Steward Employ Stats Estimating Recidivism Risk in Earnings Loss Calculations for Ex Prisoners 2010
Download original document:
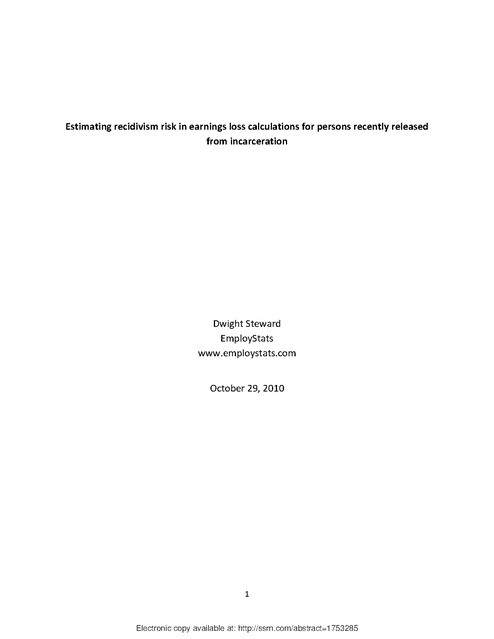
Document text
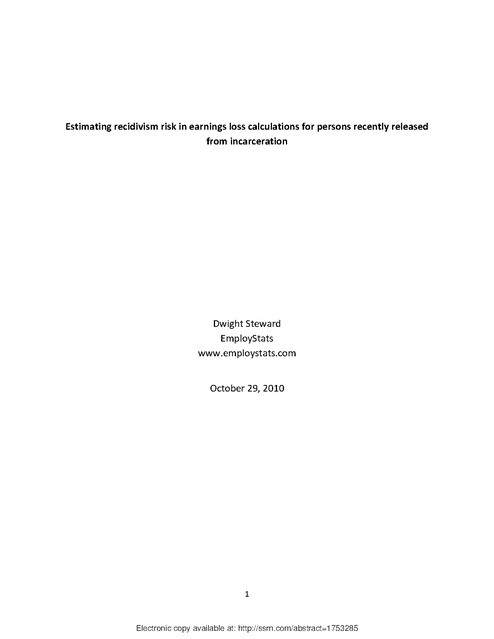
Document text
This text is machine-read, and may contain errors. Check the original document to verify accuracy.
Estimating recidivism risk in earnings loss calculations for persons recently released from incarceration Dwight Steward EmployStats www.employstats.com October 29, 2010 1 Electronic copy available at: http://ssrn.com/abstract=1753285 Introduction The risk of re‐incarceration is a complicating factor in evaluations involving earnings losses for persons who are recently released from incarceration. Criminologists and economists have shown that factors such as the ex‐inmate’s age, education, and the type of crime committed are correlated with the risk of criminal recidivism and re‐incarceration. Some studies have shown that in some groups, over 50 percent of ex‐inmates are re‐incarcerated within three years of being released (Langen and Levin (2002)). This paper provides an estimate of the re‐incarceration risk for individuals who are recently released from jail or prison. The estimates of the year‐by‐year re‐incarceration risk can be used in a standard labor force participation economic damage model to calculate earnings losses for persons who have been recently released from incarceration. Using person‐level data from the Bureau of Justice Statistics (BJS) to estimate a Cox Proportional Hazards model that accounts for factors such as race, gender, age at release, and type of crime committed, I find that in any given year within the first six years of release, the recidivism risk probability varies between 2.5 to 10.9 percent. Review of recidivism literature Collectively, recidivism studies have identified a number of factors related to the timing and likelihood that a person will be re‐incarcerated. These factors include demographics, criminal history, length of sentence received, and the activities that the individual undertook while incarcerated. In this section, I provide a brief overview of the criminal recidivism literature. Age, gender, and race of the ex‐inmate are several factors that criminologists have identified as correlated with the likelihood of criminal recidivism. Studies such as Laub and Sampson (2001), Spivak and Sharp (2007), and Bierens and Carvalho (2006) suggest that as ex‐inmates age, they become more mature and stable and may be less likely to become a recidivist. Some empirical data and research suggest that female ex‐inmates are less likely to be re‐incarcerated and some racial groups are more likely than others to be re‐incarcerated following their release from prison or jail (Spivak and Sharp (2007), Alaska Judicial Council (2007), and Texas Legislative Budget Board (2009), Mbuba (2004), Kyung Yon Jhi and Hee‐Jong Joo (2009), Bierens and Carvalho (2007)). In addition to demographic factors, some criminologists and economists suggest that the physical body type of an individual may be correlated with the propensity for criminal activity. Researchers posit that the ability to carry out certain crimes, such as burglary, robbery, and drug dealing, is correlated with physical traits, such as weight‐height proportionality and muscularity. Sheldon (1949), compared the body types, or somatotypes, of a sample of criminal offenders and college students. He found that relative to college students, criminal offenders tended to have more muscular and athletic, or what Sheldon (1949) referred to as mesomorphic type, bodies. Hooten (1969) found that criminals tended to be smaller in height and weight, and recidivism decreased as both height and weight increased. Bodenhorn, Moehling and Price (2009) find a similar negative correlation between crime rates and body weight in data on inmates incarcerated in Tennessee and Illinois state penitentiaries in the 19th century. 