Federal Reserve of Richmond Va Et Al Effect of Living Near Sex Offender on Real Estate
Download original document:
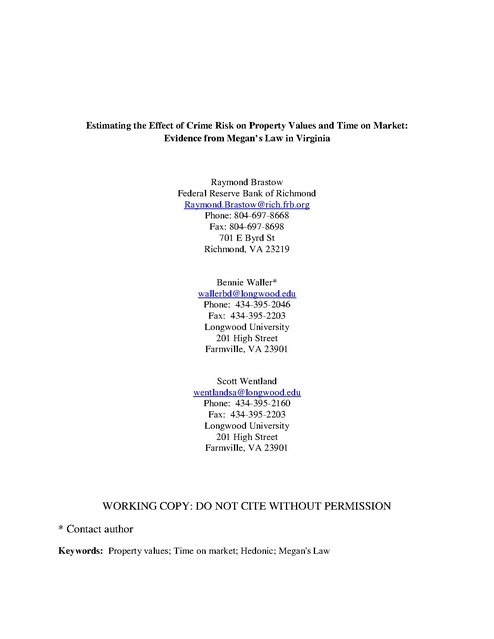
Document text
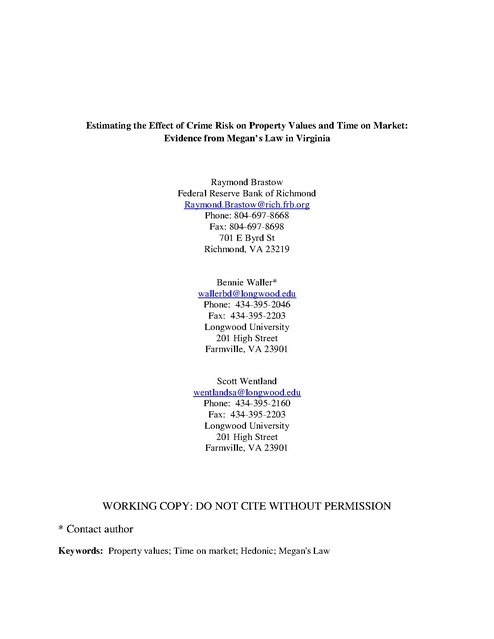
Document text
This text is machine-read, and may contain errors. Check the original document to verify accuracy.
Estimating the Effect of Crime Risk on Property Values and Time on Market: Evidence from Megan’s Law in Virginia Raymond Brastow Federal Reserve Bank of Richmond Raymond.Brastow@rich.frb.org Phone: 804-697-8668 Fax: 804-697-8698 701 E Byrd St Richmond, VA 23219 Bennie Waller* wallerbd@longwood.edu Phone: 434-395-2046 Fax: 434-395-2203 Longwood University 201 High Street Farmville, VA 23901 Scott Wentland wentlandsa@longwood.edu Phone: 434-395-2160 Fax: 434-395-2203 Longwood University 201 High Street Farmville, VA 23901 WORKING COPY: DO NOT CITE WITHOUT PERMISSION * Contact author Keywords: Property values; Time on market; Hedonic; Megan's Law 1 Abstract This paper explores the effect that living near a sex offender has on the marketability of one’s home. Specifically, we estimate the impact on a home’s sales price and the length of time it takes for the home to sell. Since the 1994 passage of Sexual Offender Act (known as Megan’s Law), persons convicted of sex crimes have been required to notify local law enforcement about their current domicile and any change of address. Since then, sex offenders’ residencies have become publicly available information allowing anyone to lookup whether a sex offender resides nearby. Using cross-sectional data from a central Virginia multiple listing service we find that sexual offenders have robust and economically large effects on nearby real estate. Our results indicate that the presence of a nearby registered sex offender reduces home values by approximately 9%. Moreover, these same homes take as much as 10% longer to sell than homes not located near registered sex offenders. These results prove robust over numerous specifications and modeling techniques commonly found in the literature. 2 Introduction The intent of this research is to examine the impact of Megan’s Law on the marketing duration and sales price of residential real estate as observed in rural areas of central Virginia. The empirical real estate literature has linked a host of housing, property, and surrounding area characteristics to sales price and marketing duration. However, relatively few have studied the effect of Megan’s Law on sales price and none have studied the Law’s effect on marketing duration. Additionally, previous studies have examined the effect of sex offenders in primarily urban settings. For example, Linden and Rockoff (2008) looked at data from Mecklenburg County, NC (a populous county containing the city of Charlotte), and Pope (2008) used data from Hillsborough County, FL (containing Tampa). This paper fills this gap in the literature by estimating the effect a nearby sex offender’s residence has on its surrounding real estate market in relatively rural areas of central Virginia. We find a substantial difference between our estimates of a sex offender’s impact in rural areas as compared to the estimates in studies of urban areas, suggesting a fundamental difference between rural and urban areas in values placed on crime risk imposed by nearby sex offenders. Literature Review While sex offender registries are nothing new, the widespread dissemination of such registries on the internet is a relatively recent phenomenon. Most states now provide detailed data about the locations, physical descriptions, and pictures of nearby sex offenders, even including details about the charges for which they have been convicted. Utilizing these new data sources, Pope (2008) and Linden and Rockoff (2008) both find that properties in close proximity to a property that is listed as the home address of a registered sex offender will suffer a loss in value. Both studies control for a variety of individual home characteristics as well as area fixed effects to control for heterogeneity in their respective real estate markets. Indeed, the loss to property value that a registered sex offender brings dissipates as distance from the sexual offenders address increases. However, each study uses sales and crime data from only a single county (Hillsborough, FL and Mecklenberg, NC, respectively). 