification reviews that began in Fiscal Year 08/09 and ended during FY 09/10 (no later than June 30, 2010). Note: This data set contains data on rules violation reports (RVRs), which are of most interest when examining institutional misbehavior (or lack thereof). Most of the observations reflect an annual review on individual inmates, but there will be some inmates who receive more than one review during a 12-month period. To avoid biasing the results toward the types of inmates who receive a single annual review, the inmates with multiple reviews need to be included in this data set. This data set has been created and provided to the Statistical consultants at UCD and UCB. California Department of Corrections and Rehabilitation Office of Research/Research and Evaluation Branch Page 35 December 19, 2011 Inmate Classification System Study The second is a longitudinal data set intended to include currently incarcerated inmates who were sentenced for crimes requiring Close Custody. This data set is comprised of four different groups of inmates: lifers, life without possibility of parole, 15 to 49 year sentences, and 50 year plus sentences. These groups vary in the amount of time they are in Close A Custody. The goal is to create a longitudinal data set going back ten years. This data set is under construction as of June 2011. Step Two: Quality Checks An analysis that is based on erroneous data will be intrinsically flawed. Therefore, an essential part of the data analysis is to review the data for possible errors and investigate the validity of any questionable observations. The UCD Statistician and UCB graduate student will perform quality checks on all data provided to them. An REB staff person will be the point of contact for all communication on correcting data and will be responsible for managing any code associated with correcting identified errors. It is incumbent upon UCD and UCB staff to keep REB apprised of any data issues. Step Three: Projected Analyses RVR Analyses The UC Davis Statistician will perform the following analyses: 1. A matching analysis will be performed to compare the RVR records (likelihood and frequency for various severity levels) of subjects with similar preliminary scores and other demographic information, comparing inmates who have mandatory minimum sentences against those without such sentences. The exact form of the matching algorithm will be determined based on preliminary analysis of the data, though initial attempts will be based on a callipered Mahalanobis distance algorithm. Similar analyses will be run for other categories of inmates whose preliminary and placement scores are not equal for other reasons. 2. The gap analyses will examine whether subjects with similar placement scores, but different preliminary scores, have a similar likelihood or number of RVRs. The variable of central interest added to this model would be the difference between the preliminary and placement scores. Separate analyses would be run for subjects in the vicinity of each of the mandatory minimum cutoff points (19, 28 and 52). These analyses will be performed using logistic regression methods to determine whether a given inmate has one or more RVRs or not. When analyzing the number of RVRs, mixed Poisson models will be used. Because the response with respect to the placement/preliminary score difference is of unknown form, the linearity of the fit will be assessed using a spline approach (a generalized additive model, or GAM) A second gap analyses would fit the likelihood or frequency of RVRs using the preliminary score by including dummy variables for each of the administrative reasons that a subject might have for having a higher placement score. It should be noted that this analysis will partly address concerns raised by Dr. Patricia Hardyman, Ph.D., Association of State Correctional Administrators, about whether the existing preliminary (or placement) score is in need of calibration (redefinition). 3. A crude analysis relating preliminary score, placement score and housing level to the California Department of Corrections and Rehabilitation Office of Research/Research and Evaluation Branch Page 36 December 19, 2011 Inmate Classification System Study likelihood and frequency of RVRs was done using GAMs while the Expert Panel was still devising the analysis plan. This analysis needs to be revisited based on the most current data and with a more deliberate choice of the complexity of the spline functions that are fit to these relationships. Because this analysis merely involves a reworking of existing results, it is a lower priority relative to other (new) analyses. The UCB graduate student will perform the following analyses under the supervision of Dr. Raphael: 1. The regression discontinuity analyses will use local polynomial fitting to estimate the likelihood and frequency of RVRs “in the neighborhood of” one of the current housing level cutoffs in order to estimate the changes in violation levels that would occur if the cutoffs were changed. Close Custody Analyses The UCD Statistician will perform the following analyses: 1. Logistic and Poisson analyses based on longitudinal data. For example, for subjects who are within their first 10 years of Close A Custody these analyses will help to determine whether (and how) violation levels decrease as a function of the number of years in Close A, with the presumption that once those levels have decreased, there is less rationale for retaining the subjects within Close A. The original intent of these analyses had been to have longitudinal data going back 10 years for all subjects, but not all of the variables of interest are available in an electronic format for that length of time. For that reason, the longitudinal data set will include four groups of inmates (lifers, life without possibility of parole, 15 to 49 year sentences, and 50 year plus sentences) who have been in Close A Custody for up to 10 years. In that way, an analysis involving variables that are available for the full 10 years can be based on a static set of subjects, while an analysis involving variables that do not go back that far will be based on within-subject changes in RVR levels over the range of years for which the data are available. To supplement these analyses, Classification Services Unit (CSU) staff will examine twelve years of escape data to identify escapes that are relevant to this study for subsequent qualitative review. In addition, REB staff will perform a literature review on escapes. Step Four: Findings Report The report on findings from the study will have several components. The UCD Statistician and UCB graduate student will provide to REB written technical explanations of their analyses, and will support REB staff in “translating” these technical reports into information that is presentable to a lay person audience. The report outline is as follows: • • • • • • • Introduction Current CDCR Inmate Classification System Initial CDCR Inmate Classification Procedures Subsequent CDCR Inmate Classification Procedures Major Project Goals and Research Questions of This Study Classification Study Team Study Methodology California Department of Corrections and Rehabilitation Office of Research/Research and Evaluation Branch Page 37 December 19, 2011 Inmate Classification System Study • • • • • • • • • • • • • • • Results Discussion (including limitations) Expert Panel Recommendations Implications for Future Research Blueprint (Appendix 1) CDC 839 Classification Score Sheet (Appendix 2) CDC 840 Reclassification Score Sheet (Appendix 3) 180 Housing Design (Appendix 4) 270 Housing Design (Appendix 5) Expert Panel Data Analysis Plan (Appendix 6) UCD Statistical Consultant Data Analysis Plan (Appendix 7) Crosswalk (Appendix 8) Literature Review on Misclassification (Appendix 9) Literature Review on Escape Risk Factors (Appendix 10) Statistical Methodology/Results Technical Report (Appendix 11) Step Five: Presentation to Executive Staff The Expert Panel, UCD Statistician, UCB graduate student, and CDCR REB and CSU staff will present the study findings and recommendations to CDCR Executive staff. Step Six: Training REB Staff Dr. Willits will guide REB staff through the steps of the statistical analyses performed for the study, addressing the following: • Documentation procedures • Quality control checks performed on the data • Writing SAS code • Preparing charts and/or graphs to illustrate analyses • Preparing presentations for a non-technical audience While the focus of the training will be on the current study, it should also provide guidance on conducting statistical analyses, in general. California Department of Corrections and Rehabilitation Office of Research/Research and Evaluation Branch Page 38 December 19, 2011 Inmate Classification System Study Appendix I Summary of Findings from the Regression-Discontinuity Analysis of Inmate Behavioral Outcomes Steven Raphael Goldman School of Public Policy UC Berkeley Sarah Tahamont Goldman School of Public Policy UC Berkeley October 19, 2011 This memo summarizes the results from our empirical analysis of the security classification system currently used by the California Department of Corrections and Rehabilitation (CDCR) and its impact on inmate behavioral infractions. Our primary goal in undertaking this analysis was to assess the net effect of being placed in a higher security level on the likelihood that inmates receive serious rules violation reports (RVRs) of various kinds; a secondary objective is to provide an analysis of the system of mandatory minimum scores used to determine housing level placement for a sizable minority of inmates. To conduct the analysis, we analyze administrative records provided to us by CDCR research staff documenting the behavior of roughly 80,000 inmates over one complete review period during the calendar year 2008. We employ a regression-discontinuity (RD) design framework to isolate the effects of higher security placement on behavioral problems. Our main findings are the following. • • • • Finding 1: We find little evidence that placement in level II institutions suppresses or exacerbates behavioral problems relative to placement in level I institutions for inmates whose preliminary scores are fairly close to the points threshold between levels I and II. Finding 2: We find little evidence that placement in level III institutions suppresses or exacerbates behavioral problems relative to placement in level II institutions for inmates with preliminary scores near the level II/III threshold. Finding 3: We find some evidence that inmates in level IV have worse behavioral outcomes than inmates in level III. In particular, there is some evidence that inmates placed in level IV are more likely to acquire division B, C, or D RVRs over the course of the review period. Again, this finding applies only to those with preliminary scores that are fairly close to the level III/IV threshold. Finding 4: Inmates with binding mandatory minimum placement scores (those with preliminary scores below the mandatory minimum) are quite well-behaved and acquire RVRs at rates that are notably lower than inmates with placement scores that are slightly above or slightly below the mandatory minimum levels across all types of RVRs. California Department of Corrections and Rehabilitation Office of Research/Research and Evaluation Branch Page 39 December 19, 2011 Inmate Classification System Study • Finding 5: The relatively better behavior of inmates with binding mandatory minimums can be explained entirely by their lower average preliminary scores and by the fact that they are older. Our interpretation of these findings is as follows. The lack of an impact of higher security level placement on behavior (and the evidence suggesting possible criminogenic effects of level IV placement relative to level III) suggests that the best prediction for how inmates with scores just above the security level threshold will behave if moved to a lower security level is their behavior at the higher security level. Alternatively stated, these results imply that small changes in the point classification system (such as moving the level II/level III cutoff from 28 to 30) would probably not result in a system-wide increase in behavioral problems. With this interpretation in mind, the relatively good behavior of those with binding mandatory minimum scores is particularly intriguing. The statistical analysis we present below shows that these inmates are relatively older, and have relatively low average preliminary scores that are ratcheted up for the purposes of determining security placement. Given that inmate characteristics readily observable to the CDCR explain their relatively good behavior, we believe that these inmates in particular provide perhaps the best prospects for targeted reforms intended to transfer portions of the inmate population to lower security levels. Regarding policy implications, we believe that the findings support the following. • Small increase in the security level cutoff thresholds will probably not result in large increases in behavioral problems. If CDCR is facing capacity constraints in higher security institutions, small adjustments in these scores should provide relief while not compromising safety. • Inmates facing binding mandatory minimums who have low preliminary scores are particularly good prospects for moving to lower security levels. In fact, the system of mandatory minimums should be rethought and perhaps abandoned in exchange for a system that allows for more case-by-case discretion in establishing minimum security levels rather than blanket administrative determinations with broad applicability. 1. Summary of security classification process and the data analyzed in this memo Security Classification Process - The process determining which security level an inmate will be housed in can be summarized as follows. For new prison admissions, the CDCR collects information on a variety of factors for each new inmate including but not limited to sentence length, age, gang affiliation, past behavioral problems during prior incarceration. Inmates are assigned a preliminary classification score based on background characteristics and prior behavior while incarcerated. The classification tool assigns weights to each of the predictive factors; factors that predict higher criminality are awarded more points (for example, longer sentence length, gang membership, being under 25 years of age). For most inmates, this preliminary score is the final classification score. However, some inmates qualify for a mandatory minimum point allocation. Mandatory minimum points are triggered by certain California Department of Corrections and Rehabilitation Office of Research/Research and Evaluation Branch Page 40 December 19, 2011 Inmate Classification System Study characteristics of the sentence (life without the possibility of parole (LWOP), other life sentences) or of the offense (offenses with violent or sexual component). This initial, or “preliminary score” is then compared to applicable mandatory minimum points and the inmates are assigned a final classification score, or “placement score,” which is the maximum of preliminary score or the mandatory minimum points. For example, an inmate with a preliminary score of 32 who is sentenced to life without the possibility of parole (LWOP) would be assigned a placement score of 52, because the mandatory minimum score (52) is higher than the preliminary score. Whereas, an inmate with a preliminary score of 32 who has an Immigration and Customs Enforcement (ICE) hold would be assigned a placement score of 32, because the mandatory minimum score (19) is lower than the preliminary score. During their incarceration, inmates go through a reclassification process at least once a year. The classification periods consist of 6 month intervals, for the most part each annual review encompasses two review periods. 10 At each reclassification hearing, behavior (good and bad) since the last hearing is reviewed, behavioral problems lead to upward point adjustments and good behavior is rewarded with downward point adjustments to the preliminary score assigned at the previous hearing. Placement scores are still set as the maximum of the new preliminary score and any applicable mandatory minimum. Placement scores generally determine an inmate’s housing security level, although there are many inmates who are housed outside of their security levels due to discretionary administrative placements, pending transfers or housing Custody Designation that effectively preempts the security classification. These administrative placements aside, inmates are assigned to security levels based on the following schema; • • • • Inmates with placement scores of 18 or lower are assigned to level I Inmates with placement scores from 19 to 27 are assigned to level II Inmates with placement scores from 28 to 51 are assigned to level III Inmates with placement scores of 52 or higher are assigned to level IV. The Data - The data that we analyze for this report includes all males housed in a CDCR institution for all of FY08/09 that are not on death row and for whom we can observe a complete review period between reclassification hearings. For each inmate, the data set includes information on all serious RVR’s acquired during the review period, demographic information about each inmate, information regarding sentencing and controlling offense, information on housing and security level, and information on several other personal and institutional characteristics. 10 Occasionally, inmates are reviewed after 1 period (6 months instead of 1 year). California Department of Corrections and Rehabilitation Office of Research/Research and Evaluation Branch Page 41 December 19, 2011 Inmate Classification System Study Key variables in our empirical analysis are the preliminary and placement scores for each inmate. We were provided with three sets of scores. The first are the scores for each inmate one day prior to the beginning of the observed review period. Hence, if a hypothetical inmate’s review period runs from January 1, 2008 through December 31, 2008, the initial set of score apply to this inmate on December 31, 2007 and should determine housing at the beginning of the review period. We also observe new preliminary and placement scores at the beginning of the review period (after the initial January 1, 2008 classification hearing for our hypothetical inmate) as well as the scores at the end of the review period following the terminal point reclassification hearing. Figures 1 and 2 show the empirical relative frequency distributions of the inmates in our analysis sample across preliminary and placement scores. Figure 1 shows the empirical distribution of inmates according to their preliminary score one day prior to the beginning of the review period while figure 2 shows the distribution of corresponding placement score. In both figures inmates with 100 or more points are lumped together as one group. For reference, each figure also shows where the point cutoff levels are between the security housing levels. Figure 1 shows a fairly even distribution of inmates across the preliminary score values. There are large masses of inmates with preliminary scores of zero (nearly 15 percent) and with scores of 100 or more (nearly 10 percent). Figure 2 shows the effects of the system of mandatory minimums on placement scores. There are notable masses of inmates at 19, 28 and 52 points (the points just above the security threshold cutoffs). Nearly 28 percent of the inmates in our sample are constrained at these minimum levels and are unable to move to lower security levels as a result. The lion’s share of the inmates with mandatory minimum scores is constrained at placement scores of 19, effectively keeping them out of level I institutions. Figure 3 shows the distribution of the change in preliminary scores occurring over the review period that we observe. Roughly 80 percent of inmates experience a drop in score between review periods (suggesting that most are fairly well behaved over the course of the year). Tables 1 and 2 present some descriptive statistics pertaining to inmates that are housed in levels I through IV. 11 The offense distributions reveal that inmates with violent controlling offenses inmates are more heavily represented among those in levels III and IV, while inmates with non-violent controlling offenses are more likely to be in housed in lower security levels. This is to be expected, because violent offenses usually carry longer sentence lengths which result in higher preliminary scores. Inmates in higher security levels also tend to be younger, more likely to be serving their first term at a CDCR institution, are more likely to be mentally ill (have an EOP or CCCMS designation) and are more likely to be in a gang. 11 The analysis is limited to inmates housed in levels I-IV. As a consequence, inmates housed in reception centers and other types of housing placements are excluded. California Department of Corrections and Rehabilitation Office of Research/Research and Evaluation Branch Page 42 December 19, 2011 Inmate Classification System Study 2. Methodology Being placed in higher security level housing may impact the incidence of behavioral infractions through several channels. First, the tighter restriction on movement, personal liberties, and time outside of cells that accompanies higher security levels may effectively suppress rules violations among inmates who would otherwise commit such infractions. We refer to this as the “suppression effect” of higher security levels. Alternatively, the average inmate in higher security level institutions is younger, more likely to be convicted for a violent offense, and through the system of classification and reclassification, more likely to have acquired serious RVRs in the past. Being housed with such inmates may increase the likelihood of getting into trouble through peer influences, a higher likelihood of conflict with another inmate, or possibly through an enhancement of anti-social attitudes associated with being housed with a more hardened population. We refer collectively to these potentially criminogenic effects of higher security level placement as “peer effects.” The net effect of higher security level placement will be the sum of the suppression and peer effects and can be either positive or negative. It is this net effect that we seek to measure. A major methodological challenge that we face in measuring such net effects concerns the fact that inmate assignment to alternative security levels is not random; in fact the assignment process assigns those inmates with a high likelihood of poor behavior to higher security institutions. An ideal research design would randomly assign inmates to security levels and then observe their behavior over an evaluation period. Random assignment would ensure that inmate characteristics (both observed and unobserved) are not systematically related to housing security assignment and that any observable differences in behavior between inmates in different security levels could be attributed to difference in housing assignment. Such experimental analysis, of course, would require randomization in the assignment process, a condition that certainly does not describe the process determining security levels that we described above. In the absence of random assignment, we must employ non-experimental empirical methods to estimate the effects of higher security level placement on behavior. Such methods usually involve statistically controlling for observable characteristics in an attempt to isolate the effects of security level, seeking out and exploiting exogenous shocks to security level that generate variation in housing level that is “as good as random,” or some combination of these two approaches. In this project, we exploit the discontinuity in housing level assignment created by the point thresholds to identify exogenous variation in security level assignment. Specifically, as assignment is determined in part by variation in one’s preliminary score, we would expect that inmates who are just above and just below a given points thresholds will experience discretely different treatments in terms of their assigned housing levels. Since inmates with such similar scores are likely to be quite similar to one another, and as we are able to model the general relationship between bad behavior and preliminary score, any discontinuous change in behavior occurring around the point thresholds can be attributable to the corresponding change in the housing security level. California Department of Corrections and Rehabilitation Office of Research/Research and Evaluation Branch Page 43 December 19, 2011 Inmate Classification System Study This research design depends crucially on there being discontinuous treatment at the points cutoffs, but also requires that all other variables that may determine behavioral infractions (age, offense history, risk scores etc) vary continuously through the cutoffs. These two requirements relate to the study design in the following manner. Regarding the first requirement, the larger the proportion of inmates experiencing a change in housing assignment as we move through a cutoff, the more precise our estimates of the effect of housing security level on behavior will be. For example, a relatively large difference in treatment (e.g. assignment to level II) between inmates with scores of 18 relative to inmates with scores of 19 makes it more likely that we would detect an effect of housing level on behavior if it exists and would also improve the precision (the margin of error) of our estimate of this net behavioral effect. The second requirement (that all other variables vary continuously through the cutoffs) basically ensures that there are not large differences in observable or unobservable characteristics between inmates just above and just below points thresholds and that any discrete change in behavior can be attributable to the change in housing security level. In conducting our analysis, a key design choice that we face is whether to use preliminary score or placement score as the key running variable for our regression-discontinuity design. Placement score has the advantage that it is more predictive of housing security level than preliminary score, since many inmates have placement scores that differ from their preliminary scores due to a binding mandatory minimum. In other words, the predictive power of the point thresholds in determining security level is greater with placement than preliminary score. A key weakness of using placement score, however, is that through the mandatory minimum system those inmates just above a threshold are notable and discretely different from those inmates just below a threshold. In particular, inmates above the thresholds via placement scores have key differences in sentences and controlling offense (more likely to be LWOP, a convicted sex offender etc) and, as we will show later, have much lower average preliminary scores than those with placement scores just below. For these reasons, our analysis will be based on variation in housing security level assignment associated with preliminary score before mandatory minimums are factored in. While the predictive power of the point thresholds in determining security level assignment is lower, there are no identifiable selection mechanisms that create large discontinuities in inmate characteristics around point thresholds when points are measured with preliminary score. Figure 4 displays the proportion of inmates housed in each security level at the beginning of the review period by their preliminary score measured just prior to the beginning of the review period. The proportion of inmates with preliminary scores that would place them in level I (less than 19) that are actually housed in level I is quite small (under 0.2). This is not too surprising since figure 2 shows a very large proportion of inmates with binding mandatory minimum scores of 19. Nonetheless, as we pass through the level I/level II point threshold, the proportion in level I drops by roughly 0.1, while the proportion in level II California Department of Corrections and Rehabilitation Office of Research/Research and Evaluation Branch Page 44 December 19, 2011 Inmate Classification System Study increases. Interestingly, a small proportion of inmates with level II points are housed in level I institutions (roughly 0.1 to 0.15). The biggest discontinuities in security level assignment are observed at the level II/III threshold and the level III/IV threshold. As preliminary score crosses the level II/III threshold (from 27 to 28 points) the proportion housed in level II institutions drops by nearly 0.4, while the proportion housed in level III increases by a similar amount. Similarly, as preliminary score crosses the level III/IV point threshold, the proportion housed in level III drops by roughly 0.6 while the proportion housed in level IV increases. We estimate the effect of being assigned to higher security levels on the RVR incidence by statistically testing for breaks in the relationship between the proportion of inmates with RVRs and preliminary score as at the point thresholds between levels. For example, in Figure 4 we observe a large increase in the proportion of inmates in level III when the preliminary score passes from 27 to 28 