2 Electronic copy available at: http://ssrn.com/abstract=1753285 Maddan, Walker, and Miller (2008) re‐investigate the relationship between an inmate’s somatotype, which they measured using the inmate’s body mass index (BMI), and the propensity for criminality using demographic data for a sample of Arkansas prisoners. The researcher’s BMI‐derived somatotypes measures support the contention that inmates with more muscular body types are more likely to be incarcerated for more violent crimes. Criminologists and economists also posit that recidivism rates are correlated with substance abuse, criminal history, and the type of adjudication received by the offender. Empirical studies, such as Alaska Judicial Council (2007) and Wexler, Melnick, Lowe, and Peters (1999), suggest that individuals who have a history of drug and alcohol abuse, especially those who are untreated for their disease, are more likely to become re‐incarcerated. The individual’s pre‐incarceration criminal history, such as arrests and other run‐ins with law enforcement and the type of crime committed have also been shown to be correlated with the likelihood of re‐incarceration (Langen and Levin (2002)). In addition to pre‐incarceration criminal activity, economic reasoning suggests that the length of sentence received by the individual could be correlated to the likelihood of re‐incarceration. Holding other factors constant, such as the individual’s age at release, it is possible that a person who receives a longer sentence will be sufficiently deterred from committing future crimes that would lead to re‐ incarceration (Bierens and Carvalho (2007)). Conversely, research suggest longer sentences and serving more incarcerated time may result in the further development of an individual’s criminal network and contacts that may lead to future crimes (Kyung Yon Jhi and Hee‐Jong Joo (2009)). Finally, the activities and programs that the inmate participated in, as well as the support system developed while incarcerated has also been shown to be correlated with recidivism. Localized studies of jail facility training programs provide some evidence that inmates who complete job training, educational programs, and substance abuse treatment while incarcerated have lower recidivism rates than inmates that do not undertake these programs (Smith and Bechtel (2005), Texas Legislative Budget Board (2009) and Kyung Yon Jhi and Hee‐Jong Joo (2009)). Kyung Yon Jhi and Hee‐Jong Joo (2009) show that inmates who are not enrolled in educational and training programs and are involved in gangs and have behavioral and conduct problems while incarcerated are more likely to become recidivists. . Estimation of reincarceration risk Estimating the probability that a person with a recent incarceration history will be re‐incarcerated is the focus of this paper. Similar to Langen and Levin (2002), Kyung Yon Jhi and Hee‐Jong Joo (2009), and other studies of criminal recidivism, I estimate a Cox proportional hazards model that provides the probability that a person who was previously incarcerated, and has been released for a certain amount of time (t years), will be returned to jail or prison. The instantaneous hazard rate is written as follows: (1) ∆ | The Cox proportional hazards model incorporates a time‐dependent baseline hazard rate time‐independent explanatory variables X . The model is written as follows: 3 and (2) , ∑ where is the baseline hazard, e is the exponential function, is the the explanatory variable. model coefficient and X is Data The Cox proportional hazards model of recidivism described above is estimated using Bureau of Justice Statistics (BJS) release records for a sample