3 While the aforementioned studies analyze home values, they omit analyses of the home’s marketing duration. Yet, marketing duration of a property has been examined and studied from numerous perspectives in prior real estate literature. Belkin, Hempel and McLeavey (1976) were one of the first to put forth the theory that list price, and changes to list price, directly impact the property’s time on market. They also site market imperfections, such as inadequate communication of price changes, that may impact time on market of properties. Miller (1978) finds a positive relationship between time on market and list price but also notes that a longer marketing duration does not necessarily result in a higher sales price. Haurin (1988) has been well cited in the marketing duration literature for his claim that the more atypical a property the longer it will remain on the market. Specifically, he cites “unusual location” as an example of an atypical characteristic that may negatively affect a property’s marketing duration. Turnbull and Dombrow (2007) present evidence that properties located near other properties listed by the same agent are able to bring a higher sales price. The authors also find that the greater the diversity of listings by an agent, the longer their listings stay on the market. Yang and Yavas (1995) suggest that higher commission rates for agents do not impact the time on market. However, they do suggest that a higher commission rate may signal that the property is more costly to sell because of its location. They specifically cite the example of a rural property being more expensive to show than a property in the city. Our study incorporates methodologies found in these articles regarding property values and marketing duration and applies them to the analysis of the effect of sexual offenders on real estate marketability. Data The data for this research are from several sources. Information about sexual offenders is contained in the Virginia Sex Offender and Crimes Against Minors Registry and is available on a public web site maintained by the Commonwealth of Virginia. 1 Each observation contains the registered sex offender’s current and prior addresses, along with a number of other personal characteristics (e.g. age, sex, race, and description of the perpetrated crime). Data on real estate transactions consist of 1 See the following website: http://sex-offender.vsp.virginia.gov/sor/ 4 observations of residential properties on the market between July 1999 and June 2009 and comes from a multiple listing service located in central Virginia. The initial housing data consists of 21,453 observations. After culling for incomplete, missing or illogical data that suggest data entry errors, the final data set consists of 13,172 sold properties. The data collected from the MLS include typical property characteristics (square footage, bedrooms and baths), and market and calendar information (location, list dates, length of listing contract). Methodology We are interested in the impact registered sex offenders have on real estate prices and the time it takes to sell a given home (i.e. marketing duration). After obtaining the longitude and latitude of the registered sex offenders’ addresses, we use the great-circle distance formula to calculate the distance from a registered sex offender’s home to a given house on the market. 