To Treat or Not To Treat: Evidence on the Prospects of Expanding Treatment to Drug-Involved Offenders, Urban Institute, 2008
Download original document:
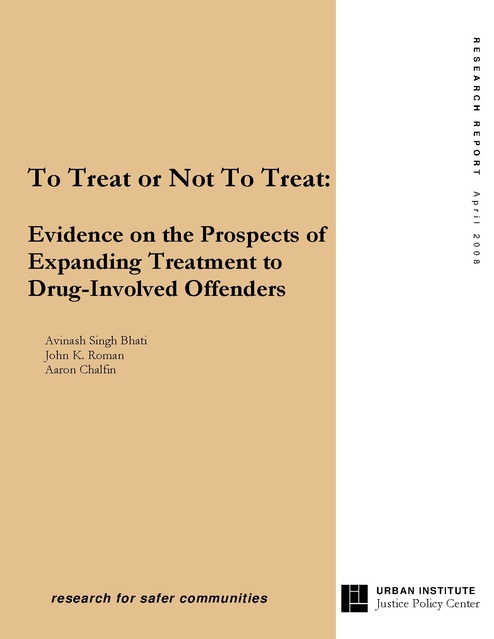
Document text
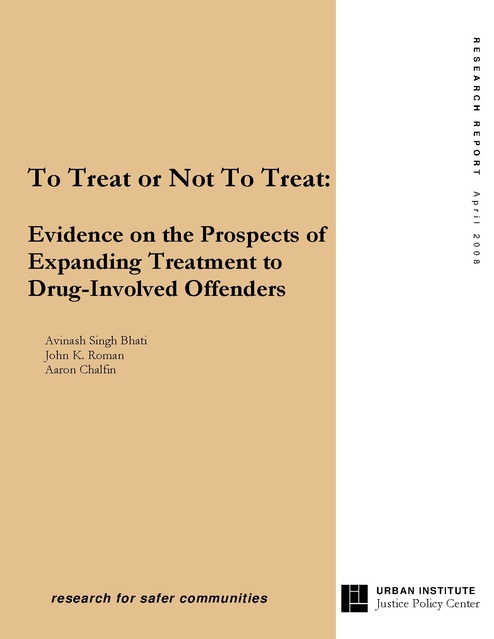
Document text
This text is machine-read, and may contain errors. Check the original document to verify accuracy.
R E S E A R C H A p r i l 2 0 0 8 Evidence on the Prospects of Expanding Treatment to Drug-Involved Offenders R E P O R T To Treat or Not To Treat: Avinash Singh Bhati John K. Roman Aaron Chalfin research for safer communities Going to Scale in the Treatment of Drug-Involved Criminal Offenders URBAN INSTITUTE Justice Policy Center Copyright © April 2008 This document was prepared under grant # 2005-DC-BX-1064 awarded by the National Institute of Justice, U.S. Department of Justice. Points of view in this document are those of the authors and do not necessarily represent the official position or policies of the U.S. Department of Justice or of other staff members, officers, trustees, advisor groups, or funders of the Urban Institute. URBAN INSTITUTE Justice Policy Center 2100 M STREET, NW WASHINGTON, DC 20037 www.urban.org The views expressed are those of the authors, and should not be attributed to The Urban Institute, its trustees, or its funders. TO TREAT OR NOT TO TREAT: EVIDENCE ON THE PROSPECTS OF EXPANDING TREATMENT TO DRUG-INVOLVED OFFENDERS Avinash Singh Bhati John K. Roman Aaron Chalfin Justice Policy Center, The Urban Institute 2100 M Street, N.W., Washington, D.C. 20037 April 2008 Contents Acknowledgments ix Abstract xi Executive Summary 1 2 xiii Background and Motivation 1.1 Introduction . . . . . . . . . . . . . . . . . . . . . . . . . . . . . . . . . . 1.2 Prior Research . . . . . . . . . . . . . . . . . . . . . . . . . . . . . . . . . 1.2.1 Drug-Crime Nexus . . . . . . . . . . . . . . . . . . . . . . . . 1.2.2 Criminal Justice System Interventions with Drug-Involved Offenders . . . . . . . . . . . . . . . . . . . . . . . . . . . . . . . 1.2.3 Therapeutic Jurisprudence and Drug Courts . . . . . . . . The Research Design 2.1 Synthetic Data . . . . . . . . . . . . . . . . . . . . . . . . . . . . . . 2.2 Data Sources and Uses . . . . . . . . . . . . . . . . . . . . . . . . . 2.2.1 Arrestee Drug Abuse Monitoring (ADAM) . . . . . . . 2.2.2 National Survey on Drug Use and Health (NSDUH) 2.2.3 Drug Abuse Treatment Outcome Study (DATOS) . . 2.2.4 Summary of the Elements of the Synthetic Data Set . i . . . . . . . . . . . . 1 1 4 4 6 7 9 11 13 13 16 17 18 ii 3 Estimation Strategy 3.1 A Profile’s Prevalence in the Population of Arrestees . . . . . . . . 3.2 Simulated Counterfactual . . . . . . . . . . . . . . . . . . . . . . . . . 3.3 Simulated Eligibility . . . . . . . . . . . . . . . . . . . . . . . . . . . . . 3.3.1 Drug Court Survey Data Describing Eligibility Criteria 3.3.2 Estimating the Size of the Drug-Court Eligible Population 3.3.3 Estimating the Number of Individuals Currently Receiving Drug Treatment . . . . . . . . . . . . . . . . . . . . . . . . 3.4 Cost and Benefit Estimates . . . . . . . . . . . . . . . . . . . . . . . . . 3.4.1 Treatment Price Estimates . . . . . . . . . . . . . . . . . . . . 3.4.2 Drug Court Price Estimates . . . . . . . . . . . . . . . . . . . 3.4.3 Estimates of the Benefits from Crime Reduction . . . . . 3.5 Key Assumptions and Caveats . . . . . . . . . . . . . . . . . . . . . . 21 21 22 26 28 30 33 34 36 37 39 44 4 Findings 47 4.1 Prevalence . . . . . . . . . . . . . . . . . . . . . . . . . . . . . . . . . . . 47 4.2 Crimes Avertable by Treatment . . . . . . . . . . . . . . . . . . . . . 48 4.3 Cost-Effectiveness of Treating Currently Eligible Drug Court Participants . . . . . . . . . . . . . . . . . . . . . . . . . . . . . . . . . . 52 4.4 Simulated Cost-Effectiveness of Expanding Criminal Justice System– Based Treatment . . . . . . . . . . . . . . . . . . . . . . . . . . . . . . . 58 4.4.1 Simulation 1: Adding Individuals with Other Pending Charges . . . . . . . . . . . . . . . . . . . . . . . . . . . . . . . . 58 4.4.2 Simulation 2: Adding Individuals with Prior Violence . . 60 4.4.3 Simulation 3: Adding Individuals with Prior Failed Treatment . . . . . . . . . . . . . . . . . . . . . . . . . . . . . . . . . . 62 4.4.4 Simulation 4: Adding Individuals with Co-Occurring Alcohol Problems . . . . . . . . . . . . . . . . . . . . . . . . . 64 4.4.5 Simulation 5: Expanding Eligibility to Include all Arrestees 66 5 Discussion 69 iii 6 References A Mathematical Appendix A.1 Interpolation Technique . . . A.2 Prevalence Estimation . . . . . A.3 Computing Variance . . . . . . A.4 Empirical Similarity Weights 73 . . . . . . . . . . . . . . . . . . . . . . . . . . . . . . . . . . . . . . . . . . . . . . . . . . . . . . . . . . . . . . . . . . . . . . . . . . . . . . . . . . . . . . . . . . . . 81 81 83 85 85 iv List of Tables 2.1 Synthetic data profiles. . . . . . . . . . . . . . . . . . . . . . . . . . . . Percent of responding adult drug courts considering individuals eligible for participation, by select attributes . . . . . . . . . . . . . 3.2 Population of arrestees eligible for drug courts . . . . . . . . . . . . 3.3 Estimated number of individuals receiving each treatment modality in drug courts . . . . . . . . . . . . . . . . . . . . . . . . . . . . . . . 3.4 Costs associated with four treatment modalities . . . . . . . . . . . 3.5 Administrative costs for drug courts . . . . . . . . . . . . . . . . . . 3.6 Price for four treatment modalities . . . . . . . . . . . . . . . . . . . 3.7 Cost of offending, pre-sentence . . . . . . . . . . . . . . . . . . . . . . 3.8 Probability that an offender is sentenced to probation, jail, or prison . . . . . . . . . . . . . . . . . . . . . . . . . . . . . . . . . . . . . . 3.9 Estimated number of months an offender is sentenced to probation, jail or prison, and total sentencing costs . . . . . . . . . . . . . 3.10 Estimates of the total cost to society. . . . . . . . . . . . . . . . . . . 12 3.1 4.1 4.2 4.3 The estimated prevalence of potential clients nationwide. . . Estimated number of crimes avertable annually by treating tential clients under inpatient treatment modalities. . . . . . Estimated number of crimes avertable annually by treating tential clients under outpatient treatment modalities. . . . . v ... po... po... 30 33 34 37 38 39 41 42 42 43 49 51 53 vi 4.4 Cost benefit analysis of treating eligible drug courts clients in available drug dourt treatment slots. . . . . . . . . . . . . . . . . . . 4.5 Cost benefit analysis of treating all eligible drug court clients (retaining all eligibility restrictions). . . . . . . . . . . . . . . . . . . 4.6 Cost benefit analysis of treating all eligible drug court clients without regard to current offense (retaining other eligibility restrictions). . . . . . . . . . . . . . . . . . . . . . . . . . . . . . . . . . . . 4.7 Cost benefit analysis of treating all eligible drug court clients without regard to past violence (retaining other eligibility restrictions). . . . . . . . . . . . . . . . . . . . . . . . . . . . . . . . . . . . 4.8 Cost benefit analysis of treating all eligible drug court clients without regard to past treatment (retaining other eligibility restrictions). . . . . . . . . . . . . . . . . . . . . . . . . . . . . . . . . . . . 4.9 Cost benefit analysis of treating all eligible drug court clients without regard to co-occurring alcohol problems (retaining other eligibility restrictions). . . . . . . . . . . . . . . . . . . . . . . . . . . . 4.10 Cost benefit analysis of treating all drug involved arrestees (with no eligibility restrictions). . . . . . . . . . . . . . . . . . . . . . . . . . 55 57 59 61 63 65 67 List of Figures 2.1 Graphical depiction of the synthetic dataset. . . . . . . . . . . . . . 19 3.1 Graphical depiction of the treatment effects computation. . . . . 25 A.1 Successfully recovering hiddens signals using empirical similarity weights: An example. . . . . . . . . . . . . . . . . . . . . . . . . . . 90 vii viii Acknowledgments The authors would like to thank those who assisted our efforts in collecting, preparing and analyzing the data used in this study. Michael Kane, Bogdan Tereshchenko, Aaron Sundquist, Jay Reid and Will Jurist provided exceptional assistance preparing the data sets for analysis. Adele Harrell and Terrence Dunworth contributed to the conceptual development of the core hypotheses tested in this study. Shelli Rossman kindly allowed us to coordinate cost data collection with the MADCE. Nancy La Vigne provided excellent comments on the final narrative. We also wish to thank three anonymous reviewers for their comments on the technical matters. We would like to thank Janice Munsterman, Jennifer Handley and Marilyn Moses at the National Institute of Justice who monitored this project for the patience and guidance. Despite their guidance, all remaining errors are our own. ix x Abstract Despite a growing consensus among scholars that substance abuse treatment is effective in reducing offending, strict eligibility rules have limited the impact of current models of therapeutic jurisprudence on public safety. This research effort was aimed at providing policy makers some guidance on whether expanding this model to more drug-involved offenders is cost-beneficial. Since data needed for providing evidence-based analysis of this issue are not readily available, micro-level data from three nationally representative sources were used to construct a synthetic dataset—defined using population profiles rather than sampled observation—that was used to analyze this issue. Data from the National Survey on Drug Use and Health (NSDUH) and the Arrestee Drug Abuse Monitoring (ADAM) program were used to develop profile prevalence estimates. Data from the Drug Abuse Treatment Outcome Study (DATOS) were used to compute expected crime reduction benefits of treating clients with particular profiles. The resulting synthetic dataset—comprising of over 40,000 distinct profiles—permitted the benefit-cost analysis of a limited number of simulated policy options. We find that roughly 1.5 million arrestees who are probably guilty (the population most likely to participate in court monitored substance abuse treatment) are currently at risk of drug dependence or abuse and that several million crimes could be averted if current eligibility limitations were suspended and all at-risk arrestees were treated. Under the current policy regime (which substantially limits access to treatment for the population we are studying) there are about xi xii 55,000 individuals treated annually—about 32,000 are at risk of dependence and 23,500 at risk of drug abuse. In total, about $515 million dollars is spent annually to treat those drug court clients yielding a reduction in offending which creates more than $1 billion dollars in annual savings. Overall, the current adult drug court treatment regime produces about $2.21 in benefits for every $1 in costs, for a net benefit to society of about $624 million. Every policy change simulated in this study yields a cost-effective expansion of drug treatment. That is, removing existing program eligibility restrictions would continue to produce public safety benefits that exceed associated costs. In particular, removing all eligibility restrictions and allowing access to treatment for all 1.47 million at risk arrestees would be most cost effective—producing more than $46 billion in benefits at a cost of $13.7 billion. Executive Summary The primary goal of this research is to determine the size of the drug-involved offender population that could be served effectively and efficiently by partnerships between courts and treatment. Despite a growing consensus that substance abuse treatment promotes desistance, few arrestees receive sufficient treatment through the criminal justice system to reduce their offending. Strict eligibility rules and scarce resources limit access to treatment, even in jurisdictions that embrace the principles of therapeutic jurisprudence. The result is that existing linkages between the criminal justice system and substance abuse treatment are so constrained that at best only small reductions in crime can be achieved. The limited access to treatment for the criminal offender population appears to be based on subjective judgments of both the risks of treating offenders in the community and the benefits of treatment. Risks are assumed to be high for most offenders, and the benefits of treatment are assumed to be low. As a result, almost all drug-involved arrestees are determined to be ineligible for participation in community-based treatment programs. An important question for the nation’s drug policymakers is whether a substantial expansion of substance abuse treatment would yield benefits from reduced crime and improved public safety. A related question is whether evidence-based strategies can be developed to prioritize participation, given limited resources. Previous efforts to address these questions have been constrained by limitations in extant data, as well as by substantial econometric challenges to combining those data. The development of new modeling techniques now allows xiii xiv disparate data sources to be combined and critical values to be imputed for missing observations. Thus, in this paper we are able to combine data from several prior studies to simulate the effects of treatment on an untreated cohort of arrestees. That is, using existing data on the drug use patterns of arrestees and data predicting outcomes from the treatment of similar cohorts, we are able to predict not only how much crime could be prevented through treatment of an arrestee population, but also which arrestees (based on their attributes) are the best (and worst) candidates for community-based treatment. We use evidence from several sources to construct a synthetic dataset to estimate the benefits of going to scale in treating drug involved offenders. We combine information from the National Survey on Drug Use and Health (NSDUH) and the Arrestee Drug Abuse Monitoring (ADAM) program to estimate the likelihood of drug addiction or dependence problems and develop nationally representative prevalence estimates. We use information in the Drug Abuse Treatment Outcome Study (DATOS) to compute expected crime reducing benefits of treating various types of drug involved offenders under different treatment modalities. We find that 1.5 million arrestees who are probably guilty (the population most likely to participate in court monitored substance abuse treatment) are at risk of abuse or dependence. We estimate that roughly 1.15 million potential clients are at risk of drug dependence and roughly 322,000 are at risk of abusing drugs. We find that several million crimes would be averted if current eligibility limitations were suspended and all at-risk arrestees were treated. Our estimates are smaller than other studies, because we restrict our study sample to those both at risk of abuse and dependence and to those likely to be found guilty, since this is the population most likely to become eligible for criminal justice system-based substance abuse treatment. Although our estimates are therefore smaller than other published estimates, the distribution of client attributes is similar. We find that substance abuse treatment in each of the four DATOS domains substantially reduces recidivism among this population. For those at risk of drug xv dependence, long-term residential