of prisoners. The inmate release record data is obtained from a 2001‐2 BJS study of the recidivism rates of persons who were released in 1994. In the study, the BJS obtained 302,309 release records from 15 state Departments of Corrections. The 15 state Departments of Corrections were selected based on their willingness to participate, prison population, and participation in an earlier study conducted by the BJS. The participating state Department of Corrections supplied FBI and state criminal history files that tracked the released inmates from the date of the inmates release through the year 2001. Using the criminal history files, the BJS drew a representative sample of 38,624 released prisoners from the release records supplied by the 15 states. Table 1 presents summary statistics for the inmate release data used in my analysis. As the table shows, the typical inmate is male (94.0%) with an average age of 33.5 years of age. As is consistent with other studies of incarceration, African‐Americans (44.0%) and Hispanics (14.0%) are over‐represented in the inmate population relative to the general population, and women (6.0%) are underrepresented. The table also presents statistics describing the BMI, or height and weight proportionality index, of the inmate sample. The BMI of the inmates is measured at the beginning of their incarceration. Generally, a BMI that exceeds a certain level suggests that the person is overweight while a BMI that falls under a certain level suggests that the person is underweight. According to the Center for Disease Control and Prevention (CDC), the average BMI is 26.6 for the U.S. general population. 1 The average inmate BMI measure of 24.41 suggests that inmates in the sample fall into the ‘normal’ height‐weight proportional range. 2 The average BMI for the inmates in the sample suggests that the inmates tend to be more height and weight proportionate than the general population when admitted to jail or prison. 3 The largest portion of individuals in the data is incarcerated for a violent crime (48.0%) and served an average sentence length of 5.73 years. The inmates in the sample served approximately 37.85% (not shown in Table 1) of their jail or prison sentence. The vast majority of released inmates had been arrested prior to their 1994 incarceration (88.0%), and about one‐third of the individuals had been previously incarcerated. Approximately 20.0% of the released inmates were certified by their respective Department of Corrections as a drug abusers while 16.0% were classified as alcohol abusers.. Less than 10% of the individuals completed an educational or vocational training program while incarcerated. The majority of 1 See: Center for Disease Control and Prevention (CDC), http://www.cdc.gov/healthyweight/assessing/bmi/adult_bmi/index.html See: Center for Disease Control and Prevention (CDC), http://www.cdc.gov/healthyweight/assessing/bmi/adult_bmi/index.html 3 See: Center for Disease Control and Prevention (CDC), http http://www.cdc.gov/nchs/data/nhanes/databriefs/adultweight.pdf 2 4 individuals (67.0%) were released to a state outside of their birth state following their incarceration stint. Findings In this section, I discuss my findings and present recidivism probabilities for different inmate sub groups. The Cox proportional hazards recidivism model coefficients are shown in Table 2. In the table, Models I through IV represent different variable specifications of the recidivism models. The relative likelihood associated with each variable can be found by exponentiation of the coefficients shown in Table 2. The proportional hazards assumption for the models is tested using visual examinations of the Kaplan‐ Meir curves and statistical tests of the model’s Schoenfeld and scaled Schoenfeld residuals. With the exception of the indicator