𝐷𝑖𝑠𝑡𝑎𝑛𝑐𝑒 = 69.1 ∗ 180 𝜋 ∗ arccos [sin 𝐿𝐴𝑇1 ∗ sin 𝐿𝐴𝑇2 + cos(𝐿𝐴𝑇1 ) ∗ cos 𝐿𝐴𝑇2 ∗ cos (𝐿𝑂𝑁2 − 𝐿𝑂𝑁1 ) (1) Following Waller, Brastow, and Johnson (2009), the present study remains agnostic with respect to the model specification debate within the literature. Indeed, we employ all three of the most common methodologies (described below) in the related real estate literature in order to determine the robustness of empirical results. Like Linden and Rockoff (2008) and Pope (2008), we estimate the effect a sex offender has on surrounding real estate sale prices (within certain radii) for properties located across the state of Virginia. One key contribution of this paper, however, is to answer an entirely new question: do homes near registered sex offenders also take longer to sell? To that end, the following general modeling framework is presented: TOMi ( X i , Li , Z i , SOi , SPi ) (2) 5 SPˆi ( xi , li , zi , SOi ) (3) Where: TOM i and SPi are vectors for property marketing time and property selling price respectively (expressed in natural log form), X i and xi are vectors of property characteristics and other control variables, Li and li are vectors for location control, Z i and zi are vectors that include variables such as the degree of over pricing and market condition, and SOi , the variable of interest, equals 1 if a registered sex offender (contemporaneously) lives near homei,2 0 otherwise. We also estimate the sheer distance between home i and the nearest registered sex offender as a continuous variable. We report OLS coefficient estimates of time on market and sales price [Equations (2) and (3)] and Weibull estimates for time on market. A third model captures potential endogeneity between sales price and marketing time. In this model, SPi is estimated in reduced form in Equation (3) for a three stage least squares (3SLS) specification, with predicted values, SPˆi , substituting for SPi in Equation (2). In a 3SLS setting, (2) and (3) form the system of equations between property price and property marketing time. In addition, 3SLS incorporates an additional step with seemingly unrelated regression (SUR) 2 As we will explain in the next section, “nearby” can mean different distances to different people. Below, we explore a number of distances (or, more specifically, radii) from the nearest sex offender (for example, .1 mile, .25 mile, .5 mile, and 1 mile). 6 estimation.3 Variables included in the equations above (vectors Xi, Li, and Zi) follow existing literature and will be discussed in greater detail in the next section. Results We find that if there is a sex offender registered at a nearby residence, then nearby homes sell for less and that those homes take longer to sell. However, the magnitudes are striking. Tables 2 (first column), 3, and 4 show that a nearby sex offender (i.e., one who resides within one-tenth mile) reduces property values by approximately 9% and increases the marketing duration of a house by approximately 10%. These are economically meaningful effects and demonstrate that central Virginia residents assign a large risk to living near a convicted sex offender. To determine sensitivity and robustness of the results, we estimated a number of model specifications. Prior studies have classified “nearby” registered sex offender in different ways. Table 2 shows the effects of using different radii for measuring the effect a sex offender has on property values, holding a number of property characteristics constant. 