points. If level III suppresses behavior relative to level II we should see a discrete downward shift in the relationship between preliminary score and the proportion with RVRs at 28 points. Conversely, if being placed in higher security levels is criminogenic, we should see a discrete upward shift in this relationship at 28 points. To formally illustrate this test, suppose we wish to estimate the net effect of placement in level IV relative to level III. Define the variable Pi as the preliminary score for inmate i defined relative to the level III/level IV cutoff, where Pi =0 when preliminary score equals 52, Pi =1 when preliminary score =53, Pi =-1 when preliminary score equals 51 and so on. Define the variable GE52i as an indicator variable equal to one for inmates with preliminary scores greater or equal to 52. Finally, define RVRi as an indicator variable equal to one if the inmate acquires an RVR over the review period. Restricting the sample to inmates with preliminary scores that would place them in level III or level IV (i.e., preliminary scores greater than 27), one would test for a structural break in the RVR incidence at 52 points by estimating the equation (1) RVRi = α + βPi + δPi + γGE 52i + λPi ∗ GE 52i + κPi ∗ GE 52i + ε i 2 2 where we allow for a quadratic relationship between the RVR incidence and preliminary score that is permitted to differ above and below the point threshold via the inclusion of two interaction terms. The parameters to be estimated are α, β, δ, γ, λ, and ĸ, and ε i is an error term. The parameter γ provides an estimate of the discrete change in the RVR incidence occurring at 52 points. Our principal empirical test essentially involved testing whether this parameter is statistically and significantly different from zero. A finding of γ > 0 implies a net criminogenic effect of being in level III while a finding of γ < 0 implies a net suppression effect. Below we estimate various specifications of equation (1) using several alternative measure of rules violations and omitting and including a large set of observable covariates. California Department of Corrections and Rehabilitation Office of Research/Research and Evaluation Branch Page 45 December 19, 2011 Inmate Classification System Study Equation (1) provides a reduced-form estimate of the effect of higher security placement on RVR incidence since the coefficient γ is basically the product of the effect of having 52 points or more on the likelihood of being placed in level IV and the effect of level IV placement on the RVR incidence. If the discontinuity in assignment at 52 points were sharp, meaning that all 52s are assigned to level IV while all 51s are assigned to level III, then γ would be interpretable as the effect of level IV assignment on behavior problems. However, since the discontinuity in assignment at 52 points is less than complete (in the parlance of non-experimental analysis, the RD is fuzzy), estimating the effect of being placed in level IV on the RVR incidence requires that we scale the estimate γ by the effect of being over 52 points on the likelihood of being placed in level IV. To do so, we estimate the following two-equation system using two-stage least squares LevelIVi = φ0 + φ1 Pi + φ2 Pi + νGE 52i + θ 0 Pi ∗ GE 52i + θ1 Pi ∗ GE 52i + η i 2 (2) 2 RVRi = α + βPi + δPi + γLevelIVi + λPi ∗ GE 52i + κPi ∗ GE 52i + ε i 2 2 where the variable LevelIVi is an indicator variable for being housed in a level IV facility and all other variables are as defined above. The two equation system in (2) employs the dummy variable GE52i as an instrumental variable for being housed in level IV. In other words, the estimated effect of level IV housing on RVR incidence measured by the parameter γ makes use of the variation in the proportion assigned to level IV caused by crossing the point threshold at 52. We estimate various specifications of equation (1) and the two-equation system in (2) for sub-samples of the data for the three thresholds between level assignments. For all models, we restrict the sample to inmates housed in state who are in level I through level IV housing (i.e., we drop inmates in reception centers or in other types of housing). To estimate the effect of level II placement relative to level I, we restrict the analysis to inmates with preliminary scores below 28 points. To estimate the effect of level III placement relative to level II placement, we restrict the analysis to inmates with preliminary scores greater than 18 but less than 52 points. Finally, to estimate the effect of placement in level IV relative to level III we restrict the analysis sample to inmates with preliminary scores that are greater than 27 but less than or equal to 70. Before proceeding to the estimation results, we must note an important caveat. The estimates presented below should be interpreted as the effects of higher level placement on RVR incidence for inmates with preliminary scores that put them “in the neighborhood” of the point threshold between security levels. That is to say, our estimate of the effect of level IV placement on RVR incidence applies to inmates with, say, 50 to 53 or 54 points on their preliminary score. The research design basically employs those inmates below the threshold as a control group for those slightly above, and uses the overall relationship between RVR incidence and preliminary score to project what RVRs would be if those above the threshold were assigned to a lower security level. The result does not apply to an inmate with a preliminary score of, for example, 100 points. Such an inmate is sufficiently far from the California Department of Corrections and Rehabilitation Office of Research/Research and Evaluation Branch Page 46 December 19, 2011 Inmate Classification System Study discontinuity threshold that extrapolations of an effect estimates for an inmate with 52 points is likely to be erroneous. Throughout the analysis to follow, we estimate the effect of security placement on the incidence of any serious RVR (defined as an A through F violation), acquiring an A1 or A2 violation, acquiring a B, C, or D violation, or acquiring and E or F violation. In separate results not reported here, we also tested for impacts of security level assignment on the number of RVR’s acquired over the course of the review period. The results are qualitatively and quantitatively similar to what we find for the more simple RVR incidence outcomes. 3. Results of RD analysis using preliminary score As our description of the data indicated, we have several alternative measures of preliminary scores that differ in terms of the date of measurement. Specifically, we observe the preliminary score coming into the review period (i.e., the score prior to the commencement of the review period). We also observe the preliminary score after the reclassification hearing at the beginning of the review period as well as the preliminary score after the reclassification hearing at the end of the review period. The last preliminary score is clearly inappropriate as it will reflect behavior over the observation period and may or may not coincide with one’s housing security level over the course of the review. The first observed preliminary score (measured prior to the beginning of the review period) provides the best predictor of where inmates are housed at the beginning of the review period. However, as many inmates improve their scores from one period to the next, many will spend much of the review period housed at a level that is different from where they are at the beginning of the review period. For example, an inmate with 55 points coming into the review period who has 51 points after the reclassification hearing at the beginning of our data will likely move down to level III shortly thereafter, and may spend the majority of his time during the observed review period at the lower level. Hence, for many inmates the preliminary score after the beginning of the review period provides a better predictor of where they do their time over the course of the review. In this section, we provide estimates using both preliminary scores. Using the latter score requires that we adjust our measurement of where the inmate is housed in the two-stage least squares analysis to account for those who move levels during the course of the review period. We discuss the details of this adjustment with the discussion of the results below. Figure 5 graphically displays the reduced-form RD analysis using the preliminary score coming into the review period (that is to say, prior to the reclassification hearing at the beginning of our data). Each figure plots the proportion of inmates that acquire an RVR by their preliminary score value. The figures also fit separate quadratic relationships allowing for breaks at the point thresholds between the security levels. We present results for any RVR, an A1/A2 violation, a B/C/D violation, or an E/F violation. The data generally show a positive relationship between preliminary score and RVR incidence, especially for A1/A2 violations and B/C/D violations. There are no visible breaks in the RVR incidence levels around the point thresholds, suggesting little evidence of an effect of higher security placement on RVRs for those near the cutoffs. This is particular notable for the level II/level California Department of Corrections and Rehabilitation Office of Research/Research and Evaluation Branch Page 47 December 19, 2011 Inmate Classification System Study III and level III/level IV thresholds given the large change in housing assignment at these thresholds documented in Figure 4. Table 3 presents the results from formal tests for discontinuity in RVR incidence at the point thresholds via estimation of equation (1) above. For each of the four RVR outcome variables, we estimate the break associated with being above the threshold omitting covariates from the analysis as well as including controls for offense, type of sentence, number of prior incarcerations served with CDCR, age, race/ethnicity, gang affiliation and length of the review period. The table only reports the coefficient on the dummy indicating being above the threshold (corresponding to γ in equation (1) above). Note: a positive significant estimate of γ would be evidence of a net criminogenic effect while a negative significant estimate of γ would be evidence of a net suppression effect). The table presents separate estimate for the three point thresholds. There is no evidence of significant changes in RVR incidence across the level I/II threshold (results in Panel A) or the level II/III threshold (results in Panel B). There is weak evidence of a positive effect of being above the level III/IV threshold for having acquired any RVR. In the model with no covariates, the positive coefficient is statistically significant at the ten percent level of confidence. This effect, however, becomes insignificant once covariates are added to the specification. All of the other coefficients in Panel C are near zero and statistically insignificant. Since none of the reduced form estimates based on estimation of equation (1) are significant, we do not present further analysis using the two-stage least squares model in equation (2) using this earliest measured preliminary score. As we noted in the introduction to this section, the preliminary score prior to the beginning of the review period provides the best predictor of housing security level at the beginning of the review period. However, it does not provide the best predictor of where inmates do their time during the review period for the many inmates slated to move after the reclassification hearing that initiated the review period. To see this, Figure 6 graphs the proportion of inmates that change security levels during the review period. 12 Roughly twelve percent of inmates change security levels, though these twelve percent are certainly not randomly distributed across the preliminary score distribution. The first figure in figure 6 shows the proportion changing security levels by the preliminary score prior to the beginning of the review period. The proportion moving is particular high above the level II/III threshold and above the level III/IV threshold. When movers are disaggregated into those who move up in security level and those who move down, we see bunching of those who move up below the point thresholds and bunching of those who move down above the point threshold. 12 We were provided with administrative records on all facility moves for all inmates in our sample during the review period. The data includes the date of the move and the security level of the new institutions. California Department of Corrections and Rehabilitation Office of Research/Research and Evaluation Branch Page 48 December 19, 2011 Inmate Classification System Study Tables 4 and 5 provide additional information on inmates that change security levels over the course of the review period. Tables 4 shows key percentiles from the empirical distribution of a variable measuring the number of days between the initiating reclassification hearing and the move date for those inmates that move. The median mover changes security levels within three months of their hearing, with those moving up taking slightly longer than those moving down. Most moves occur in within six months of the reclassification hearings. Table 5 shows that most moves occur between consecutive levels, although we do see some inmates skip levels in both downward and upward movements. These movers complicate the analysis and raise questions about the results using the earliest measured preliminary scores displayed in Table 3. To be specific, many of those who are predicted to be in higher security levels based on the earliest preliminary score actually spent much of their time in lower security levels due to point deductions at the beginning of the review period. In essence, the initial analysis may be misclassifying the housing conditions for inmates near the threshold and drawing incorrect inference as a result. Moreover, given the large proportions that move near the threshold, this may be a particularly severe problem. To address this issue, we re-estimate the RD models using the preliminary score measured after the beginning of the review period. To situate these estimates within the two-stageleast-squared framework we also need to alter our characterization of their housing assignment during the review period. We do so in the following manner. For inmates that move during the review period, we measure the proportion of time that they spent in each level. Hence, an inmate that spends one month in level IV and eleven months in level III during a twelve-month review period is measured as serving 1/12 of his time in level IV and 11/12 of his time in level III. By contrast, non-movers that spend the whole review period in level IV would be assigned a value of one for proportion of time spent in level IV and values of zero for proportion of time spent in other security levels. Figure 7 displays the relationship between the proportion of time spent in each level and preliminary score measured after the beginning of the review period. The discontinuity at the level I/II threshold becomes even murkier than that observed in Figure 4. However, we still see notable and sizable discontinuities in the proportion of time spent in level III at the level II/III threshold and the proportion of time spent in level IV at the level III/IV threshold. Hence, the test for discontinuities in behavior will be sharpest for these higher thresholds when we use the later preliminary score as the running variable for the RD analysis. Figure 8 graphs the incidence of RVRs against preliminary score measured after the beginning of the review period. Each data point provides the proportion of inmates with the particular preliminary score that acquire an RVR over the review period. Again, we fit quadratic functions to this relationship that allow for breaks (both in terms of intercept and slope) at the security level thresholds. The figures reveal little evidence of discrete increases or decreases in RVR incidence at the level I/II and level II/III thresholds. However, we do observe an increase in the incidence of any RVR and in B/C/D/ RVRs at the level III/IV threshold. California Department of Corrections and Rehabilitation Office of Research/Research and Evaluation Branch Page 49 December 19, 2011 Inmate Classification System Study Table 6 present formal tests for discontinuous breaks in RVR incidence at the threshold from estimates of the model laid out in equation (1). Within each panel, we first present estimates from models with no covariates. We then present estimates from models that add observable covariates on sentencing, offense, age and other personal characteristics. The final specification adds dummy variables for whether the inmate is an up-mover or down-mover. Again, we only present estimation results for the coefficient γ from equation (1). Beginning with the results for the level I/II cutoff, we see a slight but significant increase in RVRs for those above the cutoff for the any RVR outcome and for the B/C/D outcome. We will see, however, that the first-stage relationship between being above the threshold and assignment to level II is quite weak, and hence we do not place great confidence in this particular finding. The results for the level II/III cutoff in panel B show significant increases in RVR incidence for those above the threshold for any RVR and for E/F violations when we do not control for whether one is an up or down-mover. Adding controls however eliminates these effects, yielding an estimate of zero consistent with the results from the prior analysis using the earliest measured preliminary score. We should note that the first-stage relationship between being above the level II/III threshold and proportion of review period spent in level III is quite strong, and hence, we have more confidence in these estimates than those presented in panel A. Panel C presents the estimation results for the level III/IV threshold. Here we see consistent evidence of a significant increase in the incidence of any RVR and B/C/D RVRs associated with being slightly over the point threshold. The most complete specification suggests that those just over the thresholds are 3.5 percentage points more likely to acquire an RVR during the review period and 3.2 percentage points more likely to acquire a B/C/D RVR. Table 7 presents two-stage-least-squares estimates of the effect of serving time in a higher security level on RVR incidence using the two-equation model in (2) above. Here we present results only for the most complete mode specification from Table 7 (inclusive of all covariates and controls for having moved up or down in security levels). For each set of models, we report the F-statistic testing the significance of the instrumental variable in the first-stage regression model. As is evident, the instrument is quite weak in predicting the time spent in level II for inmates with preliminary scores surrounding the level I/II threshold. For this reason, we do not discuss these results further. However, the first-stage relationships in predicting time spent in level III for the level II/III models and for time spent in level IV for the level III/IV models are quite strong. Consistent with the reduced-form estimates in Table 7, we find no evidence of an impact, positive or negative, of assignment to level III as opposed to level II on RVR incidence. On the other hand, the results in panel C suggest that assignment to level IV increases the likelihood of acquiring any RVR by 9 percentage points, with this effect being driven entirely by an increase in the likelihood of a B, C, or D rules violation. California Department of Corrections and Rehabilitation Office of Research/Research and Evaluation Branch Page 50 December 19, 2011 Inmate Classification System Study In additional analysis that we do not report here, we re-estimated all models dropping movers from the analysis and using the preliminary score after the initiating reclassification hearing as the running variable. The results from this model yield no evidence of either peer or suppression effects for at the level I/II cutoffs and level II/III cutoffs, and some mixed evidence suggestive of a criminogenic effect of being placed in level IV. Overall, the analysis finds little evidence that the behavior near the security level thresholds differs depending on which side of the threshold one falls, although some of the results are indicative of a positive causal effect of level IV placement on RVR incidence. These results lead to us to the general conclusion that for those within the neighborhood of the security level thresholds, the average behavior of those with placement scores slightly above the cutoff is the best predictor of how they would behave should they be moved to the next lowest security level. 4. Mandatory minimums In our analysis of the effect of housing security level on behavioral outcomes, we made the deliberate research design choice to use preliminary scores as the running variable in the RD analysis rather than placement score. The reasoning behind this choice is that the administrative rules determining placement score are likely to create discontinuous breaks at the security level thresholds in observable characteristics, such as controlling offense and mean preliminary score, and perhaps unobservable characteristics, such as unobserved propensity towards violence or willingness or ability to follow orders. An important unobservable or perhaps unquantifiable factor that may also change for those bound by a mandatory minimum concerns the incentives to behave well. To the extent that moving to lower security levels is desirable, inmates bound by a mandatory minimum face less of an incentive than inmates not bound by mandatory minimums to comply with institutional rules and to engage in positive programming. In this section, we explore the relative behavioral outcomes of inmates bound by mandatory minimum point assignments. In particular we reanalyze the RD models using placement score as the running variable to highlight the relatively better behavioral outcomes for inmates with binding mandatory minimum constraints and assess whether this better behavior can be explained by observable characteristics. 13 13 Inmates with mandatory minimum point assignments fall into one of the following categories: Condemned – minimum score of 52, Life without Parole (LWOP) – minimum score of 52, CCR3375.2(a)(7) Life Inmate with special circumstances (multiple life sentences, torture, execution style) – minimum score of 28, History of Escape – minimum score of 19, Warrants “R” Suffix (Sex Offender) – minimum score of 19, Violence Exclusion – minimum score of 19, Public Interest Case – minimum score of 19, and other Life Sentence – minimum score of 19. California Department of Corrections and Rehabilitation Office of Research/Research and Evaluation Branch Page 51 December 19, 2011 Inmate Classification System Study By construction, placement and preliminary score will be the same for inmates not facing mandatory minimum and for inmates with mandatory minimums whose preliminary scores exceed their placement scores. However, for inmates who over time have improved their scores through good behavior, mandatory minimums will eventually become binding. Figure 9 displays this fact. The figure presents a plot of the average preliminary score of all inmates in our sample by single placement score values (using preliminary and placement score measured prior to the initiating review period). 14 For inmates with placement scores not equal to 19, 28, or 52, average preliminary score equals placement score. For inmates above at the point thresholds however, average preliminary scores are discretely and substantially lower relative inmates with slightly higher and slightly lower placement scores. Note: Figure 2 revealed that a substantial minority of inmates has placement scores at these mandatory minimum levels (especially at 19). In essence, the scores of these inmates continue to improve with time, yet it is not reflected in the one score that actually determines security level placement. To be sure, this would not be an issue if placement score (inclusive of the mandatory minimums) was more predictive of behavioral problems than preliminary score. Of course, this is a question that we can explore. Figure 10 presents a scatter plot of the proportion of inmates that acquire an RVR over the review period by placement scores measured prior to the beginning of the review period. For reference, we have placed circles around the data points for inmates with placement scores of 19, 28, or 52. For all of the RVR outcomes displayed in the figures, inmates with placement scores at the mandatory minimums behave discretely better than inmates with slightly higher placements as well as inmates with slightly lower placement scores. Notably, the inmates bound at the higher minimums (28 and 52) are among the best behaved relative to all other placement score levels. Figure 11 reproduces these figures using placement score measured after the reclassification hearing at the beginning of the review period. The patterns are nearly identical. These patterns beg the question of why inmates with binding mandatory minimums behave better. Certainly, the incentives associated with moving to a lower security level cannot explain this difference, as improvements in preliminary score cannot alter their housing arrangements. It is possible that persistent good behavior is driven by an incentive stay at the lowest possible Custody Designation, which determines many of the conditions of confinement within security level. It is also possible that some aspect of being placed under a mandatory minimum acts to suppress behavior in a manner difficult to observe in administrative data. An additional possibility is that differences in observable characteristics such as preliminary score and age explain the better behavior of inmates trapped at these minimum placement scores. To the extent that this is the case, a clear implication would be that the assignment process is ignoring useful information that is predictive of inmate behavioral outcomes in following a rigid set of administrative placement rules. 14 This figure looks similar regardless of the scores used. California Department of Corrections and Rehabilitation Office of Research/Research and Evaluation Branch Page 52 December 19, 2011 Inmate Classification System Study To explore this question, we first apply the RD equation (1) to the data in Figures 10 and 11 using placement score as the running variable. The discontinuity here provides a formal empirical estimate of the size and statistical significance of the difference in behavior of those with mandatory minimum scores relative to other inmates. Given the patterns in the figures we expect the structural break coefficients to be negative, sizable, and statistically significant. Next, we assess whether controlling for observable characteristics explains these differentials. Specifically, we re-estimate the models using several alternative sets of covariates and assess whether doing so eliminates the significant breaks at the point thresholds. The first specification adds controls for preliminary score and age (both entered as quadratic control functions) to the specification of equation (1). The second specification includes the more complete set of covariates employed in our regression analysis above. The one departure from equation (1) is that we control for the running variable as a cubic polynomial interacted with being above the point threshold. We make this modification due to the sharper discontinuities in behavior that we observe in the data and due to specification tests suggesting that a cubic polynomial provides a better overall fit. Table 8 presents the results of this analysis using placement score measured prior to the beginning of the review period. Beginning with the results for the level I/II cutoff in panel A, the first row of estimates provides the structural break estimates for each RVR dependent variable when no controls are included in the specification other than the cubic polynomial in the running variable, the dummy for being above the threshold, and the interaction term between the threshold dummy and the placements score polynomial terms. 