Effect of Private Sector Work Opportunities in Prison on Labor Market Outcomes of the Formerly Incarcerated, CESR-Schaeffer, 2015
Download original document:
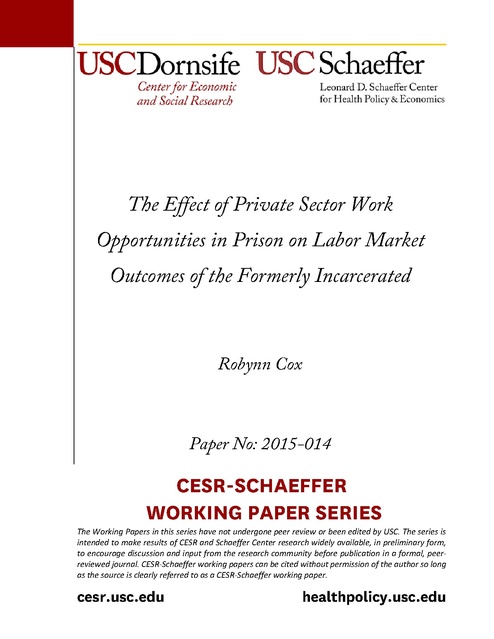
Document text
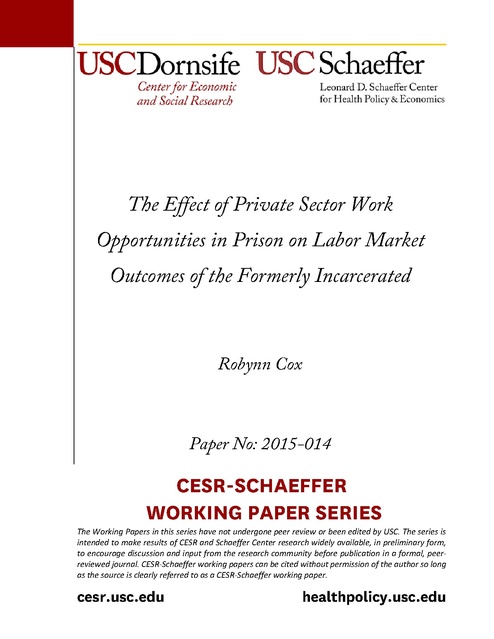
Document text
This text is machine-read, and may contain errors. Check the original document to verify accuracy.
The Effect of Private Sector Work Opportunities in Prison on Labor Market Outcomes of the Formerly Incarcerated Robynn Cox Paper No: 2015-014 CESR-SCHAEFFER WORKING PAPER SERIES The Working Papers in this series have not undergone peer review or been edited by USC. The series is intended to make results of CESR and Schaeffer Center research widely available, in preliminary form, to encourage discussion and input from the research community before publication in a formal, peerreviewed journal. CESR-Schaeffer working papers can be cited without permission of the author so long as the source is clearly referred to as a CESR-Schaeffer working paper. cesr.usc.edu healthpolicy.usc.edu ! ! ! ! The Effect of Private Sector Work Opportunities in Prison on Labor Market Outcomes of the Formerly Incarcerated Robynn Cox Spelman College USC RCMAR Scholar rcox@spelman.edu June 2015 JEL Classification: J30,J31, J64, J68, K42, K49 Keywords: Prison Labor, Incarceration, Inmate Reentry, Unemployment Duration, Employment Duration, Formerly Incarcerated, Earnings, Prison Industry Enhancement Certification Program, Job Training Programs ! ! ! ! Abstract This paper examines the effects of a private sector prison work program called the Prison Industry Enhancement Certification Program (PIECP) on unemployment duration, length of formal employment, and earnings of men and women released from various state prisons between 1996 and 2001. The labor market dynamics of formerly incarcerated men and women are also investigated. The program is found to increase reported earnings and formal employment on the extensive margin, with a stronger impact on the formal employment of women. There is little evidence that it increases formal employment along the intensive margin (i.e., duration of formal employment). Contrary to segmented labor market theories, superior employment (i.e., higher paying jobs) do not lead to increased job stability. Roughly 92% of those that obtain formal employment in the sample experience job loss; however, reincarceration rates are too low to explain this fact. An evaluation of labor market dynamics reveals that traditional human capital variables, criminogenic factors, and a few demographic characteristics determine job loss. In addition, black women, single women, and women with more extensive criminal histories face greater barriers on the labor market than their male counterparts. ! I. Introduction It is estimated that over 700,000 inmates are being released each year (West et al. 2010). Many of those released are returning to communities ill equipped to aid in the reentry process due to extreme levels of unemployment and poverty (Roman and Travis 2006). For example, roughly 12% of state inmates that were expected to be released in 1999 were homeless at the time of their arrest (Hughes and Wilson 2003). This begs the question: how will society absorb these mostly low-skilled laborers that face substantial barriers to reentry once they are released from prison? Numerous theories purport that post-release labor market opportunities could play a key role in assimilating the formerly incarcerated back into society, and in encouraging desistance from deviant behaviors (Cain 1976; Myers 1983; Western et al. 2001; Bushway and Reuter 2002; Piehl 2003; Holzer et al. 2004; Pettit and Lyons 2007). Empirical evidence suggests that criminals are responsive to employment and earnings opportunities, and that increases in both lead to a reduction in crime (Myers 1983; Gould et al. 2002; Raphael and Winter-Ebmer 2001; Holzer 2007).1 In fact, Uggen(2000) finds that work can act as a turning point in the life of an older (defined as an age greater than or equal to 26) offender. While older studies suggest that incarceration has little effect on employment, but significantly decreases earnings (see Cox 2010 for a review of the literature). Although there is some evidence that increases in criminal human capital can occur when the incarcerated are confined with individuals that have committed similar crimes (Bayer et al. 2009), other research finds that incarceration may increase employment and earnings (Lalonde and Cho 2008; Jung 2011). Specifically, Jung (2011) finds that prison sentence length is !!!!!!!!!!!!!!!!!!!!!!!!!!!!!!!!!!!!!!!!!!!!!!!!!!!!!!!!!!!!! 1 Machin and Meghir (2004) also find the same result in England and Whales. While the use of prison labor has been opposed by labor and human rights activists, this research focuses on the rehabilitative aspect of prison work programs and seeks to understand whether receiving vocational skills training 2 ! ! 1! positively associated with earnings and employment post release, and the effect is stronger for individuals who are convicted of economic crimes. It is conceivable that this effect is due to the variety of human capital investment programs (e.g., health care, education, skills training, employment, etc.) made available to rehabilitate the inmate. Mocan et al. (2005) are among the first to propose a dynamic human capital framework relating legal human capital appreciation (depreciation) during incarceration to decreases (increases) in post-release criminal activity. Simulations of their model find that skills training programs could halt the depreciation of legal human capital, and increase the expected returns to human capital investment post release, leading to greater participation in the legal labor market. Given these findings, the impact of inmate rehabilitation and training programs on post-release labor market success should be investigated further. Previous research finds that inmates who participate in education, vocational, and work programs are employed more than nonparticipants (Wilson et al. 2000). Tyler and Kling (2007) study the effect of obtaining a GED while incarcerated on the earnings of inmates once released. They find that there is a premium in the mainstream labor market for obtaining a GED for nonwhites but not for whites. Non-whites who attain a GED have higher earnings than non-white dropouts (roughly a 20 percent increase in earnings); however, these benefits dissipate over time. Moreover, there doesn’t appear to be an extra benefit from obtaining the credential over simply participating in the program. Prison work programs are another method of rehabilitation, albeit not without controversy2, used by departments of corrections in many states and is the current topic of !!!!!!!!!!!!!!!!!!!!!!!!!!!!!!!!!!!!!!!!!!!!!!!!!!!!!!!!!!!!! 2 While the use of prison labor has been opposed by labor and human rights activists, this research focuses on the rehabilitative aspect of prison work programs and seeks to understand whether receiving vocational skills training and work experience while incarcerated improves post-release labor market outcomes. For a detailed historical discussion of prison labor and the Prison Industry Enhancement Certification Program please see Cox (2009). ! ! 2! interest. Nonetheless, few studies have rigorously analyzed the influence of work programs on employment outcomes. Of the more recent studies that have been conducted, there are two that are relevant to this paper. Saylor and Gaes (1997) investigate the impact of inmate work programs “…and skills training on institutional adjustment, licit wages after release, and post release recidivism” of federal inmates (p.6). They find that individuals that participated in work or vocational/apprenticeship programs while incarcerated are 35% less likely to recidivate and 14% more likely to be employed within the first twelve months of release. However, federal inmates only make up roughly 12% of offenders incarcerated and their offense characteristics are different from state prisoners. For example, in 2005 most federal inmates were drug offenders (54%) while most state inmates were violent offenders (53%).3 Therefore, these results may not be generalizable to the majority of the imprisoned population. This is especially true if the labor market penalty varies by the type of crime committed (Waldfogel 1994; Grubb 2001) The second study is an unpublished report written by Smith et al. (2006) analyzing the effect of the Prison Industry Enhancement Certification Program (PIECP) on recidivism and labor market outcomes using the superset of the data utilized in this study. These data comprise the first nationally representative data set for PIECP, which is a unique program that offers offenders the opportunity to work for the private sector during confinement. The program allows confined individuals to gain work experience and skills (while still in custody) that could benefit them upon release from prison. In particular, PIECP allows the private sector to employ inmate labor behind state prison walls in a “free-world” work environment. Using administrative data collected on individuals released from prison between 1996 and 2001, they find that PIECP significantly decreases time from release to formal employment, significantly increases the duration of formal employment, and significantly increases reported earnings. By using !!!!!!!!!!!!!!!!!!!!!!!!!!!!!!!!!!!!!!!!!!!!!!!!!!!!!!!!!!!!! 3 Retrieved from BJS Federal Justice Statistics Program, http://www.bjs.gov/fjsrc/, and West and Sabol (2008). ! ! 3! observable characteristics to match PIECP participants to inmates that are similar but who do not participate in the program, their study attempts to overcome the missing data problem (i.e., lack of a counterfactual) inherent in program evaluation studies that are not carried out as random experiments. However, due to the limited availability of control variables for the larger study, it is unclear whether this matching strategy solves the sample selection problem. This is the first study to use the subset of the Smith et al. (2006) data for which additional control variables are available. There are a number of ways in which this research differentiates itself from its predecessor. First, given the small sample size this study compares the treatment of PIECP to one control group comprised of individuals that do not participate in PIECP (referred to as non-PIECP). Smith et al. (2006) compare PIECP participants to two control groups in their study: 1) individuals that participate in a state prison industrial work program (called traditional industries, or TI), and 2) those that do not take part in either of these official prison work programs (called other than work, or OTW). Evaluating these three classifications of prison “work” (PIECP, TI, and OTW) is complicated because it is possible for job tasks to overlap between PIECP and TI, and between TI and OTW. Nonetheless, there are various factors that make PIECP distinct from TI and OTW. In particular, PIECP participants have a substantially greater likelihood of interacting with private sector employers than both TI and OTW. It is possible that the skills developed in this type of environment could more readily translate to employment on the outside. Moreover, PIECP participants are required by law to earn at least the minimum wage for their labor, which could create a substantial intra-prison earnings differential between PIECP participants and those that do not participate in the program. Given all of these reasons, as well as the analytical findings that greater earnings during incarceration could encourage greater involvement in the legal labor market post-release (see ! ! 4! Mocan et al. 2005), this research will focus on estimating the benefits of PIECP relative to a combined TI and OTW control group. Second, since the subset of data (referred to as the PIECP sample) have additional personal characteristics that are not available in the full data set (e.g., mental and physical health status), the balancing properties of the matching estimator can be thoroughly investigated. A comparison of the normalized differences4 for these variables suggests that the covariates are balanced across the PIECP cohort and the “imputed” counterfactuals. Nevertheless, even though the sample is balanced, it might still be important to control for additional covariates within the analysis, which is not done by Smith et al. (2006), in order to improve efficiency and to further minimize bias of the point estimates (Angrist and Pischke 2009). Likewise, due to the availability of additional control variables, a broader range of demographic characteristics of the PIECP sample can be compared to national estimates in order to understand the extent to which external validity is traded for greater interval validity. While matching techniques may have improved the internal validity of the analysis, they might have done so at the expense of generalizability to the broader state prison population. Therefore, it is important to understand to what extent the PIECP sample differs from the broader population confined in state correctional facilities when making conclusions regarding the program. Finally, the primary contribution of this study is to estimate the differential effects of skill development on labor market outcomes and labor market dynamics by gender. Although the size of the female prison population has been growing at a faster rate than their male counterparts, there is a paucity of research on the labor market experiences of formerly incarcerated women. !!!!!!!!!!!!!!!!!!!!!!!!!!!!!!!!!!!!!!!!!!!!!!!!!!!!!!!!!!!!! 4 Normalized Differences for each variable are calculated by dividing the difference in the mean values of each group by the average standard deviation of the groups.! ! ! 5! Moreover, women are typically incarcerated due to crimes that are economic in nature, which suggests that women may especially benefit from skill development programs (see Cox, 2012). It is believed that participation in a real-world work environment will enhance both soft and hard skills of the offender, improve social capital, and provide opportunities to gain work experience during their incarceration, which should lead to superior labor market outcomes when compared to individuals that do not work during confinement, , ceteris paribus (Piehl 2003; Petit and Lyons 2007; Piehl 2009). Therefore, it is hypothesized that PIECP participants will gain employment faster, maintain employment longer, and earn higher wages than those that do not participate in the program. Similar to Smith et al. (2006), the results suggest that PIECP improves the hazard of formal employment. In addition, it is discovered that this effect is greater for women. Like Smith and her coauthors, it is also determined that PIECP increases the earnings of its participants. Contrary to the findings of Smith et al. (2006), there is no detectable difference between the job loss hazard of PIECP workers and the non-PIECP group once missing data and additional factors are controlled for in the analysis. In terms of labor market dynamics, it is discovered that labor market outcomes are significantly determined by traditional human capital variables, criminogenic variables, and demographic variables, all of which are associated with both cognitive and non-cognitive skill development. Finally, black women, single women, and women with a history of substance abuse seem to face additional barriers on the labor market. Overall, the results imply that PIECP is better at improving the cognitive ability (hard skills) and social networks of its participants (as measured by a greater hazard of formal employment, and increased earnings), but is less effective at enhancing non-cognitive (soft) skills (as measured by the duration of formal employment). In fact, factors that are highly correlated with non-cognitive ability are also significant to the labor market outcomes studied in ! ! 6! this analysis. Given that prior research finds that non-cognitive ability might be more important in altering criminal behavior and improving labor market outcomes (especially employment) than cognitive skills (see Heckman et al. 2006), one policy implication that could be drawn from these findings are that skill development programs should be coupled with programs that explicitly seek to develop the non-cognitive skills of its participants for successful reentry. II. The Prison Industry Enhancement Certification Program In general, there are two main work programs offered in state prisons: a job in a state ran industrial work program (referred to as traditional industries, or TI) and a job in the Prison Industry Enhancement Certification Program (PIECP). While both of these programs offer the opportunity for the worker to earn monetary compensation, and while job characteristics may overlap between TI and PIECP workers (e.g., working in an optical plant or print plant), only PIECP workers are guaranteed compensation since federal law mandates that they must earn the going market wage, but no lower than the minimum wage. If an individual does not participate in either of these work programs, then he/she could be required to help maintain prison grounds (e.g., laundry, janitorial labor, food services, etc.), attend educational or vocational training, or nothing at all. Persons falling under this category are classified as “other than work” (OTW). Depending on how states classify OTW and TI, some jobs (e.g., institutional maintenance jobs) may also overlap between these two groups (Smith et al. 2006).5 The Prison Industry Enhancement Certification Program (PIECP) merits additional discussion since it is the topic of this study and because it is a unique program that allows private industry to employ individuals incarcerated in certified state or local facilities.6 The Bureau of Justice Assistance states that PIECP !!!!!!!!!!!!!!!!!!!!!!!!!!!!!!!!!!!!!!!!!!!!!!!!!!!!!!!!!!!!! 5 6 Please see Appendix A for a more detailed description of OTW, TI, and PIECP jobs. Please see Cox (2009) for the legislative history and an in depth analysis of PIECP. ! ! 