4 Pope (2008) uses a dummy variable equal to 1 if a sex offender resides within one-tenth mile (0.1 miles) of a property and another second dummy variable for two-tenths of a mile (0.2 miles). Linden and Rockoff (2008) also use the 0.1 mile dummy, but differ in their additional use of a 0.3 mile dummy variable. The first column of Table 2 shows perhaps the most striking result of the paper: a registered sex offender living within .1 mile of one’s home will reduce the value of surrounding properties sold by about 9% (or, more precisely, 8.8%). This is more than twice the magnitude of similar estimates reported in Pope (2008) and Linden and Rockoff (2008), suggesting a 3 According to Belsley (1988), 3SLS has an edge on 2SLS in estimating systems of equations because it is more efficient, particularly when there are strong interrelations among error terms. 4 Like any multivariate analysis, we attempt to isolate the effect a sex offender has by controlling for numerous property characteristics that also affect our dependant variable (like square footage, age, number of houses on the market, whether it is vacant, number of bedrooms, bathrooms, whether it has a pool, brick exterior, hardwood floors, walk-in closet, finished basement, gas fireplace, paved driveway, fenced yard, and the acreage of the property). In addition, we control for the year and what time of year it was sold in (i.e. the season), the unemployment rate in Virginia, whether it is a townhouse or condo, and area fixed effects (in most cases, city/town). Most of these control variables are commonly used within the real estate literature. Hence, we limit our discussion in this paper primarily to the variable of interest: proximity to a registered sexual offender. 7 much higher willingness to pay to avoid crime risk in predominantly rural central Virginia. For the average homeowner, this works out to be $14,826. To put this in relative terms, homeowners value avoiding this crime risk more than they value a pool, a brick exterior, a walk in closet, a fireplace, a paved driveway, or an additional bathroom. More precisely, homeowners value avoiding this risk as much as 295 square feet in their home (i.e. homeowners might be willing to live in a significantly smaller home if they could avoid living so close to a sex offender). As the radius for the nearest sex offender widens, Table 2 also shows that this effect is still present, albeit diminished. A nearby sex offender lowers property values 8.5%, 6.6%, and 4.6% when you classify “nearby” as residing within 0.25, 0.5, and 1 mile respectively. This result is also somewhat unique, given that prior studies have not found this effect on property values beyond 0.3 miles, suggesting that there is a significant difference between the perception of “neighbor” in rural and urban areas. Residences tend to be less densely located in more rural areas like central Virginia than in urban areas like Charlotte and Tampa. Hence, rural residents may simply perceive homes within a larger radius as neighbors, resulting in a greater alertness to crime risk over larger distances. Some real estate studies (e.g. Rutherford, Springer, and Yavas (2005)) have chosen a Heckman selection model (Heckman, 1979) to correct for sample selection bias. There is good reason to suspect that our dependant variable, sales price, is only observed for a restricted, non-random sample. Quite simply, a house only has a selling price if it is actually sold. Hence, OLS estimates could be biased because a number of houses listed on the market are not sold (e.g. perhaps they are less likely to be sold because a registered sex offender lives nearby, which would bias the estimates of the houses that actually sell). Table 3 shows the coefficient estimates for the Heckman model, where the inverse Mills ratio corrects for selectivity bias by adjusting the conditional error terms such that they have a zero mean (generated from a probit model, where the binary dependent variable is whether the property has actually 8 sold). Thus, taking into account potential sample selection bias, nearby sex offenders still impose