reduces recidivism by 34%, short-term inpatient by 19%, outpatient methadone by 20%, and outpatient drug free by 30%. For those at risk of drug abuse, recidivism reductions are large (27%). Outpatient drug free is the most effective modality, reducing recidivism by 33%. Long-term inpatient reduces recidivism by 27%, short-term inpatient by 20% and outpatient methadone by 16%. The pattern of offending reduction is generally similar for those at-risk of drug abuse as it is for those at risk of dependence, where the largest reductions occur for the most prevalent (but least costly) crime types. Small (or no) reductions in crime are observed for the most serious crimes. These findings are also consistent with previous estimates. We find that under the current policy regime (which for the most part limits access to treatment for the population we are studying to drug courts) there are about 55,000 individuals treated annually, about 32,000 are at risk of dependence, and 23,500 are at risk of drug abuse. In total, we estimate that about $515 million dollars is spent annually to treat those drug court clients and that this yields a reduction in offending which creates more than $1 billion dollars in annual savings. Overall, we estimate that the current adult drug court treatment regime produces about $2.21 in benefit for every $1 in costs, for a net benefit to society of about $624 million. The benefit-cost ratio is higher for those at risk of abuse (2.71) as compared to those at risk of dependence (1.84), even though the abuse group is less prevalent in the drug court population. We estimate that there are about twice as many arrestees eligible for drug court (109,922) than there are available drug court treatment slots (55,365). We simulate the effects of treating all of these currently eligible in the four treatment modalities studied by DATOS, and find that the costs of treating these additional clients about doubles, to slightly more than $1 billion. We find that the expansion of drug treatment to this larger population remains cost-effective, although the benefitcost ratio is fractionally reduced to 2.14 from 2.21. In total, this expansion of treatment yields a benefit to society of more than $1.17 billion dollars. We also simulate several expansions of criminal justice system-based treat- xvi ment which allow many arrestees into treatment who are currently not eligible. We estimate that expanding treatment access to those with a pending case is cost beneficial, with a about $1.65 billion in total benefits. In particular, allowing those with a pending case who are at-risk of drug dependence is especially beneficial, with a benefit to cost ratio 4.13:1. We find that allowing those with past violence into court supervised treatment is as cost-beneficial as current practice, with a benefit to cost ratio of 2.15. While the addition of those at risk of abuse with prior violence is cost-beneficial (3.14:1) adding those at risk of drug dependence with prior violence is much less cost-beneficial (1.38:1). Expanding the program to include those with a history of failed treatment is also cost-beneficial (2.09:1), especially for those at risk of drug abuse (2.29:1). Allowing those with co-occurring alcohol problems into court supervised treatment yields is cost-beneficial for the entire group treated (1.73:1). However, again, these aggregate numbers mask important differences in the responsiveness of this new population to treatment. For those at risk of dependence, the results are better, with the newly added group estimated to have a benefit to cost ratio of 1.43:1. However, adding those with co-occurring alcohol problems who are at risk of drug dependence is not cost-effective (0.70:1). Finally, we consider allowing access to court-supervised treatment for all arrestees, without restriction. Treating all at-risk arrestees would cost more than $13.7 billion and return benefits of about $46 billion. We find that this approach would be cost-effective, with a benefit of $3.36 for every dollar in cost. Chapter 1 Background and Motivation 1.1. INTRODUCTION Recent innovations in the criminal justice system have linked intensive criminal justice system surveillance with substance abuse treatment. The goal of this justice-treatment partnership is to improve outcomes for drug-involved offenders and to reduce the burden of chronic drug use on private citizens, the police, courts, and corrections. These interventions, labeled therapeutic jurisprudence, are designed to identify and treat drug-involved offenders in the community in lieu of incarceration. The programs take many forms. There is widespread use of diversion programs that provide short-term pre-trial services to large numbers of low-risk arrestees. These programs may either incorporate direct judicial monitoring or may add more strenuous treatment requirements to the conditions of community supervision. More intensive and long-term treatment is managed by a judge on a separate court calendar. These programs, generally known as drug courts, serve a more serious but narrowly targeted population. Although these programs exist is many, if not most, jurisdictions, only a fraction of the drug-involved arrestee population receives these programs. Proponents of these interventions often discuss ‘going to scale’ and making them available to the entire population of eligible offenders. 1 2 To Treat or Not to Treat Despite the prominence of these initiatives, few arrestees who meet the clinical criteria for drug abuse or drug dependence receive intensive, long-term substance abuse treatment in the community in lieu of incarceration. Pre-trial diversion programs treat relatively large numbers of offenders, and many drug involved offenders receive at least a pre-trial referral to treatment. However, the dosage and duration of these programs is generally very limited. The more intensive adult drug court is a promising model for reducing recidivism. However, while there are drug courts operating in most mid- and large-sized counties in the US, most are very small and serve less than 100 clients annually. Thus, only a small number of drug-involved arrestees receive intensive long-term treatment in lieu of incarceration. There are few reliable estimates of the number of arrestees who annually enroll in and/or complete a court supervised treatment program. Using data from a census of drug courts conducted as part of the Multi-Site Adult Drug Court Evaluation (MADCE), we estimate that fewer than 30,000 adult arrestees were enrolled in drug courts in 2005, a trivial percentage of the ten million individuals arrested each year (Rossman et al. 2007) Probation and parole based treatment programs have also shown promise, but few large-scale programs exist today. The limited penetration of these programs is the result of several forces. First, tradition holds that federal sponsorship of new programs is limited to the funding of demonstration programs. So, while new programs can be implemented, identifying the resources to take these programs to scale is left to local governments. Second, the programs themselves are costly. Even cost-effective programs are more expensive to administer than business as usual, and thus require additional funding if they are to expand. Third, the beneficiaries of these programs generally are not the same party who pays for the program. For example, while the costs of drug court are born almost entirely by the court system, the court does not explicitly realize the benefits. Instead, the benefits of drug courts tend to accrue to private citizens who have a reduced risk of victimization and to corrections departments who have to house fewer offenders and Background and Motivation 3 recidivists. Thus, unless measures can be enacted that pool the risk (and the rewards) of these programs, there are substantial disincentives to implementation. Fourth, the benefits of the program to date have largely been estimated at the micro-level. Due to the small number of clients involved, much of the success of the programs comes from reduced recidivism within a small group of offenders, but not from a meaningful reduction of crime rates in the community (i.e., at the macro-level). Finally, and as a result of the aforementioned factors, even though there is a large and growing body of research that demonstrates the efficacy of these initiatives, there is substantial reluctance across the political spectrum to treat convicted (or convictable) offenders in the community in lieu of prison. The goal of this research then is to determine what would happen if none of these factors impeded a national investment in therapeutic jurisprudence. That is, suppose that there were no obstacles to the implementation of effective treatment for drug-involved offenders. How many offenders enter the criminal justice system each year who would be clinically diagnosed as drug abusers or drug dependent? How many crimes could be prevented is they received treatment? Given that treatment benefits may vary across treatment modalities, how many crimes could be reduced by each of the most common modalities? Given that amenability to treatment varies by arrestee attributes, can better (and worse) candidates for therapeutic jurisprudence programs be identified ex ante? How much would the delivery of this treatment cost? How big are the benefits? In sum, if access to treatment were expanded, how many crimes could be prevented and what are the costs and benefits off doing so? We use evidence from several sources to construct a synthetic dataset for answering the question: what are the expected benefits of ‘going to scale’ in treating drug involved offenders? To answer this question, we combine drug use and criminal justice data from the National Survey on Drug Use and Health (NSDUH) and the Arrestee Drug Abuse Monitoring (ADAM) program to estimate the prevalence of drug addiction or dependence in the arrestee population. Expected outcomes from substance abuse treatment from the Drug Abuse Treat- 4 To Treat or Not to Treat ment Outcome Study (DATOS) is used to predict the benefits from treatment in terms of reduced crime. We find that about 1.5 million (probably guilty) arrestees are at risk of abuse or dependence and that several million crimes would be averted if current eligibility limitations were suspended and all at risk arrestees were treated. 1.2. 1.2.1. PRIOR RESEARCH Drug-Crime Nexus A substantial research literature links substance use and abuse to criminality (Anglin and Perrochet 1998; Ball et al. 1983; Boyum and Kleiman 2002; Brownstein et al. 1992; Condon and Smith 2003; Dawkins 1997; DeLeon 1988a; DeLeon 1988b; Harrison and Gfroerer 1992; Inciardi et al. 1996; Inciardi 1992; Inciardi and Pottieger 1994; Johnson et al. 1985; MacCoun and Reuter 2001; Miller and Gold 1994; Mocan and Tekin 2004). Psychopharmacologic effects of substance use lead users to commit crimes while intoxicated and economiccompulsive effects lead users to commit crimes to gain resources to buy drugs (Goldstein 1985). Violence associated with the drug trade, and victimization of intoxicated, drug users contribute to drug-involved crime as well (Boyum and Kleiman 2002; Goldstein 1985; MacCoun et al. 2003). Drug sellers are often drug users (Reuter, MacCoun and Murphy 1990), and sellers who are incarcerated tend to be replaced by new suppliers (Freeman 1996; Blumstein 2000). Young drug users and suppliers are most likely to be violent (Blumstein and Cork 1996; MacCoun et al. 2003). Drug users are also more likely to be the victims of violence than non-drug users (Cottler, et al 1992), and criminal behavior increases as the frequency and intensity of use increases (Anglin et al. 1999; Anglin and Maugh, 1992; Chaiken and Chaiken 1990; Stewart et al. 2000; Vito, 1989). Criminal incidence is highest for substance abusers while they are using drugs, four to six times more than when they are not abusing narcotics (Ball et al. 1982; Gropper, 1985), a pattern that is even more pronounced among habitual Background and Motivation 5 offenders (Vito 1989). Conversely, crime decreases as drug use declines, particularly when it is income-generating crimes that decrease (Anglin et al. 1999; Chaiken and Chaiken, 1982; Degenhardt et al 2005; Inciardi 1987; Nurco et al. 1990; Speckert and Anglin, 1986). A corresponding literature suggests treatment can be effective in reducing demand and associated offending. Economic studies have found that treatment is more cost-effective than incarceration (Caulkins et al. 1997; MacKenzie 2006; Lipsey and Cullen 2007), that intensive long-term treatment is most effective (NIDA 1999), that direct interaction with a judge is more effective for serious drug users (Marlowe et al. 2004), and that violent offenses cause the greatest economic damage to communities (Cohen 2003). The Drug Abuse Treatment Outcome Studies (DATOS) found length of participation in treatment to be the key predictor of success. While attrition rates from treatment are estimated from 40% to 90%, studies show that those who remain in treatment for a sufficient period subsequently commit fewer crimes (French, et al 1993; Lewis & Ross, 1994; Simpson, et al. 1997). In particular, patients with more severe problems reported better outcomes after 90 days of treatment (Simpson et al. 1999). After one year of treatment, major outcome indicators for drug use, illegal activities, and psychological distress were each reduced on average by about 50% (Hubbard et al. 1997). Prevalence of arrest fell from 34% in the year before intake to 22% one year after enrolling in treatment (Simpson et al. 2002). The average net economic benefit from an episode of long-term residential treatment was $10,344 and $795 from an episode of outpatient treatment (Flynn et al. 1997). The number of drug users has remained relatively stable over time (Rhodes, et al 2000) suggesting a general aging of the cocaine and heroin-using population (Golub and Johnson 1997). Despite this, drug users face a significant risk of arrest and incarceration: a part-time drug seller in Washington, D.C. has a 22% risk of imprisonment in a given year, and spends about one-third of their criminal career incarcerated (Reuter et al. 1990; MacCoun and Reuter 2002). Mark Kleiman (1997) estimates that heavy users of cocaine who are arrested annually 6 To Treat or Not to Treat consume 60% of the cocaine in the United States. The Bureau of Justice Statistics estimates that about half of both federal prisoners and jail inmates abuse or are dependent on drugs (Mumola & Karberg 2006; Karberg & James 2005). Few, however, received treatment within the criminal justice system (Harrell and Roman 2001; Marlowe 2003). Among incarcerated populations, only about 15 percent received drug treatment (Karberg and James 2005). 