7! [e]xempts certified state and local department of corrections from normal restrictions on the sale of prisoner-made goods in interstate commerce. In addition the program lifts restrictions on these certified entities permitting them to sell prisoner-made goods to the Federal Government in amounts exceeding the $10,000 maximum normally imposed on such transactions (2004, p.1). In addition, PIECP encourages state and local governments to establish employment opportunities for prisoners that approximate private-sector work. In the 4th quarter ending in 2007, 38 states and 4 localities7 were certified8 in PIECP9 employing 5,401 inmates in 204 active cost accounting centers10. There are three models of employment in which the private sector can operate within PIECP: manpower, customer, and employer.11 Individuals working under the manpower model !!!!!!!!!!!!!!!!!!!!!!!!!!!!!!!!!!!!!!!!!!!!!!!!!!!!!!!!!!!!! 7 Please see Cox (2009) for a complete listing of certificate holders. According to the BJA, “[c]ertificate Holder refers to a department of corrections, or an alternate umbrella authority, which is approved by BJA for PIECP Project Certification. Certificate Holders assume monitoring and designation responsibilities with respect to their designated Cost Accounting Centers [(CAC)]. All PIECP prisoner-made goods are produced within [a CAC] that a certificate holder designates within itself, private prisons located in the same state or jurisdiction or, in the case of an umbrella authority within its membership agencies…Umbrella Authority refers to a type of Certificate Holder which is authorized by law to administer a PIECP Project and which consists of state and/or local departments of correction located within the same state. A certified umbrella authority may designate CACs within its membership agencies, as well as within members’ private prisons, and assumes responsibility for monitoring CAC compliance” (1999, p. 17007-17009) 9 Delaware, Missouri, and the Texas Red River County Department of Corrections no longer hold certificates. On May 13, 2004 the Washington State Supreme Court found inmates working in Class 1 free venture industries to be unconstitutional. However, the legislature proposed to the people an amendment to the constitution that would allow the state to employ such labor. This amendment passed in the November 2007 elections. Using a logistic regression in a public choice model, Gallagher and Edwards (1997) attempted to explain the likelihood that a state would participate in PIECP using data from 1985-1992. They find that “...states with stronger union membership, democratic governors, and high unemployment rates will be less likely to allow PIE projects” (p. 97). However, states with a rehabilitative view of prisons would be more likely to participate in PIECP. 10 According to the BJA, “[c]ost Accounting Center (CAC) refers to a distinct PIECP goods production unit of the industries system that is managed as a separate accounting entity under the authority of a Certificate Holder. All PIECP production activities are conducted within the context of a designated CAC which, generally is structured either as a customer or employer model for purposes of determining PIECP inmate benefits” (1999, 17007)) 11 Note that the type of model the private sector uses will determine the benefit structure to the inmate. According to the BJA PIECP Federal Guidelines (1999), “PIECP projects must provide inmate workers appropriate benefits comparable to those made available by the Federal or State Government to private sector employees, including workers’ compensation and, under certain circumstances, Social Security” (p.17011). Nonetheless, some states prohibit inmates from receiving workers compensation. However, “[p]rovision of comparable workers compensation benefits is acceptable as long as the CAC can demonstrate comparability of such benefits with those secured by the Federal or State Government for private sector employees” (p17011). Moreover, if the employer 8 ! ! 8! are employed by the department of corrections but are managed by the private company (Smith et al. 2006). With the customer model, the private company purchases all or part of the output from a CAC enterprise. However, “[a] customer model private sector partner assumes no major role in industry operations, does not direct production, and has no control over inmate labor” (BJA 1999, p. 17008). Finally, with the employer model “…the private sector owns and operates the CAC by controlling the hiring, firing, training, supervision, and payment of the inmate work force. The department of corrections assumes no major role in industry operations, does not direct production, and exercises minimum control over inmate labor performance” (BJA 2004, p. 17008,). The Prison Industry Enhancement Act requires prisoner participation in PIECP to be completely voluntary; and for prison “employees” to earn the prevailing local wage for similar labor, but no less than the minimum wage. In general, it has been found that PIECP wages are typically set at the Federal minimum wage (Auerbach 2001). A note added to the end of the statute requires businesses to meet the following criteria prior to employing prison labor in order to obtain the Federal exemptions on the marketability of prison made goods: 1) consult with local labor unions or similar labor organizations, and 2) ensure that paid inmate labor will not displace free labor, or be utilized in locations where there is already an abundant supply of labor in the free world to complete the tasks (Cox, 2009). As previously mentioned, jobs performed by individuals working in traditional state industries (TI) could overlap with those employed by the Prison Industry Enhancement Certification Program (PIECP) or those partaking in activities classified as “other than work” (OTW). Where job tasks do not differ, the key difference is in compensation. PIECP workers !!!!!!!!!!!!!!!!!!!!!!!!!!!!!!!!!!!!!!!!!!!!!!!!!!!!!!!!!!!!!!!!!!!!!!!!!!!!!!!!!!!!!!!!!!!!!!!!!!!!!!!!!!!!!!!!!!!!!!!!!!!!!!!!!!!!!!!!!!!!!!!!!!!!!!!!!!!!!!!!!!!!!!!!!!!!!!!!!!!!!!!!!!!!!!!!!!!! model is used, then social security benefits must be provided to the inmate. However, if the customer model is used then “…the BJA recognizes the applicability of other provisions of Federal law which may operate to preclude the provision of PIECP inmates with certain benefits, including Social Security” (p. 17011). ! ! 9! have to make at least the minimum wage while TI and OTW participants could earn anywhere from $0-$1.25 an hour. In addition to greater earnings, it is more likely that the work environment will resemble free world employment as you move from OTW, to TI, to PIECP. Only PIECP participants have the opportunity to network with private employers, this is especially true for prison employees that work under the manpower or employer models. Although information on the type of PIECP employment models is not available in the data, the effect should be captured through PIECP participation. Given the differences between PIECP participants and those that do not work in the program, the OTW and TI cohorts are combined for the remainder of the analysis and will be referred to as non-PIECP from this point on. III. Data The Smith et al. (2006) data are gathered from agency records across 5 states and include inmates incarcerated in 46 prisons, at different security levels, released between January of 1996 and June of 2001.12 The follow-up period ended in February of 2003 allowing those released from confinement to be followed from 2 to 7.5 years. The states were selected using a cluster sampling strategy in order to guarantee an adequate sample size. Using this method, states, certified prior to 1996, were ranked according to the number of Prison Industry Enhancement Certification Program (PIECP) participants. This method led to the selection of 5 states. From these states, all inmates who worked in PIECP at least one day that were released between January 1996 and June of 2001 were selected. Qualification for PIECP differs by state correctional facility and industry. Although there are similarities in criteria between most of the institutions and industries, it is not uniform (Smith et al. 2006). While participants must first volunteer for the program, Smith et al. (2006) state !!!!!!!!!!!!!!!!!!!!!!!!!!!!!!!!!!!!!!!!!!!!!!!!!!!!!!!!!!!!! 12 I would like to thank Cindy Smith for her guidance and willingness to share the data. ! ! 10! that departments of corrections generally expect their applicants to meet the following requirements: [1)] [d]isciplinary report free for 6 months[,] [2)] m]inimum and medium security levels[,] [3)] [e]nrolled in a high school or GED program or completion[,] [4)] [s]entence of at least 6 months remaining[, and] [5)] [n]o major medical problems prohibiting work (p. 23). They also state that common industry prerequisites are: [1)] [s]ubmit an application and be interviewed[,] [2)] [p]refer prior work experience, but some employers prefer to hire those who have never worked before[, and] [3)] “[f]it” with the current work force (pp. 23-24 ). Due to the voluntary nature of PIECP, and the selection criteria of employers and state department of corrections, there is a valid concern that estimating the effect of PIECP on labor market outcomes without taking into consideration this sample selection will bias the results. In fact, the issues associated with the evaluation of PIECP are similar to those confronted when analyzing job-training programs: lack of random assignment into PIECP could cause selection on observed or unobserved characteristics (Heckman and Hotz 1989). In order to address this concern, it is assumed that all of the selection mechanism is on observable characteristics, and PIECP workers are matched on observables to inmates that do not participate in the program. According to Smith et al. (2006), exact matches were made on race (white and minority), gender, and crime type (person and all other), while categorical matches were made on age at intake (5 criteria), timed served (7 criteria), and the number of disciplinary reports (10 criteria). Department of corrections data were then merged with data on reported earnings (Smith et al. 2006). Administrative data are beneficial to use because they are less likely to suffer from measurement error, attrition bias, and nonresponse (Card et al. 2010). If there is no observed wage at any point during the follow up period, then it is assumed that the individual is ! ! 11! unemployed. However, this may be an incorrect assumption, particularly for this population, since it is not uncommon for ex-offenders to take under the table jobs in which earnings are not reported. If this were the case, then variables measuring labor market outcomes would be incompletely observed. If PIECP workers were more likely to obtain formal employment with firms that report earnings data, then this would artificially inflate the gap between employment outcomes of PIECP and non-PIECP workers, causing the results to be biased. However, to the extent that formal employment represents legitimate and stable employment (i.e., superior employment), compared to informal employment, then it is this gap that should be of interest.13 This study analyzes a subset of the Smith et al. (2006) data containing 1,217 black and white offenders that have information on detailed control variables.14 Because the sample was constructed by matching PIECP participants to non-participants, the results should only be generalized to state inmates that participated in PIECP or that are similar to PIECP workers. It is !!!!!!!!!!!!!!!!!!!!!!!!!!!!!!!!!!!!!!!!!!!!!!!!!!!!!!!!!!!!! 13 Segmented labor market theory is often referred to as a theory of dual labor markets because it argues that there are two distinct labor markets: the primary labor market and the secondary labor market. The primary labor market consists of “…jobs in large firms and/or unionized jobs, which tend to be better jobs–higher paying, more promotion possibilities, better working conditions, and more stable work. The secondary labor market which roughly overlaps large sections of the external labor market, contains the low-paid jobs that are held by workers who are discriminated against and who have unstable working patterns” (Cain 1976, p. 1222). Traditionally, economists view tastes for work as exogenous variables that help to explain one’s labor market achievements. However, SLM theorists argue that taste are actually endogenous and can be determined by success in the labor market. In particular, discrimination and other systematic or random influences that cause individuals to enter the secondary labor market can trigger anti-work sentiments among low-income workers, thereby keeping them in a position of hardship (Cain 1976). Theoretically, employment can help to rehabilitate an offender, however, it would take a decent job (i.e., in the primary labor market) to draw individuals out of a life of crime. 14 There are originally 1,309 observations. However, 1 observation is dropped due to having a negative value for time served, and 91 observations that have a recorded race of minority other were removed from the sample. Moreover, there are two observations whose time from release to formal employment and time from formal employment to job loss indicated the ex-offender was employed during the follow up period. However, the censored employment variable was coded to the contrary. Since all of the other employment variables indicated employment was obtained, the censored variable was corrected to match the rest of the data. A descriptive analysis comparing this sample to the Smith et al. data determined that individuals in these data are about the same age at release and incur roughly the same number of disciplinary reports during incarceration as the superset to the data (i.e., the data used in Smith et al. 2006). However, the individuals in the smaller sample seem to be worse off than those in the superset to the data as measured by criminal history, marital status, pre-incarceration employment, and pre-incarceration earnings. In particular, the individuals in the data utilized in this study have on average greater prior arrests, more prior convictions, more previous incarcerations, have a larger proportion that have no formal employment prior to incarceration, have a greater proportion of individuals that earned wages less than $20,234 prior to confinement, and have a larger percentage of individuals that are single. ! ! 12! well known that there is a trade off between external (generalizability) and internal (causality) validity. This study uses causal inference techniques to improve internal validity, but in doing so it most likely gives up the ability to generalize to a broader group of offenders. Therefore, the estimated effects are average treatment effects on the treated. In addition, the data do not allow for determination of those individuals who are housed in a PIECP facility but are included in the non-PIECP control group so spillover effects cannot be isolated; however, it is possible that they are controlled for through facility fixed effects. Furthermore, there is no variable to control for the particular work task held by the individual during the spell of incarceration. Because of the intersection among duties performed by those that worked in traditional industries during confinement and the Prison Industry Enhancement Certification Program cohort, larger differences in outcomes between these two groups will be required to detect a statistically significant difference.15 Finally, the data do not have enough information to control for the effect of educational or vocational training that may have been required for participation in PIECP. The summary statistics for the total sample are presented in Table I. Roughly 34% of the sample participated in the Prison Industry Enhancement Certification Program (PIECP). Moreover, PIECP participants are slightly more educated, have marginally fewer disciplinary reports during imprisonment, serve a somewhat longer time in prison, have a slightly higher percentage of female participants, are more likely to have a history of substance abuse, have a marginally higher proportion that have committed a crime against a person, and have a somewhat lower percentage of participants that are single. Moreover, they have a lower !!!!!!!!!!!!!!!!!!!!!!!!!!!!!!!!!!!!!!!!!!!!!!!!!!!!!!!!!!!!! 15 The other than work (OTW) and traditional industries (TI) groups comprise the control group and (as previously mentioned) could also perform the same task depending on how the state classifies the work performed by TI and OTW. Moreover, OTW could earn wages comparable to TI. TI workers could be classified as those who perform work similar to PIECP (however, unlike PIECP, they may earn a nominal wage or nothing at all) and those who perform institutional maintenance. Finally, PIECP workers tasks can vary from habitual and labor-intensive to very skilled. ! ! 13! proportion of participants that were unemployed or out of the labor force prior to incarceration, have a higher share of individuals that worked in a skilled occupation prior to prison, have a lower percentage of individuals that have a medical problem, have a marginally lower proportion of individuals with a mental health problem, have a shorter duration of unemployment and a longer duration of formal employment upon release, have greater earnings pre-,during, and postincarceration, and have greater work experience (as measured by the number of quarters worked) pre-, during, and post- incarceration. While the descriptive statistics imply that the PIECP group may have greater levels of both hard and soft skills, the normalized differences between PIECP participants and the nonPIECP control group are small (i.e., a standardized difference of less than .5)16 for all of the covariates except for the number of quarters worked while incarcerated. In fact, if we exclude this variable, the maximum absolute value of the normalized differences is .31 and the maximum absolute value of the difference in the spread of the distribution between the two groups is .29. This suggests that the matching strategy succeeded in balancing the covariates across the different groups, such that any differences in the distributions of the variables between the PIECP and non-PIECP groups can be adjusted for by regression analysis (Imbens and Rubin 2012; Imbens, 2013).17 As previously mentioned, one drawback to using techniques that improve causal inference is that the results become less generalizable. Since inmates are matched based on some general criteria for participation in PIECP (see discussion above), it is informative to understand !!!!!!!!!!!!!!!!!!!!!!!!!!!!!!!!!!!!!!!!!!!!!!!!!!!!!!!!!!!!! 