about an 8% discount on surrounding properties.5 This general result remains robust even when we change the relevant area fixed effects. Linden and Rockoff (2008), for example, use dummy variables for neighborhoods (probably the most relevant area variable in urban settings), and other studies use similar measures to hold constant one of the most important pillars of real estate value: location. Studies incorporate area fixed effects in an attempt to control for unobserved heterogeneity across these areas so that the explanatory variables’ effects are identified from variation within a given area (or even in a given year, as is the case for year fixed effects). For most of our analysis up to this point, we have used cities/towns in which these properties reside. In central Virginia and possibly in other predominantly rural areas, the relevant area metric tends to be wider than in urban settings. But, perhaps this is too wide and there may still substantial heterogeneity within these areas. Table 3 shows that when we use zip codes or elementary school districts as the relevant area metric, we continue to find a substantial discount for homes located near a registered sex offender. While sellers tend to sell their properties at substantially lower values when a registered sex offender lives nearby, they may not be lowering their sales price enough. Sellers and their agents may have difficulty estimating a property’s expected value if a sex offender is near. That is, a reduced offer price may not attract enough potential buyers, resulting in a longer marketing duration of the home. Table 4 shows that homes located near (within .1 mile of) a registered sex offender spend abut 10% more time on the market. This works out to be about 13 days longer on the market than other similar properties, which are also competitively priced. In relative terms, this is roughly equivalent to selling your home in the “off” season of fall or winter (as compared to the summer or spring). However, as is indicated in the methodology section, a number of studies in the real estate literature employ other econometric models to analyze time on market. Given the extensive debate within 5 The fact that “lambda” is statistically significant indicates selection bias. Though, the differences between the OLS and Heckman estimates do not appear economically significant, suggesting the selection bias is present but not large. 9 the literature, our paper intends to remain neutral by employing three common techniques for modeling time on market: Heckman, Weibull, and three-stage least squares. Moreover, this neutrality comes with an additional benefit of demonstrating that our general results are not sensitive to the modeling technique, confirming the robustness6 of our findings. Table 4 shows that all techniques are generally consistent with the OLS finding that registered sex offenders increase marketing duration of nearby properties in central Virginia (ranging from approximately 6% to 10%). Concluding Remarks This study finds that residents in central Virginia home sellers must absorb a relatively large risk premium when selling property near a registered sex offender. Alternatively, home buyers are willing to pay a premium to live in safer areas. The qualitative result is not surprising and is entirely consistent with previous findings in similar studies. However, the quantitative result reveals an economically large difference between the risk premium associated with sex offenders in a rural area like central Virginia versus urban areas like Charlotte and Tampa. Moreover, our analysis of marketing duration suggests that homes located near registered sex offenders take longer to sell, signifying a general reluctance to purchase properties exposed to such risks. Certainly, no one wants to live near a registered sex