1.2.2. Criminal Justice System Interventions with Drug-Involved Offenders Given the preponderance of evidence that treatment can be effective, over the last three decades various criminal justice system innovations have emerged to treat drug and alcohol abusing offenders in the community with criminal justice system oversight. The first widespread intervention, Treatment Accountability for Safe Communities (TASC), redirected drug offenders from the court system into treatment facilities. TASC provided the link between the judicial system and treatment services and offered participation incentives in the form of case dismissal for successful completion (Nolan 2001). An outcome evaluation of five TASC programs found some evidence of reduced recidivism and drug use, but the results were mixed (Anglin et al. 1999). In the late 1980s, a more rigorous program, Intensive Supervision Probation (ISP), was developed to monitor drug offenders in the community as an alternative to incarceration with the goal of reducing prison crowding and providing more thorough supervision than regular probation (Tonry 1990). However, evaluation of the ISP program suggests that the level and intensity of treatment was minimal and the program failed to affect participant drug involvement. Overall, ISP resulted in increased incarceration rates, although the effect may have been confounded by increased participant scrutiny (Tonry 1990; Petersilia, et al. 1992; Turner, et al. 1992). Similar programs such as those mandated under Proposition 36 passed in California in 2001 and the Drug Offender Sentencing Alternative (DOSA) program in Washington serve large numbers of offenders in several states, and these have generally been Background and Motivation 7 found to yield better treatment and criminal justice outcomes (Aos et al. 2005; Longshore et al. 2004). 1.2.3. Therapeutic Jurisprudence and Drug Courts While each of these programs has linkages to the criminal justice system, they did not fully exploit the coercive powers of the criminal justice system to incentivize compliance with treatment protocols. Under the rubric of therapeutic jurisprudence, a more formal model of intensive court-based supervision, referred to as drug treatment courts, emerged in the 1990s (Hora, Schma and Rosenthal 1999; Senjo and Leip 2001; Slobogin 1995; Wexler and Winick 1991). The therapeutic jurisprudence model posits that legal rules and procedures can be used to improve psychosocial outcomes, an idea supported by a growing research consensus that coerced treatment is as effective as voluntary treatment (Anglin et al., 1990; Belenko 1999; Collins and Allison 1983; DeLeon 1988a; DeLeon 1988b; Hubbard et al. 1989; Lawental et al. 1996; Siddall and Conway 1988; Trone and Young 1996). A number of studies have found that drug treatment court participation reduces recidivism rates (Finigan, 1998; Goldkamp and Weiland 1993; Gottfredson and Exum 2002; Harrell and Roman 2002; Jameson and Peterson 1995; Peters and Murrin 2000; Wilson et al. 2006). In response, the model has proliferated, and by 2005 there were about 600 adult drug courts in operation in the United States, including most medium and large counties. Despite the pervasiveness of the drug treatment court model, drug courts routinely exclude most of the eligible population. A survey of adult drug courts in 2005 (Rossman et al 2007) found that only 12% of drug courts accept clients with any prior violent convictions. Individuals facing a drug charge, even if the seller is drug dependent, are excluded in 70% of courts for misdemeanor sales and 53% of courts for felony sales. Other charges that routinely lead to exclusion include property crimes commonly associated with drug use (theft, fraud, prostitution), young offenders with marijuana charges, and current domestic violence cases (only 20% accept domestic violence cases). Many also reject of- 8 To Treat or Not to Treat fenders based on the severity of the users drug problem. However, eligibility based on drug use severity is applied inconsistently—16% of drug courts exclude those with a drug problem that is deemed too serious, while 48% reject arrestees whose problems are not severe enough. Almost 69% exclude those with cooccurring disorders. Even among eligible participants, more than half of drug courts (52%) report they cannot accept some clients who are eligible for participation due to capacity constraints. Given these criteria, we estimate that fewer than 30,000 annually enroll in drug treatment courts. On the other hand, if the Bureau of Justice Statistics estimates of dependence and abuse are correct, several million individuals are arrested annually who use illegal substances (Mumola & Karberg, 2006; Karberg & James, 2005). Based on available evidence on the general crime reducing benefits that accrue by treating drug involved offenders, our research was motivated by the need to provide policy makers some guidance on the prospects of going to scale. That is, what crime reductions can we reasonably expect if more drug involved offenders received treatment? We explain our analytical strategy for exploring this issue next. Chapter 2 The Research Design The most straightforward way to investigate this issue would be to identify and assess a sample from the population of all arrestees nationwide who are probably guilty and at risk of drug abuse or dependence. That is, since an admission of guilt is generally required for enrollment into therapeutic jurisprudence based programs, we would need to ensure that we sample from a population of guilty arrestees. For each arrestee we would like to observe the number of crimes (by type) that could be prevented if they were treated (under various treatment modalities). Of course, such data do not exist. It is, however, possible to generate synthetic data that provide information on all the relevant quantities. If appropriately constructed, analysis of these synthetic data provides a sound basis for making valid inferences about population characteristics. Our research employs this strategy for estimating the number of potential clients and the expected number of crimes avertable by treating these potential clients under various treatment modalities. To construct our sample, we define a potential client of a therapeutic jurisprudence program as any arrestee who is probably guilty of a crime and is also at risk of substance abuse. To determine the nature of the arrestee’s substance use, we use the DSM-IV criteria to asses whether or not a drug involved offender is at risk of being dependent on drugs or of abusing drugs. We also 9 10 To Treat or Not to Treat include a set of client attributes (e.g., age, race, or gender) as traits to be included in the design of the synthetic data, e.g. as attributes along which dependency may vary. We perform all of our analyses on profiles that represent types of individuals, rather than on individual observations. Because we combine information from multiple data sources, we can not observe information about the same individuals describing their risk, dependence and responsiveness to treatment. Since we can observe common attributes–socio-demographic characteristics, and information about substance abuse and criminal histories across datasets–we create simulated (synthetic) profiles. These profiles, which are combinations of all attributes, are then used in the analysis in much the same way individual observations would be used had that information been available. From these multiple data sources, we develop an exhaustive set of ‘profiles’, where each profile is one possible combination of all of the potential client’s attributes. For example, if we simply collected data on age, race and number of prior arrests, we would develop one profile for young male arrestees with few prior arrests, one profile for young male arrestees with many priors, etc. Hence, the synthetic data used in this analysis includes a profile for every client permutation, allowing us to quantify the effect of drug treatment on every combination of client attributes and characteristics. We use simulation models to estimate outcomes for each profile, conditional on receiving each of four possible treatment modalities (described below). We use data from studies linking client traits and characteristics to outcomes to identify expected outcomes for each of these profiles. Since not all of the profiles are represented in the outcomes data (from DATOS), we estimate expected outcomes for all client profiles. Putting all of these approaches together, we are able to model expected outcomes from treatment for a wide variety of potential clients. The Research Design 2.1. 11 SYNTHETIC DATA A great deal of attention has recently been paid to the potential of using synthetic data as a way of archiving data regarding individual behavior without actually providing sensitive micro-data (Abowd and Woodcock 2001). In that literature, the main purpose of synthetic data is to replace the actual micro data with a scientifically valid replacement, allowing for the robust estimation of outcomes without violating the confidentiality of individuals represented by the data. One valuable extension of this approach is the use of many synthetic data sets to generate expected outcomes, which allows for the modeling of statistical uncertainty and the generation of standard errors and confidence intervals around outcomes. The method has been in general use in statistics for more than 25 years for handling missing data and has recently been formalized for the synthetic data problem (Reiter 2002, 2003; Ragunathan et al. 2003). Our motivation for using synthetic data is slightly different. We use this design for the purpose of bringing together information from several disparate data sources. Data for this study were developed from the National Survey on Drug Use and Health (NSDUH) and the Arrestee Drug Abuse Monitoring (ADAM) program—to estimate the likelihood of various arrestee profiles having drug addiction or dependence problems—and from the Drug Abuse Treatment Outcome Study (DATOS)—to compute expected crime reducing benefits of treating various profiles of drug involved offenders under different treatment modalities. In order to do so, we defined a detailed exhaustive set of client attributes (based on availability across these sources) as a base data set. The attributes that define the synthetic database include all characteristics available for all the databases used in this study. We began by creating a synthetic data set of 40,320 client profiles with the attributes and categories listed in table 2.1. The base synthetic data set is a cross combination of all of these attributes. Each profile in the dataset is a list of attribute combinations that represents one row in this data set. For example, one profile could be a white male, aged 25 years, who is arrested for a drug offense, who does not have a violent past nor 12 To Treat or Not to Treat Table 2.1: Synthetic data profiles. Category Attributes Age Race Gender Criminal History Current Offense Arrestee age (20, 25, 30, 35 40, or 45) Arrestee race (Black, White, or Other) Arrestee gender (Male or Female) Number of prior arrests (0, 2, 5, 10, or 20) Most serious charge at current arrest (Violent, Drug, Property, or Other) Any prior violent charges (Yes or No) Any prior treatments (Yes or No) Is arrestee currently under any criminal justice supervision (Yes or No) Is arrestee at risk of alcohol dependence or abuse (Yes or No) Non-MSA, Large MSA (more than 1 million residents), or Small MSA (less than 1 million residents) Violent History Treatment History Criminal Justice Status Alcohol problems Geographic location any past treatment, has two prior arrests, was not under active criminal justice supervision at the time of arrest, and was arrested in a MSA with less than a million residents. Another distinct profile could be for a female who has all the same remaining features. The next step is to determine how many individuals in the population are represented by each profile. That is, the proportion of the population (the prevalence) of each profile. For each profile, a value is estimated that is used for weighting the profile by its prevalence in the population of interest. Moreover, since we are interested in assessing the prevalence of potential clients at risk of drug dependence or abuse, we have generated prevalence estimates conditional on drug dependence or abuse. Independently of the profiles’ prevalence, we also generate estimates of the expected crime reducing benefits of treating potential clients under various treatment modalities. This information is obtained from DATOS, and we interpolate all expected outcomes of treatment onto the profiles in the synthetic dataset. The Research Design 13 We describe the interpolation technique as well as the steps taken to obtain prevalence and treatment effect estimates in more detail in the following chapter. Before that, we turn to a description of our main data sources and their intended uses in constructing the synthetic data. 2.2. DATA SOURCES AND USES To populate the synthetic data columns, we rely on three main data sources. We combine information from the National Survey on Drug Use and Health (NSDUH) and the Arrestee Drug Abuse Monitoring (ADAM) program to estimate the likelihood of various arrestee profiles having drug addiction or dependence problems. We also use the same sources to develop prevalence estimates of these profiles among arrestees nationally. We use information in the Drug Abuse Treatment Outcome Study (DATOS) to compute expected crime reducing benefits of treating various types of drug involved offenders under different treatment modalities. Each dataset is described in more detail below. 2.2.1. Arrestee Drug Abuse Monitoring (ADAM) ADAM data is used as the first step in defining the attributes used to construct our profiles, that is as the first source of socio-demographic, treatment and criminal information that may be common among datasets that describe risk of drug abuse or dependence. Since ADAM data is not representative, we use data from NSDUH to re-weight our ADAM sample. Funded by the National Institute of Justice, the Arrestee Drug Abuse Monitoring (ADAM) program is a research program designed to estimate illicit drug use in the population of arrestees in urban areas. Data on a stratified sample of arrestees were collected on a quarterly basis in 2000, 2001, 2002 and, again in 2003 in 39 selected metropolitan areas in the United States. The sampling frame represents all facilities in the target county and all types of offenders arrested and booked on local and state charges within the past 48 hours. Self-reported and administrative data collected 14 To Treat or Not to Treat include: (1) demographic data on each arrestee, (2) administrative criminal justice records, (3) case disposition, including accession to a verbal consent script, (4) calendar of admissions to substance abuse and mental health treatment programs, (5) data on alcohol and drug use, abuse, and dependence, (6) drug acquisition data covering the five most commonly used illicit drugs, (7) urine test results, and (8) for males, weights. ADAM contains several items that make it particularly appealing for our analysis. First, arrestees were asked about the total number of times they were arrested in the prior 12 months. We use this quantity to estimate the prevalence of arrestees nationwide (described in more detail below). The same domains are also collected for a non-probabilistic sample of female arrestees. Second, among respondents who said they used drugs or alcohol during the last 12 months, arrestees were asked six questions about their substance use to allow for a categorization of their risk of drug abuse or dependence following Diagnostic Statistical Manual (DSM-IV) definitions. The DSM-IV distinguishes between two types of drug problems, substance abuse disorder and substance dependency disorder (which is commonly referred to as addiction). Substance abuse disorder is based on the frequency of drug use, the use of drugs in particular situations, negative outcomes