16 While there is no official cutoff to determine when normalized differences are too large, Imbens (2013) suggests that variables with a normalized difference greater than .5 should be considered too large to be adequately controlled for using regression analysis. 17 Assuming the unconfoundedness assumption holds. ! ! 14! how the characteristics of this PIECP sample compare to national statistics of the general imprisoned population. Naturally, because of the nature of this study, the concentration of PIECP workers is extremely high when compared to the overall state inmate population. For example, in 2000, 39.4% of state inmates worked in general work, 3.0% did farm work, 5.3% worked in traditional industries, and 0.3% worked in PIECP (Solomon et al. 2004). Figures 1-4 compare data from Langan and Levin (2002) and Freeman (2003) to the total PIECP sample and to the complete cases sample18. According to Langan and Levin (2002), of the offenders released from prison in 1994, 50.4% of inmates were white and 48.5% were black. In the total PIECP sample 59% are white and 41% are black. Moreover, while women are only about 10% of offenders released from state prison in 1999, they are roughly 28% of these data (see Figure 1). In addition, 36% of individuals released from state prison in 1999 were under age 30 (Freeman, 2003) compared to roughly 21% of the total PIECP sample (see Figure 4 for a more detailed comparison of the age distribution). About 67% of the PIECP sample has at least one child (the average number of children for an inmate is 1.6), while only 54% for state inmates incarcerated in 1999 are parents (Glaze and Maruschak 2008; Beck and Harrison 2001). There are a high percentage of inmates with a history of substance abuse in the PIECP data: 86% had a history of alcohol or drug use. Solomon et al. (2004) report 70% of state prison inmates having ever used drugs, 57% using drugs the month before arrest, 33% using drugs at the time of offense, and 37% using alcohol at the time of offense. Moreover, 30% of the PIECP sample has a mental health problem and 10% of the sample has a medical problem with a special need compared to 16% and 10%, respectively, for the national estimates (see Figure 1). While !!!!!!!!!!!!!!!!!!!!!!!!!!!!!!!!!!!!!!!!!!!!!!!!!!!!!!!!!!!!! 18 Weights were not available for the PIECP samples. Nonetheless, means are compared to National statistics in order to better understand how representative the PIECP sample is to national prisoner characteristics. ! ! 15! the PIECP sample appears to have a higher proportion of inmates released with a mental health condition, there is no difference in the prevalence of a physical medical problem between the total sample and the national estimates. In addition, those with a history of a mental health condition or physical medical problem comprise roughly 33% of the sample, which is slightly larger than the Solomon et al. (2004) estimate of 31%. The average education level for inmates in this sample is roughly 10.8 years. Moreover, only about 5% of the sample had less than eight years of education, while national estimates report this number to be 11% in 1999 (Freeman 2003). Thus, the PIECP sample of inmates seems to be more educated. Average weekly earnings prior to incarceration are $55.60, average weekly earnings during incarceration are $51.60, and average weekly earnings post-incarceration are $168.11.19 Ex-offenders worked an average of 2 quarters prior to incarceration, 1 quarter while in prison, and 6.3 quarters after release. On average, ex-offenders have a nontrivial increase in employment after incarceration. Prior to incarceration 12% of the sample worked in food, 4% worked in retail, 8% worked in an office; 33% worked as unskilled laborers, in assembly, warehouse, or trucking; 3% were self-employed; 29% worked as skilled laborers, in construction trades, or in welding; and 10% were unemployed, a student, or disabled (receiving SSI). In the PIECP sample, property offenders, personal offenders, and drug offenders comprised 25%, 45%, and 28% respectively. Of offenders released in 1994, 22.5% were violent offenders (e.g., murder, sexual assault, and robbery), 33.5% were property offenders, and 32.6% of those released had committed a drug offense. The PIECP data have a fairly high percentage of individuals who committed crimes against a person when compared to the 1994 national estimates (see Figure 2). The mean number of previous incarcerations for the PIECP sample is !!!!!!!!!!!!!!!!!!!!!!!!!!!!!!!!!!!!!!!!!!!!!!!!!!!!!!!!!!!!! 19 Due to confidentiality, earnings could not be adjusted for inflation. ! ! 16! roughly 2, with 50% of the sample having had a prior incarceration, which is greater than the 44% national estimate (Langan and Levin 2002). Moreover, the average time served in prison in this sample is roughly 52 months (or 1,560 days) compared to 20.3 months for prisoners released in 1994 (Langan and Levin 2002). Thirty-five percent of the sample had involvement in delinquent activity as a juvenile. While, 79% of the sample obtained employment after release, 92% lost or quit their jobs during the follow up period. Although the PIECP sample seems to be older, more educated, and more white than national estimates of state inmates, it also has greater rates of physical and/or mental health problems, substance abuse, offenders committing a crime against a person, and repeat offenders. Moreover, offenders in the PIECP sample serve longer periods of time behind bars than the average state inmate. Thus, it is not clear that choosing offenders with characteristics similar to PIECP necessarily selects a “better” sample of inmates than the general state prison population. IV. Empirical Design As described in the previous section, the data used for this analysis is right-censored duration data. As a result, survival analysis techniques are used to handle the bias caused by the censoring mechanism. Therefore, the evaluation of employment outcomes will be implemented using the Cox Proportional Hazard (PH) model. The Cox PH model is a popular method used to analyze duration data due to its semi-parametric approach. Unlike other survival models, the Cox proportional hazard model does not assume a functional form for the baseline hazard. This gets around the issue of inconsistent estimators that plague fully parametric estimators if the underlying model is misspecified. Moreover, even though the estimator is inefficient, the loss in ! ! 17! efficiency is small when compared to maximum likelihood estimators for fully parametric models (Cameron and Trivedi, 2005). This estimator also controls for censored and tied data.20 The proportional hazard rate for this model is of the form: (2) λ (t | x, β ) = λ0 (t )φ ( x, β ), If φ ( x, β ) = exp( x' β ) 21 is selected then: (3) λ (t | x, β ) = λ0 (t ) exp( x' β ), β is then estimated by minimizing the log partial-likelihood function: N & # (4) ln L p ( β ) = ∑ δ i $ln φ ( x i , β ) − ln( ∑φ ( xl , β )!, i =1 l∈R ( ti ) % " where δi is an indicator variable equal to 1 for uncensored observations and zero for censored subjects, xi are time-constant regressors that vary by individual, β is a vector of parameters, & R(tj) is the set of periods at risk at tj (Cameron and Trivedi, 2005). The dependent variable used to measure the duration of unemployment is time from release to formal employment measured in quarters. The choice to supply labor will depend on the type of skills developed while incarcerated (i.e., participation in the Prison Industry Enhancement Certification Program) and a vector of time constant regressors of personal characteristics believed to influence employment decisions (Pencavel, 1986). Personal characteristics include the type of crime committed, race, age at release, age at release squared, time served, time served squared, education, number of disciplinary reports, marital status, substance abuse history, quarters worked prior to incarceration, previous occupation, number of children, mental health status, gender, health status, number of previous incarcerations, history of juvenile delinquency, gender interaction terms with all of the aforementioned variables, facility !!!!!!!!!!!!!!!!!!!!!!!!!!!!!!!!!!!!!!!!!!!!!!!!!!!!!!!!!!!!! 20 21 Tied data occurs when multiple failures happen at the same point in time (Cameron and Trivedi, 2005). This assumes φ(x,β) > 0. ! ! 18! dummies, and release year dummies. Finally, the key independent variable of interest is a categorical regressor indicating participation in the Prison Industry Enhancement Certification Program (PIECP).22 The reference group (referred to as non-PIECP) is comprised of individuals that participated in other than work (OTW) or traditional state prison industrial work programs (TI) during their incarceration. These variables are also interacted with gender and race to determine if there is a differential effect of PIECP participation for women. Due to the greater skill development and the enhanced social capital (e.g., networking with potential employers) of program participants, it is believed that PIECP workers will have a shorter duration of unemployment when compared to the non-PIECP control group. Moreover, this effect will be stronger for women. As previously mentioned, work tasks for TI participants can overlap with both PIECP and OTW; nonetheless, TI workers are included in the reference group because of efficiency concerns (due to the small sample size), and because the focus of this research is to understand how private sector work opportunities affect the reentry of the formerly incarcerated. As a result, the effect of PIECP on formal employment and earnings outcomes might be attenuated due to the possibility of similar prison work assignments between the TI and PIECP groups. The dependent variable used to measure the ex-offender’s duration of formal employment will be time from formal employment to job loss. It is important to note that much of the labor market volatility experienced by the formerly incarcerated is self-imposed since the majority of employment terminations are intentional (Schmidt and Witte 1984; Bushway et al. 2007). Therefore, this variable could be thought of as a measure of job satisfaction or job !!!!!!!!!!!!!!!!!!!!!!!!!!!!!!!!!!!!!!!!!!!!!!!!!!!!!!!!!!!!! 22 Incarceration wages and wages upon release are indirectly controlled for through PIECP. These variables are not included in the analysis in order to avoid over-controlling for the effects of PIECP. In other words, if PIECP is beneficial because it increases post-incarceration wages, then including this variable in the analysis will eliminate the effect of PIECP. ! ! 19! stability.23 In theories of segmented labor markets (or dual economies), it is believed that inferior wages and mediocre jobs bring about work instability (Cain 1976; Schmidt and Witte 1984; Pettit and Lyons 2007). These low wage, low quality, unstable positions are a result of “…the workers’ habits and attitudes (“tastes for work”) that are inimical to steady employment, to the firm’s output goals, and to upgrading oneself” (Cain 1976). To the extent that this is true, time from formal employment to job loss can be thought of as a measure of ex-offenders’ attitudes towards work. The control variables used in this analysis will be the same as those in the unemployment duration analysis discussed above. If PIECP helps workers to match with better paying jobs upon release, and if work experience during confinement helps to improve the hard and soft skills that can lead to a greater attachment to the legal labor market, then PIECP participation should lead to longer durations of formal employment when compared to the nonPIECP cohort. As in the case of unemployment duration, the effect of PIECP may be weakened due to the possible overlap in the tasks of TI and PIECP cohorts. In order to investigate the impact of the Prison Industry Enhancement Certification Program (PIECP) on earnings, program participants are compared to individuals in the nonPIECP group using Ordinary Least Squares (OLS). Unfortunately, there is no valid exclusion restriction within the data to explicitly control for selection bias stemming from the decision to work. As a result, the analysis is restricted to offenders that obtain employment after incarceration. Thus, the results cannot be generalized to individuals that do not obtain employment after incarceration. The relationship between earnings and PIECP is modeled using the following Mincerian wage equation: (6) ! = ! ! ! + !! !"#$! + !, !!!!!!!!!!!!!!!!!!!!!!!!!!!!!!!!!!!!!!!!!!!!!!!!!!!!!!!!!!!!! 23 However, since the data are measured in quarters, and because we can’t observe how many times an individual changes jobs in a quarter, this is an imperfect measure of job stability. ! ! 20! where w is the log of the post release weekly earnings, x is a vector of covariates included in the wage equation, PIECP is equal to one if the individual worked in the Prison Industry Enhancement Certification Program while incarcerated and zero otherwise, and u is the error term. The vector of covariates include education, age at release, age at release squared, number of disciplinary reports, number of years served in prison, number of years served in prison squared, race, number of previous incarcerations, prior substance abuse, an indicator variable for having committed a property offense for the original offense, an indicator variable for having committed a drug offense for the original offense, an indicator variable for having committed an offense in the other category (all offenses not classified as property, drug, or an offense against a person), having a history of juvenile delinquency, having a history of a mental health issue, an indicator variable equal to one if the individual is single (and zero otherwise), number of quarters worked pre-incarceration, number of quarters worked pre-incarceration squared, number of children, having a medical special need, gender interaction terms with all of the above covariates, and release year dummies, and facility fixed effects. The percentage of observations missing data across covariates varies from a high of 13% to a low of .1%. Therefore, as an additional robustness check, the missing data are imputed and the above analyses are re-estimated in order to gauge the extent to which the missing data might bias the results. Table III compares estimates for the descriptive statistics of the total sample and complete cases sample. By comparing the two samples, it is evident that the means for some variables change when employing list-wise deletion. While the normalized differences suggest that the discrepancy in the distributions of the variables between the total sample and complete cases sample are small, failing to control for missing data may cause slope parameter estimates to be biased. ! ! 21! Therefore, multiple imputation by chained equations is used to estimate values for observations that have covariates with omitted values. This method imputes the missing data for each independent variable by using the remaining covariates. For example, suppose that Z = (Z1,Z2,…,Zk) is a set of k random variables, each one having some omitted values. Assuming the data are missing at random, and that the unconditional probability distribution of Z, P(Z), is multivariate normal for a continuous variable (and a logistic distribution for a binary variable), then linear regression models such as Z1 = Z2tβ12 + Z3tβ13 + …+ Zktβ1k + ε1, with ε1 ~ N(0,σ12 ) can be used to obtain a random draw of values (where t represents the number of iterations) (van Buuren and Oudshoorn 1999; Wallace and Cox 2012).24 Since it is assumed that the multivariate distribution exists, and that one can obtain values from it by iteratively selecting from the conditional distribution, one can conduct multivariate imputation by splitting it into a chain of univariate equations. This switching regression approach is employed to generate a group of Y imputed data sets where estimation is performed on each one and the ultimate outcomes are derived by taking averages of all of the imputed data. One of the main benefits of the multiple imputation technique is that the estimation error is included in the estimated coefficients’ standard errors (Rubin 1987; van Buuren and Oudshoorn 1999; Schaefer 1999; Donders et al. 2006; Little and Rubin 2002; Wallace and Cox 2012). !!!!!!!!!!!!!!!!!!!!!!!!!!!!!!!!!!!!!!!!!!!!!!!!!!!!!!!!!!!!! 24 Z includes the number of quarters from release to formal employment, a binary variable indicating whether the individual obtained employment, the number of quarters from formal employment to job loss, a binary variable indicating whether the individual lost their job, weakly earnings post release, a binary variable for OTW participants, a binary variable for TI workers, education, age at release, number of disciplinary reports while incarcerated, number of years served in prison, whether the ex-offender is black, whether the ex-offender is a women, the number of previous incarceration, a binary variable indicating if the ex-offender had a history of substance abuse, binary variables for the offense in which the formerly incarcerated individual was serving time, a binary variable indicating whether there was a history of juvenile delinquency, a binary variable indicating whether the individual has a mental health issue, a binary variable indicating whether the individual is single, number of quarters worked prior to incarceration, number of kids, and a binary variable indicating that the ex-offender has a medical special need. Note that none of the dependent variables are missing values. Moreover, the variables representing occupation prior to incarceration were not imputed so the maximum number of observations for the imputed analysis is 1161. ! ! 