15 The second row provides the estimates of the structural break in behavior controlling for variation in preliminary score and age. The final row adds all covariates to the model (the set of additional explanatory variables are described in the notes to the table). For the level I/II cutoff, the results with no covariates show large decreases in RVR incidence for those above the threshold. Controlling for preliminary score and age explains nearly half of this break (as is evident by the sharp decline in the absolute value of the coefficients). Adding all explanatory variables yields estimates of the structural break that are statistically insignificant. The results for the level II/III threshold are even more pronounced. The results for the model without control variables find that RVR incidence declines by 14 percentage points for those just above the thresholds (with this estimate statistically significant at the one percent level of confidence). Controlling for preliminary score and age (the results in the next row) explains all of this decline, as can be seen in the large decline in the absolute value of the coefficient estimate that also becomes statistically insignificant. Similar results are observed when we look at specific types of RVRS. 15 Similar to our previous analysis, we measure placement score relative to the point threshold. This permits interpreting the coefficient on the structural break as the magnitude of the break in the dependent variable as we move though the threshold. California Department of Corrections and Rehabilitation Office of Research/Research and Evaluation Branch Page 53 December 19, 2011 Inmate Classification System Study Finally, we find similar patterns at the level III/IV threshold. Being above the threshold is predicted to result in a 13 percentage point decline in the proportion of inmates with an RVR. Controlling for preliminary score and age completely explains away this relationship. Again, similar patterns are observed when we look at specific types of RVRs. To be complete, Table 9 reproduces these results using placement score measured after the beginning of the review period. The findings are qualitatively identical and quantitatively comparable. 0 Proportion of inmates .05 .1 .15 Figure 1: Empirical Distribution of Preliminary Scores One-Day Prior to the Beginning of the Review Period 0 20 40 60 Preliminary Score California Department of Corrections and Rehabilitation Office of Research/Research and Evaluation Branch 80 100 Page 54 December 19, 2011 Inmate Classification System Study 0 .05 Proportion of inmates .1 .15 .2 Figure 2: : Empirical Distribution of Placement Scores One-Day Prior to the Beginning of the Review Period 0 20 40 60 Placement Score California Department of Corrections and Rehabilitation Office of Research/Research and Evaluation Branch 80 100 Page 55 December 19, 2011 Inmate Classification System Study 0 .1 Proportin of inmates .2 .3 .4 Figure 3: Distribution of Inmates by Change in Preliminary Score over the Review Period Covered by the Data -10 10 20 0 Change in preliminary score over the review period California Department of Corrections and Rehabilitation Office of Research/Research and Evaluation Branch 30 Page 56 December 19, 2011 Inmate Classification System Study Figure 4: Proportion of Inmates Housed in Each Security Level at the Beginning of the Review Period by Placement Score Measured One-Day Prior to the Beginning of the Review Period California Department of Corrections and Rehabilitation Office of Research/Research and Evaluation Branch Page 57 December 19, 2011 Inmate Classification System Study 60 20 40 Preliminary Score 80 0 60 20 40 Preliminary Score 80 Proportion with an A1A2 0 .01 .02 .03 .04 0 0 40 60 20 Preliminary Score 80 0 40 60 20 Preliminary Score 80 Proportion with any EF .05 .1 .15 .2 Proportion with any BCD .05 .1 .15 .2 Proportion with any RVR .1 .15 .2 .25 .3 .35 Figure 5: Incidence of Serious Rules Violation Reports by Preliminary Score Measured One Day Prior to the Beginning of the Review Period California Department of Corrections and Rehabilitation Office of Research/Research and Evaluation Branch Page 58 December 19, 2011 Inmate Classification System Study Figure 6: Proportion of Inmates Changing Housing Security Levels Over the Review Period by Preliminary Score Measured One Day Prior to the Beginning of the Review Period California Department of Corrections and Rehabilitation Office of Research/Research and Evaluation Branch Page 59 December 19, 2011 Inmate Classification System Study Figure 7: Proportion of Review Period Spent in Each Housing Level by Preliminary Score Measured After the Start of the Review Period California Department of Corrections and Rehabilitation Office of Research/Research and Evaluation Branch Page 60 December 19, 2011 Inmate Classification System Study 20 40 60 Preliminary Score 80 0 20 40 60 Preliminary Score 80 Proportion with an A1A2 0 .01.02.03.04.05 0 0 40 60 20 Preliminary Score 80 0 20 40 60 Preliminary Score 80 Proportion with any EF .1 .15 .2 .25 Proportion with any BCD .05 .1 .15 .2 .25 Proportion with any RVR .1 .15 .2 .25 .3 .35 Figure 8: Incidence of Serious Rules Violation Reports by Preliminary Score Measured After the Beginning of the Review Period California Department of Corrections and Rehabilitation Office of Research/Research and Evaluation Branch Page 61 December 19, 2011 Inmate Classification System Study 0 Average Preliminary Score 20 40 60 80 Figure 9: Average Preliminary Score by Placement Score Measured Prior to the Beginning of the Review Period 0 20 40 Placement Score California Department of Corrections and Rehabilitation Office of Research/Research and Evaluation Branch 60 80 Page 62 December 19, 2011 Inmate Classification System Study 20 40 60 Placement Score 80 0 60 20 40 Placement Score 80 Proportion with an A1A2 0 .01 .02 .03 .04 .05 0 0 20 40 60 Placement Score 80 0 40 20 60 Placement Score 80 Proportion with any EF .1 .15 .2 .25 Proportion with any BCD 0 .05 .1 .15 .2 Proportion with any RVR .1 .15 .2 .25 .3 .35 Figure 10: RVR Incidence by Placement Score Measured One Day Prior to the Beginning of the Review Period California Department of Corrections and Rehabilitation Office of Research/Research and Evaluation Branch Page 63 December 19, 2011 Inmate Classification System Study 20 40 60 Placement Score 80 0 20 40 60 Placement Score 80 Proportion with an A1A2 0 .01.02.03.04.05 0 0 60 20 40 Placement Score 80 0 20 40 60 Placement Score 80 Proportion with any EF .1 .15 .2 .25 .3 Proportion with any BCD 0 .05 .1 .15 .2 .25 Proportion with any RVR .1 .2 .3 .4 Figure 11: RVR Incidence by Placement Score Measured After the Beginning of the Review Period California Department of Corrections and Rehabilitation Office of Research/Research and Evaluation Branch Page 64 December 19, 2011 Inmate Classification System Study Table 1: Controlling Offense Distribution of Inmates in our Analysis Sample by Housing Security Level Level I Level II Level III Level IV Murder 0.00 0.26 0.20 0.39 Manslaughter 0.01 0.02 0.04 0.04 Robbery 0.21 0.09 0.18 0.20 Assault 0.10 0.13 0.15 0.14 Sex Offense 0.00 0.20 0.15 0.07 Kidnapping 0.00 0.04 0.02 0.03 Burglary 0.13 0.06 0.07 0.05 Larceny 0.07 0.02 0.02 0.01 Vehicle theft 0.05 0.01 0.01 0.01 Forgery/Fraud/Other Property 0.02 0.01 0.01 0.00 Drugs 0.32 0.11 0.09 0.04 DUI 0.04 0.01 0.00 0.00 Other 0.05 0.03 0.04 0.02 Proportion of regularly-housed inmates 0.07 0.25 0.39 0.29 California Department of Corrections and Rehabilitation Office of Research/Research and Evaluation Branch Page 65 December 19, 2011 Inmate Classification System Study Table 2 Average Characteristics by Housing Security level Level I Level II Level III Level IV Age 38.15 43.27 39.07 35.81 First stay 0.313 0.594 0.546 0.575 EOP/CCCMS 0.056 0.168 0.277 0.303 Gang 0.086 0.045 0.172 0.209 48 72 108 72 96 148 84 144 192 144 204 288 Black 0.333 0.292 0.312 0.365 White 0.301 0.267 0.243 0.173 Hispanic 0.324 0.365 0.373 0.400 Sentence P25 P50 P75 California Department of Corrections and Rehabilitation Office of Research/Research and Evaluation Branch Page 66 December 19, 2011 Inmate Classification System Study Table 3 RD Estimates of the Effects of Being Above the Security Classification Threshold on the Likelihood of Serious Rules Violations During the Review Period Using Preliminary Score One Day Prior to Review Period Panel A: Level I/Level II Threshold Any RVR A1/A2 B/C/D E/F No Covariates 0.010 -0.002 0.008 0.009 (0.015) (0.003) (0.009) (0.013) Covariates 0.005 -0.002 0.007 0.005 (0.014) (0.003) (0.009) (0.013) Panel B: Level II/Level III Any RVR A1/A2 B/C/D E/F No Covariates -0.015 0.006 -0.006 -0.022 (0.021) (0.005) (0.015) (0.018) Covariates -0.018 0.005 -0.010 -0.023 (0.020) (0.005) (0.015) (0.017) Panel C: Level III/Level IV Any RVR A1/A2 B/C/D E/F c No Covariates 0.033 0.002 0.004 0.016 (0.018) (0.005) (0.013) (0.015) Covariates 0.028 0.001 0.002 0.015 (0.017) (0.005) (0.013) (0.015) Standard errors are in parentheses. The figures in the table provide the coefficient from a dummy variable indicating a preliminary score (measured one day before the beginning of the review period) above the security classification threshold for the comparison indicated. All models include a quadratic function in preliminary score interacted with the threshold cutoff. The models with covariates also include thirty two offense dummies, dummy variables indicating the time of sentence (second-strike, third-strike, LWOP, determinate), dummy variables indicating first, second, third, or fourth or higher stay with CDCR, a quadratic in age, seven race/ethnicity dummies, a dummy indicating documented gang affiliation, and a linear measure of the length of the review period. a. Statistically significant at the one percent level of confidence. b. Statistically significant at the five percent level of confidence. c. Statistically significant at the ten percent level of confidence. California Department of Corrections and Rehabilitation Office of Research/Research and Evaluation Branch Page 67 December 19, 2011 Inmate Classification System Study Table 4 Distribution of the Days Between the Beginning of the Review Period and the Move Date for Inmates that Changes Housing Custody Levels During the Study Period Percentiles All Movers Those who move up Those who move down 10th 23 24 23 th 25 46 59 42 Median 88 115 76 75th 154 174 135 th 90 227 262 196 Tabulations based on the 9,747 identified inmates that move custody levels and that have complete information on the custody level at the new institution. California Department of Corrections and Rehabilitation Office of Research/Research and Evaluation Branch Page 68 December 19, 2011 Inmate Classification System Study Table 5 Distribution of Inmates Who Change Security Levels During the Review Period by Origin and Destination Security Level Destination Security Level Origin Security I II III IV Level I 0 957 319 36 II 799 0 1,509 78 III 530 2,345 0 967 IV 67 40 2,100 0 Tabulations based on the 9,747 identified inmates that move custody levels and that have complete information on the custody level at the new institution California Department of Corrections and Rehabilitation Office of Research/Research and Evaluation Branch Page 69 December 19, 2011 Inmate Classification System Study Table 6 RD Estimates of the Effects of Being Above the Security Classification Threshold on the Likelihood of Serious Rules Violations During the Review Period Using Preliminary Score After the Beginning of the Review Period Panel A: Level I/Level II Threshold Any RVR A1/A2 B/C/D E/F a b No Covariates 0.038 0.001 0.022 0.009 (0.015) (0.004) (0.009) (0.013) b b Base Covariates 0.037 0.001 0.021 0.007 (0.015) (0.004) (0.009) (0.013) Plus indicators 0.037a 0.007 0.021b 0.008 for up and down (0.015) (0.004) (0.009) (0.013) moves Panel B: Level II/Level III Any RVR A1/A2 B/C/D E/F a No Covariates 0.063 0.004 0.028 0.039b (0.023) (0.006) (0.017) (0.019) Base Covariates 0.052 0.005 0.022 0.031 (0.022)b (0.006) (0.017) (0.019)c Plus indicators 0.026 0.005 0.010 0.015 for up and down (0.022) (0.006) (0.017) (0.019) moves Panel C: Level III/Level IV Any RVR A1/A2 B/C/D E/F a a No Covariates 0.077 0.002 0.047 0.033b (0.019) (0.005) (0.015) (0.015) Base Covariates 0.064a 0.005 0.043a 0.020 (0.018) (0.005) (0.014) (0.015) Plus indicators 0.035c 0.003 0.032b -0.000 for up and down (0.019) (0.006) (0.015) (0.016) moves Standard errors are in parentheses. The figures in the table provide the coefficient from a dummy variable indicating a preliminary score (measured one day before the beginning of the review period) above the security classification threshold for the comparison indicated. All models include a quadratic function in preliminary score interacted with the threshold cutoff. The models with base covariates also include thirty two offense dummies, dummy variables indicating the time of sentence (second-strike, third-strike, LWOP, determinate), dummy variables indicating first, second, third, or fourth or higher stay with CDCR, a quadratic in age, seven race/ethnicity dummies, a dummy indicating documented gang affiliation, and a linear measure of the length of the review period. The final models add indicator variables for inmates that move up in security levels and inmates that move down in security levels. a. Statistically significant at the one percent level of confidence. b. Statistically significant at the five percent level of confidence. c. Statistically significant at the ten percent level of confidence. California Department of Corrections and Rehabilitation Office of Research/Research and Evaluation Branch Page 70 December 19, 2011 Inmate Classification System Study Table 7 IV Estimates of the Effects of Being at a Higher Security Level Using Points Above the Threshold as an Instrument for Housing Level Panel A: Level I/Level II Threshold (First Stage F-statistic = 4.66) Any RVR A1/A2 B/C/D E/F Coefficient on -1.478 -0.028 -0.840 -0.308 proportion of (1.155) (0.141) (0.679) (0.545) period in level II Panel B: Level II/Level III (First Stage F-statistic = 180.63) Any RVR A1/A2 B/C/D Coefficient on 0.126 0.023 0.047 proportion of (0.108) (0.030) (0.081) period in level III Panel C: Level III/Level IV (First Stage F-statistics = 1,285.94) Any RVR A1/A2 B/C/D c Coefficient on 0.091 0.006 0.084b proportion of (0.050) (0.015) (0.039) period in level IV E/F 0.070 (0.091) E/F -0.000 (0.041) Standard errors are in parentheses. The figures in the table provide the second-stage coefficient on the noted housing security level variable. The second stage specification include proportion of time in the upper security level of the range analyzed, a quadratic function in preliminary score (measured after the beginning of the review period) interacted with the threshold cutoff, thirty two offense dummies, dummy variables indicating the time of sentence (second-strike, third-strike, LWOP, determinate), dummy variables indicating first, second, third, or fourth or higher stay with CDCR, a quadratic in age, seven race/ethnicity dummies, a dummy indicating documented gang affiliation, a linear measure of the length of the review period, and indicator variable for those who move up security levels and an indicator variable for those who moved down. The first stage employs a dummy for being above the threshold as an instrument for the proportion of time in the indicated housing level. a. Statistically significant at the one percent level of confidence. b. Statistically significant at the five percent level of confidence. c. Statistically significant at the ten percent level of confidence. California Department of Corrections and Rehabilitation Office of Research/Research and Evaluation Branch Page 71 December 19, 2011 Inmate Classification System Study Table 8 RD Estimates of the Effects of Being Above the Security Classification Threshold on the Likelihood of Serious Rules Violations During the Review Period Using PLACEMENT SCORE Measured One Day Prior to the Beginning of the Review Period Panel A: Level I/Level II Threshold Any RVR A1/A2 B/C/D E/F No Covariates -0.168a -0.008 -0.058a -0.138a (0.026) (0.006) (0.017) (0.023) Controlling for -0.087a -0.011 -0.025 -0.076a preliminary (0.027) (0.006) (0.017) (0.023) score and age All Covariates -0.041 -0.008 -0.004 -0.044c (0.027) (0.007) (0.018) (0.023) Panel B: Level II/Level III Any RVR A1/A2 B/C/D E/F a b No Covariates -0.141 -0.006 -0.050 -0.121a (0.029) (0.007) (0.020) (0.025) Controlling for -0.031 -0.002 -0.008 -0.044c preliminary (0.030) (0.008) (0.021) (0.026) score and age All Covariates -0.032 -0.003 -0.009 -0.045c (0.030) (0.008) (0.021) (0.026) Panel C: Level III/Level IV Any RVR A1/A2 B/C/D E/F a b a No Covariates -0.133 -0.011 -0.079 -0.069a (0.019) (0.005) (0.015) (0.016) Controlling for -0.036 -0.005 -0.027 -0.015 preliminary (0.022) (0.006) (0.017) (0.018) score and age All Covariates -0.025 -0.006 -0.021 -0.006 (0.022) (0.007) (0.017) (0.018) Standard errors are in parentheses. The figures in the table provide the coefficient from a dummy variable indicating a placement score (measured one day before the beginning of the review period) above the security classification threshold for the comparison indicated. All models include a cubic function in placement score interacted with the threshold cutoff. The models with all covariates include a quadratic in preliminary score, thirty two offense dummies, dummy variables indicating the time of sentence (second-strike, third-strike, LWOP, determinate), dummy variables indicating first, second, third, or fourth or higher stay with CDCR, a quadratic in age, seven race/ethnicity dummies, a dummy indicating documented gang affiliation, and a linear measure of the length of the review period. a. Statistically significant at the one percent level of confidence. b. Statistically significant at the five percent level of confidence. c. Statistically significant at the ten percent level of confidence. California Department of Corrections and Rehabilitation Office of Research/Research and Evaluation Branch Page 72 December 19, 2011 Inmate Classification System Study Table 9 RD Estimates of the Effects of Being Above the Security Classification Threshold on the Likelihood of Serious Rules Violations During the Review Period Using PLACEMENT SCORE Measured After the Beginning of the Review Period Panel A: Level I/Level II Threshold Any RVR A1/A2 B/C/D E/F a a No Covariates -0.109 0.004 -0.063 -0.087a (0.028) (0.007) (0.018) (0.025) Controlling for 0.007 0.008 -0.015 -0.002 preliminary (0.029) (0.007) (0.019) (0.025) score and age All Covariates 0.051c 0.013c 0.008 0.021 (0.029) (0.007) (0.019) (0.025) Panel B: Level II/Level III Any RVR A1/A2 B/C/D E/F a a No Covariates -0.104 -0.004 -0.065 -0.054b (0.033) (0.008) (0.023) (0.028) Controlling for 0.049 0.006 -0.000 0.042 preliminary (0.033) (0.009) (0.000) (0.028) score and age All Covariates 0.047 0.007 -0.001 0.039 (0.033) (0.009) (0.024) (0.028) Panel C: Level III/Level IV Any RVR A1/A2 B/C/D E/F a a No Covariates -0.109 -0.004 -0.059 -0.076 (0.021) (0.006) (0.016) (0.017) Controlling for 0.026 0.009 0.015 -0.009 preliminary (0.023) (0.007) (0.018) (0.019) score and age All Covariates 0.032 0.010 0.021 -0.007 (0.023) (0.007) (0.018) (0.019) Standard errors are in parentheses. The figures in the table provide the coefficient from a dummy variable indicating a placement score (measured after beginning of the review period) above the security classification threshold for the comparison indicated. All models include a cubic function in placement score interacted with the threshold cutoff. The models with all covariates include a quadratic in preliminary score, thirty two offense dummies, dummy variables indicating the time of sentence (second-strike, third-strike, LWOP, determinate), dummy variables indicating first, second, third, or fourth or higher stay with CDCR, a quadratic in age, seven race/ethnicity dummies, a dummy indicating documented gang affiliation, and a linear measure of the length of the review period. a. Statistically significant at the one percent level of confidence. b. Statistically significant at the five percent level of confidence. c. Statistically significant at the ten percent level of confidence. California Department of Corrections and Rehabilitation Office of Research/Research and Evaluation Branch Page 73 December 19, 2011 Inmate Classification System Study Appendix J Statistical Methods and Summarized Results for Gap, Matching and Longitudinal Analyses Neil Willits, Ph.D. Statistical Laboratory, Department of Statistics UC Davis November 17, 2011 The analyses described and summarized in this Appendix have been divided into three types. The gap analyses were used to address Classification questions 1 through 4, as well as some additional questions that were raised as an offshoot from those analyses. A variant of the gap analyses was used to address custody Question 2 as well. Most of the other close custody questions were addressed based on the longitudinal analyses. The matching analyses were used as an alternate way of addressing the second classification question. The Data: The data for these analyses were of two general forms. The first data set represented a yearlong “snapshot” of prison behavior (CDCR 840) reviews for review periods that began in the 2008/9 fiscal year, restricted to prisoners who were imprisoned for the entire fiscal year. This comprised 121,374 reviews on a total of 72,322 distinct inmates. Many inmates received semiannual (or even more frequent) reviews during this period, accounting for the larger number of reviews than inmates. This data was used as the basis of the gap analyses and its offshoots. The matching analyses were based on exact matches between inmates with and without constraining mandatory minimum scores, which were drawn from this data set. Various quality control checks were run on the data, to eliminate obvious errors. For example, there were numerous reviews for which the listed beginning of review period was later than the listed end of review period, there were some obvious errors in various dates (e.g., review periods beginning prior to 1950), misspelling in various categorical variables. These errors were corrected when the appropriate correction was unambiguous and treated as missing values otherwise. The data were quite high in overall quality, with misspelling occurring in fewer than 1% of observations. The other data sets were longitudinal in nature, following groups of inmates through up to approximately 10 years of 840 reviews. These data sets focused on particular subgroups of inmates, including (1) inmates with sentences of at least 15 years and below 50 years who were in the start of their prison term, (2) inmates with sentences of 50+ years at the start of their terms, (3) inmates with single life sentences, and (4) inmates with multiple life sentences. The longitudinal analyses were based on these data sets. California Department of Corrections and Rehabilitation Office of Research/Research and Evaluation Branch Page 74 December 19, 2011 Inmate Classification System Study Dependent Variables: Occurrence of an RVR and RVR Counts: The responses (dependent variables) used in the statistical analyses were similar for all of these data sets. One form looked simply at whether an inmate had committed one or more violations of a given type. The other form of outcome looked at the number of violations of a given type that occurred during the review period. Since the review periods varied in length (even though most of them covered a period of either six or twelve months), the analyses needed to adjust for this variability. This adjustment is necessary since you wouldn’t expect an inmate to run up as many violations in a six month period as in a twelve month period. A more detailed explanation of this adjustment will appear in conjunction with the description of the analyses. To examine the form of the relationship between a continuous predictor and one of the outcome variables, generalized additive models (or GAMs) were fit to the data, which use spline methods (in this case) to fit more general curves to a relationship like this. The standard algorithms for fitting GAMs don’t allow for the inclusion of random effects (such as an inmate effect) and so the GAM models were used to estimate the form of the relationships between continuous predictors and an outcome measure, after which a mixed model GLIM was used to insert the necessary random effects into the model. Logistic (binomial) GLIMs were used for modeling the occurrence of one or more violations, and Poisson GLIMs were used for modeling RVR counts. Gap analyses: These analyses were based on the data from the 2008/09 fiscal year, restricted to include only the inmates who were listed as being housed in Level I through Level IV. Each analysis focused on inmates whose placement scores were close to one of the threshold levels used to classify inmates into housing levels. Thus each analysis was split into three sub-analyses, one looking at placement scores in the range 19±7, a second at the range 28±7 and a third at the range 52±7. There were also parallel analyses run on the dichotomous and count outcomes, and for all serious (A through F) RVRs, on A through D violations, and restricted to A violations only. This meant that each analysis was divided into 18 sub-analyses (three thresholds levels times 2 forms of outcome times three levels of RVRs). Matching analyses: These analyses contrasted inmates who have a constraining mandatory minimum (one that’s greater than their preliminary score) with inmates who have the same preliminary score but no constraining mandatory minimum. These pairs of inmates were also matched on their ethnicity, mental health code, the administrative determinants for gang affiliation, psychological condition and sex offender status. A more restrictive set of matches were run which added offense category to the criteria for an exact match. Offense category was defined based on the primary offense group that was defined for each inmate, though offenses were aggregated more broadly: California Department of Corrections and Rehabilitation Office of Research/Research and Evaluation Branch Page 75 December 19, 2011 Inmate Classification System Study Violent Sex-related Property Drug-related Other offense groups 1 through 8 and 14, offense groups 9 through 13, offense groups 15 through 22, offense groups 23 through 31, offense groups 31 through 37. Matches were made without regard to the housing level at which an inmate was being held, since when one inmate has a constraining mandatory minimum, he would typically be housed in a more secure housing level than the matched inmate who lacked the mandatory minimum. The primary focus of the matching analyses was the difference in offense counts between the matched inmates with- and without- constraining mandatory minimums. Additional analyses were run to examine how these differences differ as a function of the preliminary score level. Longitudinal Analyses: The longitudinal analyses were similar to the gap analyses, except that there was more than one year of data on most inmates and each analysis focused on a single additional factor of interest, outlined in the first Custody question: - The impact of time served on behavior among inmates with sentences of 15 to 50 years, who are in the first half dozen years of their sentence, The impact of time served on behavior among inmates with sentences of 50 or more years, who are in the first ten years of their sentence, The impact of years to the minimum earliest parole date (MEPD) on behavior among inmates with single life sentences, who are within ten years of MEPD, and The impact of years to MEPD on behavior among inmates with multiple life sentences, who are within ten years of MEPD. To get a clear picture of the importance of the factor of interest, these analyses all included the preliminary score and inmate age, which have been seen to be the most important factors in predicting inmate behavior. Summary of Results (gap analyses): Since the classification score questions were phrased in terms of placement scores rather than preliminary scores, the first of these analyses looked at the impact of placement score, housing level in the middle of the review period (hclv), and the difference between the placement and preliminary score (called ppdiff in the tables). For inmates without a constraining mandatory minimum, this predictor will be equal to zero, whereas for inmates with a constraining minimum, this predictor will indicate how much of an “upgrade” was caused by their mandatory minimum. The mid-review housing level was used, since at the start of a review period, many inmates will be scheduled to move to a different level, based on the results of the previous review. Thus the mid-review housing level is more indicative of where an inmate spent most of his time during the subsequent review period. Although each of these analyses were restricted to inmates whose placement score was within ±7 of a California Department of Corrections and Rehabilitation Office of Research/Research and Evaluation Branch Page 76 December 19, 2011 Inmate Classification System Study placement threshold, the sample sizes were still extremely large. For the analysis on the inmates with placement scores of 19±7, that subset of the data base included data from 36849 reviews. For the analysis run at the 28±7 threshold, the subset included data from 20742 reviews. For the analysis run at the 52±7 threshold, the subset included data from 14446 reviews. When sample sizes are this large, even relatively trivial effects can be highly significant (i.e., having small p-values), and so the results will be summarized primarily in terms of estimates of relative effect size (e.g., F-statistics) to facilitate comparisons between analyses and between predictors. The following table contains the F-statistics for these three predictors from an analysis that assumes that the relationship between ppdiff and the likelihood of one or more violation or the number of violations is linear in the corresponding generalized linear model. The table contains parameter estimates (slopes) for each of the factors, along with the F-statistic that was used to test for statistical significance. The F-statistic can be thought of as a signal to noise ratio, indicating