that can be linked to the use of drugs, pronounced use of drugs in the face of evidence that drugs are contributing to personal and interpersonal problems. By contrast, the conditions for substance dependency disorder are broader. These include physical symptoms, such as tolerance and withdrawal, and patterns of behavior aimed at either unsuccessfully reducing the influence of drugs or allowing for greater amounts of drugs to be taken.1 Finally, ADAM contains detailed information on the current charge, the arrest history, criminal justice status, arrestee age, race, and gender, as well as an 1 Data from ADAM describing individual responses to DSM-IV items are not included in the public release version of ADAM. Public data flag individuals as being at risk of drug dependence or abuse. Similarly, they are flagged as being at risk of alcohol dependence or abuse. We use these items to compute the probability of drug dependence or abuse. The Research Design 15 indicator for the ADAM site. We use information from the Census to classify each of the ADAM sites as belonging either to a large MSA (with over 1 million residents), a small MSA (with fewer than 1 million residents), or a non-MSA. Using all these items, we create the set of arrestee attributes for each profile. We use ADAM data (in combination with NSDUH as described below) to predict the probability that a particular profile is at risk of drug abuse or dependence. The probability of drug abuse or dependence calculated from ADAM and NSDUH data were then joined with DATOS data to predict expected changes in offending, conditional on treatment. In sum, in our analysis, we used ADAM data to predict the probability that a profile is at risk of drug abuse or dependence. However, since ADAM is not nationally representative, the prevalence of each profile is also not nationally representative for several reasons. For instance, we have combined the male and female samples in ADAM, which means our final sample is not representative even of the 39 metropolitan areas included in ADAM. Moreover, because ADAM is a study mainly of metropolitan areas, it undercounts arrestees in smaller MSAs and non-MSAs. In order to correct for these imbalances and to make ADAM data more representative of all arrestees nationwide, we utilize another data set (NSDUH) to re-weight ADAM data. The item that is re-weighted using NSDUH is the prevalence of each profile in the population. The probability that a profile is at risk of drug abuse or dependence is still calculated from ADAM, but the weight assigned to each profile (how often it appears in the population) is adjusted using NSDUH. In re-weighting the ADAM data, we are motivated by making the sample of arrestees in the ADAM data more representative of arrestees nationwide. What we attempt is different from what, for example, Rhodes and his colleagues (Rhodes at al. 2007) computed. Rhodes et al. (2007) are primarily interested in computing the arrest rate among chronic drug users. Our primary interest lies in computing the prevalence of risk of drug dependence or abuse among arrestees nationwide. 16 2.2.2. To Treat or Not to Treat National Survey on Drug Use and Health (NSDUH) Funded by the United States Department of Health and Human Services, the National Survey on Drug Use and Health (NSDUH) series (formerly titled National Household Survey on Drug Abuse) measures the prevalence and correlates of drug use in the United States. The NSDUH data represents the civilian, non-institutionalized population of the United States age 12 and older. The survey covered substance abuse treatment history and perceived need for treatment, and (as is the case for ADAM) includes questions from the Diagnostic and Statistical Manual (DSM) of Mental Disorders to apply diagnostic criteria. Moreover, the data provide detailed information on illegal involvement, which allows us to select observations from NSDUH that resemble the population in ADAM, and thus the population of interest in the study. Using information on illegal involvement over the last 12 months, we sub-set the data to include only those individuals who were arrested and booked at least once during the last year. Additionally, we restricted our NSDUH sample to adults who were 18 years and older. From the initial respondent pool of 55,031, we select about 10,347 observations for use in our study. Based on a set of elements common to ADAM and NSDUH, we computed new ADAM weights to re-weight the current ADAM sample to exploit the more representative data in the NSDUH sample. The items we include in the reweighting include age, race, gender, current offense, flags indicating risk of drug dependence, risk of drug abuse, a flag indicating risk of alcohol dependence or abuse, and geographic location (non-MSA, large MSA, or small MSA). We estimate the probability that an observation in the combined NSDUH and ADAM datasets is in ADAM. We then use this predicted probability to compute a weight which is applied to the profile’s prevalence among all arrestees nationwide. Define ti = 1 if the observation is from ADAM (the convenient sample) and ti = 0 if it is from NSDUH (a sample representing the target population more closely). Then define wi = Pr(ti = 0|xi )/ Pr(ti = 1|xi ) ∀i ∈ Nc where Nc is the size of the ADAM sample. One may further compute functions of these weights, e.g., The Research Design 17 normalized to 1, before using it to re-weight the convenient sample for analysis. After re-weighting, we confirmed that the re-weighted ADAM sample did resemble the NSDUH data, at least on the attributes utilized in the exercise. From that point onwards, we utilized the re-weighted ADAM data for all our analysis. 2.2.3. Drug Abuse Treatment Outcome Study (DATOS) Funded by the National Institute on Drug Abuse (NIDA), the Drug Abuse Treatment Outcome Studies (DATOS) began in 1990 with the goal of evaluating substance abuse treatment outcomes. DATOS is a longitudinal study of 10,010 adults entering 96 participating treatment programs in eleven statistically representative cities from 1991 to 1993. DATOS evaluated the effectiveness of four common treatment modalities on four primary topical domains: (1) cocaine use, (2) HIV risk behaviors, (3) psychiatric co-morbidity and (4) criminal behavior. Treatment modalities included Outpatient Methadone Treatment (OMT), Long-Term Residential (LTR), Outpatient Drug-Free (ODF), and Short-Term Inpatient (STI). Data were collected at baseline and 3, 6, 12, and 60 months after completion of treatment. Data from DATOS were used to estimate the crime-reducing benefits of treating drug involved offenders. That is, we wanted to link each profile, weighted by its prevalence in the population, to the expected change in criminal offending that would occur if that profile were treated. We perform the exercise by treatment modality so that we obtain estimates of the crime reducing benefits to be expected conditional on arrestee attributes, treatment modality, and risk of drug dependence or abuse. Moreover, we were able to obtain detailed crime reducing benefits for all crimes as well as select sub-types (including drug related, fraud & forgery, burglary, larceny, robbery, aggravated assaults, and sexual assaults). DATOS contains detailed information on illegal activities one year prior to treatment intake, the complete history of illegal activities prior to that, the illegal activities in the year following intake, and detailed information on our 18 To Treat or Not to Treat attributes of interest. However, DATOS represents only participants entering treatment—there are no counterfactual data. That is, there is no comparison group studied which would generate information about expected offending for non-participants. Thus, no data from DATOS are available that directly predict how much crime was prevented by treatment. Therefore, we must interpolate expected outcomes using information about expected offending predicted by client attributes. We therefore generate a simulated counterfactual from these data in order to estimate the crime reducing benefits to be expected from treatment. A technical discussion of the interpolation approach can be found in Appendix I. 2.2.4. Summary of the Elements of the Synthetic Data Set Figure 2.1 graphically depicts the kind of data file we populated using data from a various sources described above. Each row in the figure represents one of the 40,320 profiles that are a crosscombination of observable attributes. The first column, “Profile’s Prevalence in the Population of Arrestees” is a value developed from ADAM and NSDUH. The next two columns use ADAM data to predict the expected proportion of that profile that would be expected to be at risk of drug abuse and at risk of drug dependence. The following columns describe the expected reduction in the number of crimes, conditional on treatment. Each of the four treatment modalities in DATOS are measured independently, and estimates are created for both groups of drug-involved offenders (those at risk of abuse and those at risk of dependence). Once these estimates are created, the total number of crimes avertable by treatment is estimated by summing across profiles, weighting each profile by its prevalence. The next section describes the estimation strategy used to generate the values in figure 2.1. Once the cells in figure 2.1 were populated, we then calculated the expected costs of delivering treatment in each modality (and an additional cost to represent the costs to the court system of administering the program). Dependence SOURCE: Re-weighted ADAM @ Risk of Dependence @ Risk of Abuse @ Risk of Dependence @ Risk of Abuse @ Risk of Dependence @ Risk of Abuse Crime Type II @ Risk of Dependence Crime Type II @ Risk of Abuse Crime Type II Treatment Modality 4 Outpatient Drug Free Crime Type II Treatment Modality 3 Outpatient Methadone Crime Type II Treatment Modality 2 Short-Term Inpatient Crime Type II Treatment Modality 1 Long-term Residential Crime Type II Crime Type I Crime Type I Crime Type I Figure 2.1: Graphical depiction of the synthetic dataset. … Profile 2 Profile 1 Profile's Prevelance in the Population of Arrestees Abuse Expected Benefits that Accrue by Treating a Particular Profile Under … Crime Type II Crime Type I SOURCE: DATOS Crime Type I Crime Type I Crime Type I Crime Type I Probability that a particular profile will be at risk of drug … The Research Design 19 20 To Treat or Not to Treat We also translate the number of crimes into monetized values to account for the variation in harms to victims and cost to the criminal justice system that is a function of the severity of the particular crimes that are prevented by treatment. A description of the method to develop these costs and benefits follows the section on estimation. Finally, once the synthetic data were prepared, and all outcomes were estimated for each profile (that is, the reduction in offending for each profile, for those at risk of drug abuse and dependence, and for each of the various treatment modalities) we ran simulation models to compare a set of policy options to inform policy. That is, it is not enough to simply say that a profile will experience x outcomes conditional on receiving y treatment, we must compare those outcomes to current policy, and to a set of possible policies. In particular, we are interested in simulating an expansion of drug court-like programs, since these programs have been demonstrated to deliver services of sufficient dosage that it is reasonable to expect that they would yield results similar to the expected outcomes predicted by the DATOS study. The simulation models consider several possible expansions of the drug court model, and these expansions are mainly achieved by sequentially relaxing current drug court eligibility rules. A description of eligibility rules follows the description of the simulation models, which in turn is followed by a section describing the findings. Chapter 3 Estimation Strategy In this section, we describe how the prevalence estimates were computed and how the benefits from reducing new crimes by treating drug involved offenders were derived. We also list a set of key assumptions that go into our analysis. All of our estimation is based on using the empirical similarity between the profiles and raw data sources. We explain the estimation and use of these similarity weights in details in Appendix IV. In short, the expected value of any quantity (for example, the prevalence or the treatment effect) for a particular profile is the weighted average of the same quantity across all observations in the raw dataset. The weights reflect the empirical similarity of the profile to the raw data set observations. This technique allows us to interpolate values from the raw datasets onto the synthetic dataset, while allowing the functional form of the links be very flexible. 3.1. A PROFILE’S PREVALENCE IN THE POPULATION OF ARRESTEES Returning to figure 2.1, the first column, the profile’s prevalence in the population of arrestees is an estimate of the annual number of arrestees nationwide for each of the 40,320 profiles in the dataset. 21 22 To Treat or Not to Treat As noted in the previous chapter, we utilize a re-weighted version of the ADAM dataset for estimating the prevalence of particular profiles in the population of interest. Unfortunately, the ADAM dataset contains information on the number of arrests each year but not the number of arrestees. However, the re-weighted ADAM dataset does provide information on the number of times individuals were arrested in the year prior to the current booking. We use the concept of a re-capture rate to convert the number of arrests into number of arrestees. Note that if, for a particular profile, we have a low recapture rate then the number of arrestees will be approximately equal to the number of arrests (since they are being recaptured infrequently). On the other hand if, for a particular profile, we have a very high recapture rate then the number of arrestees will be relatively low (since the same arrestees are being recaptured several times). First, we estimate the number of bookings per year nationwide of a particular profile by interpolating this quantity from the re-weighted ADAM. Profiles that are more similar to high booking rate observations in ADAM will have higher interpolated booking rates than will profiles that are less similar to these high booking rate observations. In a similar manner, we calculate the recapture rate for each of these profiles. Once we estimate the re-capture rate, we can then calculate the number of arrestees (as apposed to arrests). Note that the number of arrestees is an appropriately scaled down (by the recapture rate) version of the number of arrests per profile. Finally, the estimated number of arrestees can then be combined with the rate of risk of dependence and abuse to calculate the number of arrestees in each profile that are in each condition. And thus, in this step we are able to fill in data for the first three columns in figure 2.1. A detailed description of this process is provided in the mathematical appendix. 