22! While the research design only allows the results to be interpreted based on the 5 states and the participants in the sample, it is believed that the findings of this research are still useful in understanding the benefits of work experience and training programs offered to offenders while incarcerated in state facilities. There is a focus on private sector work opportunities because it is often purported that successful reentry should entail incarcerated individuals forming networks with employers prior to exiting prison. While this data cannot explicitly control for which PIECP participants had contact with employers, it is certain that the likelihood of this contact is substantially higher for PIECP workers. Moreover, obtaining private sector employment during incarceration might improve both hard and soft skills, and act as a signal (provided this experience can be shared on the resume) to employers on the outside about the quality of the individual, which should lead to better employment opportunities. The following section presents the results of the empirical analysis, and is followed by a detailed discussion of the findings and concluding remarks. V. Results PIECP Participation and Formal Employment Outcomes If we are interested in understanding whether participation in PIECP alleviates the targeted criminogenic needs of the criminal (e.g., increased job skills and an increased likelihood of employment upon release), then it is the impact of PIECP on formal employment and wages that should be measured (Maltz, [1984]2001). Moreover, concentrating on these more concrete aspects of rehabilitation may have fewer complications with measurement than if we were to investigate the effect of PIECP on recidivism (Maltz, [1984] 2001, p. 9). Thus, the effect of the Prison Industry Enhancement Certification Program (PIECP) on the criminogenic needs of the formerly incarcerated will be evaluated by looking at how the program influences the duration of unemployment, the length of formal employment; and weekly earnings upon release from ! ! 23! confinement. By analyzing the unemployment duration, we can understand the impact of PIECP on not only the ability of the ex-offender to obtain employment upon release, but also on how long it takes for the formerly incarcerated to become employed. It is hypothesized that PIECP will decrease unemployment duration, and this effect will be stronger for women, ceteris paribus. Put differently, it is assumed that formerly incarcerated women will suffer a greater penalty for their criminal history on the labor market leading to longer durations of unemployment, but PIECP will help to narrow the employment gap, holding other factors constant. We first analyze how PIECP affects the duration of unemployment using Kaplan-Meier survival estimates. Kaplan-Meier survival estimates are equivalent to the Cox proportional hazard model with no covariates. The Kaplan-Meier survival curve labeled “Survival Model 1” in Figure 5.A. shows that PIECP participants perform better than the non-PIECP group in obtaining employment upon release from prison. Log-rank tests for equality of the survival curves suggest that the difference between non-PIECP and PIECP is statistically significant at the 10% level. Table III presents OLS and Cox regressions with (Equations 2 and 4, respectively) and without (Equations 1 and 3, respectively) covariates for the pooled sample. The results across all estimation techniques and specifications are fairly consistent: Prison Industry Enhancement Certification Program (PIECP) participants obtain employment faster than the non-PIECP group. OLS results suggest that PIECP has statistically significant shorter durations of unemployment, even after controlling for a host of covariates. The model finds that PIECP laborers obtain employment roughly 6 months prior to the non-PIECP group, ceteris paribus. Nonetheless, since the data are right censored, the analysis is re-estimated using survival data techniques. After adjusting for covariates, the survival results suggest that PIECP participants with a non-zero time ! ! 24! from release to employment have an employment hazard that is roughly 11% higher than nonPIECP workers; however, this difference is insignificant. Note that observations that find employment immediately upon release from prison are automatically dropped from the analysis using the Cox estimator (since they have a time from release to employment of zero). Therefore, the above findings do not capture those who obtain employment instantaneously, which may have attenuated the effect of PIECP. This is especially true if the primary impact of PIECP works by providing access to social networks that might more quickly lead to employment opportunities after discharge from prison. In order to recapture these observations within the analysis, the Cox model is re-estimated by assigning observations that have a time from release to employment of zero (i.e., obtain a job as soon as dismissed from prison) with the smallest recognizable number that is close to zero but not equal to zero. This can be done for the Cox estimator because it is the ranking of the numbers that is most important when estimating the coefficients. The survival curve labeled “Survival Model 2” in Figure 5.A. shows a larger difference between PIECP and the non-PIECP group once those instantly securing employment are included in the analysis, and this difference is highly significant (.1% significance level). The regressions in the columns labeled “Equation 5” and “Equation 6” in Table III report the regression results, including and excluding covariates, for this procedure. The sign of the estimated coefficients are consistent and statistically significant across both specifications: individuals that do not participate in PIECP suffer significantly longer spells of joblessness upon release from incarceration. The model suggests that PIECP workers have a hazard of formal employment that is roughly 50% above the non-PIECP hazard, holding other factors constant. Note that when comparing equations 5 and 6 to equations 3 and 4 that the gain in statistical significance is not only due to greater precision from the larger sample size, but also results ! ! 25! from a substantial increase in the magnitude of the estimated coefficient. This suggests that one of the major benefits of participating in PIECP may work by connecting offenders to employers that can higher them as soon as they are released from prison. Figure 5.B. reports Kaplan-Meier survival curves by gender. Limiting the analysis to individuals that are not employed immediately upon release, and without controlling for other covariates, the “Survival Model 1” graph shows that there is relatively little difference between male and female non-PIECP workers, but these groups have a higher unemployment survival rate than both male and female PIECP participants consistent with the pooled results. Even though female PIECP participants have the lowest survival rate out of all groups analyzed, log rank test for equality show no statistically significant difference between any of the survival curves displayed on the graph. Table IV investigates whether the effect of PIECP differs by sex with and without covariates. The signs of the regressions are fairly consistent across estimators and specifications, and the findings suggest that male and female PIECP workers have a shorter duration of unemployment than the male and female non-PIECP groups. Nonetheless, the differences between these cohorts are only statistically significant for some of the empirical specifications. Focusing only on individuals that do not secure employment immediately upon release from prison, there is no statistically significant difference in the unemployment duration between male and female PIECP laborers, nor is there a statistically significant difference between the male non-PIECP group and men and women that worked in PIECP during confinement. However, as hypothesized women PIECP participants fair better than non-PIECP women and these differences are statistically significant at the 10% significance level. In particular, female PIECP participants have a baseline hazard that is roughly 58% above women in the non-PIECP group, ceteris paribus. ! ! 26! When we include individuals that experience employment immediately upon release (using the same procedure as explained above), “Survival Model 2” in Figure 5.B. shows a more pronounced difference between the survival curves of PIECP participants and the non-PIECP control groups. In particular, there is no distinguishable difference between men and women for the non-PIECP group, but both male and female PIECP workers have a significantly lower (.1% significance level) survival rate than male and female non-PIECP workers. Moreover, women PIECP workers have a survival curve that is significantly below (5% significance level) their male PIECP counterparts. Turning to the regressions in Table IV, the column labeled “Equation 6” shows that male PIECP participants have a significantly greater hazard of formal employment than the male nonPIECP group. Moreover, female non-PIECP laborers fair worse (i.e., have a lower formal employment hazard) than female PIECP workers. In particular, the male PIECP cohort has a hazard that is 26% above the male non-PIECP baseline hazard, ceteris paribus, and this difference is significant at the 5% significance level. Likewise, female PIECP workers have a hazard that is roughly 102% above the female non-PIECP baseline hazard, holding all else constant,, and this difference is significant at the .1% significance level. While participation in the Prison Industry Enhancement Certification Program seems to lower the duration of unemployment for both men and women, these findings imply that the effect is stronger for women than men. Moreover, a huge portion of the benefits of PIECP appears to be attributable to greater opportunities for employment immediately upon release from prison.25 !!!!!!!!!!!!!!!!!!!!!!!!!!!!!!!!!!!!!!!!!!!!!!!!!!!!!!!!!!!!! 25 It should be noted that we cannot control for the type of release (supervised release, work release, etc.). Thus, an alternative explanation is that PIECP workers are more likely to participate in work release, which would suggest that work release is a mediator variable through which part of the effect of PIECP is working. Controlling for work release, therefore, might attenuate the effect of PIECP on the duration of unemployment. ! ! 27! As previously discussed, implementing list-wise deletion may bias the point estimates. Since ignoring observations with missing values could significantly alter the results, missing data are imputed using multiple imputation and the regressions (including all of the control variables) from Tables III and IV are re-estimated. The imputed results are included in these tables within the columns designated as imputed. In general, there seems to be an efficiency gain (even after taking into consideration the uncertainty from the imputation model) from including the imputed observations (as can be seen by the smaller standard errors); however, there is also evidence that using only complete cases in the analyses may marginally bias parameter estimates since the magnitudes of the variables also change. In particular, the pooled difference in the hazard for formal employment between PIECP and non-PIECP is estimated to be about 45% (instead of the 50% estimated for the complete case analysis), while the difference between the female PIECP and the female non-PIECP employment hazard is estimated to be 96% (versus 102% for the complete case estimation) for the imputed results with no change in the significance levels. There is virtually no discrepancy in the estimated difference in employment hazards for male PIECP and male non-PIECP participants when comparing the imputed results to the complete cases estimates, however, the imputed estimates are more significant (1% significance level) than the non-imputed results. All of the results are highly statistical significant leading to the conclusion that the major advantage of PIECP operates through enhancing social networks that help participants to obtain a job immediately upon release from prison, and this effect is stronger for women. We next examine the effect of the Prison Industry Enhancement Certification Program (PIECP) on the duration of formal employment. If job termination is the decision of the employer, then evaluating time from formal employment to job loss may help to gauge the softskills of the ex-offender, e.g., the ability to consistently show up to work on time. However, if ! ! 28! job termination is the choice of the formerly incarcerated, as is believed to be the case in the literature (see Schmidt & Witte, 1984), then investigating PIECP’s effect on the time from employment to job loss will provide a measure of job satisfaction and stability of the exoffender. Thus, inspecting how PIECP impacts the time from formal employment to job loss could improve our understanding of how the PIECP program influences the ex-offender’s ability to secure and maintain satisfactory employment.26 This is important because in theories of dual, or segmented, labor markets unsatisfactory job placements lead to employment in the secondary, or low-paying, labor pool. Following the logic of segmented labor market theory, helping offenders to achieve better, more pleasing jobs will lead to a decrease in criminal activity. In fact, the returns to work and crime may significantly affect participation in crime (Myers,1983, Gould et al., 2002). Moreover, the joint risk of participating in crime and work could have an important impact on crime rates. Specifically, if jobs in the secondary labor market provide easy access to criminal opportunities (e.g., burglary and larceny), then moving individuals from these low skilled, lowwage jobs to primary labor market employment may help to lower crime (Myers, 1983). It is hypothesized that PIECP will increase formal employment duration, ceteris paribus. To the extent that the program improves employment prospects for women, it is also expected that the effect will be stronger for these women, holding other factors constant. Pooled Kaplan-Meier survival estimates presented in Figure 6.A. show no difference between PIECP and the non-PIECP group. Unsurprisingly, log-rank test of equality fail to reject the null that the survival curves are equal. Table V reports the OLS and Cox models without !!!!!!!!!!!!!!!!!!!!!!!!!!!!!!!!!!!!!!!!!!!!!!!!!!!!!!!!!!!!! 26 There is no way to determine how many jobs an individual holds within each quarter with these data. Thus, time from formal employment to job loss is an imperfect measure of job satisfaction because it only allows one to determine if the individual maintained employment, and not the number of changes in employment during the observation period. ! ! 29! accounting for differential effects of PIECP by gender. The pooled OLS estimates suggest that PIECP participants maintain employment roughly 2 months longer, ceteris paribus, than the nonPIECP group and this difference is significant at the 10% significance level. While estimates that account for the censoring mechanism (see Equation 4) and observable characteristics also suggest that PIECP participants have a job loss hazard that is roughly 6% lower than the nonPIECP cohort, but this difference is not statistically significant. Figure 6.B. plots survival curves by gender; although there is no visual difference between PIECP and non-PIECP for men, the female non-PIECP group has a significantly lower survival curve than men non-PIECP (5% significance level), women PIECP (5% significance level), and male PIECP (10% significance level), but it appears that this difference dissipates over time. Nonetheless, Kaplan-Meier survival curves do not control for covariates, therefore, Table VI displays the results obtained from incorporating gender interaction terms and covariates into the analysis. For the most part, the OLS results from Equations 1 and 2 indicate no difference in the employment duration between male PIECP and female non-PIECP workers and the reference group, the male non-PIECP cohort, after controlling for other factors. However, the female PIECP cohort maintains employment almost 5 months longer than male non-PIECP workers and almost 6 months longer than their female non-PIECP counterparts, and these differences are significant at the 5% and 1%, respectively, significance level. The regression analyses from the Cox proportional hazard model (Equations 3 and 4) are similar to those from the OLS regressions: after controlling for covariates the only statistically significant difference in the job loss hazard is between the female PIECP group and the female non-PIECP cohort. In particular, Equation 4 suggests that female PIECP workers have a baseline hazard that is roughly 25% below female non-PIECP workers, and this difference is significant at the 10% significance level. ! ! 30! Similar to the evaluation of the unemployment duration, the merits of using only complete cases to estimate the effects of the Prison Industry Enhancement Certification Program (PIECP) on the formal employment duration of its participants is investigated using multiple imputation. “Imputed Equation 4” in Table VI incorporates observations with missing values for the independent variables.27 By doing so, it is estimated that there is only a statistically significant difference between male PIECP and male non-PIECP workers. In particular, male PIECP workers have a job loss hazard that is 19% above that of male non-PIECP workers, and this difference is marginally significant at the 10% significance level. Moreover, female PIECP workers have a job loss hazard that is 17% below that of female non-PIECP workers (not the 25% that was estimated using complete cases), but this difference is no longer statistically significant. Like Smith et al. (2006), there is some evidence to indicate that PIECP increases the hazard of formal employment relative to non-PIECP workers. Contrary to the findings of Smith and her coauthors, there is much less proof that PIECP increases the duration of formal employment for its participants. In addition, the analysis taking into account gender effects determines that PIECP significantly increases the hazard of employment for its male and female participants but the effect is stronger for women. It is also revealed that, after controlling for missing data and unobservable characteristics, only male PIECP participants have a significantly different hazard from their male non-PIECP counterparts, but this difference is counter to theory. In particular, male PIECP participants have a higher job loss hazard than men in the non-PIECP group. !!!!!!!!!!!!!!!!!!!!!!!!!!!!!!!!!!!!!!!!!!!!!!!!!!!!!!!!!!!!! 