how much larger a given effect is relative to the noise level in the data. These relationships are all highly significant (p < .0001) except where noted. Outcome Any RVR RVR count Any RVR RVR count Any RVR RVR count Any ABCD ABCD count Any ABCD ABCD count Any ABCD ABCD count Any A A count Any A A count Any A A count cutoff 19 19 28 28 52 52 19 19 28 28 52 52 19 19 28 28 52 52 b(placement) .0204 .0118 .0412 .0357 .0236 .0185 .0260 .0199 .0432 .0442 −.0286 .0218 .0330 .0288 .0246 .0203 -.0010 .0009 F(placement) 14.43 * 9.95 * 69.97 105.73 12.75 * 16.21 20.20 17.13 65.30 107.81 14.42 * 16.34 20.54 19.72 13.63 * 11.03 * 0.01 (ns) 0.01 (ns) b(ppdiff) −.0557 −.0546 −.0577 −.0521 −.0300 −.0271 −.0561 −.0531 −.0502 −.0479 −.0334 −.0317 −.0347 −.0420 −.0708 −.0719 −.0252 −.0229 F(ppdiff) 870.58 1378.81 358.13 427.82 209.82 261.76 690.16 870.08 214.30 271.01 170.53 219.47 177.11 298.90 139.84 160.34 73.29 81.14 F(hclv) 67.55 331.98 19.65 48.46 4.78 (ns) 14.31 * 35.26 33.72 35.26 23.34 6.16 (ns) 12.68 * 169.32 233.44 45.92 49.21 10.57 * 11.64 * In all instances, the F-statistic for the placement/preliminary difference is greater than the corresponding F-statistic for the placement score itself, often by an order of magnitude or more. In all of these analyses, the coefficient for the placement score is small and positive, whereas the coefficient for ppdiff is larger and negative, meaning that for inmates without mandatory minimums, there’s a slight increase in the likelihood/number of violations as the preliminary score (equal to the placement score) increases, but for inmates with mandatory minimums, the greater the discrepancy between the preliminary and placement scores, the lower the likelihood/number of violations is. For example, for RVR counts near the 19 threshold, an increase of one in the placement score (i.e., for an inmate without a California Department of Corrections and Rehabilitation Office of Research/Research and Evaluation Branch Page 77 December 19, 2011 Inmate Classification System Study constraining mandatory minimum) would result in an approximately 1% increase in the expected numbers of RVRs, given that the corresponding slope is around .01. However for inmates with a constraining mandatory minimum, for each point their preliminary score falls below 19, there would be an approximately 5% decrease in the expected numbers of RVRs, given that the slope with respect to ppdiff is around −.05. The significance of the housing level as a predictor of inmate misbehavior generally takes the form that the rate of misbehavior increases as the housing level becomes less restrictive. The question about the form of housing level effects was first raised, some crude analyses were done based on summary statistics, rather than the full data set. Two of these graphs appear next, one for the most broadly-defined RVR response (A through F) and the other for A through D violations. In the first graph (for all serious RVRs), the rate of violations in Level I (as a function of the preliminary score) is considerably higher than for comparable inmates housed in higher housing levels: California Department of Corrections and Rehabilitation Office of Research/Research and Evaluation Branch Page 78 December 19, 2011 Inmate Classification System Study However, when the analysis is restricted to A through D violations, the difference is much less pronounced, suggesting that the suppression effect in Level II is predominately due to the least serious (E and F) violations. California Department of Corrections and Rehabilitation Office of Research/Research and Evaluation Branch Page 79 December 19, 2011 Inmate Classification System Study The initial gap analyses all assumed that the relationships with the preliminary or placement score were linear. Subsequent analyses fitted smooth, nonlinear curves to those relationships, to gain insight into the form of the relationships. In most cases, the nonlinear curves fit significantly better than the linear ones, though this result needs to be viewed in light of the large sample sizes on which the analyses were based. For example, when looking at the likelihood of any serious RVRs as a function of the preliminary and placement scores, along with the housing level, the estimated linear relationships were as follows: In these graphs, the value old_placement represents an inmate’s placement score at the beginning of the review period in question. Equivalently, the prior preliminary score is the preliminary score at the beginning of the review period. These relationships both have strong linear components to their trends, representing the overall linear trend; for a given preliminary score, the likelihood of one or more violation decreases with the placement score, whereas for a given placement score, the likelihood of one or more violations increases with the preliminary score. At the same time, there are nonlinear aspects of these curves, most notably that the placement score plot is relatively flat for placement scores below 15, and that there’s a flattening of the preliminary score plot for scores between 5 and 15. In this analysis, the significance of the linear and nonlinear components of these trends were as follows: Component Placement (linear) Placement (nonlinear) Preliminary (linear) Preliminary (nonlinear) Housing level F-statistic 92.98 10.64 892.00 62.37 163.52 California Department of Corrections and Rehabilitation Office of Research/Research and Evaluation Branch p-value <.0001 .0011 <.0001 <.0001 <.0001 Page 80 December 19, 2011 Inmate Classification System Study This result is typical of those for other types of RVRs, as well as for the “RVR count” responses. It’s noteworthy that the F-statistic for the (linear) preliminary score is nearly ten times as large as for the corresponding F-statistic for the placement score. This can be taken as evidence that the preliminary score is a more useful (or essential) variable than the placement score when it’s being used to predict inmate behavior. It’s also noteworthy that there aren’t any sharp changes in these functions throughout the range of values that are plotted. This is in part due to the fact that the method is based on splines which are chosen to be smooth, but it also speaks to the fact that there aren’t any sharp tipping points (scores where sharp changes in inmate behavior occurs). These observations are typical of these analyses and supports the conclusion that the preliminary is a better predictor of inmate behavior than the placement score. To focus more closely on the impact of preliminary score on behavior, and to simplify these models, a series of analyses were run on the inmates that have constraining mandatory minimums and who are housed at the expected level for their placement score. Thus there are three such analyses: one for inmates with a placement score of exactly 19, housed at Level II, one for inmates with a placement score of exactly 28, housed at level III, and one for inmates with a placement score of exactly 52, housed at level IV. The factor that was examined in these analyses was the preliminary score, which could range from 0 up to the threshold level in a given analysis. The graphs will be presented for the dichotomous analyses only, since the analyses of RVR counts are qualitatively very similar. For A-F RVRs, the 19 cutoff and inmates in housing level II, the estimated curve was: Here we see the lowest violation rates for the very lowest preliminary scores, increasing to a plateau around a score of 2 through 15 and then a second increase after that. California Department of Corrections and Rehabilitation Office of Research/Research and Evaluation Branch Page 81 December 19, 2011 Inmate Classification System Study For A-D RVRs, the 19 cutoff and inmates in housing level II, the estimated violation rates were: In this graph, we again see an increase for the inmates with the very lowest preliminary scores, followed by a long plateau and an increase beyond a preliminary score of around 15. The corresponding curve for the most serious (A) violations is noisier, due primarily to the fact that A violations are comparatively rare and so a given sample will contain less information about those violations. California Department of Corrections and Rehabilitation Office of Research/Research and Evaluation Branch Page 82 December 19, 2011 Inmate Classification System Study The recurring aspect of these curves is that the inmates with very low preliminary scores (say two or less) are less likely to have violations than inmates with higher scores. When these analyses are run on the inmates with a placement score of 28, housed at level III, the curves are quite similar to the first three graphs: For A-F RVRs, the 28 cutoff and inmates in housing level III, For A-D RVRs, the 28 cutoff and inmates in housing level III, California Department of Corrections and Rehabilitation Office of Research/Research and Evaluation Branch Page 83 December 19, 2011 Inmate Classification System Study For A RVRs, the 28 cutoff and inmates in housing level III, When the analysis is run on inmates with a placement score of 52 in housing level IV, the graphs are again quite similar: California Department of Corrections and Rehabilitation Office of Research/Research and Evaluation Branch Page 84 December 19, 2011 Inmate Classification System Study For A-D RVRs, the 52 cutoff and inmates in housing level IV, Finally, for A RVRs, the 52 cutoff and inmates in housing level IV, The next extension of these analyses asked whether there were any additional factors, beyond the preliminary and placement scores and the housing level, that would help predict an inmate’s likelihood or propensity for accruing violations. The main focus of this analysis was the administrative determinants that CDCR records for each inmate. The models included both preliminary and placement scores, the housing level, and one of the administrative determinants at a time. The following table summarizes those results for each California Department of Corrections and Rehabilitation Office of Research/Research and Evaluation Branch Page 85 December 19, 2011 Inmate Classification System Study threshold and dependent variable (type of RVR). When a determinant is significantly significant, the direction of the effect is indicated in a column and colored to help identify patterns in the data. Cells colored orange through red indicate that an inmate with this administrative determinant is more likely to offend than one who lacks the determinant. The opposite is true for cells that are colored green. One set of red cells has been singled out, corresponding to the administrative determinant for the disciplinary flag (DIS). Since the determinants were defined at the 840 review at the end of a review period, having this flag set could indicate misbehavior within that review period, and so this likely isn’t a predictive relationship. The fact that this flag is highly significant signifies little; just that inmates who have this administrative determinant are apt to have misbehaved recently. The same isn’t true for the other determinants, which aren’t likely to have changed in the current review period due to events that transpired during that period, and so these are more likely to be interpretable as predictors of behavior. Among those determinants, several that stand out are that the enemies flag is typically predictive of improved behavior, as are the flags for life sentences, sex offender status and psychological conditions. For the other flags, the administrative determinants are either insignificant or else there isn’t a clear pattern of being a positive (or negative) risk factor for rules violations. The table includes the results for the determinants for discipline problems, life sentences, sex offender status and psychological conditions, along with the flag for age, which is included as a representative determinant that isn’t strongly associated with behavioral changes. California Department of Corrections and Rehabilitation Office of Research/Research and Evaluation Branch Page 86 December 19, 2011 Inmate Classification System Study change due to determinant threshold determinant type (values for DIS) 19 AGE RVR 0.245467 19 AGE RVR 0.375682 28 AGE RVR 1.414904 0.234244 28 AGE RVR 0.555453 1.974283 0.159994 52 AGE RVR 0.517954 2.945305 0.086127 52 AGE RVR 1 1.403091 8.108672 0.004405 -75.42 19 AGE ABCD 0 1 1.441947 10.2663 0.001355 -76.35 19 AGE ABCD ad_AGE 0 1 0.210367 0.395886 0.529222 28 AGE ABCD count ad_AGE 0 1 0.176071 0.433865 0.510098 28 AGE ABCD anyRVR ad_AGE 0 1 1.045863 3.696161 0.054538 52 AGE ABCD count ad_AGE 0 1 1.093033 5.272633 0.021663 52 AGE ABCD anyRVR ad_AGE 0 0 20.2857 19 AGE A anyRVR ad_AGE 0 1 -0.25667 0.553284 0.456979 28 AGE A count ad_AGE 0 1 -0.20361 0.39316 0.530643 28 AGE A anyRVR ad_AGE 0 1 -0.11978 0.077978 0.780056 52 AGE A count ad_AGE 0 1 -0.1137 0.088164 0.766524 52 AGE A anyRVR ad_DIS 0 1 -0.92437 202.3492 6.41E-46 152.03 19 DIS RVR count ad_DIS 0 1 -0.77535 335.7295 5.43E-75 117.14 19 DIS RVR anyRVR ad_DIS 0 1 -0.71844 95.15115 1.76E-22 105.12 28 DIS RVR dependent Parameter Level1 DF Estimate ChiSq ProbChiSq anyRVR ad_AGE 0 1 0.186849 0.534113 0.464883 count ad_AGE 0 1 0.241441 1.348921 anyRVR ad_AGE 0 1 0.27132 0.784785 count ad_AGE 0 1 0.272303 anyRVR ad_AGE 0 1 count ad_AGE 0 1 anyRVR ad_AGE 0 count ad_AGE anyRVR change due to determinant -66.48 count ad_DIS 0 1 -0.569 159.1013 1.78E-36 76.65 28 DIS RVR anyRVR ad_DIS 0 1 -0.27289 21.451 3.63E-06 31.38 52 DIS RVR count ad_DIS 0 1 -0.21145 29.43909 5.77E-08 23.55 52 DIS RVR anyRVR ad_DIS 0 1 -1.45314 694.3488 5.1E-153 327.65 19 DIS ABCD count ad_DIS 0 1 -1.33063 1066.323 6.9E-234 278.34 19 DIS ABCD anyRVR ad_DIS 0 1 -1.06 253.6683 4.12E-57 188.64 28 DIS ABCD count ad_DIS 0 1 -0.97912 448.4227 1.6E-99 166.21 28 DIS ABCD anyRVR ad_DIS 0 1 -0.57021 99.19824 2.28E-23 76.86 52 DIS ABCD count ad_DIS 0 1 -0.4891 136.5437 1.52E-31 63.08 52 DIS ABCD anyRVR ad_DIS 0 1 -3.22514 7693.384 0 2415.70 19 DIS A count ad_DIS 0 1 -3.26715 10748.81 0 2523.66 19 DIS A anyRVR ad_DIS 0 1 -2.89395 3809.144 0 1706.46 28 DIS A count ad_DIS 0 1 -2.90039 5211.892 0 1718.12 28 DIS A California Department of Corrections and Rehabilitation Office of Research/Research and Evaluation Branch Page 87 December 19, 2011 Inmate Classification System Study anyRVR ad_DIS 0 1 -2.11796 1606.988 0 731.42 52 DIS A count ad_DIS 0 1 -2.02903 1947.586 0 660.67 52 DIS A anyRVR ad_ENE 0 1 0.421813 172.4046 2.21E-39 -34.41 19 ENE RVR count ad_ENE 0 1 0.355491 205.1835 1.54E-46 -29.92 19 ENE RVR anyRVR ad_ENE 0 1 0.445228 123.3197 1.19E-28 -35.93 28 ENE RVR count ad_ENE 0 1 0.336955 136.8361 1.31E-31 -28.61 28 ENE RVR anyRVR ad_ENE 0 1 0.188371 18.51348 1.69E-05 -17.17 52 ENE RVR count ad_ENE 0 1 0.151701 25.18232 5.22E-07 -14.08 52 ENE RVR anyRVR ad_ENE 0 1 0.328474 87.61986 7.93E-21 -28.00 19 ENE ABCD count ad_ENE 0 1 0.322397 118.5441 1.32E-27 -27.56 19 ENE ABCD anyRVR ad_ENE 0 1 0.385402 76.88188 1.81E-18 -31.98 28 ENE ABCD count ad_ENE 0 1 0.330677 88.77737 4.42E-21 -28.16 28 ENE ABCD anyRVR ad_ENE 0 1 0.14839 10.24223 0.001373 -13.79 52 ENE ABCD count ad_ENE 0 1 0.098557 7.758101 0.005347 -9.39 52 ENE ABCD anyRVR ad_ENE 0 1 0.637895 179.0877 7.67E-41 -47.16 19 ENE A count ad_ENE 0 1 0.684234 232.1492 2.03E-52 -49.55 19 ENE A anyRVR ad_ENE 0 1 0.807503 156.9522 5.24E-36 -55.40 28 ENE A count ad_ENE 0 1 0.809659 187.8981 9.14E-43 -55.50 28 ENE A anyRVR ad_ENE 0 1 1.145488 236.9446 1.82E-53 -68.19 52 ENE A count ad_ENE 0 1 1.133506 289.9282 5.15E-65 -67.81 52 ENE A anyRVR ad_LIF 0 1 0.235131 49.82789 1.68E-12 -20.95 19 LIF RVR count ad_LIF 0 1 0.213429 66.044 4.41E-16 -19.22 19 LIF RVR anyRVR ad_LIF 0 1 0.552334 133.4498 7.21E-31 -42.44 28 LIF RVR count ad_LIF 0 1 0.43919 159.1896 1.7E-36 -35.54 28 LIF RVR anyRVR ad_LIF 0 1 0.397849 75.58947 3.49E-18 -32.82 52 LIF RVR count ad_LIF 0 1 0.341688 116.2925 4.1E-27 -28.94 52 LIF RVR anyRVR ad_LIF 0 1 0.153155 17.38394 3.05E-05 -14.20 19 LIF ABCD count ad_LIF 0 1 0.079238 6.632196 0.010015 -7.62 19 LIF ABCD anyRVR ad_LIF 0 1 0.378319 54.72549 1.39E-13 -31.50 28 LIF ABCD ABCD count ad_LIF 0 1 0.354136 74.28377 6.77E-18 -29.82 28 LIF anyRVR ad_LIF 0 1 0.399183 67.28713 2.35E-16 -32.91 52 LIF ABCD count ad_LIF 0 1 0.360212 92.29452 7.47E-22 -30.25 52 LIF ABCD anyRVR ad_LIF 0 1 -0.00924 0.042946 0.835827 19 LIF A count ad_LIF 0 1 0.01969 0.220783 0.638444 19 LIF A anyRVR ad_LIF 0 1 0.158662 6.466906 0.01099 28 LIF A California Department of Corrections and Rehabilitation Office of Research/Research and Evaluation Branch -14.67 Page 88 December 19, 2011 Inmate Classification System Study count ad_LIF 0 1 0.218946 14.03088 0.00018 anyRVR ad_LIF 0 1 0.088974 2.292347 0.130013 -19.66 28 LIF A 52 LIF A A count ad_LIF 0 1 0.072017 1.897612 0.168346 52 LIF anyRVR ad_PRE 0 1 -0.49587 7.120704 0.00762 64.19 19 PRE RVR count ad_PRE 0 1 -0.37527 7.339193 0.006747 45.54 19 PRE RVR anyRVR ad_PRE 0 1 -0.20314 0.688578 0.406648 28 PRE RVR count ad_PRE 0 1 -0.30215 3.655997 0.055868 28 PRE RVR anyRVR ad_PRE 0 1 -0.13267 0.139963 0.708318 52 PRE RVR count ad_PRE 0 1 -0.07816 0.103379 0.747812 52 PRE RVR anyRVR ad_PRE 0 1 -0.43706 4.967351 0.02583 54.81 19 PRE ABCD count ad_PRE 0 1 -0.35376 4.596965 0.032029 42.44 19 PRE ABCD anyRVR ad_PRE 0 1 0.260641 0.702176 0.402054 28 PRE ABCD count ad_PRE 0 1 0.231985 0.847027 0.357395 28 PRE ABCD anyRVR ad_PRE 0 1 0.086645 0.046362 0.829519 52 PRE ABCD count ad_PRE 0 1 -0.06367 0.047522 0.827433 52 PRE ABCD anyRVR ad_PRE 0 1 -0.71388 12.13194 0.000496 104.19 19 PRE A count ad_PRE 0 1 -0.6432 11.06682 0.000879 90.26 19 PRE A anyRVR ad_PRE 0 0 19.68242 28 PRE A anyRVR ad_PRE 0 1 -1.01588 10.84638 0.00099 176.18 52 PRE A count ad_PRE 0 1 -0.97076 12.77746 0.000351 163.99 52 PRE A anyRVR ad_PSY 0 1 0.02243 0.198069 0.656284 19 PSY RVR count ad_PSY 0 1 0.020077 0.263175 0.607947 19 PSY RVR anyRVR ad_PSY 0 1 0.120102 3.285906 0.069877 28 PSY RVR count ad_PSY 0 1 0.078467 2.755422 0.096925 28 PSY RVR anyRVR ad_PSY 0 1 0.047442 0.420455 0.51671 52 PSY RVR count ad_PSY 0 1 0.028007 0.308828 0.5784 52 PSY RVR anyRVR ad_PSY 0 1 0.057539 1.060723 0.30305 19 PSY ABCD count ad_PSY 0 1 0.023801 0.261571 0.609043 19 PSY ABCD anyRVR ad_PSY 0 1 0.139085 3.659569 0.055748 28 PSY ABCD ABCD count ad_PSY 0 1 0.127667 4.787736 0.028663 28 PSY anyRVR ad_PSY 0 1 -0.01132 0.021699 0.882891 52 PSY ABCD count ad_PSY 0 1 -0.03807 0.429168 0.512398 52 PSY ABCD anyRVR ad_PSY 0 1 0.750281 65.59549 5.54E-16 -52.78 19 PSY A count ad_PSY 0 1 0.767845 76.16955 2.6E-18 -53.60 19 PSY A anyRVR ad_PSY 0 1 0.659025 31.66434 1.83E-08 -48.26 28 PSY A California Department of Corrections and Rehabilitation Office of Research/Research and Evaluation Branch -11.99 Page 89 December 19, 2011 Inmate Classification System Study count ad_PSY 0 1 0.701776 40.3039 2.17E-10 -50.43 28 PSY A anyRVR ad_PSY 0 1 0.358236 10.52989 0.001175 -30.11 52 PSY A count ad_PSY 0 1 0.365937 13.69708 0.000215 -30.65 52 PSY A anyRVR ad_SEX 0 1 0.484565 219.9772 9.15E-50 -38.40 19 SEX RVR count ad_SEX 0 1 0.465668 320.6919 1.02E-71 -37.23 19 SEX RVR anyRVR ad_SEX 0 1 0.640571 181.1008 2.79E-41 -47.30 28 SEX RVR count ad_SEX 0 1 0.51587 215.3073 9.55E-49 -40.30 28 SEX RVR anyRVR ad_SEX 0 1 0.417235 53.50522 2.58E-13 -34.11 52 SEX RVR count ad_SEX 0 1 0.34879 72.70572 1.5E-17 -29.45 52 SEX RVR anyRVR ad_SEX 0 1 0.617968 267.1995 4.63E-60 -46.10 19 SEX ABCD count ad_SEX 0 1 0.637544 386.8886 3.94E-86 -47.14 19 SEX ABCD anyRVR ad_SEX 0 1 0.704491 163.3875 2.06E-37 -50.56 28 SEX ABCD count ad_SEX 0 1 0.624111 195.7221 1.79E-44 -46.43 28 SEX ABCD anyRVR ad_SEX 0 1 0.391222 39.93288 2.63E-10 -32.38 52 SEX ABCD count ad_SEX 0 1 0.333664 47.98699 4.29E-12 -28.37 52 SEX ABCD anyRVR ad_SEX 0 1 0.877655 294.4237 5.4E-66 -58.42 19 SEX A count ad_SEX 0 1 0.897038 344.6077 6.33E-77 -59.22 19 SEX A anyRVR ad_SEX 0 1 1.120464 167.3657 2.78E-38 -67.39 28 SEX A count ad_SEX 0 1 1.124837 200.1309 1.96E-45 -67.53 28 SEX A anyRVR ad_SEX 0 1 1.15244 117.4707 2.26E-27 -68.41 52 SEX A count ad_SEX 0 1 1.14384 143.7162 4.1E-33 -68.14 52 SEX A anyRVR ad_VIO 0 1 0.166736 25.6272 4.14E-07 -15.36 19 VIO RVR count ad_VIO 0 1 0.150357 33.90585 5.78E-09 -13.96 19 VIO RVR anyRVR ad_VIO 0 1 0.197647 18.29368 1.89E-05 -17.93 28 VIO RVR count ad_VIO 0 1 0.150351 20.68565 5.41E-06 -13.96 28 VIO RVR anyRVR ad_VIO 0 1 0.09686 3.685351 0.054892 52 VIO RVR count ad_VIO 0 1 0.122933 12.17693 0.000484 -11.57 52 VIO RVR anyRVR ad_VIO 0 1 0.240521 41.75152 1.04E-10 -21.38 19 VIO ABCD count ad_VIO 0 1 0.179189 33.0422 9.02E-09 -16.41 19 VIO ABCD anyRVR ad_VIO 0 1 0.230273 20.45743 6.1E-06 -20.57 28 VIO ABCD count ad_VIO 0 1 0.201814 24.55987 7.2E-07 -18.28 28 VIO ABCD anyRVR ad_VIO 0 1 0.141901 6.864548 0.008792 -13.23 52 VIO ABCD count ad_VIO 0 1 0.133503 10.20363 0.001402 -12.50 52 VIO ABCD anyRVR ad_VIO 0 1 0.321159 47.4671 5.59E-12 -27.47 19 VIO A count ad_VIO 0 1 0.357401 66.08237 4.32E-16 -30.05 19 VIO A California Department of Corrections and Rehabilitation Office of Research/Research and Evaluation Branch Page 90 December 19, 2011 Inmate Classification System Study anyRVR ad_VIO 0 1 0.234213 12.501 0.000407 -20.88 28 VIO A count ad_VIO 0 1 0.303689 24.0054 9.61E-07 -26.19 28 VIO A anyRVR ad_VIO 0 1 0.155767 5.23188 0.022177 -14.42 52 VIO A count ad_VIO 0 1 0.176064 8.330335 0.003899 -16.14 52 VIO A California Department of Corrections and Rehabilitation Office of Research/Research and Evaluation Branch Page 91 December 19, 2011 Inmate Classification System Study The next analyses addressed custody question 4 by considering a range of additional predictors of RVRs in an attempt to build a more a more complete model of the likelihood and numbers of violations, to see which predictors combine to make a model that represents an improvement on the gap models that have been presented so far. A “short list” of predictors was developed which have been found in other settings to be useful predictors of in-prison behavior. These included: Housing category (primarily dormitory versus cells) Inmate age, fit using spline methods to account for possible nonlinearity, Offense category (violent, property, sex-related, drug-related and other), Ethnicity, Mental health code, Risk level, History of serious/violent violations, Sentence type (determinant-driven, 2nd strike, 3rd strike, life, death and life w/o parole), Sex offender status, Street gang affiliation, Age at first arrest (categorical), Years to earliest release, fit using spline methods to account for possible nonlinearity, and Sentence length, fit using spline methods to account for possible nonlinearity, Each of these predictors were used in conjunction with the preliminary and placement scores and the mid-review housing level in models to predict likelihood/numbers of RVRs in three categories (A, A through D and A through F) and at each of the three cutoffs (19, 28 and 52). A “consensus” stepwise selection procedure was used to build models to predict these outcomes. The other factor used to guide model selection was whether a given predictor could be used in practice for classifying inmates, due to legal concerns or concerns about public safety. The only way in which this circumvented the normal stepwise selection procedure was that sex offender status was found to be a highly significant predictor of inmate behavior at an early stage, with sex offenders significantly less likely to accrue violations. Since the assignment of sex offenders is also governed by public safety concerns, lest these inmates be given an opportunity to escape, this predictor was passed over in the selection process. In order, the predictors selected were as follows, along with a general description of their impact on the predictive models: Age was the first predictor added to the model. After adjusting for preliminary and placement scores and housing level, the likelihood/number of violations decreases with age, and in a fashion that’s very nearly linear, at roughly 2 to 4% for each additional year of age, depending on the group of inmates and the severity of violation being analyzed. Since age is one of the factors considered in the preliminary score, this would indicate that for this purpose, that score under-adjusts for age. The offense category was the predictor added to the model at the second step. The strongest difference here was that inmates convicted on sex-related offenses have lower violation rates, in some cases up to 50% lower, after adjusting for the other factors in the model. As California Department of Corrections and Rehabilitation Office of Research/Research and Evaluation Branch Page 92 December 19, 2011 Inmate Classification System Study mentioned, this may not be seen as a reason to house these inmates in less secure housing, but it’s important to include this predictor in a model in order to get a clearer picture of the importance of other potential predictors. The mental health code was the predictor added to the model at the third step. Inmates with CCCMS and EOP designations can be roughly 30-40% more likely to accrue violations, with the exception of when the analysis is restricted to A violations. As is often the case, A violations are comparatively quite rare and so the analyses restricted to A violations only don’t allow as powerful inferences as analyses that are based on more broadly-defined violations. Following these three factors, the analyses became less consistent, with significance often not extending across different threshold levels or for different groups of violations. There may be additional factors that have a genuine but less pronounced impact on violation rates, but these effects are relative minor compared to the factors added at the first three steps in the model-building process (age, offense category and mental health code). Finally, the second custody questions asked specifically about the impact of housing category, most notably cell versus dorm housing, on violation rates. A set of analyses were run looking at the impact of the preliminary and placement scores, housing level, custody level and housing category on the usual range of outcome variables. The results here were mixed. At the 19 cutoff, there were no significant differences in violation rates according to housing category. At the 28 cutoff, the rate of A through F violations in celled housing was estimated to be roughly 15% lower than in dormitory housing after adjusting for the other effects in the model, and roughly 25% lower in celled housing when focusing on A through D violations. At the 52 cutoff, the rate of A through F violations in celled housing was estimated to be roughly 27% lower in celled housing and for A through D violations, it was roughly 32% lower. An additional question that was raised was whether the reason that for an inmate’s mandatory minimum was predictive of in-prison behavior. The following table gives the frequency of each reason for the inmates used in the gap analysis for each of the three placement score cutoffs. Group min. Description A B C D E F G H condemned LWOP CCR 3375.2 escape risk “R” suffix violence exclusion public interest case other life sentence No mandatory score 52 52 28 19 19 19 19 19 0 cutoff = 19 n % cutoff = 28 n % 517 12194 16074 11 2489 5564 3345 238 3946 6749 5 608 5851 1.40 33.09 43.62 0.03 6.75 15.10 16.13 1.15 19.02 32.54 0.02 2.93 28.21 cutoff = 52 n % 11 0.08 2469 17.09 326 2.26 179 1.24 2251 15.58 5722 39.61 5 0.03 519 3.59 2964 20.52 These models are similar to the other gap analyses. They all include preliminary score and housing level as factors in the model, so the comparisons are adjusted as if inmates with and without a given reason were in the same housing level. Thus the normal consequence of California Department of Corrections and Rehabilitation Office of Research/Research and Evaluation Branch Page 93 December 19, 2011 Inmate Classification System Study having one of these determinants would be a change in housing level, but these analyses look at whether they were worse (or better) behaved apart from this consequence. The following table contains an identifier for each analysis, the F-statistic and p-value for testing whether inmates are equally likely to accrue violations, regardless of the reason for their mandatory minimum (or whether they have one), along with pairwise comparisons between each of the listed reasons for a mandatory minimum and the group of inmates who have no mandatory minimum. It should be noted that since each mandatory minimum has an associated placement score, not all of these minimum are what we’ve called constraining mandatory minimum. For example, the categories D through H (outlined above) each have a mandatory score of 19 and at the 28 or 52 cutoff, these would not constrain an inmate’s placement. Dependent/cutoff Any RVR/19 RVR count/19 Any RVR/28 RVR count/28 Any RVR/52 overall F/p level Δ(level,no mandatory) 54.36 / <.0001 D (escape) +21.5% E (R suffix) -47.6% F (violence) -21.2% G (pub. interest) +105% H (other life) -18.0% 90.85 / <.0001 D +16.2% E -48.0% F -22.7% G +74.1% H -20.0% 40.37 / <.0001 C (Life inmate) -50.5% D -19.3% E -53.7% F -20.8% G +64.0% H -35.5% 47.69 / <.0001 C -41.8% D -16.0% E -44.6% F -16.5% G +152% H -25.8% 12.67 / <.0001 A (condemn.) +126% B (LWOP) -48.6% C -51.4% D -22.3% E -43.1% F -26.4% G +12.4% H -24.3% California Department of Corrections and Rehabilitation Office of Research/Research and Evaluation Branch p-value .0647 <.0001 <.0001 .2690 .0037 .0400 <.0001 <.0001 .2325 <.0001 <.0001 .1642 <.0001 <.0001 .6097 <.0001 <.0001 .1017 <.0001 <.0001 .0371 <.0001 .2205 <.0001 <.0001 .1735 <.0001 <.0001 .9050 .0128 Page 94 December 19, 2011 Inmate Classification System Study RVR count/52 Any ABCD/19 Any ABCD/28 Any ABCD/52 Any A/19 Any A/28 Any A/52 18.72 / <.0001 A B C D E F G H 61.27 / <.0001 D E F G H 27.05 / <.0001 C D E F G H 9.93 / <.0001 A B C D E F G H 59.03 / <.0001 D E F G H 54.37 / <.0001 C D E F G H 29.58 / <.0001 A B C D California Department of Corrections and Rehabilitation Office of Research/Research and Evaluation Branch +102% -42.9% -34.8% -6.3% -37.7% -23.1% -25.9% -22.6% +19.6% -49.2% -16.1% +253% +5.5% -38.0% -10.8% -50.3% -15.1% +67.1% -2.3% +17.0% -47.1% -38.9% -20.0% -42.6% -26.4% -11.6% -27.5% +11.4% -47.5% +15.3% -100% +23.9% -7.7% -65.8% -68.5% +29.4% -100% -9.8% +993% +84.9% -10.2% +7.0% .0519 <.0001 <.0001 .5767 <.0001 <.0001 .6712 .0007 .1060 <.0001 .0002 .0219 .4471 <.0001 .4828 <.0001 .0003 .5869 .8149 .8314 <.0001 .0014 .2484 <.0001 <.0001 .9038 .0065 .4782 <.0001 .0231 .9977 .0170 .4956 .0017 <.0001 <.0001 .9987 .4519 <.0001 <.0001 .5909 .7881 Page 95 December 19, 2011 Inmate Classification System Study E F G H -64.2% +42.4% -100% +0.6% <.0001 <.0001 .9989 .9680 Summary of Results (matching analyses): When inmates were matched on their preliminary score, ethnicity, mental health code, the administrative determinants for gang affiliation, psychological condition and sex offender status, there were a total of 6568 unique matched pairs that had complete agreement on these criteria. Each matched pair contained one inmate with a constraining mandatory minimum and one without one. The first part of this analysis examined the difference between RVR characteristics (and age) between the two groups. The results were as follows: Dependent Any RVR RVR count Any A-D A-D count Any A A count Age mean difference −.073 −.143 −.009 −.012 .004 .003 3.876 t-statistic −9.73 −10.949 −1.707 −1.885 1.749 1.330 30.31 p-valueComments: <.0001 higher in non-mandatory group <.0001 higher in non-mandatory group .0879 .0595 .0803 .1834 <.0001 higher in mandatory min. group As can be seen, the likelihood and count of broadly-defined RVRs were higher within the non-mandatory group, and the comparison for A through D RVRs showed higher numbers in the non-mandatory group as well, though these results were marginally insignificant. For A violations, the numbers were insignificantly higher in the mandatory minimum group. The mandatory minimum group was also significantly older than the no-minimum group (by about 4 years), which may tie into these differences. These differences are hard to interpret, since due to the standard way of assigning inmates to housing, most of these pairs would contain a no-mandatory inmate at one housing level and a “mandatory minimum” inmate housed at a more secure housing level. Thus the mandatory/no-mandatory differences are confounded with possible differences due to housing level, as well as with age. Analyses were also run that compared the likelihood or count of RVRs of a given type in matched inmates, as a function of the matched preliminary score. These analyses were run using generalized additive models within each of the groups. The following graphs summarize those results for this group of matched inmates, focusing on the “Any RVR” outcomes, rather than the “RVR count” outcomes. The set of graphs for the count outcomes were extremely similar to the ones presented here. California Department of Corrections and Rehabilitation Office of Research/Research and Evaluation Branch Page 96 December 19, 2011 Inmate Classification System Study Any RVR’s: Any A-D violation: California Department of Corrections and Rehabilitation Office of Research/Research and Evaluation Branch Page 97 December 19, 2011 Inmate Classification System Study Any A violation: In the first two of these graphs, the likelihood of RVRs is higher for inmates without mandatory minimums throughout most of the range of preliminary scores. By contrast, the two lines are quite similar for most of the range when focusing entirely on A violations. A second set of analyses was run in which the offense category was added as another matching criterion. When this criterion was added, the number of exact matched reduced to 4700. The results in most ways were quite similar to the first set of matching analyses: Dependent Any RVR RVR count Any A-D A-D count Any A group A count Age mean difference −.080 −.150 −.013 −.015 .006 t-statistic −8.82 −9.23 −1.943 −1.882 2.496 p-valueComments: <.0001 higher in non-mandatory group <.0001 higher in non-mandatory group .0521 .0599 .0126 slightly higher in mandatory .005 5.993 1.901 32.77 .0573 <.0001 higher in mandatory min. group The only qualitative difference here is that the slight increase in A violations in the mandatory minimum group is now marginally significant rather than marginally insignificant. Similar patterns are also seen in the graphs that plot the likelihood of various types of violation in each group, as a function of the preliminary score: California Department of Corrections and Rehabilitation Office of Research/Research and Evaluation Branch Page 98 December 19, 2011 Inmate Classification System Study Any RVRs: Any A-D RVRs: California Department of Corrections and Rehabilitation Office of Research/Research and Evaluation Branch Page 99 December 19, 2011 Inmate Classification System Study Any A violation: For both A-F and A-D RVRs, there’s a higher likelihood of an RVR in the no-mandatory group than for the mandatory minimum group. Unlike the first set of graphs, the lines cross for very low preliminary scores. This is an artifact of the matched sample, since for a preliminary score of zero, there were only 27 matches, and housing assignments were quite irregular among those inmates, with most of the mandatory minimum inmates being housed in Level I, while most of the no-mandatory inmates were housed in Level II. Other than this artifact, the most notable difference relative to the first set of graphs is that the difference due to having a mandatory minimum (lower likelihood of violations) in the A-D graph doesn’t become apparent until a preliminary score of around 20. Below that level, the two groups are quite comparable. As has been mentioned previously, the interpretation of the matching analyses is problematical, since these comparisons often involve inmates who have identical preliminary scores but who are housed at different housing levels. Summary of Results (longitudinal analyses): The longitudinal analyses were based on subsets of data that were developed, focusing on four subgroups of inmates: 1. 2. 3. 4. Inmates with sentences of 15 to 50 years (11252 reviews on 6168 inmates), Inmates with sentences of 50+ years (391 reviews on 217 inmates), Inmates with single life sentences (40292 reviews on 20573 inmates), and Inmates with multiple life sentences (14194 reviews on 6729 inmates), California Department of Corrections and Rehabilitation Office of Research/Research and Evaluation Branch Page 100 December 19, 2011 Inmate Classification System Study who were followed over as many past years as possible, given that most types of data aren’t available before a point in time where they started being compiled electronically. The key variables in these analyses included two of the ones used in previous analyses (preliminary score, housing level), along with custody level and at least one additional variable, either the number of years than an inmate had served to date, or else the number of years until the inmate’s minimum (earliest possible) parole date (MEPD). These analyses used generalized additive models to adjust for all of the factors except the one additional variable, since the question of interest was how behavior changed over time with respect to that additional factor. These analyses included the individual inmate as a random factor in the analyses, since over half of the inmates were represented by more than one 840 review in these analyses. As with the other analyses that fit nonlinear functions to responses, the results can be summarized in terms of the significance of the various effects, but given the sample size, the form of an effect is perhaps more to the point than the significance. Thus while a table of pvalues will be presented, the accompanying graphs are more germane in getting at the questions that had been posed. The following table contains all of those p-values. In the table, the significance of each model effect is summarized in terms of an F-statistic and the corresponding p-value. Significance of effects in longitudinal analyses Data set public15 Dependent anyRVR RVRcount anyABCD ABCDcount anyA Acount hclv 2.83 .0592 5.47 .0042 0.57 .5628 0.71 .4931 0.00 .9952 0.02 .9795 clvl 9.45 <.0001 15.88 <.0001 11.02 <.0001 16.47 <.0001 13.37 .1977 14.80 .0028 prelim(linear) 96.85 <.0001 100.54 <.0001 86.80 <.0001 90.83 <.0001 44.59 <.0001 40.64 <.0001 prelim(spline) 21.07 <.0001 25.59 <.0001 11.02 .0009 14.01 .0002 6.11 .0135 6.22 .0126 years(linear) 6.94 .0084 5.27 .0217 3.51 .0609 2.19 .1391 0.02 .8844 0.01 .9154 years(spline) 5.05 .0247 6.50 .0108 4.09 .0431 3.35 .0671 13.13 .0003 12.66 .0004 clvl 0.06 .8022 2.71 .1008 0.00 .9779 2.40 prelim(linear) 9.66 .0020 12.76 .0004 4.39 .0369 7.19 prelim(spline) 9.07 .0028 13.14 .0003 8.91 .0030 10.10 years(linear) 0.09 .7699 0.69 .4081 0.55 .4574 0.00 years(spline) 3.41 .0657 3.76 .0534 4.09 .0440 3.86 Data set public50 Dependent anyRVR RVRcount anyABCD ABCDcount hclv 0.09 .9174 0.02 .9912 0.97 .3789 0.87 California Department of Corrections and Rehabilitation Office of Research/Research and Evaluation Branch Page 101 December 19, 2011 Inmate Classification System Study anyA Acount .4206 1.26 .2836 0.01 .9852 .1222 3.80 .0521 0.05 .8257 .0077 6.98 .0086 0.02 .8989 .0016 116.59 <.0001 0.01 .9365 .9945 2.48 .1165 0.02 .8925 .0501 85.74 <.0001 0.01 .9026 prelim(spline) 117.04 <.0001 125.93 <.0001 33.95 <.0001 43.56 <.0001 23.48 <.0001 21.35 <.0001 years(linear) 12.70 .0004 9.08 .0026 1.79 .1811 1.32 .2501 0.81 .3668 0.61 .4335 years(spline) 42.12 <.0001 37.76 <.0001 14.29 .0002 21.76 <.0001 7.62 .0058 8.13 .0044 prelim(spline) 50.98 <.0001 42.19 <.0001 18.26 <.0001 19.00 <.0001 12.61 .0004 10.98 .0009 MEPD(linear) 3.86 .0496 0.65 .4218 0.21 .6459 0.06 .8045 3.66 .0557 3.67 .0553 MEPD(spline) 9.37 .0002 4.23 .0396 6.37 .0116 5.10 .0239 5.42 .0199 4.67 .0306 prelim(spline) 29.19 <.0001 25.61 <.0001 16.46 <.0001 13.05 years(linear) 9.99 .0016 4.11 .0425 5.88 .0153 2.41 years(spline) 17.23 <.0001 9.69 .0019 12.55 .0004 7.04 Data set public single (life sentences) Dependent anyRVR RVRcount anyABCD ABCDcount anyA Acount hclv 7.25 .0007 15.64 <.0001 3.93 .0197 6.55 .0014 3.75 .0235 4.00 .0182 clvl 8.32 .0002 3.87 .0208 4.30 .0136 2.00 .1350 3.13 .0438 3.00 .0497 prelim(linear) 279.91 <.0001 304.93 <.0001 171.72 <.0001 202.52 <.0001 36.28 <.0001 34.98 <.0001 Data set public single (life sentences) Dependent anyRVR RVRcount anyABCD ABCDcount anyA Acount hclv 3.60 .0273 6.55 .0014 2.35 .0955 2.53 .0798 1.37 .2543 1.53 .2157 clvl 11.28 <.0001 6.42 .0016 5.15 .0058 3.61 .0270 2.32 .0978 2.11 .1216 prelim(linear) 150.12 <.0001 141.33 <.0001 82.22 <.0001 93.54 <.0001 15.89 <.0001 14.49 .0001 Data set public multiple (life sentences) Dependent anyRVR RVRcount anyABCD ABCDcount hclv 4.22 .0147 4.91 .0074 2.94 .0530 3.15 clvl 2.51 .0814 1.50 .2232 2.11 .1216 1.45 prelim(linear) 98.40 <.0001 93.77 <.0001 89.59 <.0001 81.89 California Department of Corrections and Rehabilitation Office of Research/Research and Evaluation Branch Page 102 December 19, 2011 Inmate Classification System Study anyA Acount .0427 0.42 .6570 0.44 .6447 .2353 2.33 .0976 1.99 .1369 <.0001 26.76 <.0001 26.02 <.0001 .0003 8.34 .0039 7.54 .0060 .1204 0.15 .6948 0.04 .8355 .0080 6.86 .0088 7.00 .0081 prelim(spline) 7.02 .0081 10.77 .0010 3.21 .0733 3.51 .0612 5.93 .0149 7.78 .0053 MEPD(linear) 0.31 .5780 0.42 .5147 0.40 .5292 0.38 .5355 1.02 .3127 0.89 .3467 MEPD(spline) 2.91 .0882 2.44 .1180 2.21 .1373 1.99 .1586 7.43 .0065 7.69 .0056 Data set public multiple (life sentences) Dependent anyRVR RVRcount anyABCD ABCDcount anyA Acount hclv 1.47 .2289 1.45 .2343 0.71 .4915 0.78 .4600 1.88 .1522 1.93 .1451 clvl 1.48 .2286 1.57 .2091 0.53 .5911 0.49 .6149 1.38 .2521 1.37 .2538 prelim(linear) 17.60 <.0001 21.07 <.0001 8.68 .0032 9.56 .0020 2.85 .0914 2.91 .0879 From this table, it’s clear that the single best predictor of inmate behavior is still the preliminary score and so the impact of the other predictors need to be interpreted in the context provided by an inmate’s preliminary score. Thus an inmate with a low preliminary score will pose a minimal risk of misbehavior even if some of the other factors indicate a slight elevation in the risk of a violation. Moreover, the preliminary scores for most prisoners decrease over time, and because these models include preliminary score as a predictor, the effect of years in prison are viewed conditional on the preliminary score, meaning that it’s as if the preliminary score isn’t changing. The next graph looks at the relationship between preliminary score and years served in the group serving 15-50 year sentences, demonstrating this decreasing pattern in preliminary scores: California Department of Corrections and Rehabilitation Office of Research/Research and Evaluation Branch Page 103 December 19, 2011 Inmate Classification System Study Until the sample sizes get particularly small (for inmates more than five years into their sentence), the relationship is nearly linear, with the score decreasing on the average by about two points per year. The patterns in the other three subgroups are quite similar to this one. The following graphs are being presented to convey the form that the relationship between violations and either the years served in a sentence or the years to earliest parole (MEPD). Since these are complicated models, they have to assume something about what the values of the other predictors are. Because these are fairly arbitrary assumptions (and not germane when looking at the form of a predictor’s impact), these are labeled “comparative plots”. To keep the number of graphs manageable, only the graphs for the likelihood of one or more RVR of a given type will be presented. As an aside, the graphs for an RVR count are very similar to the corresponding graph for one or more RVR of the same type. California Department of Corrections and Rehabilitation Office of Research/Research and Evaluation Branch Page 104 December 19, 2011 Inmate Classification System Study The following graphs look at the impact of the number of years served in a sentence (labeled “stay_yrs” in the graphs). Within the group of inmates with 15 to 50 year sentences (the “temp15” data set), the graph for the model of all A through F violations is: In this graph, as in most of these graphs, the likelihood of one or more RVRs is fairly stable through the first four years of a sentence, after which the likelihood begins to increase. The corresponding graph for A-D violations is: California Department of Corrections and Rehabilitation Office of Research/Research and Evaluation Branch Page 105 December 19, 2011 Inmate Classification System Study The corresponding graph for one or more A violation is: In the subset of inmates with sentences of 50+ years (temp50), the graphs show a somewhat different pattern, with the likelihood of violations increasing at first and then decreasing toward the end of the 10 year period. It should be noted that this subset was considerably smaller than for the 15-50 group, so these graphs tend to be more erratic. The graph for one or more A through F violations is: California Department of Corrections and Rehabilitation Office of Research/Research and Evaluation Branch Page 106 December 19, 2011 Inmate Classification System Study The graph for one or more A through D violations is: The graph for one or more A violations is: This pattern is somewhat different, though it should be emphasizes that not only is the number of inmates small, but the likelihood of A violations within this group is also small. California Department of Corrections and Rehabilitation Office of Research/Research and Evaluation Branch Page 107 December 19, 2011 Inmate Classification System Study Within the single life sentence group (tempsingle), the likelihood of one or more A through F violations increases over time, assuming that the other model predictors are unchanged. This is a fairly fanciful assumption, since we’ve seen that it’s typical for an inmate’s preliminary to decrease over time. For A through D violations, the corresponding graph is California Department of Corrections and Rehabilitation Office of Research/Research and Evaluation Branch Page 108 December 19, 2011 Inmate Classification System Study and for A violations, the graph is Thus in this group, an inmate whose preliminary score was decreasing steadily would be increasingly a good behavior risk, while one whose preliminary score wasn’t decreasing would be less of a good risk as his sentence progressed. The other question posed for this group was whether an inmate’s behavior would tend to improve as he approached his MEPD. The following three graphs portray these relationships. It should be noticed that as time passes, the MEPD decreases, so an inmate would move “right to left” in these graphs as time passed. California Department of Corrections and Rehabilitation Office of Research/Research and Evaluation Branch Page 109 December 19, 2011 Inmate Classification System Study The graph for one or more A through F violations is: In this graph, we see that improvement steadily improves when an inmate is within 10 years of his MEPD, with about half of this improvement having taken place when he’s approximately 8 years from his MEPD. The corresponding graph for one or more A through D violations exhibits a similar pattern as MEPD approaches zero: California Department of Corrections and Rehabilitation Office of Research/Research and Evaluation Branch Page 110 December 19, 2011 Inmate Classification System Study For one or more A violations, the graph is somewhat different, though it should be noted that A violations are rare and so these trends are poorly estimated: Similar patterns were examined in the group of inmates with multiple life sentences. The relationship with the number of years an inmate had served to date for one or more A through F violations is: California Department of Corrections and Rehabilitation Office of Research/Research and Evaluation Branch Page 111 December 19, 2011 Inmate Classification System Study For A through D violations, the estimated relationship is: For one or more A violations, the graph is: All of these graphs show a generally increasing worsening in behavior over time, albeit conditional on the preliminary score. California Department of Corrections and Rehabilitation Office of Research/Research and Evaluation Branch Page 112 December 19, 2011 Inmate Classification System Study When looking at the impact of MEPD on behavior within the subgroup of inmates with multiple life sentences, the graph for one or more A through F violations is: The graph for one or more A through D violations is; California Department of Corrections and Rehabilitation Office of Research/Research and Evaluation Branch Page 113 December 19, 2011 Inmate Classification System Study Finally, the graph for one or more A violations is: All three of these graphs show a general improvement over time as an inmate gets within 10 years of his MEPD (reading right to left on the graphs). This effect is in addition to the improvement in behavior that you’d expect to see as the inmate ages and/or his preliminary score decreases. California Department of Corrections and Rehabilitation Office of Research/Research and Evaluation Branch Page 114 December 19, 2011 Inmate Classification System Study Appendix K CDCR Inmate Classification Score System Study Crosswalk CLASSIFICATION SCORE RESEARCH QUESTIONS Research Question(s) Findings 1. After reviewing ranges of scores for the current Classification System (0 – 18, 19 – 27, 28-51 and 52+), can natural “tipping points” or scores be There are no natural tipping points. identified which indicate an increase in the predicted probability of inprison violence? 2. Does analysis of the in-prison CCR 3375.2 inmates with a Mandatory Minimum behavior of inmates sentenced under score of 28 pose no greater risk of misconduct “CCR 3375.2 (a) (7) Life inmate than other inmates with similar scores. Inmates (multiple/execution style murders; with CCR 3375.2 Mandatory Minimums AND low escapes)” (who have a mandatory preliminary scores pose less risk for misconduct minimum classification score of 28) than inmates with no Mandatory Minimum and indicate the need to restrict their preliminary/placement scores of 28. housing to Level III institutions? Inmates at each of the cutoff thresholds, including the Level III threshold of 28, can be moved down to a lower housing level without the expectation that misconduct will increase. 3. Could the Mandatory Minimum Score LWOP inmates with placement scores of 52 due code “B” for LWOP, currently at 52 to Mandatory Minimums pose no greater risk of (Level IV) be reduced to allow LWOP misconduct than other inmates with similar scores. inmates to house in Level III 270, LWOP inmates with placement scores of 52 due new design facilities, without to Mandatory Minimums AND lower preliminary compromise to institutional security scores are less at risk for misconduct than or public safety? inmates with no Mandatory Minimum and placement scores of 52. 4. Could the Mandatory Minimum Score code “C” (CCR 3375.2) be adjusted to cover Close Custody inmate’s exclusively, without compromise to institutional security or public safety? California Department of Corrections and Rehabilitation Office of Research/Research and Evaluation Branch Inmates at each of the cutoff thresholds, including the Level IV threshold of 52, can be moved down without the expectation that misconduct will increase. Data were not available to determine differences between 270 degree and 180 degree housing. Custody Designations were not designed to predict inmate misconduct and should not be used to do so (see below). Moreover, Custody Designations do not predict escape (or consequent threat to public safety). Thus, changing an inmate’s Custody Designation would not alter the institutional security or public safety. Page 115 December 19, 2011 Inmate Classification System Study CUSTODY DESIGNATION RESEARCH QUESTIONS Research Question(s) Findings 1. Can the following proposed changes Questions 1a – 1d presume that Custody be made without jeopardizing safety? Designation relates to misconduct in ways that are a. Can Close A Custody be reduced unfounded. Custody Designations were created from five to two years, and Close B to manage inmates who were seen as posing Custody be increased from within high-risk to public safety if they escaped. Lethal seven years to within ten years, of Electrified Fences have lowered the possibility of their Minimum Earliest Parole Date escape to near zero (Appendix F). (MEPD) for inmates with multiple life sentences? [CCR-3377.2 (b)(3)(B) Because Custody Designations are unrelated to & (c)(3)(B)] the risk of misconduct, changes to inmate custody b. Can Close B Custody be reduced status is not expected to negatively affect from ten to five years for inmates behavior or institutional security. with sentences of 50 or more years? [CCR-3377.2(c)(3)(A)] c. Can Close B Custody be increased from within seven years to within ten years of their MEPD for inmates with life sentences? [CCR-3377.2 (b) (3) (C)] d. Can Close B Custody be reduced from four to two years for inmates with sentences of 15 to 50 years? [CCR-3377.2(c)(3)(D)] 2. Does the need for increased The escape data presented in Appendix F show supervision for Close B Custody that stated “need” for increased supervision is equate to the need to be assigned to questionable. Manual case reviews performed on celled housing rather than dormitory those cases where inmates assigned to Level II housing within a secure perimeter? through Level IV housing successfully escaped In other words, can this population of reveals that all but two of the escapes are inmates be moved from celled irrelevant to this study since they actually occurred housing to dorm housing without an when the inmates were authorized to be outside of increase in the predicted probability their secured housing level (e.g., out to court, out for medical treatment). of in-prison violence? Regression analyses are used to examine the impact of preliminary and placement scores, housing level, custody level and housing category (cells vs. dormitories) on RVRs. The results are mixed. At the 19 threshold, there are no significant differences in RVRs with respect to housing category. At the 28 threshold, the rate of A-F violations in celled housing is approximately 13 percent lower than in dormitories and about 24 percent lower for A-D violations. At the 52 threshold, the rate of A-F violations in celled housing is approximately 26.5 percent lower than California Department of Corrections and Rehabilitation Office of Research/Research and Evaluation Branch Page 116 December 19, 2011 Inmate Classification System Study CUSTODY DESIGNATION RESEARCH QUESTIONS Research Question(s) Findings in dormitories and about 31.5 percent lower for AD violations (see Appendix J, pp. 93). 3. What criteria could be used to A literature review that explores factors related to change an inmate’s Custody inmate escapes reveals that the research on Designation? classification practices related to escapes is limited, and thus no current practices may be conceived as being ‘evidence-based.’ The sparse literature on escapes leaves no definitive criteria that could be used to change an inmate’s Custody Designation. Despite this limitation, CDCR’s Close Custody criteria are, for the most part, supported by some research. All criteria except High Notoriety / Public Interest / Management Concern have been identified as potential escape risk factors. The full literature review on escapes may be found in Appendix M. 4. Can the attributes of inmates that increase or decrease the risk of inprison violence be identified? If so, should those attributes be used to identify a subpopulation of inmates that can be removed from Close Custody? And, if so, how many inmates currently assigned Close Custody could be reduced to Medium custody? The attributes of inmates that increase or decrease the risk of in-prison violence are identified by the inmate’s preliminary classification score. Because Custody Designations do not measure risk of inmate misconduct ALL Close Custody inmates can be moved to Medium Custody with the expectation that it will not affect levels of misconduct. However, if CDCR wants to move groups of inmates downward in terms of supervision or security level, the inmates that pose the lowest risk of misconduct can be identified based upon their preliminary score. Age may also be taken into consideration. The number of inmates could easily be estimated based upon preliminary classification score. 5. How many inmates could safely have their Custody Designation changed without an increase in the predicted probability of in-prison violence? 6. Do the current regulatory criteria for Close Custody accurately identify escape risk potential based upon evidence based practices? Follow-up analysis to be performed by CDCR, Office of Research, Offender Information Services Branch staff. California Department of Corrections and Rehabilitation Office of Research/Research and Evaluation Branch Page 117 December 19, 2011 Because escapes in Levels II – IV are near zero, and because all of the Custody Designations are unrelated to risk of misconduct, there is little evidence to support them. Inmate Classification System Study CUSTODY DESIGNATION RESEARCH QUESTIONS Research Question(s) Findings Moreover, the literature review (see Appendix M) shows that the Close Custody criteria currently used by CDCR have been identified as escape risk factors. However, not enough research has been conducted to conclusively state that any of these criteria are in fact evidence based 7. Is there any specific data that reflects No. There is not enough escape data available to the tendency for an offender to develop a cohort of escapees and conduct an attempt to escape based on analysis on length of sentence. The CDCR sentence length? If so, is there a Successful Escapes Report (Appendix F) shows suitable range (time remaining to that other than walk-aways, there have been very serve) that can be identified to allow few escapes since 1999. The literature review for adjustment and identify risk? completed for question #6 did not show that sentence length was a risk factor for escape. However, it did show that most escapes occur during the first half of a inmates prison sentence. 8. Have electrified fences reduced the A review of escapes of Level III inmates which number of escape attempt from those have occurred in the last 12 years shows that secure environments? none occurred in institutions with electrified fences. This suggests that inmates who are deemed public safety risks could be housed in a lower level provided an electrified fence was present. 