3.2. SIMULATED COUNTERFACTUAL The next step in the analysis is to calculate the crime reduction benefits that can be expected from treating drug involved offenders. There are several ways one Estimation Strategy 23 can generate this quantity. First, and optimally, if we could randomize individuals into the treatment and control groups, then the difference between their outcomes (say, recidivism) could provide us an average treatment effect. Or, in the absence of randomization, we could use some quasi-experimental design and use observational data to recover treatment effects. There exists an extensive literature on establishing causal effects from observed data. Reviewing that literature is beyond the scope of this paper (see the exchange between Heckman (2005a,b) and Soble (2005) for an extensive review of the issues and controversies surrounding causal analysis). The common thread in all of the non-experimental approaches to generating the treatment effect estimates is the need for an appropriate counterfactual. Since the same individual cannot be observed under treatment and not under treatment, we need some way to generate an estimate of what would have happened to treated individuals had they not been treated. There are three essential components to the development of the counterfactuals. First, we have observed outcomes (e.g., recidivism) that we wish to compare. Second, we potentially have a set of observed attributes (e.g., age, race, gender, etc.) that may be relevant to take into account when comparing those who do and do not receive treatment. Finally, there is the unknown—what we don’t know or see about the individual—that may be relevant for computing an appropriate counterfactual. Experimental methods are designed to mitigate the effect of unobserved states and traits to allow comparisons across those who do and do not receive treatment. Typically, all available evidence is used to make sure that the groups are as comparable as possible either by matching them, weighting them, or randomly assigning them, and then multivariate regression (or some variant thereof) is used to account for heterogeneity. In this research effort, we take a different approach to estimating the crime reducing benefits of treating drug involved offenders. We use data only on a sample of individuals receiving various forms of treatment. However, rather than seek to identify an appropriate comparison group to compare the outcomes of the treated group with, we simulate a sample of identical twins for each individ- 24 To Treat or Not to Treat ual based on their attributes. What we are missing for this sample is the outcome (e.g. recidivism) that would have been observed in the absence of treatment. We simulate this quantity. One of the most consistently documented criminological associations is the link between prior and future criminal activity (Nagin and Paternoster 1991). Individuals who were criminal in the past have a strong likelihood of being criminal in the future. Although criminologists do not speak with one voice about the explanation for this persistence in, and more interestingly desistance from, criminal activity (Piquero et al. 2003), the theoretical debate underlying this linkage centers on the causal interpretation attributed to the link between past and future crime (Nagin and Paternoster 1991). None, however, deny that this link exists. In a similar manner, it is a well established fact that the offending rate evolves non-monotonically—first increasing then decreasing—with age (Farrington 1986). In this research effort, we utilize this link between past and future offending as a way to generate plausible counterfactuals. DATOS provides us information on how many crimes individuals committed one year prior to intake and then during the first year post-intake. A simple comparison of the pre-intake and post-intake offending rate would be an incorrect way of assessing the effects of treatment precisely because of the age-crime link noted above. Hence, despite knowing how much crime individuals committed post-intake, we lack an appropriate counterfactual to compare this number to—how many crimes they would have committed had they not been treated. To estimate this counterfactual, we model the link between the pre-intake offending rate and various individual attributes. Crucial among these are clients’ age and criminal history. Then, we use this model to project what the clients’ offending rate would have been had they aged by a year and increased their criminal history by a particular amount. We augment the criminal history by the observed pre-intake offending rate. This yields a simulated offending rate one year out for an arrestee who moved one year further along the age crime curve and one who had a higher criminal history but did not receive treatment. We Annual offending rate at intake Age Effect AGE AT INTAKE 1 YEAR Treatment effect: Reduction in the annual offending rate from what was anticipated Criminal History Effect The Factual The Counterfactual AGE Age-crime curves (with low and high criminal history) estimated from pre-treatment data Figure 3.1: Graphical depiction of the treatment effects computation. OFFENDING RATE Anticipated annual offending rate at age at intake + 1 years and more criminal history Estimation Strategy 25 26 To Treat or Not to Treat treat this estimate as the simulated counterfactual. We then compare these simulated counterfactuals (for each profile) with the actual offending rates during the one-year follow-up period to compute the treatment effect. Figure 3.1 provides a graphical presentation of how we define the treatment effect. Since the counterfactual is an exact replica (twin) of our sample members so that all attributes are identical (with the only exception being that the twin has aged by one year and has acquired some more criminal history) we control for all observed and unobserved differences. We define the difference between the factual and the counter-factual as the treatment effect. Note that the links between offending and age as well as offending and criminal history are both flexible functional form. As such, depending on who the individual is and how old the individual is, the counterfactual could have a higher, lower or stable. The advantage of this strategy over conventional quasi-experimental designs is that we do not have to adjust the control group to make it comparable to the treatment group: every observable (and unobservable) attribute is identical. Thus, we have generated an expected outcome for the treatment group one year into the future by exploiting the relationship between their current offending behavior, age, criminal history, and other attributes. Expected treatment effects were developed for each offender profile in our synthetic dataset. Moreover, estimates were generated conditional on drug dependence or abuse and conditional on treatment modality. 3.3. SIMULATED ELIGIBILITY As discussed earlier, the drug court is the place within the criminal justice system where long-term, high dosage treatment is most likely to be delivered. While other substance abuse diversions exist in the criminal justice system, only drug courts are designed to oversee the delivery of treatment that is consistently of sufficient duration and dosage to modify behavior as predicted by DATOS. We therefore use the drug court as a model for the delivery of services within the criminal justice system. Estimation Strategy 27 For the purposes of our study, the adult drug court model provides two important pieces of information. The first important piece of information provided by drug courts concerns who is likely to be served if criminal justicebased substance abuse treatment goes to scale. From our synthetic data we are able to create estimates of the benefits of serving different profiles with varying attributes. However, these profiles are artificial, and do not reflect real-world decision-making about the trade-offs between prosecuting substance abusing offenders who are likely to be found guilty and be sentenced, or, deferring prosecution while these offenders are treated in the community. Operational drug courts, however, make explicit policy choices between serving and prosecuting drug-involved offenders based on observable offender attributes. These choices are reflected in choices about who is, and is not, eligible for drug courts. We use this information to estimate a conditional eligibility probability for each profile and assess whether current eligibility rules lead to a net benefit. We then incrementally relax those eligibility rules to simulate whether individuals who are currently not eligible for drug courts can be efficiently treated. Second, drug courts give us a place to observe the expected costs of delivering services to drug-involved offenders. That is, the expansion of substance abuse treatment would likely yield two additional sources of cost—the cost associated with the direct provision of treatment, and the costs of coordinating and monitoring service delivery. In this study, we use extant data on treatment costs, as described later. We also estimate the costs of drug court that are associated with supervising offenders and model those expenses in our cost model. In sum, we do not observe treatment costs from extant drug courts, only the costs of supervision, and derive treatment cost estimates from other sources. The discussion that follows describes the survey data used to stratify profiles by eligibility rules. The section that follows describes drug court cost. 28 3.3.1. To Treat or Not to Treat Drug Court Survey Data Describing Eligibility Criteria Data on adult drug court eligibility were gathered from a survey of 600 drug courts in 2005 administered as part of the Multi-Site Adult Drug Court Evaluation (MADCE) conducted by the Urban Institute, RTI International and the Center for Court Innovation. The web-based survey queried drug court administrators about 70 different characteristics of court operation. Several of these items pertained to their court’s eligibility criteria. Other data include the location, size and demography of their drug court, which we used to identify the probability that a profile would be served by each type of drug court. The survey sample includes all adult drug courts that had been in operation at least one year during FY 2005. In total, 378 (63%) of adult drug courts responded to the survey. For this portion of the study, we sought to estimate the probability that each profile would be eligible for drug court participation. In order to estimate the probability of drug court eligibility for each profile, relevant survey questions were mapped to attributes that correlate to eligibility in the synthetic dataset. In most cases, there was a variable in the survey that measured an identical construct as a variable in the synthetic dataset. For instance, the survey reports that many drug courts exclude as potential participants anyone with prior violence, and prior violence is also observable in the synthetic data. Across all adult drug courts, dozens of eligibility restrictions can be found. Some of those eligibility rules are subjective, and others are not routinely recorded in administrative data. Thus, not all of those attributes are observable in the synthetic data. However, we were able to identify six attributes (number of prior arrests, past violence, past treatment, age, gender, and alcohol abuse) that are used to determine eligibility However, several survey questions were not perfect matches, but a reasonable approximation could be developed. For instance, geographic location is categorized as urban, suburban, or rural in the synthetic data, but was defined as MSA with > 1 million residents, MSA with < 1 million residents and non-MSA Estimation Strategy 29 on the drug court survey. To make these variables comparable, we recoded MSA > 1 million as “urban,” MSA < 1 million was coded as “suburban” and non-MSA was coded as “rural.” Likewise, while the synthetic dataset specified numbers of prior arrests, the drug court survey asked administrators about the number of prior convictions offenders were allowed to have while retaining eligibility. To create a consistent metric, the number of prior arrests was multiplied by 0.66, the weighted average probability of conviction conditional upon arrest, for all index crimes (following Harrell, Roman and Cavanagh 1998). We identified individuals with prior treatment history, who were determined to be ineligible if the individual either failed to successfully complete prior treatment or were past enrollees in drug court (regardless of the outcome). A court was classified as excluding arrestees with an active criminal justice status if they exclude individuals with another pending criminal case, or individuals currently on probation or parole. Table 3.1 describes the percentage of drug courts excluding offenders with each of the six attributes. In total, we estimated eligibility probabilities for all ten attributes used to construct the profiles. No drug court excluded based on race, so those data are not included in table 3.1. Eligibility based on drug use severity (at risk of abuse or at risk of dependence) and the availability of treatment are discussed below. There is substantial variation across offender attributes in the likelihood of being excluded (found ineligible) for drug court. Only a handful of drug courts exclude offenders on the basis of age. Though few drug courts exclude offenders based on number of prior arrests, the overwhelming majority of drug courts (88%) exclude offenders with a violent history. Approximately half of drug courts exclude offenders with prior treatment history and about one third exclude offenders with a history of alcohol abuse. Half of all adult drug courts exclude offenders who are currently on probation or parole or have another open criminal case. The overall percentage of arrestees who are excluded is high, because the presence of a single exclusionary attribute is generally sufficient for an individual to not be accepted into drug court. In addition to limiting the 30 To Treat or Not to Treat Table 3.1: Percent of responding adult drug courts considering individuals eligible for participation, by select attributes Criteria Pr(eligible) Offender’s Age Age <= 20 Age > 20 Offender’s Prior Criminal History Number of Prior Arrests > 2 Number of Prior Arrests > 5 Number of Prior Arrests > 10 Number of Prior Arrests > 20 Current Most Serious Offense Violent Property Drug Other Offender has past violence Offender has past treatment history Offender has active criminal justice status Offender has alcohol abuse problems 0.99 1.00 0.98 0.93 0.85 0.85 0.37 0.94 0.99 0.93 0.12 0.51 0.50 0.66 Source: Urban Institute analysis of MADCE surveys. number of individuals treated in drug court due to eligibility limitations, drug courts also limit the number of people who are treated due to limitations on the number of clients who can participate in a drug court at any one time; we return to this topic below. 