offender, but empirical results indicate that the more tightly knit communities of central Virginia are willing to pay more to avoid such a risk. This study’s results are consistent with the notion that residents in rural areas consider larger areas when defining who constitutes as a “neighbor” and assessing subsequent risks. The results may also reflect 1) a greater aversion to crime, 2) more households with families compared to more densely populated urban areas, or some combination of factors that contribute to this rather striking magnitude. Further research may shine light on the differences between rural and urban areas and perhaps the sources of such differences. 6 The final version of this working paper will include additional qualitative robustness checks that are not present in the present version. 10 Exhibit 1: Variable legend Variable Definition Tom Lprice lnlp Sprice Comm NoMkt Age Sqft Bedrooms Fullbath Halfbath Garage Fire Brick Vinyl Hardwood Ceramic Fullbase Vacant Area 1 Area 2 Area 3 Area 4 Area 5 Area 6 Listtime Frmsd Winter Spring Summer Fall Time on market measured from date of original listing to contract date Listing price ln(listing price) Sales price Commission rate Property sold in less than 4 days. Age of property Square footage of property Number of bedrooms Number of full bathrooms Number of half bathrooms Dummy variable, 1 if property has a garage, 0 otherwise Dummy variable, 1 if property has a fireplace, 0 otherwise Dummy variable, 1 if property has brick exterior, 0 otherwise Dummy variable, 1 if property has vinyl floors, 0 otherwise Dummy variable, 1 if property has hardwood floors, 0 otherwise Dummy variable, 1 if property has ceramic flooring, 0 otherwise Dummy variable, 1 if property has full basement, 0 otherwise Dummy variable, 1 if property was vacant when listed Dummy variable, 1 if property located in area 1, 0 otherwise Dummy variable, 1 if property located in area 2, 0 otherwise Dummy variable, 1 if property located in area 3, 0 otherwise Dummy variable, 1 if property located in area 4, 0 otherwise Dummy variable, 1 if property located in area 5, 0 otherwise Dummy variable, 1 if property located in area 6, 0 otherwise Chronological time variable 30 year fixed rate mortgage at property contract date Dummy variable, 1 if property was listed in winter, 0 otherwise Dummy variable, 1 if property was listed in spring, 0 otherwise Dummy variable, 1 if property was listed in summer, 0 otherwise Dummy variable, 1 if property was listed in fall, 0 otherwise 11 Variable Table 1a: Housing Descriptive Statistics Mean Std. Dev. Min Max TOM Lprice Sprice Compensation Nomkt Age Sqft Bedrooms Fullbath Halfbath Garage Fire Brick Vinylsiding Hardwood Ceramictile Fullbase Vacant Area 1 Area 2 Area 3 Area 4 Area 5 Area 6 Listtime Frmsd Winter Spring Summer Fall 108.9579 172831.1 167533 2.868985 .0107402 26.46229 1927.133 3.194715 2.001468 .4180189 .3778396 .6709937 .5409519 .5027816 .5475197 .2424664 .5745634 .3290063 .0582599 .1646577 .0295163 .4817648 .0030134 .0511513 24.79818 6.132768 .2638696 .3001854 .2481069 .187838 963 6190000 2650000 7.5 1 470 8578 8 6 4 1 1 1 1 1 1 1 1 1 1 1 1 1 1 41 8.64 1 1 1 1 88.23393 127118.8 103914.4 .3641925 .103081 28.50594 796.0701 .7806939 .6926693 .5318478 .484866 .4698704 .4983394 .5000116 .497756 .4285915 .4944281 .4698704 .2342434 .3708857 .1692551 .4996867 .0548142 .2203147 8.319312 .4866796 .4407464 .4583562 .4319309 .3905979 0 8500 6800 0 0 0 417 1 1 0 0 0 0 0 0 0 0 0 0 0 0 0 0 0 2 4.81 0 0 0 0 Table 1b: Sex Offender Descriptive Statistics Variable Obs Mean Std. Dev. Min Max Male Black White Age Violent crime 0 0 0 16 0 1 1 1 93 1 8986 8986 8986 8986 8986 .977298 .3281772 .6486757 44.48453 .8188293 .14896 .4695758 .4774106 13.00889 .385181 12 Table 2 The effect of a Nearby Registered Sex Offender on the Selling