9. Has it been determined that longer A literature review of misclassification (see sentences correlate with greater Appendix N) was completed and a number of instances of misconduct? factors were found to contribute to the misclassification of inmates. One of those factors was length of sentence. Although there is little research available, multiple studies found that LWOPS (life without the possibility of parole) were less likely to engage in violent behavior than inmates sentenced to 10-14 years, 15-19 years and more than 30 years. 10. Has it been determined that those offenders with initial placement scores of Level III or Level IV, have a N/A greater tendency to move up or down in points (excluding LWOP offenders)? 11. Does an offender’s security level along with the application of Close See previous results. Custody have an effect on in-custody behavior? 12. Does the existing Close Custody Regulatory Policy identify an N/A offender’s propensity for misconduct or is security level a better indicator? California Department of Corrections and Rehabilitation Office of Research/Research and Evaluation Branch Page 118 December 19, 2011 Inmate Classification System Study CUSTODY DESIGNATION RESEARCH QUESTIONS Research Question(s) Findings 13. Is data available that identifies age In the Gap Analysis, age was the first factor and physical impairment as factors applied to the model. After adjusting for that would allow for reduced custody preliminary score, placement score and housing - regardless of term length and level it’s estimated that the likelihood/number of security level? violations decrease between one and four percent for each additional year of age, depending on the cutoff point used in the gap analysis and the type of RVR. This was a linear decrease, so there is no ‘age cut-point’ where violations drop dramatically. Furthermore, the literature review on escapes showed that escapes typically occur when inmates are in their 20s or mid 30s. These two findings suggest that as inmates age, the need for Close Custody decreases. It is also possible that age is being underutilized in the calculation of the preliminary score as well as the utilization of Close Custody. 14. Can it be determined if Minimum A and Minimum B custody can be combined into one custody level? a. Do we have data to support the need for both Minimum Custody levels? b. Which facilities currently house N/A offenders with Minimum A Custody? c. How many offenders currently excluded from Camp/MSF/CCF due to new medical classification criteria are assigned Minimum A Custody? California Department of Corrections and Rehabilitation Office of Research/Research and Evaluation Branch Page 119 December 19, 2011 Appendix L Cohort Descriptives 16 Table 1. HOUSING LEVEL I Age n II % n III % n IV % n TOTAL % n % 18-19 10 0.2% 31 0.1% 304 0.7% 249 0.9% 594 20-24 485 8.0% 733 3.2% 4,691 11.5% 3,179 11.2% 9,088 0.6% 9.2% 25-29 956 15.8% 1,853 8.0% 6,182 15.1% 5,478 19.3% 14,469 14.7% 30-34 902 14.9% 2,556 11.1% 5,759 14.1% 5,273 18.5% 14,490 14.7% 35-39 952 15.7% 3,283 14.3% 5,162 12.6% 4,545 16.0% 13,942 14.2% 40-44 1,013 16.8% 3,962 17.2% 5,265 12.9% 3,751 13.2% 13,991 14.2% 45-49 941 15.6% 4,023 17.5% 5,480 13.4% 2,972 10.5% 13,416 13.6% 50-54 481 8.0% 3,042 13.2% 3,891 9.5% 1,669 5.9% 9,083 9.2% 55-59 204 3.4% 1,794 7.8% 2,091 5.1% 768 2.7% 4,857 4.9% 60+ 101 1.7% 1,751 7.6% 2,023 5.0% 550 1.9% 4,425 4.5% 6,045 100.0% 23,028 100.0% 40,848 100.0% 28,434 100.0% 98,355 100.0% Total Mean Age 38.2 43.5 38.8 36.0 39.0 Race White 1,798 29.7% 6,234 27.1% 9,640 23.6% 5,019 17.7% 22,691 23.1% Hispanic 1,968 32.6% 8,384 36.4% 15,917 39.0% 11,219 39.5% 37,488 38.1% 32.1% Black 2,010 33.3% 6,744 29.3% 12,342 30.2% 10,482 36.9% 31,578 Asian 28 0.5% 283 1.2% 456 1.1% 233 0.8% 1,000 1.0% Native American 48 0.8% 225 1.0% 394 1.0% 293 1.0% 960 1.0% 0.2% Pacific Islander Other Total 11 0.2% 63 0.3% 89 0.2% 65 0.2% 228 182 3.0% 1,095 4.8% 2,010 4.9% 1,123 3.9% 4,410 4.5% 6,045 100.0% 23,028 100.0% 40,848 100.0% 28,434 100.0% 98,355 100.0% Commitment Offense Category Person 2,024 33.5% 17,380 75.5% 30,845 75.5% 24,654 86.7% 74,903 76.2% Property 1,607 26.6% 2,338 10.2% 4,506 11.0% 2,002 7.0% 10,453 10.6% Drug 1,914 31.7% 2,377 10.3% 3,639 8.9% 1,027 3.6% 8,957 9.1% 498 8.2% 928 4.0% 1,857 4.5% 749 2.6% 4,032 4.1% 2 0.0% 5 0.0% 1 0.0% 2 0.0% 10 0.0% 6,045 100.0% 23,028 100.0% 40,848 100.0% 28,434 100.0% 98,355 100.0% Other Missing Sex Reg Flag Yes No Total 12 0.2% 6,100 26.5% 8,977 22.0% 3,961 13.9% 19,050 19.4% 6,033 99.8% 16,928 73.5% 31,871 78.0% 24,473 86.1% 79,305 80.6% 6,045 100.0% 23,028 100.0% 40,848 100.0% 28,434 100.0% 98,355 100.0% Mental Health Code CCCMS/EOP No Mental Health Code Total 346 5.7% 4,018 17.4% 10,996 26.9% 9,128 32.1% 24,488 24.9% 5,699 94.3% 19,010 82.6% 29,852 73.1% 19,306 67.9% 73,867 75.1% 6,045 100.0% 23,028 100.0% 40,848 100.0% 28,434 100.0% 98,355 100.0% 94.5% Serious/Violent Yes 4,589 75.9% 21,551 93.6% 38,720 94.8% 28,083 98.8% 92,943 No 1,456 24.1% 1,477 6.4% 2,128 5.2% 351 1.2% 5,412 5.5% 6,045 100.0% 23,028 100.0% 40,848 100.0% 28,434 100.0% 98,355 100.0% Total 16 Only includes reviews where housing level is available. California Department of Corrections and Rehabilitation Office of Research/Research and Evaluation Branch Page 120 December 19, 2011 Cohort Descriptives (continued) Table 1. (cont’d.) HOUSING LEVEL I II III IV TOTAL Custody Max 5 0.1% 108 0.5% 503 1.2% 815 2.9% 1,431 Close A 0 0.0% 0 0.0% 2,020 4.9% 5,714 20.1% 7,734 1.5% 7.9% Close B 0 0.0% 1,766 7.7% 15,333 37.5% 14,195 49.9% 31,294 31.8% Medium A 90 1.5% 17,619 76.5% 21,913 53.6% 7,660 26.9% 47,282 48.1% Medium B 414 6.8% 2,200 9.6% 475 1.2% 16 0.1% 3,105 3.2% Minimum A 531 8.8% 127 0.6% 66 0.2% 0 0.0% 724 0.7% Minimum B 4,991 82.6% 1,175 5.1% 300 0.7% 6 0.0% 6,472 6.6% 14 0.2% 30 0.1% 156 0.4% 27 0.1% 227 0.2% 0 0.0% 3 0.0% 82 0.2% 1 0.0% 86 0.1% 6,045 100.0% 23,028 100.0% 40,848 100.0% 28,434 100.0% 98,355 100.0% Unclassified Unknown Total RVR n % n % n % n % Yes 1,841 30.5% 3,703 16.1% 9,383 23.0% 8,533 30.0% No Total n 23,460 % 23.9% 4,204 69.5% 19,325 83.9% 31,465 77.0% 19,901 70.0% 74,895 76.1% 6,045 100.0% 23,028 100.0% 40,848 100.0% 28,434 100.0% 98,355 100.0% A1/A2 Violations Yes No Total 70 1.2% 171 0.7% 522 1.3% 880 3.1% 1,643 1.7% 5,975 98.8% 22,857 99.3% 40,326 98.7% 27,554 96.9% 96,712 98.3% 6,045 100.0% 23,028 100.0% 40,848 100.0% 28,434 100.0% 98,355 100.0% B, C, D Violations Yes No Total 502 8.3% 1,318 5.7% 4,284 10.5% 4,867 17.1% 10,971 11.2% 5,543 91.7% 21,710 94.3% 36,564 89.5% 23,567 82.9% 87,384 88.8% 6,045 100.0% 23,028 100.0% 40,848 100.0% 28,434 100.0% 98,355 100.0% E,F Violations Yes 1,461 24.2% 2,577 11.2% 5,685 13.9% 4,065 14.3% 13,788 14.0% No 4,584 75.8% 20,451 88.8% 35,163 86.1% 24,369 85.7% 84,567 86.0% 6,045 100.0% 23,028 100.0% 40,848 100.0% 28,434 100.0% 98,355 100.0% Total Preliminary Score Level I II III IV Placement Score Level I II III IV I n 8,770 Table 2. Placement Score Level II III % n % n % 23.7% 24,389 65.9% 2,600 7.0% 10,020 94.5% 389 3.7% 24,662 97.4% I n 5,158 761 119 7 % 58.8% 2.2% 0.4% 0.0% Table 3. Housing Level II III n % n % 2,636 30.1% 952 10.9% 19,119 55.6% 14,375 41.8% 1,155 4.2% 22,868 82.7% 118 0.4% 2,653 9.6% California Department of Corrections and Rehabilitation Office of Research/Research and Evaluation Branch IV n % 1,257 3.4% 199 1.9% 671 2.6% 25,398 100.0% IV n 24 154 3,509 24,747 % 0.3% 0.4% 12.7% 89.9% TOTAL n % 37,016 100.0% 10,608 100.0% 25,333 100.0% 25,398 100.0% TOTAL n % 8,770 100.0% 34,409 100.0% 27,651 100.0% 27,525 100.0% Page 121 December 19, 2011 Appendix M Literature Review on Escape Risk Factors Executive Summary The California Department of Corrections and Rehabilitation (CDCR) is currently examining both the external and internal components of its classification system. An external system places an inmate in a prison, while an internal system places an inmate in housing and programming within a prison. The purpose of this literature review is to determine whether the current regulatory Close Custody criteria are based on evidence-based practices that accurately identify escape risk. An additional goal of this review was to identify other criteria that might be used to change an inmate’s custody classification. Specifically, this literature review addresses the following research question: Do the current regulatory criteria for Close Custody accurately identify escape risk potential based upon evidence-based practices? The research on escape is first discussed, followed by a comparison made between the research and CDCR’s Close Custody criteria. The main findings are: • The research on classification practices is so limited that the Close Custody criteria cannot be confirmed as evidence-based. While there are various studies that appear to support the criteria, the quality and amount of research is inadequate to conclude that they are evidence-based. • There are important limitations in the escape literature. In particular, multiple counting methods are employed, definitions of escape vary, and sample sizes are small. In addition most of the literature examines minimum security inmates because they escape more frequently than medium or maximum security inmates. • Many of the publications are dated. Most research on escapes was conducted before valid classification systems were in place and before electrified perimeter fences became standard security features of prisons. Demographic changes may also affect the relevance of some studies to the current inmate population. • Risk factors can be divided into two groups: static (which cannot be changed) and dynamic (which can be changed). The most frequently identified static risk factors for escape attempts include: ° Age: escapees are typically in their 20s or early 30s ° Adult Criminal History: escapees often have previous convictions and incarcerations ° Juvenile Criminal History: escapees were found to have a record of juvenile convictions and incarcerations ° Property Crimes: escapees tend to have convictions for property-related crimes ° Length of Time Already Served: escapes typically occur early on in an inmate’s sentence California Department of Corrections and Rehabilitation Office of Research/Research and Evaluation Branch Page 122 December 19, 2011 ° Previous Escape Attempts: inmates who escape often have previous escape attempts in their record ° Race/Ethnicity: in older studies, escapees were more frequently white than black, although more recent research did not support this finding The most frequently identified dynamic risk factors for escape attempts include: ° Holds, Detainers, Denial of Parole: escapees who have legal actions that lengthened their stay in prison beyond their expected release date are more likely to escape ° Institutional Misconduct: escapees are more likely to have disciplinary problems while in prison ° Relationship Problems: inmates may have be more likely to escape because of relationship problems, such as a relationship ending, divorce, or death in the family ° Substance Abuse: escapees are likely to have alcohol or drug addictions, although the significance of these are unclear as substance abuse is also found in inmates who do not attempt escapes Table A compares CDCR’s Close Custody Regulations with the static and dynamic escape risk factors. Table A Escape Risk Factor Static Age Adult Criminal History Juvenile Criminal History Property Crimes Length of Time Already Served Previous Escape Attempts Race/Ethnicity Dynamic Holds, Detainers, Denial of Parole Institutional Misconduct Relationship Problems Substance Abuse High Notoriety/Public Interest/Management Concern California Department of Corrections and Rehabilitation Office of Research/Research and Evaluation Branch CDCR Close Custody Criteria x x x x Risk Factors Identified in the Literature x x x x x x x x x x x x Page 123 December 19, 2011 CDCR’s Close Custody criteria are, for the most part, supported by some research. All criteria except High Notoriety/Public Interest/Management Concern have been identified as escape risk factors. However, not enough research has been conducted to conclusively state that any of these criteria are evidence-based. Furthermore, there are escape risk factors found in the literature that are not addressed by the CDCR Close Custody criteria. Age and relationship problems might be explored as additional criteria, although the latter could be difficult to objectively define or identify. In sum, there is not enough empirical research available to confirm whether or not CDCR’s Close Custody criteria are evidence-based, although there is some research that implicitly supports them. This does not mean they are wrong, but rather that there is not enough research to confirm that they are or are not the right criteria to use. As a result, decisions to maintain or change current CDCR Close Custody practices should not be based upon the available research alone. California Department of Corrections and Rehabilitation Office of Research/Research and Evaluation Branch Page 124 December 19, 2011 Literature Review on Escape Risk Factors Full Review Introduction An inmate classification system is critical for determining where inmates should be placed within a prison system in order to ensure the safety and security of individuals working and living in institutions, as well as those in the community. The literature sometimes discusses two types of systems: external and internal. An external system places an inmate in a prison, while an internal system places an inmate in housing and programming within a prison. Within the California Department of Corrections and Rehabilitation (CDCR), the pointand-level Inmate Classification Score System is considered an external system, while the CDCR Close Custody Designations are considered to be part of an internal system. The Custody Designation an inmate receives while in custody at CDCR determines the type of supervision s/he receives once s/he is in the institution. It may also impact the jobs or programs to which s/he may be assigned. The purpose of the Custody Designation is to determine supervision control levels based upon problematic behavior or an individual’s potential for escape and threat to the community if an escape occurs. The CDCR designation is primarily based on the following factors (although other reasons may also be considered): - The inmate’s total term, sentence, or remaining time-to serve - The inmate’s escape history - Receipt of an active law enforcement felony hold - An inmate who is considered to be High Notoriety or is designated as a Public Interest Case or a Management Concern 17 - A finding of guilt for a serious, felony level, Rules Violation Report (RVR) CDCR is currently examining both the internal and external components of its classification system. The purpose of this literature review is to compare the current regulatory CDCR Close Custody criteria with evidence-based practices, to validate current classification practices, and to potentially identify different/additional criteria that should be used to determine an inmate’s Custody Designation. This literature review addresses the following research question in the Inmate Classification System Study: Do the current regulatory criteria for Close Custody accurately identify escape risk potential based upon evidence-based practices? 17 Per CCR Title 15. Crime Prevention and Corrections, Section 3000. Definitions, a Management Concern is defined as a behavior observed or documented in the inmate’s criminal history that demonstrates to a classification committee that the inmate has a propensity towards violence against self or others; has a history of inciting or pressuring others toward criminal behavior; preys on more vulnerable members of society; or portrays a level of criminal sophistication and/or access to large amounts of drugs, money, or power. This may include disruptive groups and prison gang members or affiliates. California Department of Corrections and Rehabilitation Office of Research/Research and Evaluation Branch Page 125 December 19, 2011 Methodology The following databases were searched for relevant literature on inmate escape attempts and successful escapes: • Academic Search Complete • Google Scholar • JSTOR • MEDLINE with Full Text • PsychARTICLES • PsychINFO • Psychology and Behavioral Sciences Collection • SocINDEX with Full Text The search terms varied across the databases due to varying design and use of subject vocabulary, but the predominant terms used were: • Escapes • Fugitives from Justice Free-text searching terms included: • Escape Risk Factors • Prison Escapes • Inmate Escapes • Predicting Inmate Escapes • Absconders Criteria for inclusion: • Adult male prison inmates • Escapes from all security-level institutions • Mental health literature, where the focus is on individuals are committed for criminal behavior Criteria for exclusion: • Escapes from confinement of a military or political nature, such prisoner-of-war camps, where confinement is not due to behavior as an individual • Female inmate escapes, as the Classification Study includes only male inmates • Validity of assessment instruments to predict escape California Department of Corrections and Rehabilitation Office of Research/Research and Evaluation Branch Page 126 December 19, 2011 Twenty-six articles were selected and either downloaded or ordered from the State Library. After this initial review, 16 were found to be useful. Publication dates were between 1959 and 2011. Limitations Age of Literature Most research on escape-risk factors was conducted before the use of validated classification systems, better-designed secure housing, and electrified fences—all of which may reduce the need to look at inmates’ personal escape risk factors. Also, because much of the literature is dated, it is not evident if the identified risk factors apply to today’s inmate population. This is an important limitation, as technological advances may have rendered much of the prior research obsolete. For example, there were only six inmate escapes from CDCR’s secure-custody facilities in 2010. Likewise, the New York Department of Correctional Services (2011) reported only one inmate escape in 2009 and no escapes in 2008. Therefore, it is likely that many individual-level factors that have historically been associated with escape risk may have lost some of their predictive value in modern institutions where such events are increasingly rare. Multiple Counting Methods and Definitions There is no one national database that tracks prison escapes. A few national surveys suggest approximate numbers and rates of escapes, but the difference between the highest and lowest estimates varies by 176% (Culp, 2005). In addition, the literature does not always specify the type of facility from which an escape was made. While escapes are attempted from all security level facilities, the majority are from minimum security facilities. Culp (2005) estimated that around 89% of escapes are from minimum security environments. This type of escape is frequently called a “walkaway” or “awol” (absent without leave). Some studies make a distinction between these types of escapes and attempts from secured facilities, while other studies do not. Also, “escape” and “escape attempt” are not clearly differentiated in the research. For the sake of consistency the general term “escape” will be used in this literature review. Small Samples Escape attempts are not routine events in any correctional system. Most inmates do not try to escape. As a result, the number of escapees in any one study is small. This is especially true of escapes from maximum security settings. Study Population The research focuses on lower security level inmates because they escape more frequently than inmates in medium or maximum security prisons. It is not known if the risk factors identified in most of the research would apply to inmates in medium- or maximum-level prisons. California Department of Corrections and Rehabilitation Office of Research/Research and Evaluation Branch Page 127 December 19, 2011 Escape Risk Factors Risk factors can be divided into two groups: static and dynamic. Static risk factors cannot change (e.g., gender, age at first arrest, age at first incarceration). Dynamic risk factors can change (e.g., drug use, number of rules violation reports received in a one year period). Static Escape Risk Factors Static risk factors are those that cannot change either because they are demographic in nature (e.g., race) or they are events that occurred in the past (e.g., juvenile escape attempt). Culp (2005), in his review of static and dynamic risk factors, concluded that static risk factors are more reliable in predicting escapes. The following are the main static escape risk factors discussed in the literature: Age Young age, ranging from 20 to 31 years, is the most consistently identified risk factor for escape. Several studies found that the majority of escapees in their studies were under the age of 30 (Cochrane, 1948 as cited in Loving, Stockwell and Dobbins, 1959; Morrow, 1969). Morgan (1967) compared a group of South Carolina escapees with a control group of similar inmates who did not attempt to escape and found that “significantly more” escapees were younger than 25 years. Muir-Cochrane, Gorta and Sillavan (1991), in their analysis of 812 escapees in New South Wales, Australia, indicated that 58% were under the age of 24 and Mosel (1996) described the typical absconder as under the age of 26. Sandhu (1996) found the average age of the inmates he studied to be 27. Culp (2005), in his review of 88 escapes by 135 individuals, was able to identify the average age of 117 individuals as 27 years old. The State of New York Department of Correctional Services (2011) analysis of escapes between 2006 and 2010 found that, of the individuals who attempted to escape, 80% were less than 31 years old, while only 36% of the inmate population as a whole was less than 31 years old. Anson (1983) approached escape attempts from a slightly different perspective, but obtained similar results. He looked at 11 characteristics of 17 male prisons, such as age and size of the institution, ratio of staff to inmates, level of supervision, and age of their populations. He also found that more escapes were attempted where the inmate population was younger. Young age is consistently identified as an escape risk factor. Some possible explanations are that younger inmates have not been incarcerated long enough to adjust to the environment or that they are more physically capable to attempt an escape than older inmates. Adult Criminal History Inmates who escape tend to have committed more crimes in the past than inmates who do not escape. In a Kentucky Bureau of Corrections study (1978), only 16% of escapees were first-time adult offenders, while 30% of non-escapees were adult first-time offenders. Sixty-five percent of the walkaways in the Montiuk and Johnson (1992) study had 20 or more previous convictions as an adult. Juvenile Criminal History California Department of Corrections and Rehabilitation Office of Research/Research and Evaluation Branch Page 128 December 19, 2011 Several studies identify a link between a juvenile criminal history and adult prison escapes. Dobbins and Stockwell (1960), while not giving specific percentages, did find that escapees had significantly more juvenile commitments than non-escapees. Seventeen percent of the escapes in the Kentucky Bureau of Corrections (1978) had been incarcerated as juveniles, compared to 8% of non-escapees. In studying walkaways from a Canadian minimum security facility, Johnston and Motiuk (1992) noted that every walkaway had a juvenile conviction. The convicted murderers in Sandhu’s (1996) study were also described as having juvenile records, although no specifics were given. Property Crimes Property crimes were the most frequently mentioned crimes associated with the risk of escape (Gorta and Sillivan, 1991; Kentucky Bureau of Corrections, 1978; Johnston and Motiuk, 1992; Loving, Stockwell, Dobbins, 1959; State of New York Department of Correctional Services, 2011; Sturrock, Porporino, Johnston, 2008). A couple of reasons may explain the prevalence of property-related crimes in research about escapees. Because most escapes are from minimum-security settings, research has focused on minimumsecurity inmates who are less likely to have been convicted of violent crimes that would place them in medium- or maximum-security housing. Furthermore, escapees are more likely to be younger offenders who commit lower-level property crimes at an early stage in their criminal career. Although property crime is the most frequently identified crime that is associated with escapes, research has also been conducted on inmates convicted of violent crimes. Sandhu (1996) compared a group of 31 escapees convicted of first or second degree murder to 88 individuals with similar convictions who did not attempt escape. Escapees were found to be characterized by other risk factors, such as a more lengthy adult criminal record, a juvenile record, substance abuse problems, and more disciplinary actions than non-escapees. These are all risk factors of escapees convicted of property crimes, so the type of crime an individual was convicted of may not be useful in predicting escapes. In sum, the frequency of property crimes among escapees could be due to the fact that the escapees are typically housed in minimum-security settings, where most escapes occur. Length of Time Already Served The majority of studies that addressed time served indicated that escape attempts occur before inmates serve half of their sentence. Dobbins and Stockwell (1960) cited a 1948 study that found that most escapees had served less than 40% of their term. Morgan (1967) found that “significantly more” escapees served less than half their sentences than nonescapees. The average sentence of escapees studied by Johnston and Motiuk (1992) was four years, with the average length of time from admission to escape being 371 days, or a little over one year. Twenty percent of the escapees in Sandhu’s (1996) study escaped in the first year of sentences, ranging from 10 years to life or condemned to death. Gorta and Sillivan’s (1991) study of Australian escapees presents a mixed picture. They made two comparisons. The first compared a group of individuals who attempted to escape within two weeks of arrival to prison to those who attempted escapes after more than two weeks. The second compared a group of individuals who attempted to escape within 60 days of their release to those who attempted to escape who had more than 60 days left on their sentence. In both comparisons, inmates who tried to escape within two weeks of their California Department of Corrections and Rehabilitation Office of Research/Research and Evaluation Branch Page 129 December 19, 2011 arrival and those who had less than 60 days to serve were younger, had shorter sentences, were more likely to have had been convicted of a property-related crime, and had served less time on a prior sentence. The inmates who escaped early in their sentence fit the profile of a young inmate convicted of a property-related crime. Moore and Hammond (2000) did not find a link between time served and escape among individuals committed to secure psychiatric facilities for crime-related reasons. There have also been descriptive accounts of individual inmates spending years preparing for escapes by gaining the trust of prison staff with their good behavior (Singer, 2006). While research findings differ on the relationship between time served and escape risk, there is some support for the idea that inmates will attempt to escape before serving half of their sentence. One theory is that inmates eventually get “invested” in the time they are in prison; after serving most of their sentence they do not want to risk postponing their parole date. Previous Escape Attempts A number of studies demonstrated that previous escape attempts are an indication of a higher risk of future attempts. The Kentucky Bureau of Corrections (1978) escapees had 31% prior escapes compared to 6% of non-escapees. Over 40% of walkaways in one study had previously escaped at least one time (Johnston and Motiuk, 1992). Sandhu (1996) found that 20% of escapees made one or more previous attempts. Race/Ethnicity Several studies observed that more white inmates escaped than inmates who are members of racial or ethnic minority groups, both in number and percentage of the total inmate population. Loving, Stockwell and Dobbins (1959), in their study of 100 escapees from the Louisiana State Penitentiary, found that between 1949 and 1959, twice as many white inmates attempted to escape than black inmates, even though black inmates outnumbered white inmates by three to one. In Sandhu’s (1996) study of escapees convicted of murder or other violent crimes, 74% were white, followed by black (17%) and Native American (9%). Moore and Hammond (2000), in their study of criminal, mentally ill absconders from secured correctional mental health facilities, also found the majority of escapees were white. In both cases these rates were disproportionate to the racial composition of the population as a whole. More recently, though, Culp (2005) examined nationwide surveys of correctional agencies and found that racial differences in escapees are declining. The State of New York Department of Correctional Services (2011) reported that 60% of