27 Due to the insignificant findings in Table V, the discussion of the imputed analysis will focus on the results from Table VI. ! ! 31! Overall, the results imply that PIECP might work to increase the social capital (i.e., connections with employers) and hard skills (as measured by the time from release to formal employment) of its participants, which helps to improve their ability to obtain employment. Nonetheless, there doesn’t appear to be evidence that the program improves the soft skills (as measured by the duration of formal employment) of its participants. PIECP Participation and Reported Earnings The previous section determined that the Prison Industry Enhancement Certification Program (PIECP) decreases the time from release to employment, but does not lead to longer spells of formal employment. As previously mentioned, this implies that PIECP might improve social capital and cognitive (hard) skills, but does little to enhance the non-cognitive ability (soft skills) of its participants. However, an alternative explanation is that PIECP participants obtain employment faster, but the quality of these more quickly obtained jobs is inferior to those obtained by non-PIECP workers. One measure of job quality is earnings. Thus, this section will examine how PIECP participation affects free labor market wages. Further justification for analyzing the effect of PIECP on earnings is that in several theories of criminal behavior, higher earnings can lead to a desistance from unlawful behavior. For example, in the economic model of crime, wages are the opportunity costs to committing a crime; therefore, individuals that earn higher wages will be less likely to participate in criminal behavior because it will be more costly to do so. As previously mentioned, segmented labor market theories also predict that better employment and higher earnings in the primary labor market will lead to desistance from criminal behavior through a change in taste for work. It is hypothesized that PIECP workers will have greater reported weekly earnings than individuals who do not participate in the program during incarceration. It is also hypothesized that women ! ! 32! will earn less than their male counterparts but participation in PIECP will help to narrow the gender wage gap. OLS is used to analyze the impact of PIECP participation on weekly reported earnings on the subset of individuals who obtain employment after release. The results are presented in Table VII. Surprisingly, gender interactions with PIECP are insignificant to the model; as a result, they are excluded from the subsequent analysis. Equation 1 investigates whether there is a difference in earnings between PIECP and non-PIECP without adjusting for control variables. Individuals that worked in PIECP during confinement earn roughly 19% more than PIECP participants, and this difference is statistically significant at the 5% significance level. Equation 2 includes control variables without their interactions with gender. The results show that after controlling for other factors, PIECP participants earn about 21% more than the non-PIECP group and this difference is significant at the 5% significance level. While gender interactions with PIECP are not significant, gender interaction terms with the remaining covariates are jointly significant to the model at the .1% significance level; therefore, Equation 3 incorporates gender interaction terms. The magnitude of the effect of PIECP on weekly earnings decreased slightly to roughly 20%, and the significance level increased to 10%. Turning to “Imputed Equation 3” in Table VII, we see a decrease in the estimated coefficients and standard errors, indicating that analyzing only the complete cases may cause biased point estimates. Nevertheless, the results suggest that the difference between PIECP participant earnings and the non-PIECP group is about 16%, and this difference is statistically significant at the 5% significance level, ceteris paribus. Even after controlling for missing data and additional covariates, there is still a positive significant difference between the earnings of PIECP and non-PIECP workers. These findings generally support the conclusions from Smith et al. (2006) that PIECP improves the earnings of its participants. ! ! 33! VI. Discussion The previous labor market analysis finds that participants in the Prison Industry Enhancement Certification Program (PIECP) are employed more quickly and that this effect is stronger for women. It is also discovered that PIECP participants earn significantly more than their non-PIECP counterparts, but this effect does not vary by gender. Nonetheless, even though PIECP workers have greater employment and earn significantly more than their non-PIECP counterparts, there exist little evidence that the program increases the duration of formal employment. Quite the reverse, after controlling for observable characteristics and missing data, the results suggest that male PIECP have a significantly higher job loss hazard. These results imply that higher pay alone is not enough to lead to stable employment, contrary to segmented labor market theories that purport that better quality employment will change tastes for work and increase job stability. Heckman et al. (2006) find non-cognitive ability significantly influences labor market outcomes and criminal behavior. Therefore, it is reasonable to conclude that PIECP may not be very effective at enhancing the non-cognitive ability of its participants. One way to indirectly test this non-cognitive ability hypothesis is by comparing recidivism outcomes. Heckman et al. (2006) find that non-cognitive ability might be more important in altering criminal behavior than cognitive traits. This indicates that if the Prison Industry Enhancement Certification Program improves non-cognitive ability, then program participants should have higher survival recidivism curves compared to non-participants. Put differently, if PIECP does not influence non-cognitive ability then there should be no difference in recidivism between those that worked in PIECP during confinement and those that did not. Figure 7.A. and 7.B. display arrest, conviction, and incarceration survival curves for the PIECP and non-PIECP groups for the pooled sample and by gender. Figure 7.A. shows that ! ! 34! there is no difference between the recidivism of PIECP and non-PIECP groups for the pooled sample, which provides further evidence that PIECP does not affect the non-cognitive ability of its participants. However, when the recidivism survival curves are disaggregated by gender, as seen in Figure 7.B., there is a significant difference between the survival curves of the PIECP and non-PIECP groups. In particular, female PIECP participants have significantly higher arrest survival rates than male PIECP workers (10% significance level) and the male non-PIECP group (5% significance level). Although there is no significant difference in the arrest survival curves between female PIECP and female non-PIECP, Figure 7.B. shows that women PIECP workers have the highest survival rates out of all groups. Similar to the job loss analysis, this does seem to provide additional, albeit weak, support that PIECP might be more effective at improving the non-cognitive skills of women. While there is no difference in the survival curves for conviction for any of the cohorts, the male PIECP group has higher incarceration survival rates than the female PIECP group (10% significance level) and the female non-PIECP cohort (1% significance level). Similar to the survival analysis for arrests, the difference in incarceration seems to vary by gender, not PIECP status. The same pattern emerges with the sample recidivism data presented in Table VIII.B. Regardless of PIECP status, women have a lower probability of arrest than men, but they have a higher probability of incarceration, suggesting that differences in recidivism are driven by gender and not PIECP. The absence of an effect on criminal behavior implies that PIECP has no influence on criminal behavior, and, thus, no effect on non-cognitive ability. What is more interesting is almost the entire sample (roughly 92%) experiences job loss during the follow up period. One explanation for this occurrence is that these periods of job loss correspond to high rates of incarceration for the overall sample. However, Figure 8, which compares the Kaplan-Meier survival curves for job loss and incarceration, shows a very steep ! ! 35! survival curve for job loss relative to a flat curve for incarceration, indicating that it is very unlikely that re-incarceration is explaining the widespread job loss. Moreover, Table VIII shows that the estimated sample recidivism rates are far lower than national estimates. This begs the question: if job loss is not due to high rates of recidivism, what are the factors that determine job loss in this sample?28 To answer this question the labor market dynamics of men and women are investigated in order to understand the factors that impact the risk of employment, the risk of job loss, and earnings. Table IX presents the fully specified imputed results from Tables III-VII for the duration of unemployment, the duration of formal employment, and the log of weekly earnings. Beginning with the duration of unemployment, the results show that prior work experience, previous occupation, having a physical medical special need, and marital status significantly influence the employment hazard. In particular, each additional quarter worked prior to prison increases the hazard of formal employment by roughly 4%, and this increase is significant at the .1% significance level, holding other factors constant. Thus, the post-release formal employment hazard of a person that has a year of work experience prior to incarceration is estimated to be 15% higher than someone with no work experience prior to confinement, all else equal, and this effect does not significantly vary by gender. Moreover, a single person will have a formal employment hazard that is roughly 12% below that of a non-single person; and an individual with a physical ailment will have a hazard of formal employment that is roughly 15% below a person without a physical health problem, ceteris paribus. These differences do not vary by gender and are significant at the 10% significance level. Although previous occupation is !!!!!!!!!!!!!!!!!!!!!!!!!!!!!!!!!!!!!!!!!!!!!!!!!!!!!!!!!!!!! 28 I would like to thank an anonymous referee for pointing this out. ! ! 36! jointly insignificant, a one-tailed hypothesis29 test reveals that individuals with a previous occupation in retail have a formal employment hazard that is roughly 36% greater than those that were unemployed or out of the labor force prior to incarceration, and this difference is significant at the 10% significance level. Factors that have differential effects on the hazard of formal employment by gender are race (5% significance level), the number of previous incarcerations (10% significance level), and the number of disciplinary reports during incarceration (10% significance level). The number of disciplinary reports has an unexpected positive effect on the formal employment hazard for women, ceteris paribus, while this variable has the anticipated negative sign for men. Specifically, each disciplinary report lowers the formal employment hazard for men by an insignificant .5%, but increases the formal employment hazard for women by roughly 1.3 %, ceteris paribus. For women, this variable could be correlated with unobservable characteristics (such as confidence), holding criminal history constant, which may lead to greater success with obtaining employment. In addition, each additional prior incarceration has no statistically significant effect on the formal employment hazard for men, but significantly (10% significance level) lowers the formal employment hazard for women by roughly 4.3%, ceteris paribus. Finally, while black men have an employment hazard that is a statistically insignificant 3% below that of white men (holding other factors constant), black women have an employment hazard that is a significant (5% significance level) 27% lower than black men. Moving on to the job loss hazard (also shown in Table IX), we see that there are many factors explaining the duration of formal employment. In general, years of education (5% significance level), number of quarters worked prior to incarceration (1% significance level), !!!!!!!!!!!!!!!!!!!!!!!!!!!!!!!!!!!!!!!!!!!!!!!!!!!!!!!!!!!!! 29 It is hypothesized that anyone with a previous occupation should have a shorter duration of unemployment, a greater duration of formal employment, and greater earnings than those that were unemployed or out of the labor force prior to prison. ! ! 37! previous occupation (jointly significant at the 5% significance level), mental health (1% significance level), time served (.1% significance level), time served squared (.1% significance level), the number of previous incarcerations (5% significance level), the type of offense committed (jointly significant at the 5% significance level), and history of juvenile delinquency (1% significance level) significantly affect the job loss hazard. In terms of the human capital variables, each additional year of education leads to a reduction in the job loss hazard of about 6%, each additional quarter worked decreases the job loss hazard by about 3.2%, men that have a previous occupation in an office have a job loss hazard that is 6.7% below men that were not working prior to prison, men that worked in unskilled professions prior to incarceration have a job loss hazard that is 8.8% above those that were unemployed or out of the labor force before prison, men that were self-employed have a job loss hazard that is roughly 78% above those unemployed or out of the labor force prior to incarceration, men that were in skilled professions prior to confinement have a job loss hazard that is 11% below those that were unemployed or out of the work force prior to prison, men that worked in the food industry prior to incarceration have a job loss hazard that is 2.8% below those that were unemployed or out of the work force prior to confinement, and men that worked in retail prior to prison have a job loss hazard that is 51% above those that were not working prior to incarceration. Even though the joint hypothesis test finds that previous occupation is jointly significant to the model, only men that were self-employed prior to incarceration had a statistically significant (1% significance level) difference in the job loss hazard from those that were either unemployed or out of the labor force prior to incarceration. In addition, individuals with a mental health issue have a job loss hazard that is roughly 35% above individuals that do not. It is interesting to note that while physical health seems to be an important factor for obtaining a job, it is mental health that is relevant to maintaining employment. ! ! 38! Turning to the criminogenic variables, each additional year of time served decreases the job loss hazard until about 13 years of incarceration, past this point each additional year of incarceration will increase the job loss hazard, holding other factors constant. Moreover, the job loss hazard increases by about 4% for each prior incarceration, and a person with a history of juvenile delinquency has a job loss hazard that is about 31% greater than those that do not, ceteris paribus. Finally, the type of offense committed appears to have a differential effect on the job loss hazard: men committing drug crimes and crimes in the other category have a job loss hazard that is 12% and 63%, respectively, above men that committed crimes against a person, while men committing property crimes have a job loss hazard that is roughly 4% below men that commit crimes against a person, ceteris paribus. Only the difference in the job loss hazard between those that committed crimes in the “other” category was individually statistically different (1% significance level) from those that committed crimes against a person. Except for the type of occupation and the type of crime committed, no other factors significantly vary by gender for the duration of formal employment analysis. Specifically, the job loss hazard of women for all occupations held prior to imprisonment is lower than men that worked in the same occupations. Specifically, women that worked in an office job have a job loss hazard that is roughly 27% below men that worked in an office, women that worked in unskilled professions have a job loss hazard that is about 25% below men that worked in the same occupation, women that were self-employed prior to confinement have a job loss hazard that is 28% below their male counterparts, women that worked in skilled professions have a job loss hazard roughly 26% below men in skilled professions, women employed in the food industry prior to incarceration have a job loss hazard that is 46% below their male counterparts, and women that worked in retail prior to imprisonment have a job loss hazard that is roughly 69% below men that were employed in retail. Only the differences between men and women ! ! 