3.3.2. Estimating the Size of the Drug-Court Eligible Population The size of the drug court eligible population was estimated in three steps. In the first step, a probability of eligibility for each profile in the synthetic database was estimated. To do this, we estimated the probability that each profile would be found to be eligible for drug court by multiplying the conditional probabilities Estimation Strategy 31 of treatment for each of the ten characteristics along which the synthetic dataset was stratified. Each profile’s probability of drug court eligibility is based on the assumption that each characteristic is statistically independent. So, for example, we would observe that a profile included those with past violence (which had a 88% likelihood of exclusion) and included individuals with alcohol problems (which a 34% chance of exclusion). Thus, the conditional probability of exclusion was 92% on those two characteristics alone. This process was then repeated for the other five eligibility restrictions. The second step in the eligibility estimation process was to weight the probabilities in the first step by the probability that an arrestee at risk of drug abuse and dependence. Based on responses to the MADCE survey, all drug courts were assumed to accept those at risk of drug abuse (those offenders with less serious substance abuse problems) as eligible for drug courts. Thus, for this group, there was no decrease in the probability that a profile was eligible. However, many drug courts (61.5%) exclude those with the most serious drug problems. So, we multiply the probability that a profile is eligible by 38.5% for all profiles in order to estimate the number at risk of drug dependence who are eligible for drug court participation. The third step is to adjust these probabilities by a scaling factor to account for the limited number of drug court treatment slots. That is, even though most arrestees at risk of drug abuse or dependence are ineligible for participation in drug courts based on the eligibility criteria described above, there are still more individuals eligible for drug court than there are drug court placements. On average, we estimate that 16% of profiles for arrestees at risk for drug abuse are eligible for drug court. We estimate that 6% of profiles for arrestees at risk for dependence are eligible for drug court. Though there is considerable variation, for both groups more than half of profiles have less than a 10% probability of drug court eligibility. Combining both at risk populations, the weighted average probability of drug court eligibility was 7%, for a total of 109,921 drug court eligible arrestees. Again, there are too few drug court slots to treat all of those 32 To Treat or Not to Treat 109,921 eligible, so we must scale down the eligible number into order to estimate the size of the population that is both eligible and for whom a treatment slot is available. To complete the third step and estimate the number of drug court eligible arrestees, we first estimated the number of drug court slots (109,921) using the survey of drug court administrators. Next, we multiply the probability that a profile is eligible in the second step, by the probability that an eligible profile would actually secure one of the scarce drug court slots. We are then able to make two important estimates—the number of individuals who are eligible for drug court, and the number of eligibles who actually receive treatment under a drug court regime. Among drug courts that responded to the survey, the average number of participants currently enrolled was 93. In order to devise a national estimate of the number of drug court clients annually enrolled, we assume that 93 is the average number of drug court clients. Thus, we assume that the average drug court that responded to the survey is the same as average drug court that did not respond to the survey. Rossman et al. (2008) reports that drug courts from larger jurisdictions were more likely to respond than drug courts from smaller jurisdiction, so this is probably a conservative assumption. Finally, the number of enrollees per court per year (93) was multiplied by 600, which is the population of adult drug courts in operation in 2005 that had been in operation for at least one year. Rossman et al. (2008) report substantial efforts were made to identify all drug courts meeting these criteria, and that it is likely that some of the non-responding drug courts were not currently in operation, so this is also probably a conservative assumption. Thus, we estimate that there were 55,365 adult drug court participants in 2005. As shown in table 3.2, there were substantially more arrestees eligible for drug court than there were treatment slots. To estimate the number of at-risk arrestees who were actually treated in drug court, we weight the eligible population by the probability a slot would be available. Since the number of available 33 Estimation Strategy Table 3.2: Population of arrestees eligible for drug courts Size of the at-risk population Size of the drug court-eligible population Estimated number of drug court slots Percentage of drug court eligible arrestees who were treated at drug court Percentage of all arrestees who are eligible and treated at drug court Urban Rural Suburban Overall 525,224 39,238 485,223 36,250 460,891 34,433 1,471,338 109,921 18,998 25,106 11,261 55,365 48.4% 69.3% 32.7% 50.4% 3.6% 5.2% 2.4% 3.8% Source: Urban Institute analysis of MADCE surveys. slots and the drug court eligible population both vary by the size of their jurisdiction, we calculate these probabilities by type of the jurisdiction. In summary, of the almost 1.5 million arrestees at risk of drug abuse or dependence, 109,921 (about 7%) met drug court eligibility requirements. Of the 109,921 eligibles, approximately half (55,364) were actually enrolled in a drug court program. In aggregate, just 3.8% of the at-risk arrestee population was treated in drug court. 3.3.3. Estimating the Number of Individuals Currently Receiving Drug Treatment In order to estimate the cost-effectiveness of treating arrestees, we must determine what type of treatment would be received. There are no existing estimates of the number of individuals in drug court who receive each of the four treatment modalities considered in this paper. As such, we relied on estimates of the number of individuals receiving each of the four treatment modalities nationwide, irrespective of delivery via drug court. To create these estimates, we multiplied the number of drug court slots by the proportion of individuals na- 34 To Treat or Not to Treat Table 3.3: Estimated number of individuals receiving each treatment modality in drug courts Long-term Residential Short-term Residential Outpatient Methadone Outpatient Drug-Free Total Urban Rural Suburban Overall 1,221 2,339 3,396 12,042 18,998 1,613 3,091 4,488 15,913 25,105 724 1,386 2,013 7,138 11,261 3,558 6,816 9,897 35,093 55,364 Source: Urban Institute analysis of MADCE surveys. tionwide who receive each of the four treatment modalities. Thus, we implicitly assume that treatment delivered through drug courts follows the same distribution of treatment modalities nationally. Table 3.3 displays these results by regional type. Across all three regional types, outpatient drug-free therapy was, by far, the most common service offered to substance-involved individuals, comprising 63% of treatments. Methadone (18%) was the second most common treatment followed by short-term residential (12%) and long-term residential (6%). As we incrementally relax the eligibility restrictions to test the cost-effectiveness of different policy regimes later in this paper, the same approach is used. So, for example, when we consider the cost-effectiveness of treating all drug court eligibles, regardless of whether there is currently a treatment slot, we would apply the same weighting scheme as described above to estimate the distribution of those drug court eligibles across treatment modalities. 3.4. COST AND BENEFIT ESTIMATES In the language of cost-benefit analysis, the eligibility estimates described in the preceding section are quantities. That is, first we estimate how many individuals would be found in each policy regime (e.g., where we could consider the costeffectiveness of treating only those who are eligible for drug court, all who are Estimation Strategy 35 eligible for drug court regardless of treatment availability, all at-risk arrestees, or some other combination). Then, we estimate how many crimes would be averted if those policy regimes were implemented. The simulation results are described in the next section. Once we have estimated these quantities they must be multiplied by the price of each outcome to estimate the cost or benefit that results. That is, the cost of treating any given profile is the product of the price of the outcomes and the number of outcomes. Price therefore has two components. The first component is the value of the resources that must be added in order to implement that policy regime (this is commonly referred to as the cost, but in cost-benefit parlance the net cost refers to the product of prices and quantities). In our models, there are two prices associated with each policy regime (e.g., two kinds of resources consumed in administering each policy). There are the prices associated with each of the four treatment modalities and there are the prices associated with the criminal justice system’s oversight of treatment. We estimate the first set of prices from extant data for each of the four treatment modalities. We estimate the second set of prices from a survey of a small sample of drug courts undertaken as part of this study and the MADCE. It is worth noting that the drug court prices are just the price of criminal justice oversight. Treatment prices are not included in the estimate of the price of criminal justice oversight, they are estimated separately. Second, there are the prices associated with changes in the number of crimes. That is, if a policy regime results in fewer crimes, than the price is the savings to private citizens (from reductions in the number of victimizations) and to the criminal justice system (from reductions in the number of offenses investigated and the number of offenders processed through the system). If the policy leads to additional crimes, than the prices will reflect the loss to private citizens who experience more victimizations and to the criminal justice system which must investigate more crime and process more offenders. We label these prices as benefits, and note that depending on the outcome of the simulations, the benefits may be positive or negative. 36 To Treat or Not to Treat In the section that follows, we first describe how the costs of the DATOS treatment modalities were estimated. This is followed by a discussion of the estimation of costs of drug court administration. Finally, we describe how the benefits of changes in the policy regimes from reductions (or increases) in the number of crimes were estimated. 3.4.1. Treatment Price Estimates The cost of each new policy regime is divided into two categories: (1) the cost of providing four different drug treatment modalities (long-term residential treatment, short-term residential treatment, outpatient methadone treatment and outpatient drug-free treatment) and (2) the administrative cost of drug court as a conduit for delivery of these treatment services. Data on the cost of treatment modalities is drawn from DATCAP, a data collection instrument and interview guide designed in the early 1990s to assess treatment programs along a set of standard accounting and economic principles. Resource categories include personnel, supplies and materials, contracted services, buildings and facilities and other miscellaneous items (Bradley French and Racal 1994; Roebuck French and McClellan 2003). Roebuck, French and McClellan gathered information on 53 outpatient and 32 residential (inpatient) programs between 1993 and 2002, calculating average costs for a number of adult treatment modalities including longand short-term residential treatment, outpatient methadone treatment and outpatient drug-free treatment. Table 3.4 details the average costs for each of these modalities. The cost of methadone treatment (over an average of 99 weeks) is $8,609. The cost of long-term residential treatment (20 weeks) is $16,448 and the cost of short-term residential treatment (3 weeks) is $3,287. All costs have been translated into 2007 dollars, using the Consumer Price Index as a deflator. 37 Estimation Strategy Table 3.4: Costs associated with four treatment modalities Average length Economic Price per of stay (weeks) treatment episode Long-term Residential Short-term Residential Outpatient Methadone Outpatient Drug-Free 20 3 99 17 $16,448 $3,287 $8,609 $3,557 Source: Roebuck, French and McClellan gathered information on 53 outpatient and 32 residential (inpatient) programs between 1993 and 2002. 3.4.2. Drug Court Price Estimates To estimate the price of treatment delivery using a drug court as the delivery mechanism, we conducted phone interviews with fifteen drug court administrators located in New York, Florida, South Carolina, Illinois and Washington State.1 In order to estimate personnel costs, drug court administrators were asked to provide fully-loaded salaries of personnel involved in drug court who were not directly involved in the provision of treatment. In addition, administrators were asked to estimate the percentage of each staff member’s time that was allocated to drug court. The costs of monitoring offenders was estimated as the product of the number of drug tests administered per offender by the number of offenders given the average length of enrollment in drug court. Finally, the costs of offender fines and sanctions were considered. These costs included fees and fines paid for by drug court clients as well as the cost of jail days assigned as a punishment for failure to comply with court rules. Table 3.5 lists the per-individual costs of drug court, excluding the cost of treatment. These costs include (1) personnel not directly involved in treatment, e.g. not including drug court clinical staff, (2) drug and alcohol testing and fees and (3) fines and jail terms during the course of drug court. The costs of treatment are not included in these estimates. Capital costs are also not included 1 This portion of the study was done in conjunction with the MADCE. The fifteen sites represent a sample of the 23 drug courts participating in that study (67%). 38 To Treat or Not to Treat Table 3.5: Administrative costs for drug courts County Average Price Osceola (FL) Volusia – Deland (FL) Volusia – Daytona (FL) Fulton (GA) York (SC) Auburn (NY) Wayne (NY) Kane (IL) Finger Lakes (NY) (misdemeanor) Finger Lakes (NY) (felony) Cook (IL) Syracuse (NY) Thurston (WA) King – Seattle (WA) King – Kent (WA) $2,313 $9,826 $9,826 $4,003 $3,160 $5,516 $8,390 $4,087 $3,528 $7,260 $5,071 $1,477 $6,905 $3,830 $3,830 Weighted (by size) Average $4,060 Source: Urban Institute survey of drug court administrators. in these estimates. After weighting to account for variation in drug court size, the average price of drug court is $4,060. Table 3.6 describes the price of four treatment modalities that will be used to simulate the costs of going to scale. These are just $4,060 (from table 3.5) added to the cost estimates provided in table 3.4. We note that it is possible that our price estimates may overestimate the true average price of treatment if drug courts were substantially expanded. Many of the costs of treating drug court clients are fixed and do not vary (or vary minimally) with the size of the drug court. For example, a drug court is likely to have a single drug court coordinator and a single judge even if the population of the court increases substantially. In the presence of fixed costs, average costs will decline as the number of clients enrolled increases. Since increasing the scale of 39 Estimation Strategy Table 3.6: Price for four treatment modalities Modality Long-term Residential Short-term Residential Outpatient Methadone Outpatient Drug-Free Price $20,508 $7,347 $12,669 $7,617 Source: Urban Institute analysis of Roebuck, French and McClellan and MADCE survey data. drug court entails increasing drug court enrollments, the cost of treating drug court clients is not expected to increase linearly. Instead, the cost per client is expected to diminish at the margins. Thus, the real price of drug court plus treatment may be lower if the average size of the drug court increases substantially. However, we cannot model this change in price since we cannot observe this in our data (even the largest drug courts in our sample are smaller than the average drug court would have to be if every drug involved arrestee were eligible for treatment). This results in a conservative bias if any. That is, if a change in policy regime yields a positive benefit to cost ratio it will have done so using what are likely to be the upper bound of expected prices. If prices were actually lower, the benefit to cost ratio would increase. 