escapes were made by black inmates, who comprised 51% of the inmate population, 30% were by white inmates, (21% of the population), and 10% were made by Hispanic inmates, ( 26% of the population). Although Race/ethnicity was cited as an escape risk in older research, no studies were ever performed to find an explanation. Other Static Risk Factors Identified in the Literature Some other static risk factors mentioned in the literature include age at first arrest (Dobbins and Stockwell, 1960; Loving, Stockwell, & Dobbins, 1959), childhood abuse or neglect (Johnston and Motiuk, 1992; Morgan, 1967; Sandhu, 1996), and unstable work history California Department of Corrections and Rehabilitation Office of Research/Research and Evaluation Branch Page 130 December 19, 2011 (Johnston and Motiuk, 1992; Morrow, 1969). However, no research has been conducted to explain why these factors appear among inmates who escape. Dynamic Factors Associated with Escape Attempts Dynamic factors are those that can change, such as behavior while in prison or use of drugs. Although the research findings on dynamic risk factors are not as clear as those on static factors, the following are some dynamic factors that have been examined: Holds, Detainers, Denied Parole Inmates know their expected release date. The risk of escape increases when they are told pending legal action could interfere with that date. Loving, Stockwell and Dobbins (1959) cited a study from 1948 that found escapees were more likely to have detainers, but no specific numbers were provided. Being denied parole was the reason for attempting to escape given by 8.5% of the inmates who were interviewed in another study (Duncan and Ellis, 1973). The Kentucky Bureau of Corrections (1978) found that 34% of escapees, but only 12% of non-escapees, had time added to their stay in prison. Institutional Misconduct Some studies suggest that inmates who escape also get into trouble within the prison more often inmates who do not escape. The Kentucky Bureau of Corrections (1978) found that escapees tend to be in trouble with contraband more often than non-escapees. Over 75% of the walkaways in the Johnston and Motiuk (1992) study had one or more disciplinary reports. Sturrock, Porporino, Johnston (2008) cite a 1975 dissertation that concluded that inmates who escape have been placed in solitary confinement (comparable to CDCR Administrative Segregation or Security Housing Units) escape more than inmates who have not been placed in such housing. Relationship Problems Inmates may escape in order to deal with relationship or family problems, such as separation, divorce, illness or death in the family, problems with relatives, or economic difficulties of relatives. In one study these problems were given as the main motivation for escape by over 15% of inmates who had escaped and were then caught (Duncan and Ellis, 1973). When asked what was on their mind at the time of escape, 42% of walkaways cited family/marital problems (Johnson and Motiuk, 1992). Sandhu (1996) listed news of a wife’s illness and problems with a girlfriend among the reasons given by inmates for their escapes. Relationship problems have been shown to be a risk factor for escape attempts. Inmates will escape when faced with a personal problem that they believe they cannot address from a distance. Substance Abuse Escapees have been found to have substance abuse problems (Morrow, 1969; Johnston and Motiuk, 1992; Sandhu, 1996; Muir-Cochrane and Mosel, 1998). However, the significance of this is unclear. Definitions of substance abuse vary and many inmates who do not escape also have substance abuse problems. Studies have also produced conflicting results. Morrow (1969) reported higher rates of alcoholism in escapees (42%) compared to non-escapees (25%), while Sandhu however found that 29% of escapees were serious alcoholic abusers as opposed to 48% of non-escapees. Two studies suggested differences California Department of Corrections and Rehabilitation Office of Research/Research and Evaluation Branch Page 131 December 19, 2011 in the use of alcohol and drugs. The Kentucky Bureau of Corrections (1978) asserted that escapees were more likely to use alcohol and none-escapees more likely to use drugs, although no data were provided to support this. Sandu (1996) found that 32% of escapees were serious drug users, compared to 47% on non-escapees. Other Dynamic Risk Factors Identified in the Literature Additional dynamic risk factors identified in the literature include problems with prison staff or dissatisfaction with available programs or services (Duncan and Ellis, 1973; Sandhu, 1996). Anson (1983) and Johnston and Motiuk (1992) identified too much free time or boredom as reasons why inmates escaped. Despite its notable limitations, the research literature on inmate escape risk factors identifies some factors repeatedly. Age at escape attempt is the most consistently documented static factor. There is research to support adult and juvenile criminal histories and length of time served, but the evidence is not as strong. Although dynamic escape risk factors have not been studied in depth, a few appear consistently in the literature. Family or relationship problems are the most documented dynamic risk factors. Comparison Between Escape Research and CDCR Close Custody Criteria Table A compares CDCR’s Close Custody Regulations with the static and dynamic escape risk factors. As depicted in this table, CDCR’s Close Custody criteria are, for the most part, supported by some research. All criteria except High Notoriety/Public Interest/Management Concern have been researched as possible escape risk factors. However, there is not enough research to conclusively state that the criteria are evidence-based. Furthermore, there are escape risk factors found in the literature that are not addressed by the CDCR Close Custody criteria. Age and relationship problems might be explored as additional criteria, although the latter could be difficult to objectively define or identify. California Department of Corrections and Rehabilitation Office of Research/Research and Evaluation Branch Page 132 December 19, 2011 Table A Escape Risk Factor Static Age Adult Criminal History Juvenile Criminal History Property Crimes Length of Time Already Served Previous Escape Attempts Race/Ethnicity Dynamic Holds, Detainers, Denial of Parole Institutional Misconduct Relationship Problems Substance Abuse High Notoriety/Public Interest/Management Concern CDCR Close Custody Criteria x x x x Risk Factors Identified in the Literature x x x x x x x x x x x x Do the current regulatory criteria for Close Custody accurately identify escape risk potential based upon evidence-based practices? In sum, there is not enough empirical research available to confirm whether CDCR’s Close Custody criteria are evidence-based, although there is some research that implicitly supports them. This does not mean they are wrong, but rather that there is not enough research to confirm that they are or are not the right criteria to use. As a result, decisions to maintain or change current CDCR Close Custody practices should not be based upon the available research alone. California Department of Corrections and Rehabilitation Office of Research/Research and Evaluation Branch Page 133 December 19, 2011 Bibliography Anson, R. H. (1983). Correlates of Escape: a Prelimiary Assessment of Georgia Prisons. Criminal Justice Review, 38-42. Culp, R. F. (2005). Frequency and Characteristics of Prison Escapes in the United States: an Analysis of National Data . Prison Journal, 85 (3), 270-291. Dobbins, D., & Stockwell, F. (1960). Individual and Social Correlates of Prison Escapes. Journal of Consulting Psychology, 24 (1), 95. Duncan, D., & Ellis, T. (1973, May-June). Sitiuational Variables Associated with Prison Escapes. American Journal of Corrections, 29-30. Gorta, A., & Sillavan, T. (1991). Escapes from New South Wales Gaols: Placing the Risk in Pespective. ANZJ Crim, 24, pp. 204-218. Johnston, J. C., & Motiuk, L. L. (1992). Factors Related to Unlawful Walkaways from Minimum Security Institutions. Correctional Service Canada. Kentucky Bureau of Corrections. (1978). Blackburn Correctional Complex Escape Study 1978. Loving, W., Stockwell, F., & Dobbins, D. (1959). Factors Associated with Escape Behaviour in Prison Libraries. Federal Probation, 23 (3), 49-51. Moore, E., & Hammond, S. (2000). When Statistical Models Fail; Problems in the Prediction of Escape and Absconding Behaviour from High-Security Hospitals. Journal of Forensic Psychiatry, 11 (2), 359-371. Morgan, D. (1967). Individual and Situational Factors Related to Prison Escapes. American Journal of Correction, 29 (2), 30-31. Morrow, W. R. (1969). Escapes of Psychiatric Offenders. Journal of Criminal Law, Criminology, and Police Science, 60 (4), 464-471. Muir-Cochrane, E., & Mosel, K. (1998). Absconding: a review of the Literature 1996-1998. International Journal of Mental Health Nursing (17), 370-378. Sandu, H. (1996). A Profile of Murderer Escapees. Journal of the Oklahoma Criminal Justice Research Consortium, 3. Singer, M. (2006, October 9). Escaped. New Yorker, 82 (32). State of New York Department of Correctional Services. (2011). Inmate Escape Incidents 20062010. Sturrock, R. C., Porporino, F. J., & Johnston, J. C. (2008). Literature Review on the Factors Related to Escape from Correctional Institutions. Correctional Service Canada. California Department of Corrections and Rehabilitation Office of Research/Research and Evaluation Branch Page 134 December 19, 2011 Appendix N Literature Review on Misclassification Executive Summary To support the current California Department of Corrections and Rehabilitation (CDCR) Inmate Classification Score System Study, this literature review examines research on inmate misclassification and presents information on current steps other state correctional systems are taking to reduce their inmate population. A total of 26 journal articles, research reports and government reports formed the basis of this review. Additional information was obtained from conversations with officials in other state correctional systems. Misclassification • Misclassification research focuses more on overclassification than underclassification. • Factors in misclassification can be broadly separated into three groups: Convictions and Sentencing Basing classification decisions on the type of crime for which an individual was convicted and the length of their sentence. Behavior as a criminal, especially with respect to violent crimes such as murder, may not be indicative of behavior as an inmate, and longer sentences do not necessarily lead to more misconduct. Inappropriate Use of Demographic Factors Unaddressed mental illness has been found to increase misconduct, leading to higher classification requirements, and classification systems created for male inmates may or may not be applicable to female inmates. Staff Use of Classification Systems Effective use of any classification system is all too often hindered by inadequate training, limited experience, and the use of subjectivity in making classification decisions. • Underclassification appears to sometimes foster better behavior. Inmates placed in less restrictive housing than their classification score requires tend to behave no worse than the inmates appropriately placed in that environment and they also tend to have lower recidivism rates. • Overclassification appears to be criminogenic, i.e., it is associated with increased criminal behavior. Inmates placed in more restrictive housing than their classification score requires will act like the inmates who were appropriately housed in restrictive housing, and inmates who were overclassified return to prison more than inmates who were correctly classified. • Although research is somewhat limited, it is clear that misclassification is a serious problem. Although underclassification can be a problem, it appears to be relatively insignificant compared to the repercussions of overclassification. In-prison behavior and recidivism appear to be lower when inmates are placed in less secure settings than in more secure ones. California Department of Corrections and Rehabilitation Office of Research/Research and Evaluation Branch Page 135 December 19, 2011 • A number of factors need to be considered in reducing misclassification: 1) Basing inmate supervision levels on violent crime convictions does not correctly identify risk of institutional violence nor recidivism; 2) Insensitivity to the impact of mental illness on behavior can produce incorrect classification decisions; 3) Female classification can probably be improved if based on need factors, and not solely behavior. Research indicates that a classification system should have the following components in order to minimize the risk of misclassification: Implementation of a classification system that has been proven to be both valid and reliable. Full automation and recording of each classification decision. A centralized, adequately staffed classification unit that is responsible for monitoring and preparing policies and procedures. A process for the initial and annual reclassification of each inmate. Use of over-rides for agency-approved reasons. Recent Developments in State Correctional Classification Systems • By the first decade of the 21st century, many state correctional agencies began efforts at validating their classification systems, exploring the need for gender-responsive classification systems, and determining how to best use computer systems in all areas of classification work. These concerns were prompted by sentencing laws such as ThreeStrikes, which increased the prison population (including a growing female population), litigation by inmates regarding conditions of confinement, and the increasing use computer systems. • Currently the majority of state correctional agencies are focused on reducing costs, but are not looking to their classification systems to achieve this reduction. Rather, they are expanding eligibility for programs that allow inmates to earn sentence reductions and developing programs for parolees that are intended to reduce the number returning to custody. Most of new programs were initiated in the last two years. Their actual effect on the size of the inmate population remains to be seen. CDCR’s Inmate Classification System Study is characteristic of the research on classification found in the literature. It uses data analysis rather than experimental designs with randomized groups. Its Mandatory Minimum Scores, which require inmates to be placed in security levels based on their convictions for specific crimes, is not evidence-based. It is important to note that research suggests that overclassification may be criminogenic for some inmates. Unique among state correctional agencies, CDCR is proposing to amend its classification system in order to relieve overcrowding. This would come about by moving appropriately reclassified inmates from more-crowded secure-housing units to the less-crowded facilities for lower-level offenders. . California Department of Corrections and Rehabilitation Office of Research/Research and Evaluation Branch Page 136 December 19, 2011 Literature Review on Misclassification Full Review Introduction Inmate classification systems are developed and implemented to assign inmates to custodylevel appropriate placements that maximize safety and security for the staff and inmates within and the community without its walls. The literature sometimes discusses two types of systems: external and internal. An external system places an inmate in a prison, while an internal system places an inmate in housing and programming within a prison. Within the California Department of Corrections and Rehabilitation (CDCR), the external system is characterized by the pointand-level Inmate Classification Score System. The internal system is characterized by the CDCR Close Custody Designations. The consensus in the literature is that external systems are fairly well developed while internal systems need further refinement. Current CDCR projections anticipate great availability of lower security level housing in the near future due to the transfer of lower custody-level inmates to the counties. In order to maximize the use of this anticipated housing space, CDCR is examining both the internal and external components of its classification system. The purpose of this literature review is to inform this process. In particular, this literature review addresses two issues regarding inmate classification systems: 1) misclassification, and 2) what other states are doing with their classification systems. The first issue, misclassification, refers to the incorrect placement of an inmate in a prison. Overclassification refers to the placement of an inmate in a setting that is unnecessarily restrictive; underclassification refers to a placement in a setting that does not provide adequate security and supervision. Misclassification may be due to incorrect classification decisions or to deficiencies in a classification system. The second issue concerns the changes that have occurred in the past few years to classification systems in other states. Methodology The following databases were searched for relevant literature on inmate classification systems: • Academic Search Complete • JSTOR • Google Scholar • MEDLINE with Full Text • PsychARTICLES • PsychINFO • Psychology and Behavioral Sciences Collection • SocINDEX with Full Text California Department of Corrections and Rehabilitation Office of Research/Research and Evaluation Branch Page 137 December 19, 2011 The search terms varied across the databases (which varied in design and subject terms), but the predominant terms used were: • Prisoners and Classification • Criminal Rehabilitation • Female Offenders and Classification • Prisoners and Mental Health and Classification Free-text searching terms included: • Misclassification and Prisoners • Underclassification and Prisoners • Overclassification and Prisoners Criteria for inclusion in order to focus on misclassification: • Adult jail or prison inmates • Classification policies that are not evidence-based • Factors that negatively affect the quality of classification actions, such as subjectivity • Factors that may have been incorrectly or inadequately addressed in classification systems, such as mental illness or gender Criteria for exclusion that do not address misclassification: • Descriptive-only reports on current systems that did not address misclassification • Experimental designs of proposed classification systems • Studies of statistical or other methodologies used to validate systems Fifty-four articles or reports were selected and either downloaded or ordered from the State Library. After this initial review, 26 were found to be relevant. Publication dates were between 1970 and 2011. Additional information on recent efforts by other state correctional systems to address overcrowding was obtained through telephone and email conversations with Classification staff in the New York State and Illinois Departments of Correction. I. Misclassification Inmate classification systems basically implement correctional policy and, therefore, tend to reflect the concerns of the era in which they were developed. Concerns about “super predators” in the 1980s and 1990s are reflected in more restrictive classification systems. Later research has found that the actual threat posed by super predators was much less than originally thought and was based largely on anecdotal evidence that the mass media magnified into mythic proportions. By the first decade of the 21st century, classification research and development began focusing on validating classification systems, exploring the need for gender-responsive classification systems, and automating all areas of the classification process. These concerns have been prompted by sentencing laws such as Three-Strikes, which increased the prison population (including a growing female population), litigation by inmates regarding conditions of confinement, and the increasing use of computers in correctional work. More recent attention to prison costs and lack of rehabilitative services have prompted correctional researchers and policy makers to look at ways to reduce inmate restrictions and at the same time maintain – or even increase -- institutional and community (public) safety. California Department of Corrections and Rehabilitation Office of Research/Research and Evaluation Branch Page 138 December 19, 2011 No single article identified for this literature review focused solely on underclassification. Where underclassification is discussed, it is primarily in terms of something to avoid. Berk (2006) in his study of the CDCR classification system described how CDCR officials preferred the possibility of ten inmates being placed in overly restrictive housing than to have one inmate placed in housing that was not restrictive enough. Furthermore, there may be some reluctance to publishing studies on underclassification as it would imply a failure in public safety. On the other hand, overclassification is discussed from a number of angles, which will be described below. While overclassification may prompt litigation, exacerbate mental illness and even increase recidivism, it is not seen as an immediate threat to public safety. Limitations in the Research Limited Research Experimental research on misclassification, in which inmates are randomly assigned to study and control groups and subjected to various conditions to test their responses, is extremely limited due to safety and legal concerns. Most studies analyze data on previous behavior of different groups of inmates. Therefore, study findings for the most part are predictions of possible future behavior, not assessments of actual recent behavior. There is limited empirical research (experimental or observational) to support classification practices and policies that are intended to control inmate behavior. In their meta-analysis, Byrne and Hummer (2007) only identified seven research studies published between 1984 and 2006 that examined the relationship between classification decisions and inmate behavior. They concluded that the classification systems they examined do not predict inmate behavior and do not reduce prison violence. Sample Sizes The number of subjects in studies ranged from widely. The percentage of the total inmate population within a system used for research also varied. As a result, it is difficult to compare and generalize findings. Definitions of Behavior Differ The literature on classification frequently refers to violent misconduct on the part of prison inmates. However, the specific behavior is usually not stated. It could be murder, assault, pushing or shoving, taking part in a group disturbance under pressure from other inmates, etc. It is also important to note that behavior that is tolerated without taking disciplinary measures in one correctional system can cause disciplinary action in another. Also, within one system, individual institutions, and even individuals within an institution, can differ in their disciplinary attitudes. Inmate Populations May Vary The characteristics of inmate populations (e.g., race, ethnicity, age) vary based on the geographic location and age of the literature. Research conducted in southern states typically refers to white and black inmates, while newer reports on inmates in southwestern or western states include white, black, and Hispanic inmates. Correctional System Organization The majority of the articles in this literature review focus on state prison inmates. However, states differ in how their prisons are organized and how their offenders are sentenced. For example, individuals classified as minimum security inmates in California prisons may be placed in state jails in Texas or in community correctional facilities in Ohio. California Department of Corrections and Rehabilitation Office of Research/Research and Evaluation Branch Page 139 December 19, 2011 Contributing Factors to Misclassification The available research has identified multiple factors that contribute to misclassification. They can be broken into three categories: convictions and sentencing, inmate demographic factors, and staff use of classification systems. Convictions and Sentencing Criminal Conviction Superficially it may appear logical that an individual convicted of a violent crime like murder requires the strictest level of supervision in prison. However, research does not definitively support a connection between behavior as a criminal and behavior as a prison inmate. Research has shown that classification policies based on conviction for violent crimes result in overclassification. Individuals convicted of violent crimes tend to be better behaved in prison than individuals convicted of nonviolent crimes (Waldo, 1970; Alexander, 1994; Austin, 2003; Sorenson and Cunningham, 2010). For example, one study found that individuals convicted of murder were 8.5% less likely to commit rule violations that are defined as having the potential to result in a violent outcome when compared to inmates with drug, property, or public order convictions. Sentence Length “Nothing left to lose” is a phrase used when discussing inmates with life sentences, or “LWOPs” (life without possibility of parole). LWOPs are seen as inmates who have no incentive to behave because they have no possibility of leaving prison. However, the little research that has been conducted on the relationship between length of sentence and behavior found that sentence length is not a predictor of in-prison behavior (Austin, 2003; Cunningham and Sorensen, 2006). Cunningham and Sorenson (2006) compared the behavior of inmates sentenced to life without the possibility of parole to the behavior of inmates with various sentences ranging from 10 to 30 or more years. The LWOPS had the same average age at admission as those in the comparison groups and were similar demographically in other respects as well. They found that LWOPS were 21% less likely to engage in violent behavior than inmates serving 10 – 14 years, 15% less likely than inmates serving sentences of 15 – 19 years, and 7% less like than inmates serving sentences of 30 or more. Inappropriate Use of Demographic Factors Mental Illness Mental illness contributes to preventable overclassification of some inmates. Those with mental illness are more likely to misbehave than those who do not suffer from mental illness and, consequently, are more likely to be disciplined more often. Their classification scores rise, which can lead them to be placed in adminstrative segregation. This restrictive environment can exacerbate their mental illness, and thus lead to more misbehavior. Kupers (2009) described this downward spiral of mentally ill inmates in his report on administrative segregation (Ad Seg) in Mississippi. The litigation that prompted the Mississippi Department of Corrections (MDOC) to create a new classification system also resulted in mental health treatment for inmates in Ad Seg who have been diagnosed as being seriously mentally ill. The Correctional Association of New York (2004) also studied mentally ill inmates in New York State prisons by reviewing records and conducting interviews with staff and inmates. Findings were that inmates with known mental health issues comprised approximately 11% of the total inmate population, but represented 20% to 60% of the population in secure housing, depending on the institution under examination. Recommendations resulting from California Department of Corrections and Rehabilitation Office of Research/Research and Evaluation Branch Page 140 December 19, 2011 this study included expanding treatment programs, training correctional officers in mental health issues, creating suicide prevention programs in all secure units, and creating an independent review board to ensure that reforms are actually made. Due to litigation and changes in the law that took place in 2008 and 2009, the New York State Department of Corrections has expanded treatment for mentally ill inmates. For example, the Special Housing Unit (SHU) Exclusion Law of 2008 provides for alternative placement for all inmates with serious mental illness and disciplinary confinement requirements. A contributing factor to the over-respresentation of mentally ill inmates in Ad Seg is the “bad versus mad” dilemma (Toch, 2007). This occurs when custody staff refer a mentally ill inmate who is difficult to manage to the mental health staff for treatment. Mental health staff will then affirm that the inmate is mentally ill, but state that his misbehavior was not due to his illness, but rather due to malingering. It appears that custody staff and mental health staff each want to the other to take responsibility for managing these inmates. Gender The increase in the female prison population over the past two decades created an interest in gender-responsive classification of female inmates. All states use some form of classification system for female, as well as male, inmates. Most use the same system for both genders, but questions have been raised about the validity of this practice. In an examination of 10 state classification systems, Hardyman, Austin and Tulloch (2002) found that 4 of 10 states that were in the process of revalidating their classification systems wanted to assess the need for either