variable for race, there is no evidence of a significant violation of the proportional hazards assumption. To determine the potential impact of the violation of the proportional hazards assumption for the race variable, a separate set of Cox models that were stratified by race was estimated. The variable specification in the race‐stratified Cox models mirrored the variable specifications in the non‐race stratified models. The variable coefficients and standard errors obtained from the stratified approach were consistent with the variable coefficients and standard errors obtained from the non‐stratified approach shown in Table 2. 4 Since the results were consistent using the two approaches, the practical impact of the proportionality violation is in all likelihood small. The recidivism models suggest that there are a number of factors that need to be considered when estimating the earnings loss for individuals who are recently released from incarceration. First, the recidivism models suggest that gender and race are factors that are significantly correlated with the likelihood of recidivism. While women are significantly less likely to be re‐incarcerated, African‐ American and Hispanic inmates are more likely to be re‐incarcerated after being released from jail or prison. Although the results are significant and consistent across the models, the correlation between race and recidivism should be interpreted with caution since the BJS data does contain complete socio‐ economic information, such as income and education, for the inmate or the inmate’s family. It is possible that the race variable in the BJS data is simply a de‐facto proxy for differences in the socio‐ economic status of the inmates in the sample. Second, the analyses suggest that the type of crime committed is related to recidivism. Individuals who were convicted of property, drug, and public order crimes were more likely than persons who committed violent crimes to be re‐incarcerated. As has been found in other studies (Bierens and Carvalho (2007) and Kyung Yon Jhi and Hee‐Jong Joo (2009)), individuals who committed property crimes, such as burglary and public order crimes, which include those related to weapons, prostitution, and probation violators, were the most likely offenders to return to incarceration. This finding is also consistent with the proposition that some violent crimes are ‘crimes of passion’ that are not as subject 4 The coefficients and standard errors for the stratified model are available upon request. 5 to repetition. The expected sentence length for violent crimes may also serve as a separate deterrent to potential recidivism. Third, my results indicate that the likelihood of recidivism is correlated with an individual’s criminal history. Individuals who had a criminal history of arrests were approximately 1.8 times more likely to be re‐incarcerated while those who had been incarcerated previously were approximately 1.3 times more likely to become recidivist. Individuals who committed rules infractions while incarcerated were slightly more likely to become recidivist following release from jail or prison. Fourth, the recidivism models indicate that individuals, who were incarcerated in their home state and were released to their home state, were significantly more likely to return to incarceration. Similarly, persons, who served time in U.S. facilities but were born outside of the U.S., were less likely to return to prison following their release. Both of these findings suggest that serving time away from the inmate’s home state may assist in breaking up of negative relationships, networks, and connections that could lead to recidivism. Nonetheless, these findings should be viewed with some caution because the data does not capture potential post‐release incarcerations committed