39! that worked in retail and food occupations prior to incarceration were statistically significant at the 5% significance level. As previously mentioned, the effect of the type of crime committed for the incarceration offense also significantly varies by gender. Women that committed property crimes have a job loss hazard that is roughly 18% above men that committed property crimes, women that committed drug crimes have a job loss hazard that is roughly 28% below their male counterparts, and women that committed crimes in the other category have a job loss hazard that is roughly 86% above men that were incarcerated for crimes in the other category. The difference between men and women that were incarcerated for crimes in the other category is significant at the 10% significance level, while the other differences are not individually significant. Finally, there are a number of variables that significantly explain the log of weekly earnings. Education, job experience, mental health, time served, and the number of previous incarcerations are statistically significant to the model at the .1%, 1%, 1%, .1%, and 1% significance levels, respectively. In particular, each additional year of education increases weekly earnings by roughly 7.5%, a person with a history of mental illness earns about 27% less than a person that does not have a record of mental health problems, and each additional prior incarceration is associated with a 7.7% reduction in weekly earnings, holding other factors constant. Increases in prior work experience and the number of years served in prison cause an increase in weekly earnings, but they do so at a diminishing rate. For example, maximum weekly earnings occur at about 15 years of time served, additional time served past this point leads to decreases in earnings, ceteris paribus. Moreover, marital status and substance abuse have a statistically significant differential effect by gender. Women with a prior substance abuse problem earn roughly 37% less than men with a history of substance abuse, ceteris paribus, and ! ! 40! this difference is significant at the 10% significance level, while, single women earn 32% less than single men, and this difference is significant at the 5% significance level. To conclude, labor market outcomes are influenced by human capital investments, criminogenic factors, and a few demographic characteristics. Black women, single women, and women with a more extensive criminal record (as measured by prior incarcerations) face additional barriers in the labor market, ceteris paribus. The findings indicate that cognitive skills are important for obtaining a job, maintaining employment, and improving earnings; however, variables that are also highly correlated with non-cognitive ability (Heckman et al. 2006) significantly affect labor market outcomes. Returning to the original concern of job loss, holding program participation constant, individuals that are most successful at maintaining employment are more educated, have more work experience, do not have a history of mental health problems, were not self employed prior to incarceration, served a maximum of 13 years in prison, did not commit an offense in the other category, have fewer prior incarcerations, and do not have a history of juvenile delinquency. Moreover, if they are women, they also worked in food or retail occupations prior to incarceration. VII. Conclusion The Prison Industry Enhancement Certification Program (PIECP) program is a unique federal initiative that allows private industry to utilize prison labor for the manufacturing of goods and services. The program is thought to benefit the public (e.g., the state, tax payers, victims) and the inmate (e.g., enhanced skills, leading to higher paying jobs upon release). This paper investigates how participation in PIECP affects the labor market outcomes of men and women released from prison between January of 1996 and June of 2001. It also provides a detailed investigation into the labor market dynamics of formerly incarcerated men and women. In an attempt to improve causal inference, the data are compiled of individuals who participated ! ! 41! in PIECP while in prison, and those that did not experience the treatment but are similar to its participants. Therefore, caution should be taken when generalizing these results to the state prison population at large. Nonetheless, while descriptive analyses reveal that this sample is older, whiter, more educated, and has more women than national estimates, the sample also appears to have greater criminogenic needs and more mental health problems than the state prison population at large. The findings indicate that PIECP may help to increase the formal employment hazard of its participants, with a stronger effect for female PIECP workers. These results are heavily dependent on the model taking into consideration individuals that obtain employment immediately upon release from prison, which implies that PIECP operates by removing supply side barriers to employment faced by the formerly incarcerated (Holzer 2007). There is little proof that PIECP has a significant impact on the duration of formal employment, suggesting that the program is less effective at enhancing the soft skills (non-cognitive ability) of its participants. What’s more, after controlling for missing data and observable traits, male PIECP participants are found to have a significantly higher job loss hazard. This implies that PIECP increases formal employment along the extensive margin, but has little effect on the intensive margins. In terms of earnings, PIECP significantly improves the post-release earnings of its participants, but this effect does not vary by gender. Job loss is extremely high in the sample, with 92% of those employed losing their jobs during the follow up period. Re-incarceration rates, which are well below national estimates, are too low to explain this fact. A detailed analysis of labor market dynamics reveal that job loss is determined by variables associated with both non-cognitive and cognitive factors. In general, labor market outcomes are explained by human capital investments, criminogenic factors, and some demographic characteristics. It is also discovered that black women, single women, and ! ! 42! women with more extensive criminal histories face greater barriers on the labor market than their male counterparts. Given the caveats to the data previously discussed, there are some interesting implications that can be taken from these results. The findings uncover that exposure to a private sector work environment improves reported earnings and formal employment along the extensive margin. Contrary to segmented labor market theories, better job quality, as measured by greater reported earnings, is not enough to improve participation in the labor market: Prison Industry Enhancement Certification Program (PIECP) participants did not significantly maintain employment for longer periods despite having greater reported weekly earnings. In fact, the findings imply that if work preferences remain unchanged, then greater earnings could lead male PIECP participants to decrease their formal employment on the intensive margin. Heckman et al. (2006) suggests that non-cognitive, not cognitive, ability is most important in influencing wage and employment outcomes, and that non-cognitive ability is the leading factor explaining differences in criminal participation. The fact that PIECP is found to have no effect on criminal behavior or job stability suggests that non-cognitive skills cannot be improved by simply exposing those incarcerated to a private sector work environment. More research is needed to precisely isolate the mechanisms through which prison work programs operate to influence labor market outcomes, and to investigate the relative effectiveness of cognitive versus non-cognitive skill development on the labor market success of the formerly incarcerated. In addition, research should be undertaken to understand the labor market experiences of formerly incarcerated women. Finally, given the small sample size of the data used in this study, additional data should be collected to confirm these results, and to investigate the effects of state ran prison industrial work programs on the reentry of the formerly incarcerated. ! ! 43! References Angrist, J. D., & Pischke, J. S. (2009). Mostly harmless econometrics: An empiricist's companion. Princeton, NJ: Princeton University Press. Atkinson, R., & Rostad, K.A. (2003). Can inmates become an integral part of the U.S. workforce?. Paper presented at the Urban Institute Reentry Roundtable. New York, NY: New York University Law School. Auerbach, B. J. (2001). Emerging practices: Wage policies and practices for the prison industries enhancement certification program (PIECP). Washington, DC: Bureau of Justice Assistance. Bayer, P., Hjalmarsson, R., & Pozen, D. (2009). Building criminal capital behind bars: Peer effects in juvenile corrections. The Quarterly Journal of Economics, 124(1), 105-147. Beck, A. J., & Harrison, P. M. (2001). Prisoners in 2000. Washington, DC: Bureau of Justice Statistics.. Retrieved from http://bjs.gov/index.cfm?ty=pbdetail&iid=927. Becker, G. S. (1968). Crime and punishment: An economic approach. The Journal of Political Economy, 76(2), 169-217. Block, M. K., & Heineke, J.M. (1972). A comment on uncertainty and household decisions. Review of Economic Studies, 39(4), 523-525. Block, M. K., & Heineke, J.M. (1973). The allocation of effort under uncertainty: The case of risk-averse behavior. The Journal of Political Economy, 81(2), 376-385. Block, M. K., & Lind R.C. (1975). Crime and punishment reconsidered. The Journal of Legal Studies, 4(1), 241-247. Block, M. K., & Heineke, J.M. (1975). A labor theoretic analysis of the criminal choice. The American Economic Review, 65(3), 314-325. Bonczar, T. P. (2003). Prevalence of Imprisonment in the U.S. Population 1974-2001 (NCJ ! ! 44! 197976). Washington, DC: US Department of Justice. Retrieved from http://bjs.ojp.usdoj.gov/content/pub/pdf/piusp01.pdf. Bonczar, T. P., & Beck, A.J. (1997). Lifetime likelihood of going to gtate or federal prison (NCJ160092). Washington, DC: US Department of Justice. Retrieved from http://www.ojp.usdoj.gov/bjs/pub/pdf/Llgsfp.pdf. Borus, M.E., Hardin, E. & Terry, P.A. (1976). Job-placement services for ex-offenders: An evaluation of the michigan comprehensive offender manpower program (comp) jobplacement efforts. The Journal of Human Resources, 11(3), 391401. Bureau of Justice Assistance. (1999). Prison industry enhancement certification program guideline (RIN 1121–AA36). Federal Register, 64(66), 17000-17014. Retrieved from http://frwebgate.access.gpo.gov/cgi-bin/getdoc.cgi?dbname=1999_register&docid=998575-filed.pdf. Bureau of Justice Assistance. (2004). Prison industry enhancement certification program (NCJ 203483). Washington, DC: US Department of Justice. Retrieved from http://www.ncjrs.gov/pdffiles1/bja/203483.pdf . Bureau of Justice Statistics’ Federal Justice Statistics Program. Bureau of Prisons - Extract from BOP's online Sentry System, FY 2005. Washington, DC: US Department of Justice. Retrieved from http://www.bjs.gov/fjsrc/. Bureau of Justice Statistics. (2009). Key facts at a glance. Washington, DC: US Department of Justice. Bushway, S., & Reuter, P. (2002). Labor markets and crime risk factors. In D. P. Farrington, D.L. McKenzie, L. Sherman, & B.C. Welsh (Ed.), Evidenced-Based Crime Prevention (pp. 198-240). London and New York: Routledge. ! ! 45! Bushway, S. D., Stoll, M. A., & Weiman, D. (Eds.). (2007). Barriers to reentry?: The labor market for released prisoners in post-industrial America. New York, NY: Russell Sage Foundation. Cain, G. G. (1976). The challenge of segmented labor market theories to orthodox theory: a survey. Journal of Economic Literature, 14(4), 1215-1257. Cameron, A.C., & Travedi, P.K. (2005). Microeconometrics: Methods and applications. New York, NY: Cambridge University Press. Card, D., Chetty, R., Feldstein, M., & Saez, E. (2010). Expanding Access to Administrative Data for Research in the United States. In American Economic Association, Ten Years and Beyond: Economists Answer NSF's Call for Long-Term Research Agendas. Retrieved from: http://dx.doi.org/10.2139/ssrn.1888586! Chandra, Amitabh (2003). Is the convergence in the racial wage gap illusory (No. w9476), Cambridge, MA: National Bureau of Economic Research. Charles, K.K., Luoh, M.C. (2010). Male incarceration, the marriage market, and female outcomes. The Review of Economics and Statistics, 92(3), 614-627. Chung, C.F., Schmidt, P., & Witte, A.D. (1991). Survival analysis: a survey. Journal of Quantitative Criminology, 7(1), 59-98. Cox, R. (2009). An Economic Analysis of Prison Labor (Doctoral Dissertation). Economics Dissertations. Retrieved from: http://works.bepress.com/robynn_cox/1 Cox, R. (2010). Crime, incarceration, and employment in light of the great recession. The Review of Black Political Economy, 37(3-4), 283-294. Cox, R. (2012). The Impact of Mass Incarceration on the Lives of African American Women. The Review of Black Political Economy, 39(2), 203-212. ! ! 46! Cox, R. (2012). Erratum to: The Impact of Mass Incarceration on the Lives of African American Women. The Review of Black Political Economy, 39(2), 213-215. Donders, A.R.T., van der Heijden, G.J.M.G., Stijnen, T. and Moons, K.G.M. (2006). Review: A gentle introduction to imputation of missing values. Journal of Clinical Epidemiology, 59 (10), 1087-1091. Ehrlich, I. (1973). Participation in illegitimate activities: A theoretical and empirical investigation. The Journal of Political Economy, 81(3), 521-565. Ehrlich, I. (1996). Crime, punishment, and the market for offenses. Journal of Economic Perspectives, 10(1), 43-67. Eide, E. (2000). Economics of criminal behavior. In B. Bouckaert & G. De Geest (eds.), Encyclopedia of law and economics, volume V. The economics of crime and litigation (pp. 345-389). Cheltenham, United Kingdom: Edward Elgar Publishing Limited. Retrieved from http://encyclo.findlaw.com/8100book.pdf. Finlay, K. & Neumark, D. (2010). Is marriage always good for children?: Evidence from families affected by incarceration. Journal of Human Resources, 45(4), 1046-1088 Freeman, R. B. (1996). Why do so many young american men commit crimes and what might we do about it? The Journal of Economic Perspectives, 10(1), 25-42. Freeman, R. (2003). Can we close the revolving door?: Recidivism vs employment of exoffenders in the US. Paper presented at the Urban Institute Reentry Roundtable. New York, NY: New York University Law School. Gallagher, D. J. & Edwards, M.E. (1997). Prison industries and the private sector. Atlantic Economic Journal, 25(1), 91-98. Glaze, L. E., & Maruschak, L. M. (2008). Parents in prison and their minor children (NCJ ! ! 47! 222984). Washington, DC: US Department of Justice. Retrieved from http://bjs.gov/content/pub/pdf/pptmc.pdf. Glaze, L. E. & Parks, E. (2012). Correctional populations in the United States, 2011 (NCJ 239972). Washington, DC: US Department of Justice. Retrieved from http://www.bjs.gov/content/pub/pdf/cpus11.pdf . Gould, E. D., Weinberg, B. A., & Mustard, D. B. (2002). Crime rates and local labor market opportunities in the United States: 1979–1997. Review of Economics and Statistics, 84(1), 45-61. Government Accounting Office. (2000). State and federal prisoners: profiles of inmate characteristics in 1991 and 1997 (GAO/GGD-00-117). Washington, DC: US Genearl Accounting Office. Retrieved from www.gao.gov/archive/2000/gg00117.pdf. Grogger, J. (1992). Arrests, persistent youth joblessness, and black/white employment differentials. The Review of Economics and Statistics, 74(1), 100-106. Grogger, J. (1995). The effect of arrests on the employment and earnings of young men. The Quarterly Journal of Economics, 110(1), 51-71. Grogger, J. (1998). Market wages and youth crime. Journal of Labor Economics, 16(4), 756-791. Grubb, F. (2001). The market evaluation of criminality: Evidence from the auction of British convict labor in America, 1767-1775. The American Economic Review, 91(1), 295-304. Heckman, J. J., & Hotz, V. J. (1989). Choosing among alternative nonexperimental methods for estimating the impact of social programs: The case of manpower training. Journal of the American Statistical Association,84(408), 862-874. Heckman, J. J., Stixrud, J., & Urzua, S. (2006). The Effects of Cognitive and Noncognitive Abilities on Labor Market Outcomes and Social Behavior. Journal of Labor Economics, 24(3), 411-482. ! ! 48! Heineke, J. M. (1978). Economic models of criminal behavior: An overview. In J. M. Heineke (Ed.), Economic Models of Criminal Behavior (pp. 1-33). New York, NY: North-Holland Publishing Company. Heineke, J. M. (1988). Crime deterrence, and choice: testing the rational behavior hypothesis. American Sociological Review, 53(2), 303-305. Holzer, H. J. (2007). Collateral costs: the effects of incarceration on the employment and earnings of young workers (No. 3118). Bonn, Germany: Institute for the Study of Labor. Holzer, H. J., Offner, P., & Sorensen, E. (2005). Declining employment among young black lesseducated men: The role of incarceration and child support. Journal of Policy Analysis and Management, 24(2), 329–350. Holzer, H. J., Raphael, S., & Stoll, M. A. (2004). How willing are employers to hire exoffenders. Focus, 23(2), 40-43. Holzer, H., Raphael,S. and Stoll, M.A. (2006). Perceived criminality, criminal background checks, and the racial hiring practices of employers. Journal of Law and Economics, 49(2), 451-480. Hughes, T.A., Wilson, D.J., & Beck, A.J. (2001). Trends in state parole, 1990–2000 (NCJ 184735). Washington, DC: US Department of Justice. Retrieved from www.bjs.gov/content/pub/pdf/tsp00.pdf. Hughes, T. A., & Wilson, D. J. (2003). Reentry trends in the United States. Washington, DC: US Department of Justice, Bureau of Justice Statistics. Retrieved from http://www.bjs.gov/content/pub/pdf/reentry.pdf. Imbens, G. (2013). Lectures on Evaluation Methods. Lecture Presented at the 2013 Main Causal Inference Workshop. Chicago, IL: Northwestern University. Imbens, G. and Rubin, D. (2012). Causal Inference in Statistics and Social Sciences. ! ! 49! Unpublished manuscript. Johnson, R. C., & Raphael, S. (2009). The effects of male incarceration dynamics on acquired immune deficiency syndrome infection rates among African American women and men. Journal of Law and Economics, 52(2), 251-293. Jung, H. (2011). Increase in the length of incarceration and the subsequent labor market outcomes: Evidence from men released from illinois state prisons. Journal of Policy Analysis and Management, 30(3), 499–533. Kalbfleish, J. D., Prentice, R.L. (1980). The statistical analysis of failure time data. New York, NY: Wiley-Interscience. Kiefer, N. M. (1988 ). Economic duration data and hazard functions. Journal of Economic Literature, 26(2), 646-679. Kling, J. R., & Krueger, A.B. (2001). Costs, benefits and distributional consequences of inmate labor (Working Paper #449). Princeton, NJ: Princeton University Industrial Relations Section. Kling, J. R. (1999). The effect of prison sentence length on the subsequent employment and earnings of criminal defendants (No. 208). Princeton, NJ: Princeton University, Woodrow Wilson School of International Affairs. Krienert, J. L. (2005). Bridging the gap between prison and community employment: An initial assessment of current information. Criminal Justice Studies, 18(4), 293-303. LaLonde, R. J., & Cho, R. M. (2008). The impact of incarceration in state prison on the employment prospects of women. Journal of Quantitative Criminology, 24(3), 243–265. Lancaster, T. (1990). The econometric analysis of transition data. New York, NY: Cambridge University Press. Langan, P. A., & Levin, D.J. (2002). Recidivism of prisoners released in 1994 (NCJ 193427). ! ! 