a separate system for female offenders, or potential modifications to current systems for female classification. Oklahoma concluded that modifying the cutoff points between classification levels in their current system for women was appropriate, but a separate system was unnecessary. Oregon, Rhode Island, and Virginia did not find a need for separate systems or modifications to their current systems. Montana, while not making gender issues central to their research, found that a newly developed instrument to assess predatory behavior was valid for male and female inmates. Van Voorhis et.al., (2001) identified the following concerns with female offenders through a survey of all 50 state correctional systems: • Ten states overrode more than 15% (rates ranged from 18 to 70%) of female inmates’ classification scores, which indicates that their systems were not working well with women. • Respondents from 49 states identified needs and problems that they believed were unique to female inmates, such as the need for trauma treatment and the impact of their incarceration on their children, but only 8 states attempted to classify female inmates differently from male inmates. While research has demonstrated that female inmates were violent less often than male inmates, seven risk predictors of assault (severity of prior offense, severity of current offense, number of prior felony convictions, current age, stability factors, escape history, and history of institutional violence) were the same for both genders (Van Voorhis, et.al., 2001). Research on female offender classification differs from research on male offender classification in that it sometimes includes recommendations to add a component based on needs such as mental health treatment, substance abuse treatment, or recovery from abuse (Austin, 1993; Farr, 2000; Hardyman, 2002). No explanation is given as to why these needs should be addressed for women, but not men. California Department of Corrections and Rehabilitation Office of Research/Research and Evaluation Branch Page 141 December 19, 2011 At this time the literature does not provide conclusive evidence that female inmates require their own classification system; however, research strongly suggests a need to at least include gender-responsive items for female offender classification. Staff Use of Classification System Inadequate Staff Training and Experience Any classification system is only as good as the people using it. Inadequate training and/or experience will affect the quality of classification decisions. A number of studies emphasize the need for strong training and ongoing monitoring to ensure the system is used correctly (Alexander, 1994; Owens, Will and Camp, 1995; Austin, 2003). Subjectivity Kupers (2009) described Mississippi’s experience with the overclassification of inmates assigned to the Ad Seg unit in the Mississippi State Penitentiary. Prior to 2002, classification was based on subjective decision-making. In response to litigation, the MDOC, working in conjunction with Dr. James Austin, created a new, objective classification system. After its introduction, more than 75% of Ad Seg inmates were reclassified to a lower security level. After these inmates were moved to less restrictive housing, serious incidents in the institution fell by 70%. As of 2009, 1% of the inmates in the Mississippi State Penitentiary were in Ad Seg, compared to 3% of inmates in other states’ prisons. Bonta and Motiuk (1990) found that when subjective assessments were used, 16% of jail inmates were found to be eligible for halfway houses. However, when an objective assessment instrument was used, 51% were considered eligible. Austin and Chan (1993), in a study on classifying female inmates, revealed that 40% of all classification decisions in the Indiana Department of Corrections were overrides (classification decisions that conflict with established criteria), 37.8% of which were upward (primarily from minimum to low-medium and high-medium to maximum). Potential Consequences of Misclassification There are a number of possible consequences of misclassification, ranging from better-thanexpected behavior to murder. In Alexander’s (1994) study, prison staff and inmates in Pennsylvania and Nevada were interviewed as part of a qualitative assessment of classification. Instead of using statistical analysis, Alexander looked at a small set of cases in order to discern factors that influence inmate behavior. He looked at both under- and overclassified individuals. His findings were that most high-custody inmates are not involved in serious misconduct, but have higher infraction rates than inmates housed in lower custody settings. Lower custody inmates who engaged in serious misconduct had lower infraction rates than inmates housed in high security settings. Underclassification The findings of research on underclassification are mixed. There is some research indicating that there were no significant differences in overall or serious misconduct among inmates who were housed in environments that were less restrictive than their classification level would suggest necessary (Bench and Allen, 2003; Camp and Gaes, 2005). Fewer restrictions did not result in more misbehavior. However, underclassification can result in obvious problems. One example cited in the literature is that regarding a private prison in Youngstown, Ohio that housed 1,700 male offenders from the District of Columbia (Clark, 1998). A new security level, “high medium,” was informally California Department of Corrections and Rehabilitation Office of Research/Research and Evaluation Branch Page 142 December 19, 2011 created because the private prison’s contract prohibited accepting high security; excessive overrides were used and 274 inmates who had been classified as maximum elsewhere became high medium. In addition, over 200 inmates who should have been kept separate due to enemy concerns were mixed in with the population as a whole. After two murders, several riots, and the escape of 6 inmates at once, inmates were reclassified, resulting in 19 transferring to maximum security settings. Misclassification, however, was not the only problem at this prison and it is not clear to what degree it contributed to the behaviors mentioned above. Overclassification Overclassification may have an effect on inmates beyond their time in prison. Chen and Shapiro (2004; 2007) conducted a study on the Federal Bureau of Prisons classification system, which has five levels: minimum, low, medium, high, and administrative. They found that moving an inmate from a minimum security (least supervised) to a low security (one level more supervised than minimum) setting doubles the inmate’s chances of being rearrested within three years. Gaes and Camp (2009) found similar results in their study on a group of CDCR Level III inmates, some of whom were randomly assigned to Level III environments and some of whom were placed in Level I environments. Level III inmates housed in Level III settings had a 31% higher chance of returning to prison than Level III inmates in Level I settings. These studies suggest there may be some truth to the cliché about prison being a crime school. Inmates who are overclassified may learn new criminal behaviors through interaction with more experienced criminals. Components of Classification Systems That Can Minimize Misclassification Research has been conducted to identify the components needed for a classification system to minimize the risk of misclassification. Austin (2003), under the auspices of the National Institute of Corrections (NIC), concluded the following components are crucial to have in a classification system in order to reduce the potential for misclassification: • The use of criteria that have been demonstrated through research to use both reliable and valid factors to assess a prisoner’s custody level; • A centralized classification unit that is adequately staffed with well trained professional personnel who have control over all inter-agency transfers; • A centralized classification unit that is responsible for monitoring institutional classification units and preparing all policies and procedures that pertain to classification; • A fully automated classification system such that each classification decision, and the factors used to make each decision, is recorded and available for analysis; • An initial and reclassification process where all prisoners are reviewed at least annually to update and possibly modify the prisoner’s current classification level; and, • The use of over-rides to allow staff to depart from the scored classification level for reasons approved by the agency. II. Recent Developments in State Classification Systems The second part of this literature review looks at recent changes to state classification systems and how individual state correctional departments are attempting to modify their policies in light of current economic hardships. In the first few years of the 21st century, state correctional departments were eager to analyze, validate, and improve their classification systems. A tremendous growth in inmate population California Department of Corrections and Rehabilitation Office of Research/Research and Evaluation Branch Page 143 December 19, 2011 due to truth-in-sentencing and three strike laws, more female inmates, and the building of “supermax,” expensive maximum custody housing contributed to this interest. Other reasons include inmate lawsuits regarding living conditions and the growing use of automated systems. The National Institute of Corrections (NIC) funded a 15-month studiy in which 10 states addressed issues regarding their classification systems (Hardyman, Austin and Tulloch, 2002). States volunteered to take part in the studies and were selected based on their commitment to analyzing and improving their classification systems and practices. NIC technical specialists assisted the participants in identifying goals and methologies, and provided training for any new practices resulting from the studies. The final study report illustrated both the similarity and diversity of issues state correctional departments faced with respect to their classification systems. Each state had its own reasons for participating, but the most frequent was the need to validate their classification system. Delaware Department of Corrections This department’s goal was to design and validate an objective classification system in order to improve the efficiency and effectiveness of its classification practices. Initial and reclassification custody assessment instruments were developed and refined to create statistically strong instruments for identifying the safety and security risk an offender poses. The combination of the factors of current offense, other offense/bail status, escape history, current age, criminal history, time to serve and institutional program performance was found to be effective in assessing an inmate’s level of risk. The new classification tools were included in the department’s automated information system. Montana Department of Corrections In response to litigation, the department agreed to modify its classification policy and instruments to identify predatory and vulnerable inmates. Data analyses demonstrated that the modified instrument differentiated predatory and vulnerable inmates and suggested that it was appropriate for both male and female inmates. Oklahoma Department of Corrections This department’s goal was to refine its classification risk factors to better assess the risks posed by female offenders. In particular, the age, current offense, criminal history, and escape items were revised. The custody scale cut points were also adjusted to create statistically distinct custody levels. Oklahoma has implemented the revised instruments and custody scales and addressed the availability of its services and programs to ensure that women are placed within the least restrictive custody level possible. Oregon Department of Corrections Alternative classification scoring criteria were created and simulated in order to assess the negative impacts of a truth-in-sentencing initiative and other sentencing reforms that had altered the number and type of offenders and expected lengths of stay. Because the classification system in place at the time relied heavily upon an inmate’s expected time to serve, it was anticipated that the system would overclassify its inmate population. As a result of this validation effort, minor revisions to the instruments and custody matrix were implemented. The department also studied the need for a separate classification system for women, which led to plans to pilot test Rhode Island Department of Corrections The department wanted to revalidate and adjust its objective classification system to ensure its appropriateness for both male and female inmates and to design a systematic California Department of Corrections and Rehabilitation Office of Research/Research and Evaluation Branch Page 144 December 19, 2011 administrative review process to document the transfer to minimum custody of inmates with sentences of less than 6 months. Analysis of the existing system suggested the need to create a subset of predatory institutional infractions to identify aggressive inmates, develop an offense severity index that focused on institutional risk, and modify the custody scale cut points. Pilot testing of the administrative screening process suggested that the instrument would serve as a simple, objective mechanism for identifying cases appropriate for minimum custody. The analysis also suggested the need to revise the department’s disciplinary code; develop a public safety screening instrument for work release and community housing decisions; discontinue regular custody assessments for minimum-custody inmates; restrict discretionary overrides; provide intensive, ongoing training to all classification staff; develop a strong, centralized classification unit; and upgrade the automated information system. Tennessee Department of Correction Before this study, the department discontinued the use of an initial classification instrument because it had been found to frequently overclassify inmates. For example, over 90% of inmates who were initially classified as close custody when they were received by the department dropped to minimum custody after four months (the time of their first reclassification). While a revised initial classification tool had been developed, the department wanted to determine if it should use it or continue without an initial classification form. Findings indicated that the revised instrument was an improvement over the original. Following some minor adjustments, plans were made to begin using the new initial classification instrument. Texas Department of Criminal Justice Policies, procedures, and operations pertaining to inmates in Ad Seg were reviewed with the goal being to reduce the number of inmates in this type of housing. Based on statistical data and a review of departmental policies, the following recommendations were made: continue single-celling, revise the criteria for placement and retention; and develop ongoing management reports to monitor and evaluate the Ad Seg policies. In addition, it was suggested that an anti-gang housing unit program be created. Virginia Department of Corrections This department wanted to create an instrument that would tie basic classification information to housing and work/program assignments. Information on an inmate’s demographic factors and criminal and incarceration history were used to assess security needs. This information was then used to make decisions on housing and education or work assignments. The preliminary instrument was tested and refined. Six levels of risk and appropriate housing based upon risk were identified. The department adopted use of this new system. The department also wanted to know if it should create a separate classification for female inmates. The new system was found to be valid for women as well as men, and it was determined that a separate system was not needed. Wisconsin Department of Corrections The reliability and validity the department’s classification system were assessed, as well as prevalence of racial bias in the system and the frequency of discretionary overrides. The analyses indicated that the system was reliable; however, the validity of the risk assessment was questionable and it appeared that the system overclassified many inmates. For example approximately 10% of the inmate population were classified as high risk but assigned to California Department of Corrections and Rehabilitation Office of Research/Research and Evaluation Branch Page 145 December 19, 2011 minimum custody. No racial bias was evident in the risk or Custody Designation process because equal proportions of black and white inmates were assigned to the various risk and Custody Designations. Key recommendations for improving the reliability, validity, and automation of the system were provided. Stricter controls on overrides and additional training for staff were also suggested. Wyoming Department of Corrections This department had two goals: assistance with staff training and the development of organizational and/or procedural changes necessary for the full implementation of their classification system. Intensive staff training that included reliability testing was provided, as was a detailed classification manual. A comprehensive classification policy that provided for a centralized classification unit and independent audits to periodically review a random sample of the classification instruments for accuracy and completeness was implemented. Audits completed at the four facilities indicated scoring error rates of less than 10%. The Current Situation Large correctional systems are a financial burden to state governments. Recent economic conditions have prompted state correctional departments to reevaluate their management of inmates. The Vera Institute’s national survey, The Continuing Fiscal Crisis in Corrections: Setting a New Course (2010), in which 44 states participated (California did not), identifed short-term and longterm efforts being made to reduce costs. In the short-term, every state was implementing at least one type of cost-cutting measure, the most common being staff reductions or hiring freezes. California Department of Corrections and Rehabilitation Office of Research/Research and Evaluation Branch Page 146 December 19, 2011 States are hoping to reduce costs long-term in other ways, as described below: Reducing Technical Violations: • Alabama now limits to 90 days the sentences for eligible nonviolent technical offenders; • Kentucky allows its parole officers to sentence violators to county jail for up to 10 days, with a total of 30 days per year; • Colorado allows community punishment for low-level nonviolent offenders; • Iowa can either revoke probation or extend it for up to one year. Alternatives to Incarceration: • Vermont has made probation the standard sentence for misdemeanors and nonviolent felonies; • Florida eliminated prison sentences for certain nonviolent felonies; • Louisiana and Vermont now allow courts to sentence offenders to home confinement; • Florida now diverts nonviolent offenders to recidivism reduction programs; • Vermont created a system of community reparative boards to determine alternative sentences for nonviolent offenders; eligibility for alternative sentencing has also been expanded to second time offenders; • South Carolina crated alternative sentences for some drug offenses; • Washington created alternative sentences for nonviolent offenders who have custody of children under the age of 18. Relaxing Mandatory Sentences: • New York eliminated mandatory minimum sentences and reinstated judicial discretion in low-level drug cases; • New Jersey amended its drug laws to allow judges to apply mandatory minimum sentences or probation for certain offenses; • Minnesota allows judges to deviate from mandatory minimum drug cases if the prosecutor requests it; • Rhode Island eliminated some mandatory minimum sentences; • Delaware amended its mandatory minimum sentencing policies by allowing courts to alter sentences of one year or less for individuals with serious medical problems requiring continuous treatment. Expanding Release Opportunities: • Oregon and Mississippi expanded eligibility for education-based credits to inmates convicted of high-level offenses; • Louisiana made good-time credits retroactive to 1992, except for inmates convicted of violent crimes and sex offenders; • Colorado increased the number of days nonviolent, program-compliant inmates can earn to reduce their sentences each month from 10 to 12 days. Restructuring Conditions of Release: California Department of Corrections and Rehabilitation Office of Research/Research and Evaluation Branch Page 147 December 19, 2011 • Indiana now requires inmates who have served 21 years and earned four years of credit time to be referred to the parole board for possible release; • Kansas and New York created early release programs for terminally ill inmates who are incapacitated and pose no threat to the community; • Kentucky allows nonviolent offenders convicted of low-level felonies with 180 days left on their sentences to be released to home incarceration; • South Carolina now requires nonviolent offenders who have been in prison for at least two years to be released before their release date; this state has also expanded eligibility for parole or work release within three year of release date to include inmates convicted of drug offenses; • New Hampshire now automatically releases inmates who have not previously paroled within nine months of their maximum sentence; • Louisiana lowered requirements for parole, now requiring only a majority vote by parole board members instead of unanimity; • West Virginia created an accelerated parole program whereby inmates are eligible for early release if they complete individualized rehabilitative programs; this state has also expanded annual parole reviews to eligible individuals who are serving life sentences; • Vermont now releases all nonviolent offenders to furlough programs if they have served their minimum sentences and completed rehabilitative program goals; individuals are now eligible within 180 days before the end of a minimum sentence, instead of the previous 90 days. It is important to note that all of these strategies to reduce state prison populations were enacted either in 2009 or 2010. It is too soon to tell what kind of impact they are having. The actual number of inmates who are leaving prison early or who do not recidivate due to these policy changes has not yet been determined. In addition, states may not be able to fully implement these initiatives due to political pressures. For example in 2009, Illinois developed a program that allowed some offenders to earn credits to shorten their sentences. Inmates who were convicted of driving under the influence (DUI) were included in the category of nonviolent offenders who qualified for the program. Public anger over letting felons with DUIs out early became so intense that the program was shut down. It remains to be seen how many of the states’ new efforts to reduce the prison population will actually achieve their goals. Conclusion Although research is somewhat limited, it is clear that misclassification is a serious problem. Although underclassification can be a problem, it appears to be relatively insignificant compared to the repercussions of overclassification. In-prison behavior and recidivism appear to be lower when inmates are placed in less secure settings than in more secure ones. A number of factors need to be considered in reducing misclassification: 1) Basing inmate supervision levels on violent crime convictions does not correctly identify risk of institutional violence nor recidivism; 2) Insensitivity to the impact of mental illness on behavior can produce incorrect classification decisions; 3) Female classification can probably be improved if based on need factors, and not solely behavior. California Department of Corrections and Rehabilitation Office of Research/Research and Evaluation Branch Page 148 December 19, 2011 The research literature is clear regarding the components required for a classification system that minimizes misclassification. These components are: 1) The classification system must be one that has been proven to be both valid and reliable on similar populations; 2) It must be fully automated and must record each classification decision; 3) It must be operated from an adequately staffed, centralized unit that is given the responsibility and authority for its monitoring as well as for the development of its policies and procedures; 4) It must include a process for the initial and the regular reclassification of each inmate on at least an annual basis and; 5) Its use of over-rides must be limited to agency-approved reasons. California Department of Corrections and Rehabilitation Office of Research/Research and Evaluation Branch Page 149 December 19, 2011 Bibliography Alexander, J. A. (1994). Understanding Why Inmates are Misclassified; the Use of Qualitative Methods in Evaluating Objective Prison Classification Systems. National Council on Crime and Delinquency. Annin, P. (1996, January 22). 'Superpredators' Arrive. Newsweek, 127 (4), p. 57. Austin, J. (2003). Findings in Prison Classification and Risk Assessment. Issues in Brief, National Institute of Corrections. Austin, J., and Chan, L. E. (1993). Indiana Department of Corrections Women Classification Study. National Council on Crime and Delinquency. Bench, L. L., and Allen, T. D. (2003). Investigating the Stigma of Prison Classification. Prison Journal, 83 (4), 367-382. Berk, R. A. (2006). Forecasting Dangerous Inmate Misconduct. University of California . California Policy Research Center. Bonta, J., and Motiuk, L. L. (1990). Classification to Halfway Houses: a Quasi-Experimental Evaluation. Criminology, 28 (3), 497-506. Byrne, J., and Hummer, D. (2007). In Search of the "Tossed Salad Man" and Others Involved in Prison Violence. Aggression and Violent Behavior . Camp, S., and Gaes, G. (2005). Criminogenic Effects of the Prison Environment on Inmate Behavior; Some Experimental Evidence. Crime and Delinquency, 51, 425-442. Chen, K. M., and Shapiro, J. M. (2004). Does Prison Harden Inmates? A Discontinuity Approach. Cowles Foundation for Research in Economics. Yale University. Chen, M. K., and Shapiro, J. M. (2007). Do Harsher Prison Conditions Reduce Recidivism? Amerian Law and Economics Review, 9 (1), 1-29. Clark, J. L. (1998). Report to the Attorney General Inspection and Review of the Northeast Ohio Correctional Center (NEOCC). Correctional Association of New York. (2004). Mental Health in the House of Corrections. Cunningham, M. A. (2006). Nothing to Lose? A Comparative Examination of Prison Misconduct Rates Among Life-Without-Parole and Other Long-Term High-Security Inmates. Criminal Justice and Behavior, 33 (6), 683-705. Cunningham, M. D., and Sorenson, J. R. (2007). Predictive Facotrs for Violent Misconduct in Close Custody. Prison Journal, 87 (2), 241-253. Cunningham, M. R. (2008). Assertions of "Future Dangerousness" at Federal Capital Sentencing: Rates and Correlates of Subsequent Prison Misconduct and Violence. Law and Human Behavior, 32, 46-63. Farr, K. A. (2000). Classification for Female Inmates: Moving Forward. Crime and Delinquency, 46 (1), 3-17. Gaes, G. and Camp, S. (2009). Unintended conseqences: experimental evidence for the criminogenic effect of prison security level placement on post-release recidivism. Journal of Experimental Criminology, 139-162. California Department of Corrections and Rehabilitation Office of Research/Research and Evaluation Branch Page 150 December 19, 2011 Hardyman, P. L., Austin, J. and Tulloch, O.C. (2002). Revalidating External Prison Classification Systems: the Experience of Ten States and Model for Classification Reform. George Washington University, Institute on Crime, Justice and Corrections. Kupers, T. D. (2009). Beyond Supermax Administrative Segregation; Mississippi's Experience Rethinking Prison Classification and Creating Alternative Mental Health Programs. Criminal Justice and Behavior OnlineFirst, 1-14. Owens, C. E., Will, J., and Camp, H. (1995). Inmate Risk and Needs: Developing an Objective Means for Classifying Florida's Inmate Population. University of North Florida, Northeast Florida Center for Community Initiatives. Sorenson, J. and. (2010). Conviction Offense and Prison Violence: a Comparative Study of Murderers and Other Offenders. Crime and Delinquency, 56 (1), 103-125. Toch, H. (2007). Violence in Prisons, Revisited. Journal of Offender Rehabilitation , 45 (3/4), 128. Van Voorhis, P., Peiler, J., Presser, L. Spiropoulis, G. and Sutherland, J. (2001). Classification of Women Offenders: A National Assessment of Current Practices and the Experience of Three States. Center for Criminal Justice Research. University of Cincinnati. Vera Institute of Justice ( 2010). The Continuing Fiscal Crisis in Corrections: Setting a New Course. Waldo, G. P. (1970). The "Criminality Level" of Incarcerated Murderers and Non-Murderers . Journal of Criminal Law, Criminology and Police Science, 61 (1). California Department of Corrections and Rehabilitation Office of Research/Research and Evaluation Branch Page 151 December 19, 2011