3.4.3. Estimates of the Benefits from Crime Reduction The purpose of treating drug-involved arrestees is to reduce anti-social behaviors, including crime. If this occurs, the burden on the public and governmental agencies from offending will be reduced. Thus, the critical benefits to consider in our models are those associated with reduced offending. There are two potential groups of beneficiaries of reduced offending: (1) potential victims (private citizens) whose welfare is enhanced as the number and severity of offending by drug involved offenders is reduced and (2) public agencies (and taxpayers) which 40 To Treat or Not to Treat will consume fewer resources in the investigation and processing of offenders if there are fewer crimes committed as a result of treatment. Estimating the benefits to public agencies from reduced crime is relatively straightforward. First, we estimate the average unit costs to two public agencies— police agencies and departments of correction—of investigating, arresting, and incarcerating offenders. Data on the cost of arrest were drawn from estimates in Roman, Woodard, Harrell and Riggs (1998). Data on the cost of supervision come from a variety of sources. Data on the probability of conviction given arrest and the probability of being assigned prison, jail or probation given a conviction comes from DuRose and Langan (2004). Data on the cost of state prison comes from the Bureau of Justice Statistics (Stephan 2001). Data on the daily cost of jail is derived from estimates in Roman and Chalfin (2006). We repeat the same process for the estimation of benefits to private citizens. Benefits to private citizens occur when the number and/or severity of crimes are reduced. To estimate these benefits, we first estimate the unit price of a victimization. The unit price of a victimization has two components: tangible and intangible costs. Tangible costs of crime include direct costs of victimization such as medical bills, rehabilitation costs, and lost wages from being unable to work and are estimated from data on victim injuries. Intangible costs include psychological harm associated with victimization, including fear, pain, and suffering and are estimated using the sizes of jury awards made by civil juries. We use extant estimates of the costs of victimization to estimate the benefits of drug treatment delivered via drug court. The cost of harms to victims for aggravated assault, other assaults, robbery, burglary, larceny/theft, stolen property offenses, fraud and drug offenses are drawn from five sources extant economics of crime literature: Cohen (1988), Cohen, Miller and Rossman (1993), Miller, Cohen and Wiersma (1996), Rajkumar and French (1997) and McCollister (2007). In order to reduce the degree to which final results are sensitive to changes in the choice of monetized harms, for each offense category, an average of extant estimates was taken. These estimates are reflected in the column entitled ‘Cost to Victims’ in 41 Estimation Strategy Table 3.7: Cost of offending, pre-sentence Aggravated Assault Robbery Burglary Larceny/Theft Stolen Property Drug Offenses Cost to Cost of Pre-sentence Cost Victim Arrest Detainment Adjudication $47,009 $30,253 $2,643 $819 $819 $31 $2,603 $2,563 $2,563 $675 $675 $675 $1,080 $1,080 $1,080 $1,080 $1,080 $1,080 $5,502 $5,502 $5,502 $2,751 $2,751 $5,502 Source: The costs of crime to victim, both tangible and intangible are estimated as the average of estimated prices from Cohen (1988), Cohen, Miller and Rossman (1993), Miller Cohen and Wiersma (1996), Rajkumar and French (1997). Estimates of the cost of arrest are from Roman, Woodard, Harrell and Riggs (1998). For offenses not reported, we assume an arrest cost of $675. Data on the length of pre-sentence detainment come from Roman, Woodard, Harrell and Riggs (1998). These data are not available by offense. Roman, Woodard, Harrell and Riggs (1998) report a per minute trial cost of $3.50. We assume a mean adjudication length of 40 hours for murder and rape; 10 hours for larceny and stolen property offenses and 20 hours for all other offenses. Data on the daily cost of jail are from Roman and Chalfin (2006). table 3.7. The expected costs of supervision have been expressed in net present value, discounted at a rate of 5% per annum. Next, we estimate the cost of incapacitating convicted offenders. First, we calculate the likely disposition for a convicted offender (table 3.8), since the costs of probation, jail and prison vary, following Durose and Langan (2004). We then calculate the expected time served for each sentence type (table 3.9). These expected sentence lengths are then multiplied by the average expected percentage of the sentence to be served to yield the expected length of stay, by sentence type, by crime (not shown). The data in tables 3.7, 3.8 and 3.9 can then be combined in table 3.10, to show the total expected cost of crimes to both victims and public agencies. Costs at each stage of processing are multiplied by the probability that the stage had a positive outcome (e.g., the costs of arrest are only counted for those cases where 42 To Treat or Not to Treat Table 3.8: Probability that an offender is sentenced to probation, jail, or prison Prison Aggravated Assault Robbery Burglary Larceny/Theft Stolen Property Drug Offenses Jail Probation 42% 29% 71% 15% 46% 26% 36% 31% 36% 31% 38% 27% 29% 14% 28% 33% 33% 35% Source: Data on the probability of each type of sentence is developed from Durose and Langan (2004). Table 3.9: Estimated number of months an offender is sentenced to probation, jail or prison, and total sentencing costs Probation (mos) Aggravated Assault Robbery Burglary Larceny/Theft Stolen Property Drug Offenses 54 91 50 34 34 45 Jail Prison % Sentence (mos) (mos) Served 7 11 7 6 6 6 39 52 40 36 36 36 66% 58% 49% 52% 52% 43% Cost of Sentence $22,736 $43,932 $20,378 $13,062 $13,062 $11,609 Source: Data on the cost of incarceration in state prisons comes from Stephan (2001). Data on the daily cost of jail comes from Roman and Chalfin (2006). $47,009 $30,253 $2,643 $819 $819 $803 $31 56% 26% 13% 18% 16% 16% 0.025% 56% 26% 13% 18% 18% 43% 20% $2,603 $2,563 $2,563 $675 $675 $675 $675 $5,502 $5,502 $5,502 $2,751 $2,751 $5,502 $1 $22,736 $43,932 $20,378 $13,062 $13,062 $13,593 $1 Cost of Cost of Cost of Arrest Adjudication Sentencing $54,436 $36,066 $4,233 $2,095 $2,095 $2,280 $33 Total Cost to Society Source: Data on the probability a committed crime is reported is estimated from Sourcebook of CJS 2002, p 275 Table 4.19. Probability of an arrest is derived from Crime Reported to Police (BJS). Data on the probability of each type of sentence and the percentage of time served come from Durose and Langan (2004). Aggravated Assault Robbery Burglary Larceny/Theft Stolen Property Fraud Drug Offenses Cost to Prob of Prob of Victims a Report an Arrest Table 3.10: Estimates of the total cost to society. Estimation Strategy 43 44 To Treat or Not to Treat an arrest was made). The first column of table 3.10 is the cost to victims of a crime, which is the same data as shown in table 3.7, and is the total cost to private citizens from a single criminal incident of each type. The other columns in table 3.10 describe the costs to the criminal justice system from each crime. Unlike private costs, public costs vary by the likelihood that the average case proceeds to each subsequent stage of processing. The total costs to the criminal justice system then are the sum of the conditional probability of an event (multiplied by the price of each event). That is, the criminal justice costs only occur if an event occurs; for instance, if a crime is not reported, than there are no criminal justice system costs. So, for instance, the total cost to the criminal justice system of a sexual assault is $4,464 multiplied by the probability that a crime is reported (43%) multiplied by the probability that an arrest is made, for a total of $845. The same process is applied to expected cost of sentencing, so the average cost of sentencing per committed crime is 67, 038 ∗ 0.43 ∗ 0.44, or $12,864. Generally speaking, as the seriousness of the crime decreases, the proportion of the social costs that fall on public agencies (as opposed to victims) decreases. For example, while costs to victims comprise 87% of the cost of an aggravated assault, they comprise just 38% of the cost of a theft. Thus, given that the effect sizes associated with the various treatment modalities are positive, potential victims are expected to reap the greatest benefits of expanding drug treatment to offenders whose offending is primarily violent. Public agencies are expected to see the most savings if drug treatment is expanded to offenders whose offending is comprised primarily of drug and property offenses. Next, we use data from the impact analysis to estimate the number of arrests and convictions that were prevented by drug treatment via drug court. 3.5. KEY ASSUMPTIONS AND CAVEATS There are several assumptions concerning the data and the model that should be noted. First, the treatment effects we have computed from the DATOS Estimation Strategy 45 data are based on underlying data that was reported regarding arrests (not offenses). Not every crime is reported and not every reported crime is cleared. Therefore, prior to our analysis, we computed a scaling factor that converts the number of arrests into the number of offenses. This scaling was based on available information on crime reporting rates and clearance rates. We obtained data for the clearance rates from the 2003 Sourcebook of Criminal Justice Statistics (http://www.albany.edu/sourcebook/) by gender and race groups. We obtained the reporting rate from Hart and Rennison (2003). Details on the conversion factors are available from the author upon request. Second, the prevalence estimates we report are for potential clients that would accept treatment if offered. In our experience with substance abuse diversion and alternative to incarceration programs suggests that only those who are likely to be found guilty are likely to enroll (since a plea is generally required for admittance). Thus, we need some way to identify potentially guilty clients. Every year a proportion of arrests will be declined for prosecution and every year a proportion of defendants will not be convicted. Hence, we created a scaling factor that converts the total number of arrestees for a particular profile into the total number of arrestees who are probably guilty. To do so, we used data on conviction rates and declination rates. These data were obtained and used at the offense type level. Conviction rates were obtained from the 2003 Sourcebook of Criminal Justice Statistics (http://www.albany.edu/sourcebook/) and declination rates were obtained from Chapter 2 of the 2003 Compendium of Federal Justice Statistics (http://www.ojp.gov/bjs/abstract/cfjs03.htm). Details on these data are also available from the authors upon request. Third, in both DATOS and the NSDUH, several of the questions needed to compute a drug dependence or abuse score were either not available or were worded slightly differently. For example, the question relating to whether or not respondents had used drugs (or alcohol) to alleviate feelings of sadness, anger, or boredom was not available. In our analysis, we have identified the closest proxy for the variables used in ADAM. Despite the slightly different definitions, we 46 To Treat or Not to Treat confirmed that our definitions did not create large imbalances in the data sets. For example, across all data sets used, we found that, of respondents at risk of drug dependence or abuse, the number at risk of dependence were about three times as large as the proportion of respondents at risk of abusing drugs. Fourth, once interpolated onto the synthetic data, we combine and transform our estimates in various ways. This necessitates the combination of their variances as well. Combining the variances of two or more random variables is straight forward if we can make the assumption that they are independent. In our analysis, since the estimated variances are conditional on profile attributes, we need only make the less restrictive assumption of conditional independence. In other words, we assume that for all individuals of a particular profile, the variation in, say, the prevalence of drug dependence is unrelated to variation in, say, the expected treatment benefits. We recognize that this is a limiting assumption and that some of our variance estimates may be biased (either too small or too large). Unfortunately, combining variance estimates across several thousand profiles would be extremely complicated if we were to permit there to be covariances. Fortunately, the point estimates of all quantities should be unaffected by this assumption. Fifth, the results are very sensitive to the selection of a price of crime to victims. We modeled the data using Cohen et al. (1993) and Rajkumar and French (1997) and found an overall benefit to cost ratio of 2.73:1 for the current drug court regime. We re-ran the models using a blended estimate of the five most cited estimates of the costs of crimes to victims and this results in a much smaller estimate of the benefits of the current drug court regime of 2.14:1. Although none of results changed, we note that the estimates are sensitive to price. Finally, we note that there are three kinds of transformations we perform on estimated quantities in the synthetic data that necessitate computing new variances. These include scaling, summing and taking products. A description of our approach can be found in the mathematical appendix. Chapter 4 Findings In this section, we discuss our findings. We begin with a discussion of the estimated prevalence of drug abuse and drug dependence, which constitute the population of potential treatment clients, and then present findings regarding the number of crimes avertable by going to scale. 