Price of a Home (1) - OLS Independent Variables so_tenthmile so_quartermile so_halfmile so_onemile sqft lnage onmkt vacant bedrooms baths pool onestory brick hardwood walkincloset finbase fireplacegas paveddrive fencedyard condo townhouse fall winter spring acreage vaunemp constant Area Fixed Effects Year Fixed Effects Observations R-squared (2) - OLS Coefficient t-stat -0.0885 -8.27 0.0003 -0.1099 0.0000 -0.0823 -0.0250 0.0782 0.0615 0.0184 0.0651 0.1058 0.0251 -0.0669 0.0772 0.0629 0.0063 -0.0468 -0.1465 -0.0041 -0.0227 -0.0067 0.0072 -0.0814 11.9153 46.99 -57.59 0.86 -16.16 -4.8 13.98 11.23 3.68 14.27 20.29 4.77 -12.53 16.77 13.36 1.07 -2.75 -19.66 -0.61 -3.25 -1.09 6.97 -5.62 120.3 (3) - OLS Coefficient t-stat -0.0856 -14.7 0.0003 -0.1070 0.0000 -0.0801 -0.0245 0.0756 0.0560 0.0163 0.0626 0.1076 0.0243 -0.0685 0.0757 0.0613 0.0098 -0.0544 -0.1444 -0.0040 -0.0234 -0.0070 0.0072 -0.0825 11.9253 48.05 -55.9 0.79 -15.7 -4.75 13.69 10.25 3.27 13.83 20.73 4.65 -12.9 16.52 13.11 1.68 -3.22 -19.4 -0.61 -3.36 -1.17 7.01 -5.73 121.2 (4) - OLS Coefficient t-stat -0.0665 -14.7 0.0003 -0.1070 0.0000 -0.0814 -0.0241 0.0761 0.0556 0.0164 0.0643 0.1077 0.0245 -0.0659 0.0761 0.0638 0.0087 -0.0458 -0.1372 -0.0034 -0.0224 -0.0057 0.0072 -0.0832 11.9329 13172 13172 13172 0.7496 0.7520 0.7513 *T-statistics in all of the above regressions are robust 47.39 -55.4 0.79 -16.0 -4.66 13.73 10.18 3.29 14.16 20.71 4.68 -12.4 16.6 13.62 1.48 -2.7 -18.4 -0.52 -3.22 -0.94 7.02 -5.75 120.4 Coefficient t-stat -0.0462 -9.26 0.0003 -0.1095 0.0000 -0.0836 -0.0249 0.0785 0.0578 0.0187 0.0658 0.1053 0.0242 -0.0647 0.0752 0.0673 0.0076 -0.0445 -0.1422 -0.0042 -0.0231 -0.0066 0.0072 -0.084 11.9437 46.93 -56.7 0.9 -16.3 -4.79 14.11 10.51 3.74 14.41 20.18 4.61 -12.1 16.36 14.28 1.28 -2.64 -19 -0.64 -3.31 -1.09 7.01 -5.77 120.1 13172 0.7495 13 Table 3 The effect of a Nearby Registered Sex Offender on the Sales Price of a Home (1) - OLS Independent Variables so_tenthmile sqft lnage onmkt vacant bedrooms baths pool onestory brick hardwood walkincloset finbase fireplacegas paveddrive fencedyard condo townhouse fall winter spring acreage vaunemp constant lambda (IMR) City/town Fixed Effects Zip Code Fixed Effects Elementary School Fixed Effects Year Fixed Effects Observations R-squared (2) - Heckman (3) - OLS (4) - OLS Coefficient t-stat Coefficient* z-stat Coefficient t-stat Coefficient t-stat -0.0885 -8.27 -0.0806 -5.61 -0.0615 -5.87 -0.0444 -4.09 0.0003 -0.1099 0.0000 -0.0823 -0.0250 0.0782 0.0615 0.0184 0.0651 0.1058 0.0251 -0.0669 0.0772 0.0629 0.0063 -0.0468 -0.1465 -0.0041 -0.0227 -0.0067 0.0072 -0.0814 11.9153 46.99 -57.59 0.86 -16.16 -4.8 13.98 11.23 3.68 14.27 20.29 4.77 -12.53 16.77 13.36 1.07 -2.75 -19.66 -0.61 -3.25 -1.09 6.97 -5.62 120.3 0.0003 -0.1000 0.0000 -0.0827 -0.0181 0.0722 0.0580 0.0091 0.0559 0.0996 0.0297 -0.0505 0.0691 0.0587 0.0054 -0.0282 -0.1391 -0.0080 -0.0182 -0.0053 0.0050 -0.0761 12.274 .8158 15.34 -19.21 -0.56 -2.09 -2.36 6.99 4.2 3.48 7.48 8.89 1.57 -0.89 6.72 8.85 0.74 -3.84 -4.23 -0.52 -1.12 -0.39 13.2 -6.58 51.68 12.03 0.0003 -0.1117 0.0000 -0.0769 -0.0142 0.0666 0.0585 0.0237 0.0620 0.0934 0.0263 -0.0706 0.0717 0.0596 0.0055 -0.0715 -0.1301 0.0004 -0.0212 -0.0080 0.0075 -0.0727 11.813 49.77 -60.26 0.81 -15.96 -2.92 12.79 11.2 4.92 14.45 18.72 5.21 -13.92 16.46 13.48 0.97 -4.53 -17.9 0.07 -3.19 -1.38 6.97 -5.23 111.25 0.0003 -0.1034 0.0000 -0.0740 -0.0149 0.0659 0.0503 0.0241 0.0606 0.1068 0.0242 -0.0714 0.0687 0.0570 0.0106 -0.0643 -0.1394 -0.0031 -0.0222 -0.0079 0.0073 -0.0750 11.77 47.19 -52.27 0.81 -15.23 -3.01 12.50 9.33 4.99 13.61 20.92 4.83 -13.92 15.71 12.68 1.88 -3.93 -18.95 -0.49 -3.35 -1.37 7.08 -5.48 113.84 13172 21340 13172 13172 0.7496 n/a 0.7718 0.7746 *Heckman Coefficients (dy/dx) are calculated using the mfx compute, pred(ycond) postestimation command T-statistics in all of