outside the 15 states in the data or in jurisdictions outside of the U.S. Fifth, participation in jail or prison facility educational programs is significantly correlated to lower recidivism rates while participation in in‐house substance abuse programs is not. Individuals who completed educational and vocational programs while incarcerated were over a quarter less likely than those who did not complete such programs to become a recidivist. Likewise, participation in a substance abuse treatment program is negatively correlated with recidivism, but the correlation is not statistically significant. These findings are consistent across all four of the model specifications. Sixth, similar to the studies mentioned previously, I find that the inmate’s body type is correlated with the likelihood of recidivism. Individuals who were overweight or obese relative to their heights, i.e. inmates who had higher body mass indexes (BMI), were less likely to become a recidivist than those who were in the normal BMI range. For instance, an individual who was obese at the beginning of their incarceration is about one‐tenth less likely than a person in the normal weight‐height range to be re‐ incarcerated following their release. Individuals who were underweight at admission tended to have lower recidivism rates, but this finding is not statistically significant. Seventh, the recidivism models indicate that older individuals are significantly less likely to be re‐ incarcerated. A person who was age 45 or older when released from incarceration is about half as less likely as a person who was age 30 to 34 to return to jail or prison. In contrast, a person who was between the ages of 18 and 24 at release is nearly one‐third more likely than a person who was age 30 to 34 at release to be re‐incarcerated. Eighth, my results suggest that the length of the inmate’s sentence does not matter in the recidivism calculus, but the actual amount of time served does. As shown in Table 2, the coefficients on the length of sentence are generally statistically insignificant in all four models. In contrast, the coefficients on the time served variables are statistically significant and indicate that the actual time served by the inmate is 6 negatively related to recidivism. For instance, individuals who served more than 61 months incarcerated were one‐fourth to one‐fifth less likely than a person who served 19 to 24 months (the comparison group) to be re‐incarcerated following their release from jail or prison. Individuals, who served very short terms, less than six months, were significantly more likely to be re‐incarcerated following their release. The estimates of the year‐by‐year re‐incarceration risk derived from the recidivism models described above can be used in a standard labor force participation economic damage model to calculate the expected earnings for persons who have been recently released from incarceration. Case specific estimates can be derived by evaluating the Cox model in equation (2) using the appropriate values for the independent explanatory variables X . The baseline hazard , which is not specified can be 5 estimated using kernel techniques. Table 3 presents recidivism hazard rates for ex‐inmate sub‐groupings, including age at release, type of crime committed, and race for up to six years following the individual’s release from incarceration. The table presents the year‐by‐year recidivism risk calculated for different sub‐groupings using equation (2) evaluated at the mean values of the other variables in the equation. Generally, the risk of recidivism increases each year until approximately the fifth year following the inmate’s release. Beginning in the fifth post‐incarceration year, the risk of recidivism declines in the last two years in which the ex‐inmate was tracked. For