50! Washington, DC: US Department of Justice. Retrieved from http://bjs.ojp.usdoj.gov/content/pub/pdf/rpr94.pdf. Little, R.J.A. and Rubin, D.B. (2002) Statistical Analysis with Missing Data (2nd ed.). Hoboken, NJ: J Wiley & Sons. Lochner, L. & Moretti, E. (2004). The effect of education on crime: evidence from prison inmates, arrests, and self-reports. American Economic Review, 94 (1), 155-189. Lopoo, L. M., & Western, B. (2005). Incarceration and the formation and stability of marital unions. Journal of Marriage and Family, 67(3), 721-734. Ludwig, J., Duncan, G.J., & Hirschfield, P. (2001). Urban poverty and juvenile crime: Evidence from a randomized housing-mobility experiment. The Quarterly Journal of Economics, 116 (2), 655-679. Machin, S. & Meghir, C. (2004). Crime and economic incentives. Journal of Human Resources, 39(4), 958-979. Maltz, M.D. ([1984] 2001). Recidivism. Originally published by Academic Press, Inc., Orlando, Florida. Internet edition available at http://www.uic.edu/depts/lib/forr/pdf/crimjust/recidivism.pdf. Mocan, H. N., Billups, S.C., & Overland, J. (2005). A dynamic model of differential human capital and criminal activity. Economica, 72, 655-681. Myers, S.L., Jr. (1983). Estimating the economic model of crime: Employment versus punishment effects. The Quarterly Journal of Economics, 98 (1), 157-166. Needels, K. E. (1996). Go directly to jail and do not collect? a long-term study of recidivism, employment, and earnings patterns among prison releasees. Journal of Research in Crime & Delinquency, 33(4), 471-496. ! ! 51! Pager, D., Western, B., & Suggie, N (2009). Sequencing disadvantage: barriers to employment facing young black and white men with criminal records. The ANNALS of the American Academy of Political and Social Science, 623(1), 195-213. Pencavel, J. (1986). Labor supply of men: a survey. In O. Ashenfelter & R. Layard (Eds.) Handbook of labor economics, Volume 1 (pp. 3-102). Amersterdam, The Netherlands: Elsevier Science B.V. Petersik, T. W., Nayak, T.K., & Foreman, M.K. (2003). Identifying beneficiaries of PIE inmate incomes: Who benefits from wage earnings of inmates in the prison industry enhancement (PIE) program? Washington, DC: The George Washington Center for Economic Research. Pettit, B., & Lyons, C. J. (2007). Status and the stigma of incarceration: The labor-market effects of incarceration, by race, class, and criminal involvement. In S.D. Bushway, M.A. Stoll, & D. Weiman (Eds.) Barriers to reentry? The labor market for released prisoners in post-industrial America (pp. 203-226). New York, NY: Russell Sage Foundation. Piehl, A. (2003). Crime, work, and reentry. Paper presented at the Urban Institute Reentry Roundtable. New York, NY: New York University Law School. Retrieved from www.urban.org/UploadedPDF/410856_Piehl.pdf. Piehl, Anne Morrison (2009). Preparing prisoners for employment: The power of small rewards (Civic Report No. 57). New York, NY: Manhattan Institute for Policy Research. Pinard, M. (2010). Reflections and perspectives on reentry and collateral consequences. The Journal of Criminal Law and Criminology, 100(3), 1213-1224. Pryor, F. L. (2005). Industries behind bars: an economic perspective on the production of goods and services by U.S. prison industries. Review of Industrial Organization, 27, 1-16. Raphael, S., & Winter‐Ebmer, R. (2001). Identifying the effect of unemployment on ! ! 52! crime. Journal of Law and Economics, 44(1), 259-283. Roman, C.G. & Travis, J. (2006). Where will I sleep to- morrow? Housing, homelessness, and the re- turning prisoner. Housing Policy Debate, 17(2), 389–418 Royston, P. (2005). Multiple imputation of missing values: update. Stata Journal, 5(2), 188-201. Retrieved from: http://www.stata-journal.com/sjpdf.html?articlenum=st0067_1. Rubin, D. B. (1987). Multiple Imputation for Non-response in Surveys. Hoboken, NJ: John Wiley & Sons, Inc.. Sampson, R. J., & Laub, J.H. (1992). Crime and deviance in the life course. Annual Review of Sociology, 18, 63-84. Saylor, W. G., & Gaes, G. G. (1997). Training inmates through industrial work participation and vocational and apprenticeship instruction. Corrections Management Quarterly, 1(2), 3243. Saylor, W. G., & Gaes,G.G. (2001). The differential effect of industries and vocational training on post release outcome for ethnic and racial groups: Research note. Corrections Management Quarterly, 5(4), 17-24. Schafer, J.L. (1999). Multiple imputation: a primer. Statistical Methods in Medical Research, 8(1), 3-15. Schmidt, P., & Witte, A.D. (1984). An economic analysis of crime and justice. Orlando, FL: Academic Press, Inc. Sjoquist, D. L. (1973). Property crime and economic behavior: some empirical results. The American Economic Review, 63(3), 439-446. Smith, C. J., Bechtel, J., Patrick, A., Smith, R.R., & Wilson-Gentry, L. (2006). Correctional industries preparing inmates for re-entry: recidivism & post-release employment. Unpublished report. Retrieved from ! ! 53! http://www.ncjrs.gov/pdffiles1/nij/grants/214608.pdf. Solomon, A. L., Johnson, K.D., Travis, J., & McBride, E.C. (2004). From prison to work: the employment dimensions of prisoner reentry. Washington, DC: Urban Institute. Stata. (2003). Survival analysis and epidemiological tables. College Station, Texas: Stata Press. Travis, J. (2002). Invisible punishment: An instrument of social exclusion. In M. Mauer & M. Chesney-Lind (Eds.) Invisible punishment: The collateral consequences of mass imprisonment (15-36). New York, NY: The New Press. Tyler, J. H., & Kling, J.R. (2007). Prison-based education and re-entry into the mainstream labor market. In S.D. Bushway, M.A. Stoll, & D. Weiman (Eds.) Barriers to reentry? The labor market for released prisoners in post-industrial America (pp. 227-256). New York, NY: Russell Sage Foundation. Uggen, C. (2000). Work as a turning point in the life course of criminals: A duration model of age, employment, and recidivism. American Sociological Review, 65(4), 529-46. Uggen C, Manza J, Thompson M. (2006). Citizenship, democracy, and the civic reintegration of criminal offenders. The Annals of the American Academy of Political and Social Science, 605(1), 281–310. van Buuren, S., & Oudshoorn, K. (1999). Flexible multivariate imputation by MICE (Report PG/VGZ/99.054). Leiden, The Netherlands: Netherlands Organization for Applied Scientific Research. Retrieved from: http://www.stefvanbuuren.nl/publications/Flexible%20multivariate%20%20TNO99054%201999.pdf. Wallace, S. and Cox, R. (2012). The impact of incarceration on food insecurity among households with children (DP 2012-14), Lexington, KY: University of Kentucky Center for Poverty Research. ! ! 54! Waldfogel, J. (1994). The effect of criminal conviction on income and the trust "reposed in the workmen". The Journal of Human Resources, 29(1), 62-81. West, H.C. & Sabol, W.J. (2008). Prisoners in 2007 (NCJ 224280). Washington, DC: US Department of Justice. Retrieved from http://www.bjs.gov/content/pub/pdf/p07.pdf. West, H.C., Sabol, W.J., & Greenman, S.J. (2010). Prisoners in 2009 (NCJ 231675). Washington, DC: US Department of Justice. Retrieved from http://bjs.ojp.usdoj.gov/content/pub/pdf/p09.pdf. Western, B., Kling, J.R., & Weiman, D.F. (2001). The labor market consequences of incarceration. Crime and Delinquency, 47(3), 410-427. Western, B., & Pettit, B. (2000). Incarceration and racial inequality in men's employment. Industrial and Labor Relations Review, 54(1), 3-16. Western, B., & Pettit, B. (2005). Black-white wage inequality, employment rates, and incarceration. American Journal of Sociology, 111(2), 553-578. Wilson, D. B., Gallagher, C.A., & MacKenzie, D.L. (2000). A meta-analysis of correctionsbased education, vocation, and work programs for adult offenders. Journal of Research in Crime and Delinquency, 37(4), 347-368. Witte, A. D. (1980). Estimating the economic model of crime with individual data. The Quarterly Journal of Economics, 94(1), 57-84. Witte, A. D., & Tauchen, H. (1994). Work and crime: An exploration using panel data (Working Paper No. 4794). Cambridge, MA: National Bureau of Economic Research. Wooldridge, J. M. (2002). An econometric analysis of cross section and panel data. Cambridge, MA: Massachusetts Institute of Technology Press. ! ! 55! Appendix A: Definitions of PIECP, TI, and OTW (From Smith et al. 2006, pp. 28-31) Other than work (OTW) – Individuals involved in other prison activities (e.g., education or drug treatment programs) but not industry work. Those classified as OTW are not necessarily inactive while incarcerated. In addition, individuals classified as OTW and TI could perform the same task (i.e., laundry). However, one state may classify the task as OTW and the other as TI. OTW can be classified into two groups: 1) inmates that decide not to work and 2) those located in compulsory work states (which require the inmate to provide labor or go to school) that select jobs that demand minimal exertion and time. Some receive a nominal wage comparable to TI (e.g., $.25/hour). Traditional Industries (TI) – There are two kinds of Traditional Industries workers. One group performs work comparable to PIECP; however, the inmate does not earn a market wage and the manufactured goods cannot be purchased in the private sector. For instance, the inmate may not earn anything or could make a nominal amount such as a minimum of $.25 per hour up to about $1.25/ hour. The second group of workers does institutional maintenance (e.g., semi-skilled maintenance, administrative support, etc.). Each state determines what is considered to be a traditional industry within that state. Prison Industry Enhancement Certification Program –The tasks range from habitual manual labor (e.g., assembly line) to highly skilled labor (e.g., sheet metal welding). Inmates who work in facilities with the employer and manpower models have normal dealings with and are managed by a free world employee. This could transform the corrections atmosphere to an employment setting during the workday. This program is explained in detail in the body of the article, thus, the reader is referred to the section on PIECP for a more detailed discussion of the program. ! ! 56! Appendix B: Tables Table I. Summary Statistics and Normalized Differences PIECP Variable Years of Education Age at Release Number of Disciplinary Reports Time Served (years) Black Female Number of Previous Incarcerations History of Substance Abuse Offense Type: Person Offense Type: Property Offense Type: Drug Offense Type: Other History of Delinquency as a Juvenile Mental Health Issue (Prison Records Indicated a Mental Health Problem) Single Number of Children Previous Occupation: Office Previous Occupation: Unskilled labor/Assembly/Warehouse /Trucking Previous Occupation: Self-Employed Previous Occupation: Skilled Labor/construction Trades/Welding Previous Occupation: Food Previous Occupation: Retail Previous Occupation: Disabled (SSI)/ Student/Unemployed Medical Special Need (Prison Records Indicated Inmate Has a Physical Medical Special Need) Release to Employment (Quarters) Proportion of Individuals Employed PostIncarceration Employment to Job Loss(quarters) Proportion of Individuals with Job Loss Post-Incarceration Pre-Incarceration Weekly Earnings Post-Incarceration Weekly Earnings Number of Quarters Worked Prior to Incarceration Number of Quarters Worked Post Incarceration Non-PIECP Differences in Normalized Spread of Difference Distribution 0.08 -0.08 -0.03 0.02 -0.07 -0.10 0.05 0.11 0.04 0.01 0.02 0.01 0.00 0.23 0.12 -0.18 0.03 0.00 -0.04 -0.02 -0.02 -0.01 0.10 0.14 -0.04 -0.03 N 356 413 413 413 413 413 400 364 413 413 413 413 410 Mean 10.86 36.43 3.18 4.37 0.42 0.29 1.64 0.89 0.46 0.24 0.27 0.03 0.33 S.D. 1.93 8.22 6.67 3.46 0.49 0.45 3.09 0.31 0.50 0.43 0.45 0.18 0.47 N 719 804 804 804 804 804 788 720 804 804 804 804 798 Mean 10.70 36.69 3.66 4.22 0.40 0.28 1.63 0.85 0.44 0.26 0.28 0.02 0.35 S.D. 2.08 8.03 7.36 3.09 0.49 0.45 2.45 0.38 0.50 0.44 0.45 0.16 0.49 356 0.30 0.46 702 0.31 0.47 -0.02 -0.02 413 402 394 0.37 1.63 0.08 0.48 1.64 0.27 804 782 767 0.40 1.62 0.08 0.49 1.75 0.24 -0.07 0.01 0.02 -0.01 -0.07 0.11 394 0.33 0.47 767 0.32 0.48 0.02 -0.01 394 0.02 0.15 767 0.04 0.20 -0.08 -0.27 394 0.31 0.46 767 0.29 0.45 0.04 0.04 394 394 0.12 0.05 0.33 0.22 767 767 0.12 0.03 0.31 0.18 0.01 0.09 0.06 0.22 394 0.08 0.28 767 0.13 0.35 -0.14 -0.22 392 0.08 0.27 753 0.12 0.31 -0.14 -0.14 413 2.67 5.37 804 4.52 6.42 -0.31 -0.18 413 0.86 0.34 804 0.76 0.41 0.28 -0.19 357 5.80 5.01 608 5.45 5.20 0.07 -0.04 357 0.92 0.27 608 0.91 0.28 0.02 -0.03 412 395 55.74 202.06 112.16 186.28 802 788 49.04 151.09 113.01 190.04 0.06 0.27 -0.01 -0.02 413 2.50 4.87 803 1.81 3.63 0.16 0.29 413 7.07 5.80 804 5.98 6.39 0.18 -0.10 Normalized Differences are calculated by dividing the difference in the mean values of each group by the average standard deviation of the groups. Differences in the spread of the distributions between two groups are calculated for each variable by taking the difference in the log of the standard deviation for each group. ! ! 57! Table II. Comparison of Total Sample to Complete Cases Sample Total Sample Variables PIECP Years of Education Age at Release Number of Disciplinary Reports Time Served (years) Black Female Number of Previous Incarcerations History of Substance Abuse Offense Type: Person Offense Type: Property Offense Type: Drug Offense Type: Other History of Delinquency as a Juvenile Mental Health Issue (Prison Records Indicated a Mental Health Problem) Single Number of Children Previous Occupation: Office Previous Occupation: Unskilled labor/Assembly/Warehouse /Trucking Previous Occupation: Self-Employed Previous Occupation: Skilled Labor/construction Trades/Welding Previous Occupation: Food Previous Occupation: Retail Previous Occupation: Disabled (SSI)/ Student/Unemployed Medical Special Need (Prison Records Indicated Inmate Has a Physical Medical Special Need) Release to employment (Quarters) Proportion of Individuals Employed PostIncarceration Employment to Job Loss(quarters) Proportion of Ex-offenders with Job Loss Post-Incarceration Pre-Incarceration Weekly Earnings Post-Incarceration Weekly Earnings Number of Quarters Worked Prior to Incarceration Number of Quarters Worked Post Incarceration N 1217 1075 1217 1217 1217 1217 1217 1188 1084 1217 1217 1217 1217 1208 Mean 0.34 10.75 36.6 3.5 4.27 0.41 0.28 1.63 0.86 0.45 0.25 0.28 0.02 0.35 S.D. 0.47 1.99 8.11 7.19 3.5 0.49 0.45 2.61 0.34 0.5 0.43 0.45 0.15 0.48 N 898 898 898 898 898 898 898 898 898 898 898 898 898 898 Mean 0.33 10.72 36.34 3.2 4.39 0.47 0.32 1.93 0.85 0.45 0.25 0.28 0.02 0.34 S.D. 0.47 2.04 7.7 7.04 3.57 0.5 0.47 2.72 0.36 0.5 0.43 0.45 0.15 0.48 Normalized Differences 0.03 0.02 0.03 0.04 -0.03 -0.12 -0.09 -0.11 0.03 0 -0.01 0 0.01 0 1058 0.3 0.46 898 0.27 0.45 0.07 1217 1184 1161 0.39 1.63 0.08 0.49 1.73 0.27 898 898 898 0.45 1.62 0.08 0.5 1.69 0.27 -0.11 0 0 1161 0.33 0.47 898 0.32 0.47 0.01 1161 0.03 0.18 898 0.03 0.18 0 1161 0.29 0.46 898 0.28 0.45 0.03 1161 1161 0.12 0.04 0.33 0.19 898 898 0.13 0.04 0.33 0.19 -0.02 0.01 1161 0.11 0.31 898 0.12 0.33 -0.04 1145 0.1 0.3 898 0.08 0.28 0.07 1217 3.89 6.41 898 3.94 6.53 -0.01 1217 0.79 0.41 898 0.79 0.4 0 965 5.58 5.13 713 5.87 5.36 -0.06 965 0.92 0.28 713 0.89 0.31 0.07 1214 1183 51.31 168.11 112.72 183.39 897 888 41.12 176.22 103.04 194.41 0.09 -0.04 1216 2.04 4.23 898 1.41 3.26 0.17 1217 6.35 6.09 898 6.67 6.27 -0.05 ! ! Complete Cases 58! Table III. Time from Release to Employment OLS Variable PIECP Cox Proportional Hazard Model Imputed Imputed Imputed Equation 1 Equation 2 Equation 2 Equation 3 Equation 4 Equation 4 Equation 5 Equation 6 Equation 6 -1.8492*** -2.0328*** -1.6925*** 0.1626* 0.1009 0.1819 ŧ 0.3174*** 0.4050*** 0.3686*** (0.4543) (0.6001) (0.4951) (0.0805) (0.1206) (0.1346) (0.0595) (0.1122) (0.0875) Control Variables Release Year Fixed Effects Facility Fixed Effects N r2 1217 0.0187 X X X X X X 898 0.2444 1161 672 X X X X X X 510 642 1217 X X X X X X 898 1161 a. ŧp<.10, *p<.05, **p<.01,***p<.001 b. Clustered standard errors (at the facility level) are in parentheses. c. PIECP is an indicator variable equal to 1 for individuals that worked in the Prison Industry Enhancement Certification Program during confinement and 0 otherwise. The Reference group is comprised of individuals that either participated in a traditional prison industrial work program (TI) or did not participate in a work program during confinement (OTW). d. Control variables: indicator variables for the type of crime committed (person, property, drug, other), race (black), age at release, age at release squared, time served, time served squared, education, number of disciplinary reports, marital status, substance abuse history, quarters worked prior to incarceration, previous occupation, number of children, mental health status, gender, physical health status, number of previous incarcerations, and history of juvenile delinquency e. Observations vary due to missing values for some of the control variables, and due to 545 observations having a time from release to employment equal to zero. f. Cox Equation 5 & 6 assign a number arbitrarily close to zero, but not equal to zero, to the observations that have zero quarters of unemployment upon release from prison; thus the number of observations increase from Cox Equation 3 to Cox Equation 5 and from Cox Equation 4 to Cox Equation 6. This can be done when using the Cox model since estimation of the parameters relies on the ranking of the numbers. g. Previous occupation was not imputed so the maximum number of observations for the imputed model is 1161 not 1217. Table IV. Time from Release to Employment with