the above regressions are robust 14 Table 4 The effect of a Nearby Registered Sex Offender on the Marketing Duration (or, Time on Market) of a Home (1) - OLS Independent Variables so_tenthmile lnlp sqft lnage onmkt vacant bedrooms baths pool onestory brick hardwood walkincloset finbase fireplacegas paveddrive fencedyard condo townhouse fall winter spring acreage vaunemp constant lambda Area Fixed Effects Year Fixed Effects Observations Chi-squared (2) - Heckman (3) - Weibull (4) – 3SLS Coefficient t-stat Coefficient* z-stat Coefficient + z-stat Coefficient t-stat 0.1043 0.1486 0.0001 -0.0457 0.0002 0.1148 0.0094 -0.0435 -0.0237 -0.0058 -0.0594 -0.0043 0.0126 -0.1260 -0.0214 -0.0477 0.0099 0.2338 0.1760 0.1078 0.0926 -0.0073 0.0009 -0.1111 2.878 4.58 7.8 5.77 -8.22 2.35 9.23 0.96 -3.66 -1.51 -0.47 -4.83 -0.35 0.88 -8.98 -1.68 -3.92 0.67 5.88 6.72 6.05 4.7 -0.47 2.91 -3.43 9.03 0.0601 0.0871 0.0001 -0.0712 0.0001 0.1591 -0.0028 -0.0212 0.0084 -0.0015 -0.0412 0.0030 0.0360 -0.0989 -0.0031 -0.0273 0.0252 0.1207 0.1538 0.1218 0.0898 0.0272 0.0016 0.0361 3.46 3.87 4.23 -6.69 1.54 5.36 0.27 -2.62 -0.67 -1.9 -3.9 -1.72 1.66 -5.76 -1.9 -3.47 0.83 3.7 4.27 5.88 4.08 1.48 1.29 2.82 0.3511 -.8254 0.34 -2.99 0.0785 0.1415 0.0001 -0.0522 0.0002 0.0831 0.0050 -0.0397 -0.0276 -0.0209 -0.0579 0.0049 -0.0287 -0.1452 -0.0591 -0.0362 -0.0155 0.2429 0.2179 0.0776 0.0292 -0.0574 0.0005 -0.1761 1.3383 3.78 9.15 7.54 -12.55 4.83 8.18 0.61 -3.99 -2.08 -2.05 -5.67 0.47 -2.51 -11.94 -5.41 -3.43 -1.19 7.11 11.11 5.7 1.91 -4.52 1.76 -6.98 24.51 0.0761 0.1405 0.0000 -0.0663 0.0001 0.1609 0.0006 -0.0256 0.0039 -0.0052 -0.0451 -0.0050 0.0363 -0.0980 -0.0087 -0.0351 0.0239 0.1420 0.1549 0.1167 0.0892 0.0257 0.0011 0.0291 2.409 2.50 5.12 2.52 -10.36 1.47 10.76 0.05 -1.71 0.21 -0.34 -3.02 -0.32 2.06 -5.58 -0.54 -2.32 1.28 2.83 5.72 5.64 4.06 1.39 1.87 0.76 5.73 21340 21340 21340 13172 n/a 2881.89 3321.35 1356.23 *Heckman Coefficients (dy/dx) are calculated using the mfx compute, pred(ycond) postestimation command +Weibull Coefficients (dy/dx) are elasticities evaluated at their respective sample medians 15 16 References Belsley, David A., Two- or Three-stage Least Squares? Computational Economics. 1988, 1, 2130. Belkin, J., D. J. Hempel and D. W. McLeavey, An Empirical Study of Time on Market Using Multidimensional Segmentation of Housing Markets, Journal of the American Real Estate and Urban Economics Association, 1976, 4, 57-75 Haurin, D., The Duration of Marketing Time on Residential Housing, AREUEA Journal 1988, 16(4): 396-410. Heckman, J., Sample Selection Bias as a Specification Error, Econometrica, 1979, 47 (Feb.): 153-61 Jud, Donald G., Terry G. Seaks, and Daniel T. Winkler, Time on the Market: The Impact of Residential Brokerage, The Journal of Real Estate Research 1996, 12(3): 447-458. Knight, John R., Listing Price, Time on Market and Ultimate Selling Price: Causes and Effects of Listing Price Changes, Real Estate Economics 2002, 30(2): 213-237. Linden, L. and J.E. Rockoff, Estimates of the Impact of Crime Risk on Property Values from Megan’s Laws, American Economic Review, 2008, 98:3, 1103-1127. Miller, N. G., Time on the Market and Selling Price, AREUEA Journal, 1978, 6(2): 164-174. Pope, J.C., Fear of crime and housing prices: Household reactions to sex offender registries, Journal of Urban Economics, 2008, 64, 601-614. Rutherford, R.C., T.M. Springer, and A. Yavas. Conflicts Between Principals and Agents: Evidence from Residential Brokerage. Journal of Financial Economics, 2005, 76:3, 627 - 65. Turnbull, G. K., and J. Dombrow. Individual Agents, Firms, and the Real Estate Brokerage Process. Journal of Real Estate Finance and Economics, 2007, 35:1, 57-76. Yavas, A. and S. Yang. The Strategic Role of Listing Price in Marketing Real Estate: Theory and Evidence. Real Estate Economics, 1995, 23:3, 347 - 68.