instance, as shown in the table, a person who was aged 25 to 29 at the time of their release, has a 0.05 chance of being re‐incarcerated in the first year following their incarceration. The risk of recidivism for ex‐inmates in this age group increases each year until year five, in which the risk of recidivism probability reaches 0.10, and then decreases in the last two years that the person is tracked in the data. Care should be exercised when interpreting and using the recidivism probabilities for the last year. It is not clear from the data that the BJS actually tracked all of the inmates throughout the entire last year of the study. Therefore, while it is possible that due to the factors discussed above, recidivism risk does in fact decrease significantly in the last year, it is not possible to rule out the possibility that this finding is in part an artifact of the BJS data. Conclusions In sum, this paper provides an estimate of the re‐incarceration risk for individuals who are recently released from jail or prison. The estimates of the year‐by‐year re‐incarceration risk in this paper can be used in a standard labor force participation economic damage model to calculate earnings losses for individuals. I find that in any given year within the first six years of release, the recidivism risk for typical ex‐inmate varies between a 2.5 to 10.9 percent. In my study I use person‐level data from the Bureau of Justice Statistics (BJS) to estimate a Cox Proportional Hazards model that accounts for factors such as race, gender, age at release, and type of crime committed. 5 See for example, Stata, Survival Analysis and Epidemiological Tables, Reference Manual Release 11, p. 231-240 7 8 Table 1: Variable Summary Statistics Variable African‐American Female Hispanic Body Mass Index (BMI) at intake Mean Min Max 0.44 0.06 0.14 0 0 0 1 1 1 BMI =wgt (lbs.) * 703/hgt²(in.) 24.41 13.19 90.22 Age (Yrs) at release 18‐24 yrs old at release 25‐29 yrs old at release 30‐34 yrs old at release 35‐39 yrs old at release 40‐44 yrs old at release >45 yrs old at release 33.47 0.19 0.21 0.22 0.17 0.1 0.11 14.5 0 0 0 0 0 0 87.96 1 1 1 1 1 1 Born outside of U.S. Inmate released in home state 0.06 0.33 0 0 1 1 Violent crime committed Property crime committed Drugs crime committed Public order crime committed 0.48 0.22 0.2 0.1 0 0 0 0 1 1 1 1 Length of sentence received (Yrs) Life sentence received 0‐3 yrs sentence received 3‐5 yrs sentence received 5‐7 yr sentence received 7‐10 yr sentence received 10‐15 yr sentence received 15‐20 yr sentence received 20‐30 yr sentence received 30‐Life sentence received 5.73 0.09 0.34 0.24 0.11 0.1 0.06 0.03 0.02 0.01 0 0 0 0 0 0 0 0 0 0 183 1 1 1 1 1 1 1 1 1 Pre‐incarceration arrest record Pre‐incarceration prison record 0.88 0.34 0 0 1 1 1‐6 months served incarcerated 7‐12 months served incarcerated 0.23 0.2 0 0 1 1 6 6 See Center for Diease Control and Prevention (CDC), http://www.cdc.gov/healthyweight/assessing/bmi/adult_bmi/index.html. 9 13‐18 months served incarcerated 19‐24 months served incarcerated 25‐30 months served incarcerated 31‐36 months served incarcerated 37‐60 months served incarcerated >61 months served incarcerated 0.14 0.18 0.07 0.06 0.12 0.1 0 0 0 0 0 0 1 1 1 1 1 1 Had rules infractions as inmate Drug abuser prior to incarceration Alcohol abuser prior to incarceration Alcohol treatment program completion Educational program completion Vocational program completion 0.24 0.2 0.16 0.01 0.06 0.04 0 0 0 0 0 0 1 1 1 1 1 1 10 Table 2: Cox Recidivism Models Coefficient Variable African‐American inmate Female inmate Hispanic inmate 14‐17 yrs old at release 18‐24 yrs old at release 25‐29 yrs old at release 35‐39 yrs old at release 40‐44 yrs old at release >45 yrs old at release Property Drugs Public Order Underweight1 Overweight Obese Born outside of