Gender Interacted with PIECP Status OLS Variable Cox Proportional Hazard Model Imputed Imputed Imputed Equation 1 Equation 2 Equation 2 Equation 3 Equation 4 Equation 4 Equation 5 Equation 6 Equation 6 Male PIECP -1.4304** (0.4716) -1.2389* (0.5680) -1.0870* (0.4886) 0.139 ŧ (0.0954) 0.0651 (0.1925) 0.0966 (0.1776) 0.2629*** (0.0636) 0.2323* (0.1009) 0.2346** (0.0798) Female PIECP -2.2270** (0.8551) -4.5489 (7.5614) -3.5256 (7.1423) 0.2733* (0.1588) 0.7330 (1.6089) 0.7427 (1.5019) 0.4496*** (0.1245) 0.2182 (1.1200) 0.6247 (0.8503) Female non-PIECP 0.6892 (0.7220) -1.1791 (7.8208) -0.5025 (7.2083) 0.0268 (0.1126) 0.2781 (1.5804) 0.1557 (1.5077) -0.0092 (0.0684) -0.4839 (1.1345) -0.0498 (0.8527) X X X X X X X X X X X X X X X X X X X X X X X X 898 0.2713 1161 503 641 898 1159 Control Variables Female * Control Variables Release Year Dummies Facility Dummies N R-squared 1217 0.0213 672 1217 a. ŧp<.10, *p<.05, **p<.01,***p<.001 b. Clustered standard errors (at the facility level) are in parentheses. c. Male PIECP is an indicator variable equal to 1 for men that worked in the Prison Industry Enhancement Certification Program during confinement and 0 otherwise. Likewise Female PIECP is equal to 1 for women that worked in the Prison Industry Enhancement Certification Program and 0 otherwise. Female non-PIECP is equal to 1 for women that worked in either a traditional prison industrial work program (TI) or did not participate in a work program during confinement (OTW). The reference group is men that worked in either TI or OTW. d. Control variables: indicator variables for the type of crime committed (person, property, drug, other), race (black), age at release, age at release squared, time served, time served squared, education, number of disciplinary reports, marital status, substance abuse history, quarters worked prior to incarceration, previous occupation, number of children, mental health status, gender, physical health status, number of previous incarcerations, history of juvenile delinquency, facility dummies, and release year dummies. e. Observations vary due to missing values for some of the control variables, and because 545 observations have a time from release to employment equal to zero. f. Cox Equation 5 & 6 assign a number arbitrarily close to zero, but not equal to zero, to the observations that have zero quarters of unemployment upon release from prison; thus the number of observations increase from Cox Equation 3 to Cox Equation 5 and from Cox Equation 4 to Cox Equation 6. This can be done when using the Cox model since estimation of the parameters relies on the ranking of the numbers. g. Previous occupation was not imputed so the maximum number of observations for the imputed model is 1161 not 1217. Moreover, 2 facility dummies were dropped due to inadequate observations to calculate the standard errors resulting in 1159 observations instead of 1161. ! ! 60! Table V. Time from Employment to Job Loss OLS Variables PIECP Cox Proportional Hazard Model Imputed Imputed Equation 1 Equation 2 Equation 2 Equation 3 Equation 4 Equation 4 0.8866* (0.3888) Control Variables Release Year Fixed Effects Facility Fixed Effects N R-squared 1217 0.0068 0.7301 ŧ (0.5133) 0.4050 (0.4621) X X X X X X 898 0.2718 1161 -0.0449 (0.0659) 965 -0.0651 (0.1052) 0.0463 (0.0881) X X X X X X 708 917 a. ŧp<.10, *p<.05, **p<.01,***p<.001 b. Clustered standard errors (at the facility level) are in parentheses. c. PIECP is an indicator variable equal to 1 for individuals that worked in the Prison Industry Enhancement Certification Program during confinement and 0 otherwise. The Reference group is comprised of individuals that either participated in a traditional prison industrial work program (TI) or did not participate in a work program during confinement (OTW). d. Control variables: indicator variables for the type of crime committed (person, property, drug, other), race (black), age at release, age at release squared, time served, time served squared, education, number of disciplinary reports, marital status, substance abuse history, quarters worked prior to incarceration, previous occupation, number of children, mental health status, gender, physical health status, number of previous incarcerations, and history of juvenile delinquency. e. Observations vary due to missing values for some of the control variables f. 252 observations had no reported earnings during the follow up period. g. Previous occupation was not imputed so the maximum number of observations for the imputed model is 922 not 965. Moreover, 3 facility dummies were dropped due to inadequate observations to calculate the standard errors resulting in a maximum of 917 observations instead of 922 for the imputed analysis. ! ! 61! Table VI. Time from Employment to Job Loss with Gender Interacted with PIECP Status OLS Variables Cox Proportional Hazard Model Imputed Imputed Equation 1 Equation 2 Equation 2 Equation 3 Equation 4 Equation 4 Male PIECP 0.3798 (0.4519) 0.0945 (0.5469) -0.3439 (0.4562) 0.0410 (0.0726) 0.0640 (0.1321) 0.1760 ŧ (0.0962) Female PIECP 1.4578* (0.6522) -5.3845 (7.9599) -0.7614 (5.2757) -0.0585 (0.0924) -0.1504 (2.2660) 0.0959 (1.3108) Female non-PIECP -0.7165 (0.4492) -7.3059 (7.7094) -2.7624 (5.1230) 0.2054* (0.0840) 0.1437 (2.2311) 0.2865 (1.3225) X X X X X X X X X X X X X X X X 898 0.2910 1161 708 917 Control Variables Female * Control Variables Release Year Dummies Facility Dummies N R-squared 1217 0.0125 965 a. ŧp<.10, *p<.05, **p<.01,***p<.001 b. Clustered standard errors (at the facility level) are in parentheses. c. Male PIECP is an indicator variable equal to 1 for men that worked in the Prison Industry Enhancement Certification Program during confinement and 0 otherwise. Likewise Female PIECP is equal to 1 for women that worked in the Prison Industry Enhancement Certification Program and 0 otherwise. Female OTW or TI is equal to 1 for women that worked in either a traditional prison industrial work program (TI) or did not participate in a work program during confinement (OTW). The reference group is men that worked in either TI or OTW. d. Control variables: indicator variables for the type of crime committed (person, property, drug, other), race (black), age at release, age at release squared, time served, time served squared, education, number of disciplinary reports, marital status, substance abuse history, quarters worked prior to incarceration, previous occupation, number of children, mental health status, gender, physical health status, number of previous incarcerations, and history of juvenile delinquency. e. Observations vary due to missing values for some of the control variables f. 252 observations had no reported earnings during the follow up period. g. Previous occupation was not imputed so the maximum number of observations for the imputed model is 922 not 965. Moreover, 3 facility dummies were dropped due to inadequate observations to calculate the standard errors resulting in a maximum of 917 observations instead of 922 for the imputed analysis. ! ! 62! Table VII. OLS Regressions of Log Weekly Reported Earnings Variables PIECP Control Variables Female * Control Variables Release Year Dummies Facility Dummies N R-squared Imputed Equation 1 Equation 2 Equation 3 Equation 3 0.1943* 0.2067* 0.2007 ŧ 0.1595* (0.0875) (0.1095) (0.1108) (0.0877) X X X X X X X X X 925 0.2190 699 0.3877 699 0.4216 X X X X 883 a. ŧp<.10, *p<.05, **p<.01,***p<.001 b. Clustered standard errors (at the facility level) are in parentheses. c. PIECP is an indicator variable equal to 1 for individuals that worked in the Prison Industry Enhancement Certification Program during confinement and 0 otherwise. The Reference group is comprised of individuals that either participated in a traditional prison industrial work program (TI) or did not participate in a work program during confinement (OTW). d. Control variables: education, age at release, age at release squared, number of disciplinary reports, number of years served in prison, number of years served squared, black, number of previous incarcerations, history of a substance abuse, an indicator variable for having committed a property offense for the original offense, an indicator variable for having committed a drug offense for the original offense, an indicator variable for having committed an offense in the other category (all offenses not classified as property, drug, or an offense against a person), having a history of juvenile delinquency, having a history of a mental health issue, being single, number of quarters worked pre-incarceration, number of quarters worked pre-incarceration squared, number of children, and having a medical special need. e. 252 observations have no reported earnings, and are treated as unemployed (i.e., not included in the wage analysis), 34 observations have missing reported earnings, and an additional 42 observations are missing information on previous occupation. Moreover, 6 outliers (individuals with a log of weekly earnings less than 1) are dropped from the analysis. f. Previous occupation and the log of weekly earnings are not imputed so the maximum number of observations for the imputed analysis is 883. ! ! 63! Table VIII. Probability of Recidivism Estimates A. PIECP Sample Compared to National Estimates Time from Release 6 months 1 year 2 years 3 years Arrested PIECP Sample National 11% 30% 21% 44% 34% 59% 41% 68% Convicted PIECP Sample National 5% 11% 11% 22% 21% 36% 27% 47% Incarcerated PIECP Sample National 2% 5% 4% 10% 7% 19% 10% 25% Convicted Men Women 6% 5% 11% 11% 20% 22% 27% 27% Incarceration Men Women 1% 2% 3% 4% 7% 10% 8% 13% National Estimates from Langan and Levin (2002)! B. PIECP Sample by Gender Arrested Time from Release Men Women 6 months 12% 10% 1 year 23% 17% 2 years 35% 32% 3 years 42% 38% ! ! 64! Table IX. Complete Regressions with Gender Interaction Terms Release to Employment Employment to Loss (Cox Proportional Hazard Model) (Cox Proportional Hazard Model) Variables Human Capital Variables PIECP Coefficient Gender Interction Term Coefficient Gender Interction Term Log Weekly Earnings Coefficient Gender Interction Term 0.1480* (0.0877) Male PIECP 0.2346** (0.0798) 0.1760* (0.0962) Female PIECP 0.6247 (0.8503) 0.0959 (1.3108) Female OTW or TI -0.0498 (0.8527) 0.2865 (1.3225) Years of Education -0.0010 (0.0191) -0.0137 (0.0405) -0.0603* (0.0327) 0.0501 (0.0505) 0.0719*** (0.0227) -0.0323 (0.0429) 0.0349*** (0.0083) 0.0083 (0.0155) -0.0326** (0.0124) 0.0219 (0.0220) 0.0671** (0.0274) 0.0145 (0.0435) -0.0022ŧ (0.0017) -0.0002 (0.0022) Number of Quarters Worked Prior to Incarceration Number of Quarters Worked Prior to Incarceration^2 Previous Occupation:Office 0.0375 (0.3238) 0.4334 (0.3629) -0.0696 (0.2806) -0.3202 (0.4020) 0.2565 (0.2887) 0.2327 (0.3819) Previous Occupation: Unskill Labor/Assembly/ Warehouse/Trucker/Landscaper 0.2906 (0.2498) -0.5449 (1.0110) 0.0842 (0.1891) -0.2940 (0.2545) 0.1914 (0.2148) -0.1331 (0.8549) Previous Occupation: Self-Employed 0.0534 (0.2271) -0.1548 (0.2758) 0.5764** (0.2265) -0.3285 (0.2303) 0.0137 (0.2796) -0.0309 (0.2984) Previous Occupation: Skilled Labor/Contruction Trades/Welding 0.2645 (0.2349) 0.0207 (0.3083) -0.1131 (0.1955) -0.3031 (0.3402) 0.3542 (0.2371) -0.1166 (0.3783) Previous Occupation: Food 0.1171 (0.2520) 0.1105 (0.3138) -0.0283 (0.2184) -0.6151* (0.2570) 0.3005 (0.2048) 0.2640 (0.2715) 0.3051ŧ (0.2355) -0.1425 (0.2927) 0.4107 (0.4139) -1.1656* (0.5070) 0.0367 (0.5139) 0.9023 (0.5827) Mental Health Issue (Prison Records Indicated a Mental Health Problem) 0.0170 (0.0792) -0.0153 (0.1163) 0.3010** (0.1141) -0.1845 (0.1730) -0.3149** (0.1103) 0.1406 (0.1777) Medical Special Need (Prison Records Indicate Inmate Has a Physical Medical Special Need) -0.1610ŧ (0.1196) 0.1355 (0.2704) 0.1540 (0.1368) 0.1343 (0.2513) -0.0663 (0.1385) 0.0336 (0.4304) -0.0052 (0.0071) 0.0179ŧ (0.0097) 0.0016 (0.0088) 0.0091 (0.0143) -0.0038 (0.0055) -0.0031 (0.0103) Time Served (Years) 0.0216 (0.0323) -0.0467 (0.0414) -0.1637*** (0.0477) 0.0106 (0.0642) 0.1065*** (0.0304) 0.0667 (0.0410) Time Served (Years)^2 -0.0009 (0.0016) 0.0023 (0.0019) 0.0064*** (0.0019) -0.0017 (0.0023) -0.0036** (0.0013) -0.0021 (0.0017) Previous Occuapation: Retail Criminogenic Variables Number of Disciplinary Reports ! ! 65! Table IX. Complete Regressions with Gender Interaction Terms Continued Release to Employment Employment to Loss (Cox Proportional Hazard Model) (Cox Proportional Hazard Model) Variables Criminogenic Variables Continued Number of Previous Incarcerations Gender Interction Term Coefficient -0.0400ŧ Log Weekly Earnings Coefficient Gender Interction Term Coefficient Gender Interction Term 0.0024 (0.0184) (0.0215) 0.0400* (0.0184) -0.0010 (0.0228) -0.0806** (0.0283) 0.0106 (0.0306) 0.0480 (0.1433) -0.1557 (0.1974) -0.0831 (0.1347) 0.2657 (0.2962) 0.1752 (0.1113) -0.4118ŧ (0.3029) Offense Type: Property 0.1327 (0.0977) -0.0662 (0.1416) -0.0371 (0.1327) 0.1657 (0.2957) -0.0434 (0.0873) -0.1694 (0.2449) Offense Type: Drug 0.0301 (0.1118) -0.1261 (0.1464) 0.1176 (0.1394) -0.3243 (0.2318) -0.1443 (0.1100) 0.1694 (0.2198) Offense Type: Other -0.1009 (0.3075) -0.3787 (0.3499) 0.4912** (0.1903) 0.6192ŧ (0.3307) -0.4102 (0.3477) -0.5310 (0.8039) 0.0450 (0.0797) -0.0870 (0.2207) 0.2737** (0.1123) -0.0132 (0.1499) -0.0105 (0.0716) 0.0623 (0.1164) History of Substance Abuse History of Delinquency as a Juvenile Demographic Variables Female 1.1134 (1.6205) Black -0.0295 (0.0685) -0.3135* (0.1422) 0.0422 (0.0925) 0.0320 (0.1603) -0.0879 (0.1281) 0.1881 (0.1959) Age at Release 0.0061 (0.0335) 0.0241 (0.0477) -0.0334 (0.0476) -0.0546 (0.0668) 0.0518 (0.0599) -0.0304 (0.0806) Age at Release^2 -0.0003 (0.0004) -0.0001 (0.0006) 0.0004 (0.0006) 0.0005 (0.0009) -0.0006 (0.0008) 0.0006 (0.0011) Single -0.1247ŧ (0.0916) -0.1403 (0.1511) -0.0276 (0.1084) 0.1640 (0.1831) -0.0418 (0.0986) -0.3918* (0.1714) -0.0260 (0.0275) 0.0202 (0.0503) 0.0160 (0.0344) 0.0508 (0.0637) -0.0304 (0.0292) -0.0177 (0.0479) Number of Children Facility Dummies Release Year Dummies N X X X X X X 1159 917 883 a. ŧp<.10, *p<.05, **p<.01,***p<.001 b. Clustered standard errors (at the facility level) are in parentheses. c. Male PIECP is an indicator variable equal to 1 for men that worked in the Prison Industry Enhancement Certification Program during confinement and 0 otherwise. Likewise Female PIECP is equal to 1 for women that worked in the Prison Industry Enhancement Certification Program and 0 otherwise. Female OTW or TI is equal to 1 for women that worked in either a traditional prison industrial work program (TI) or did not participate in a work program during confinement (OTW). The reference group is men that that worked in either TI or OTW. ! ! 66! Appendix C: Figures Figure'1.'Selected'Demographics'of'Released'Offenders' 0.80$ 0.67$0.68$ 0.70$ 0.59$ 0.60$ 0.54$ 0.53$ 0.50$ 0.50$ 0.49$ 0.47$ 0.41$ 0.40$ NaConal$EsCmate$ 0.32$ 0.30$ 0.30$ 0.27$ 0.28$ 0.20$ 0.10$ PIECP$(Total$Sample)$ PIECP$(Complete$Cases)$ 0.16$ 0.10$ 0.10$0.10$ 0.08$ 0.00$ Female$ White$ Black$ Parents$ Physical$Health$ Problem$ Mental$Health$ Problem$ Source for National Statistics: Glaze & Maruschak (2008), BJS Prisoners in 1999 series, and Langan & Levin (2002) Figure'2.'Distribu.on'of'Offenses'Of'Released'Offenders'' 0.70% 0.59% 0.60% 0.50% 0.50% 0.45% 0.45% 0.44% 0.40% 0.34% Na>onal% 0.33% PIECP%(Total%Sample)% 0.28% 0.28% 0.30% PIECP%(Complete%Cases)% 0.25% 0.25% 0.23% 0.20% 0.11% 0.10% 0.02% 0.02% 0.00% Person% Property% Drug% Other% Prior%Prison% Source for National Statistics: Langan & Levin (2002) ! ! 67! Figure'3.'Criminal'History'Of'Released'Offenders' 10.0$ 8.8$ 9.0$ 8.0$ 7.0$ 6.5$ 6.4$ 6.0$ Na?onal$$ 5.0$ 4.4$ 4.3$ 4.4$ 3.8$ 4.0$ PIECP$(Total$Sample)$ 4.1$ PIECP$(Complete$Cases)$ 3.0$ 1.7$ 2.0$ 1.0$ 0.0$ Time$Served$ Mean$Prior$Arrest$ Mean$Prior$Convic?ons$ Source for National Statistics: Langan & Levin (2002) Figure'4.'Age'Distribu+on'of'Released'Offenders' 0.30% 0.24% 0.25% 0.20% 0.19% 0.22% 0.21% 0.20% 0.23% 0.19% 0.19% 0.18% 0.17% 0.17% 0.15%0.15% 0.15% 0.15% 0.14% Na2onal% PIECP%(Total%Sample)% 0.12% PIECP%(Complete%Cases)% 0.10% 0.06% 0.05% 0.05% 0.00% ≤24% 25.29% 30.34% 35.39% 40.44% ≥45% Source'for'Na+onal'Sta+s+cs:'Freeman'(2003)'' ! ! 68! Figure 5. Release to Employment 5.A. Kaplan-Meier Survival Estimates Time from Release to Employment 0.25 0.50 0.75 1.00 Survival Model 2 0.00 0.00 0.25 0.50 0.75 1.00 Survival Model 1 0 10 20 Number of Quarters Non-PIECP 30 0 PIECP 10 20 Number of Quarters Non-PIECP 30 PIECP 5.B. Kaplan-Meier Survival Estimates by Gender Time from Release to Employment 0.25 0.50 0.75 1.00 Survival Model 2 0.00 0.00 0.25 0.50 0.75 1.00 Survival Model 1 0 10 20 Number of Quarters 30 0 30 Non-PIECP Male Non-PIECP Female Non-PIECP Male Non-PIECP Female PIECP Male PIECP Female PIECP Male PIECP Female ! ! 10 20 Number of Quarters 69! Figure 6. Employment to Loss 6.A. Kaplan-Meier Survival Estimates 0.00 0.25 0.50 0.75 1.00 Time from Employment to Job Loss 0 10 20 30 Number of Quarters Non-PIECP PIECP 6.B. Kaplan-Meier Survival Estimates by Gender 0.00 0.25 0.50 0.75 1.00 Time from Employment to Job Loss 0 10 20 30 Number of Quarters Non-PIECP Male PIECP Male Non-PIECP Female PIECP Female ! ! 70! Figure 7. Kaplan-Meier Recidivism Survival Curves 7.A. Kaplan-Meier Survival Estimates Time from Release to Conviction 0.00 0.25 0.50 0.75 1.00 0.00 0.25 0.50 0.75 1.00 Time from Release to Arrest 0 500 1000 1500 2000 2500 0 500 Number of Days 1000 1500 2000 2500 Numer of Days Non-PIECP PIECP Non-PIECP PIECP 0.00 0.25 0.50 0.75 1.00 Time from Release to Incarceration 0 500 1000 1500 2000 2500 Number of Days Non-PIECP PIECP 7.B. Kaplan-Meier Survival Estimates by Gender 0.00 0.25 0.50 0.75 1.00 Time from Release to Conviction 0.00 0.25 0.50 0.75 1.00 Time from Release to Arrest 0 500 1000 1500 Number of Days 2000 2500 0 500 1000 1500 Number of Days 2000 2500 Non-PIECP Male Non-PIECP Female Non-PIECP Male Non-PIECP Female PIECP Male PIECP Female PIECP Male PIECP Female 0.00 0.25 0.50 0.75 1.00 Time from Release to Incarceration 0 500 1000 1500 Number of Days 2000 2500 Non-PIECP Male Non-PIECP Female PIECP Male PIECP Female ! ! 71! Figure 8. Kaplan-Meier Survival Curves Employment versus Incarceration Kaplan-Meier Survival Curves 0.25 0.50 0.75 1.00 Time from Release to Incarceration 0.00 0.00 0.25 0.50 0.75 1.00 Time from Employment to Job Loss 0 10 20 30 0 Quarters 20 30 Quarters ! ! 10 72!