U.S. Inmate released in home state Life Sentence Model I 0.2708*** (0.0266) ‐0.1372** (0.0481) 0.0647 (0.0407) 0.3649* (0.1574) 0.2452*** (0.0358) 0.1241*** (0.0345) ‐0.0831* (0.0382) ‐0.2969*** (0.0475) ‐0.7028*** (0.0567) 0.4274*** (0.0319) 0.2286*** (0.0334) 0.4023*** (0.0404) ‐0.0013 (0.0695) ‐0.0661* (0.0280) ‐0.1045* (0.0420) ‐0.2287*** (0.0586) 0.2254*** (0.0270) 0.3600*** (0.0434) Model II Model III Model IV 0.2559*** (0.0263) ‐0.0754 (0.0478) 0.0591 (0.0407) 0.2506*** (0.0263) ‐0.0994* (0.0476) 0.0758 (0.0406) 0.2591*** (0.0266) ‐0.1253** (0.0478) 0.0822* (0.0407) 0.0065 (0.0694) ‐0.0695* (0.0280) ‐0.1136** (0.0419) ‐0.2559*** (0.0584) 0.2266*** (0.0269) 0.2640*** (0.0426) 11 0.4161*** (0.0310) 0.2144*** (0.0325) 0.3678*** (0.0394) 0.0049 ‐0.0061 (0.0694) (0.0694) ‐0.0738** ‐0.0762** (0.0280) (0.0280) ‐0.1138** ‐0.1105** (0.0419) (0.0420) ‐0.2758*** ‐0.2562*** (0.0583) (0.0587) 0.2368*** 0.2758*** (0.0268) (0.0268) 0‐3 yrs Sentence 5‐7 yr Sentence 7‐10 yr Sentence 10‐15 yr Sentence 15‐20 yr Sentence 20‐30 yr Sentence 30‐Life Sentence Prior arrest record Prior prison record 1‐6 months served 7‐12 months served 13‐18 months served 25‐30 months served 31‐36 months served 37‐60 months served >61 months served Had infractions as inmate Drug abuser Alcohol abuser Alcohol treatment Education program Vocational program ‐0.0032 (0.0338) 0.0111 (0.0471) ‐0.1186* (0.0494) ‐0.0814 (0.0656) ‐0.0837 (0.0872) ‐0.3077** (0.1167) ‐0.3045 (0.1720) 0.5670*** (0.0548) 0.2810*** (0.0256) 0.1165** (0.0415) 0.0728 (0.0424) ‐0.0864 (0.0477) ‐0.0603 (0.0614) ‐0.2569*** (0.0712) ‐0.1638** (0.0553) ‐0.1980** (0.0689) 0.0769* (0.0354) 0.0994 (0.0532) 0.1331** (0.0515) 0.1087 (0.5808) ‐0.3206*** (0.0630) ‐0.2140** ‐0.0070 (0.0337) ‐0.0346 (0.0470) ‐0.1847*** (0.0491) ‐0.1644* (0.0653) ‐0.1770* (0.0870) ‐0.3543** (0.1168) ‐0.3766* (0.1717) 0.6506*** (0.0543) 0.3366*** (0.0253) 0.1261** (0.0414) 0.0626 (0.0423) ‐0.1304** (0.0474) ‐0.1074 (0.0612) ‐0.3292*** (0.0709) ‐0.2417*** (0.0548) ‐0.2960*** (0.0684) 0.0941** (0.0352) 0.1216* (0.0531) 0.1234* (0.0517) 0.0988 (0.5804) ‐0.3484*** (0.0630) ‐0.2288** 12 0.6541*** (0.0543) 0.3399*** (0.0252) 0.2082*** (0.0390) 0.0982* (0.0419) ‐0.1171* (0.0475) ‐0.0830 (0.0611) ‐0.3048*** (0.0700) ‐0.2172*** (0.0537) ‐0.2004** (0.0671) 0.0839* (0.0349) 0.1091* (0.0525) 0.0939 (0.0510) 0.1849 (0.5800) ‐0.3404*** (0.0629) ‐0.2065** 0.6066*** (0.0545) 0.3062*** (0.0259) ‐0.0091 (0.0341) 0.0701 (0.0523) 0.1430** (0.0502) 0.3103 (0.5802) ‐0.3568*** (0.0628) ‐0.2266** (0.0801) Age at release (0.0800) ‐0.0306*** (0.0015) Imprisonment sentence (yrs) Time served (0.0800) ‐0.0291*** (0.0015) ‐0.0234*** (0.0028) (0.0799) ‐0.0289*** (0.0015) ‐0.0295*** (0.0027) ‐0.0006 (0.0006) chi2 2408.744 2228.626 2206.963 2258.924 N 20,733 20,733 20,733 20,733 *, **, and *** denotes p‐value<0.05, p‐value<0.01, and p‐value<0.001 respectively 1. The variables underweight, overweight, and obese are constructed from the inmate’s BMI index at the time of admission to jail or prison. Underweight, overweight, and obese, is defined as a BMI less than 18.5, 24.9 to 29.9, and greater than 29.9, respectively. Source: Center for Diease Control and Prevention (CDC), http://www.cdc.gov/healthyweight/assessing/bmi/adult_bmi/index.html 13 Table 3: Recidivism Probabilities by ex‐Inmate Sub‐grouping Gender Time Male Race Female White Black In Facility Training Hispanic Vocational Educational None <1 year 0.047105 0.041067 0.041613 0.054555 0.044394 0.038757 0.034837 0.048003 1 to 2 years 0.064917 0.056596 0.057348 0.075184 0.061181 0.053413 0.04801 0.066154 2 to 3 years 0.069066 0.060212 0.061013 0.079988 0.065091 0.056826 0.051078 0.070382 3 to 4 years 0.085031 0.074132 0.075117 0.098478 0.080138 0.069962 0.062886 0.086652 4 to 5 years 0.094651 0.082519 0.083616 0.109618 0.089204 0.077877 0.070001 0.096455 5 to 6 years 0.082695 0.072095 0.073053 0.095772 0.077936 0.06804 0.061158 0.084271 0.02469 0.025018 0.032799 0.02669 0.023301 0.020944 0.02886 6 to 7 years 0.02832 Time Property Crime Committed Public Drugs Order Violent <1 year 0.065676 0.056029 0.066948 0.039219 1 to 2 years 0.09051 0.077215 0.092263 0.05405 2 to 3 years 0.096293 0.082149 0.098158 0.057504 3 to 4 years 0.118552 0.101139 0.120848 0.070797 4 to 5 years 0.131963 0.11258 0.134519 0.078807 5 to 6 years 0.115294 0.09836 0.117527 0.068852 6 to 7 years 0.039485 0.033685 0.04025 0.023579 Time <1 year Age at Release 14‐17 Yrs 18‐24 Yrs 25‐29 Yrs 30‐34 Yrs 35‐39 Yrs >45 Yrs 0.067149 0.057078 0.051534 0.049488 0.04358 0.035836 0.025127 1 to 2 years 0.09254 0.078661 0.071021 0.068201 0.060059 0.049387 0.034628 2 to 3 years 0.098453 0.083687 0.075559 0.072559 0.063897 0.052543 0.036841 3 to 4 years 0.12121 0.103032 0.093025 0.089332 0.078668 0.06469 0.045358 4 to 5 years 0.134922 0.114688 0.103549 0.099438 0.087568 0.072009 0.05049 5 to 6 years 0.117879 0.100201 0.090469 0.086877 0.076506 0.062913 0.044112 6 to 7 years 0.04037 0.034316 0.030983 0.029752 0.0262 0.021545 0.015106 14 40‐44 yrs References Bierens, Herman, and Jose Carvalho. "Semi‐Nonparametric Competing Risks Analysis of Recidivism." Journal of Applied Econometrics 22, no. 5 (2007): 971‐993. Bodenhorn, Howard, Carolyn Moehling, and Gregory Price. "nals: Stature and Crime in Early America." NBER Working paper Series w15945 (2010). http://papers.ssrn.com/sol3/papers.cfm?abstract_id=1598061 (accessed October 28, 2010). Connolly, Michele, and Val Shepperd. "Statewide Criminal Justice Recidivism and Revocation Rates." Legislative Budget Board 1 (2009). http://www.lbb.state.tx.us/PubSafety_CrimJustice/3_Reports/Recidivism_Report_2009.pdf (accessed October 28, 2010). "Criminal Recidivism in Alaska." Alaska Judicial Council, January 1, 2007. http://www.ajc.state.ak.us/reports/1‐07CriminalRecidivism.pdf (accessed October 28, 2010). Hooten, Earnest. The American Criminal: An Anthropological Study. 1939. Reprint, Santa Barbara: Greenwood Press, 1969. Langan, Patrick, and David Levin. "Recidivism of Prisoners Released in 1994. ." United States Department of Justice ‐ (2002). http://www.ojp.usdoj.gov/bjs/pub/pdf/rpr94.pdf (accessed October 28, 2010). Laub, John, and Robert Sampson. "Understanding Desistance from Crime." Crime and Justice 28 (2001): 1‐69. Maddan, Sean, Jeffery Walker, and J. Mitchell Miller. "Does size really matter? A reexamination of Sheldon's somatotypes and criminal behavior." The Social Science Journal 45, no. 2 (2008): 330‐ 344. Mbuba, Jospeter. Juvenile Recidivism: An Analysis of Race and Other Socio‐Demographic Predictors 15 within Three Intervention Modalities in the State of Louisiana. baton rouge: louisiana state university, 2004. Sheldon, William. Varieties of Delinquent Youth: An Introduction to Constitutional Psychiatry. New York: Harper And Brothers Publishers, 1949. Smith, Cindy, Jennifer Bechtel, Angie Patrick, Richard Smith, and Laura Wilson‐Gentry. "Correctional Industries Preparing Inmates for Re‐entry: Recidivism & Post‐release Employment." U.S. Department of Justice ‐ (2006). http://www.ncjrs.gov/pdffiles1/nij/grants/214608.pdf (accessed October 28, 2010). Spivak, Andrew, and Susan Sharp. "Inmate Recidivism as a Measure of Private Prison Performance." Crime and Delinquency 54, no. 3 (2008): 482‐508. Wexler, Harry, Gerald Melnick, Lois Lowe, and Jean Peters. "3 year Reincarceration Outcomes for Amity In‐Prison Therapeutic Community and Aftercare in California." The Prison Journal 79, no. 3 (1999): 321‐336. Yon Jhi, Kyung, and Hee‐Jong Joo. "Predictors of Recidivism among Major Age Groups of Parolees." Justice Policy Journal 6, no. 1 (2009). http://www.cjcj.org/files/predictors_of.pdf (accessed October 28, 2010). 16