4.1. PREVALENCE Table 4.1 presents our estimates of the number of individuals arrested annually who are either at risk of drug dependence or of abusing drugs. We estimate that roughly 1.15 million potential clients are at risk of drug dependence and roughly 322,000 are at risk of abusing drugs. Together this constitutes about 1.47 million arrestees who are probably guilty and at risk of drug dependence or abuse. The distribution of client attributes varies by the severity of drug problems. For instance, 23% of those at risk of dependence are female, compared to 18.5% of those at risk of abuse. The biggest difference between those at risk of abuse and dependence concerns prior treatment. Slightly less than 40% of those at risk of dependence have a prior treatment episode, compared to slightly more than 60% of those at risk of abuse. The other notable difference is that 44% of those at risk of dependence also have problems with alcohol, compared with only 47 48 To Treat or Not to Treat 37% of those at risk of abuse. Since these latter two differences are currently used to determine eligibility for criminal justice system-based treatment, those differences will have important implications for the effectiveness of different eligibility regimes. This is discussed in greater detail in the following section. Our estimates are based on a population of potential clients for criminal justice system-based treatment, and includes only those who are at risk of dependence or abuse, and likely to be found guilty. We are not attempting to estimate the prevalence of drug use among arrestees. Thus, we expect our estimates of the number of potential clients (those at risk of drug abuse and drug dependence) to be less than the total number of drug users in the arrestee population for two reasons. First, there are some arrestees who use drugs, and/or are under the influence at the time of their arrest, but who are not at risk of dependence or abuse. Second, not every arrestee is probably guilty, and therefore eligible for treatment under court supervision. Brecht et al. (2003) report findings from a study testing similar hypotheses, but focuses on drug use among arrestees, rather than just those at risk of abuse and dependence, and who are probably guilty. Brecht et al. finds that nationwide nearly 6.4 million arrestees are drug users. Moreover, they find that, among arrestees nationwide, the number of male drug users is nearly three times the number of female drug users. In our analysis, the number of potential clients are lower but we find a similar break down by gender (at least among clients at risk of drug dependence). In a similar way, they also find that the prevalence of drug use remains fairly stable among arrestee of all ages. Our analysis mirrors this finding, albeit on a smaller scale. 4.2. CRIMES AVERTABLE BY TREATMENT Next, we estimate the reductions in recidivism rates to be expected by treating potential clients under various modalities. We find that treatment in each of the domains substantially reduces recidivism. For those at risk of drug dependence, long-term residential reduces recidivism by 34%, short-term inpatient by 19%, 49 Findings Table 4.1: The estimated prevalence of potential clients nationwide. Clients at risk of dependence Low Mean High Clients at risk of abuse Low Mean High All 1,145,034 1,149,019 1,153,004 320,090 322,320 324,550 Gender Male 878,933 882,668 886,403 260,674 262,833 264,992 Female 264,961 266,350 267,740 58,928 59,487 60,046 Race Black 417,079 419,299 421,518 120,907 122,109 123,311 White 388,685 390,764 392,842 89,852 90,760 91,667 Other 336,381 338,956 341,532 107,807 109,451 111,096 Current Offense Violent 191,877 193,387 194,898 74,962 75,909 76,856 Property 313,127 315,231 317,334 64,899 66,102 67,305 Drug 387,348 389,714 392,079 97,667 98,824 99,981 Other 248,795 250,687 252,579 80,348 81,485 82,622 Any Violent History Violent History 616,959 620,136 623,313 169,804 171,526 173,248 No Violent History 526,477 528,883 531,289 149,376 150,794 152,212 Any Treatment History Treatment History 692,490 695,608 698,727 125,848 127,407 128,966 No Treatment History 450,930 453,411 455,891 193,318 194,913 196,508 Any Alcohol Problems Alcohol Problem 638,779 641,810 644,841 200,606 202,405 204,205 No Alcohol Problem 504,622 507,209 509,796 118,597 119,915 121,232 Geographic Location Rural 381,142 383,590 386,039 100,192 101,633 103,073 Urban 383,593 385,815 388,037 74,171 75,076 75,982 Suburban 377,390 379,613 381,837 144,169 145,611 147,053 Current Age Age: 20 170,093 171,410 172,726 48,167 48,858 49,548 Age: 25 177,994 179,336 180,679 47,670 48,335 49,000 Age: 30 177,591 178,985 180,379 48,472 49,152 49,832 Age: 35 170,339 171,767 173,196 46,648 47,334 48,020 Age: 40 157,345 158,962 160,579 43,025 43,896 44,767 Age: 45 146,106 147,829 149,552 42,460 43,566 44,673 Age: 50 139,063 140,730 142,397 40,112 41,179 42,246 Criminal History Criminal History: 0 166,745 168,176 169,607 59,946 60,804 61,662 Criminal History: 2 178,123 179,582 181,040 62,602 63,456 64,310 Criminal History: 5 198,376 199,861 201,347 66,821 67,679 68,536 Criminal History: 10 241,259 242,791 244,323 71,517 72,390 73,263 Criminal History: 20 355,934 358,609 361,283 56,572 57,991 59,409 Source: Urban Institute analysis. 50 To Treat or Not to Treat outpatient methadone by 20%, and outpatient drug free by 30%. Note that the reductions are no uniform by crime type, and in particular the most serious have the highest rates of recidivism. For in stance, only long-term residential reduces recidivism for aggravated assault and robbery, and outpatient drug free actually increases the number of aggravated assaults. For less serious crimes with higher prevalence rates, the reduction in recidivism is generally large. For those at risk of drug abuse, recidivism reductions are large, but the 27% reduction in recidivism is not quite as great as the reduction in recidivism for those at risk of drug dependence. The effectiveness of different modalities is slightly different as well. Outpatient drug free is the most effective modality, reducing recidivism by 33%. Long-term inpatient reduces recidivism by 27%, short-term inpatient by 20% and outpatient methadone by 16%. The pattern of offending reduction is generally similar for those at-risk of drug abuse as it is for those at risk of dependence. One minor difference is that the recidivism reductions for the most serious offenses is positive for all modalities, although the recidivism reductions are very small, generally 1% or 2%. The findings here generally corroborate what has been found elsewhere in the literature. For example, Holloway, Bennett and Farrington’s (2006) metaanalysis results found that drug treatment may reduce offending by 29-36%. More interesting, however, are findings regarding the total number of crimes avertable by treating potential clients. Table 4.2 and table 4.3 provide these estimates. A cautionary note on reading these tables. The modalities are conditioning variables. Hence, the first three columns in table 4.2, for example, produces the estimates (and upper and lower confidence bounds) of the expected crimes avertable if every potential client at risk of drug dependence was treated under Modality 1 (long term residential) or under Modality 2 (short term inpatient). As such the number cannot be summed or aggregated across modalities. Moreover, the number of all non-drug crimes avertable is not an aggregation of all sub-crime types. Respondents were asked to identify the number of times Source: Urban Institute analysis. 689,274,887 3,809,215 44,102 338,054 734,169 -25,553 48,933 -72,923 162,791,624 166,526,868 170,262,111 1,488,310 1,522,185 1,556,060 119,675 126,802 133,929 222,233 231,258 240,283 131,204 139,992 148,780 -25 1,354 2,732 -44,294 -40,561 -36,828 60,135 63,523 66,911 679,518,218 3,705,355 18,079 315,206 716,014 -28,147 43,212 -80,103 260,065,953 2,919,438 210,188 157,162 166,007 101,948 11,249 1,933 Modality 2: Short Term Inpatient Drug 669,761,550 All Non-Drug 3,601,495 Fraud -7,945 Burglary 292,359 Larceny 697,859 Robbery -30,741 Aggravated Assault 37,490 Other Assaults -87,284 255,738,040 2,860,013 203,498 149,074 155,715 97,549 9,622 -542 High 251,410,127 2,800,589 196,808 140,987 145,423 93,150 7,995 -3,018 High Clients at risk of abuse Low Mean Modality 1: Long Term Residential Treatment Drug 1,012,217,240 1,024,164,494 1,036,111,748 All Non-Drug 6,967,517 7,048,988 7,130,460 Fraud 1,318,702 1,349,364 1,380,026 Burglary 912,634 942,097 971,561 Larceny 1,766,153 1,797,942 1,829,732 Robbery 148,311 154,299 160,287 Aggravated Assault 97,979 101,884 105,788 Other Assaults -19,586 -12,826 -6,067 Clients at risk of dependence Low Mean Table 4.2: Estimated number of crimes avertable annually by treating potential clients under inpatient treatment modalities. Findings 51 52 To Treat or Not to Treat they were arrested for any crime and then subsequently the number of times they were arrested for particular crime types. The estimates can, however, be summed across risk criteria. For example, the total number of all non-drug crimes avertable by treating offenders at risk of drug dependence or abuse under modality 1 (long term residential treatment) is a little over 9.9 million (7,048,988 + 2,860,013). Similarly, we expect that roughly 1.27 billion drug related crimes could be averted by treating all offenders at risk of drug dependence or abuse using long term residential treatment. Benefits can be expected vary, of course, by treatment modality. For example, long term residential treatment is the most effective treatment modality— would avert the most crimes if everyone at risk of dependence or abuse was treated under it. However, the modality effectiveness rankings are crime typespecific. Notably, the lowest number of drug crimes are averted if all potential clients are treated under modality 4 (outpatient drug free). On the other hand, outpatient drug free is an effective modality for averting non-drug crimes. In fact, if all offenders at risk of drug dependence were treated under this modality, roughly 6.7 million non-drug crimes would be averted. Only 3.7 million and 3.3 million non-drug crimes would be averted if these same individuals were treated under short term inpatient and outpatient methadone modalities respectively. There are also several crime types for which the evidence is insufficient to conclude any crime reductions. For example, among offenders at risk of dependence, it is unclear if short term inpatient treatment will reduce any frauds or whether outpatient methadone will reduce any aggravated assaults. Similarly, among offenders at risk of abusing drugs, it is unclear if short term inpatient treatment or outpatient drug free can avert any robberies. 4.3. COST-EFFECTIVENESS OF TREATING CURRENTLY ELIGIBLE DRUG COURT PARTICIPANTS The crimes avertable by treating individuals will vary depending on the subgroup that is selected for treatment. Additionally, not all individuals can or will Source: Urban Institute analysis. 127,163,645 892,080 16,139 94,193 167,853 5,886 -13,387 111,245 134,805,945 925,981 22,417 102,094 177,491 7,357 -11,280 120,487 354,283,621 363,442,051 159,544,034 163,696,423 167,848,811 6,779,782 6,901,924 1,527,269 1,562,353 1,597,436 264,776 282,627 82,356 88,058 93,761 173,516 188,167 136,203 144,134 152,065 1,200,833 1,225,470 82,118 87,846 93,574 24,604 27,818 -1,213 407 2,026 -7,045 -2,532 11,548 13,244 14,940 317,778 326,508 135,005 143,929 152,852 119,521,345 858,179 9,861 86,291 158,215 4,415 -15,494 102,003 Modality 4: Outpatient Drug Free Drug 345,125,191 All Non-Drug 6,657,640 Fraud 246,925 Burglary 158,866 Larceny 1,176,197 Robbery 21,390 Aggravated Assault -11,558 Other Assaults 309,049 593,763,103 3,455,760 66,844 471,383 989,094 20,235 6,219 571,363 High 578,123,548 3,370,857 48,990 447,468 958,940 15,423 2,852 544,708 Clients at risk of abuse Low Mean Modality 3: Outpatient Methadone Drug 562,483,993 All Non-Drug 3,285,954 Fraud 31,135 Burglary 423,554 Larceny 928,786 Robbery 10,611 Aggravated Assault -516 Other Assaults 518,054 Clients at risk of dependence Low Mean High Table 4.3: Estimated number of crimes avertable annually by treating potential clients under outpatient treatment modalities. Findings 53 54 To Treat or Not to Treat be treated under the same treatment modality. The associated costs and benefits of treating those individuals will also vary. Therefore, to study the effectiveness of treating drug involved offender, we run six simulations models. In these simulations, we begin with the full sample in table 4.1, and then put restrictions on the sample to reflect the current policy regime. The first two describe the currently eligible population for drug courts, and are discussed below. The next four describe the effects of relaxing existing eligibility criteria, and these are discussed in the next section. Table 4.4 describes the first simulation which describes the effectiveness of the currently eligible drug court population. That is, although there are almost 1.5 million individuals arrested each year who are at risk of drug abuse and dependence, and who are likely to be found guilty, only a fraction of those arrestees are currently being treated. Thus, we begin our simulations by estimating the size of the population that is currently being treated by the criminal justice system. Since there are no national data to identify who is currently being treated, we begin by identifying adult drug courts as the mechanism in the criminal justice system that is most likely to deliver the long-term intensive treatment for which effect sizes are available from the DATOS study. We believe adult drug courts are a reasonable population to study, since any large scale expansion of treatment in the criminal justice system will require a significant infrastructure to manage, and the adult drug court management model is the most reasonable candidate. Thus, we begin in table 4.4 by estimating the size of the population that is currently treated in adult drug courts. We find that there are about 55,000 individuals treated annually, about 32,000 of which are at risk of dependence, and 23,500 are at risk of drug abuse. Our estimates suggests that by far the most common treatment modality is out-patient drug free, which is consistent with the drug court literature. In total, we estimate that about $515 million dollars is spent annually to treat those drug court clients. We find that there is a substantial reduction in criminal offending that results 1.84 248,627,369 1.95 281,675,271 2.56 339,070,917 2.08 554,651,149 2.21 624,223,730 2.34 693,794,936 2.71 2.86 375,596,361 412,119,665 1.73 215,580,233 Net BC Ratio Net $ Saved 174,003 35,398,083 24,247 19,691 35,950 -322 -2,088 279 656,080,414 1,105,256,814 1,136,696,776 1,168,136,740 21,740,872 49,687,880 52,484,962 55,282,088 46,663,534 71,662,023 77,506,484 83,351,072 15,131,850 69,369,787 72,342,089 75,314,391 -11,681,056 -19,178,456 -15,396,575 -11,615,055 -86,997,983 -199,585,945 -184,476,281 -169,368,240 -7,249,007 -10,518,107 810,859 12,140,259 633,688,624 1,066,693,997 1,139,968,313 1,213,241,254 169,929 34,445,357 23,020 18,310 34,531 -427 -2,274 19 73,488,167 50,435,232 126,299,462 269,223,458 519,446,318 639,119,284 20,383,360 43,685,407 13,795,785 -13,412,585 -95,656,411 -12,847,150 595,067,689 165,854 33,492,631 21,793 16,929 33,112 -532 -2,460 -242 72,964,468 50,075,815 125,399,413 267,304,888 515,744,583 3,583 6,865 9,969 35,345 55,762 High 71,338 19,881,225 9,535 11,024 7,223 -324 -1,073 -167 72,440,768 49,716,398 124,499,363 265,386,318 512,042,847 30,752,734 31,049,494 31,346,255 21,105,728 21,309,396 21,513,064 52,852,778 53,362,801 53,872,824 112,662,456 113,749,636 114,836,816 217,373,696 219,471,328 221,568,959 3,558 6,816 9,898 35,093 55,365 Overall Mean 69,060 19,367,251 8,940 10,320 6,585 -372 -1,179 -295 3,532 6,767 9,827 34,841 54,968 Low 1,528 2,928 4,252 15,076 23,785 1,514 2,900 4,212 14,934 23,560 Clients at risk of abuse Mean High 1,500 2,873 4,172 14,791 23,335 Low Total Treatment Benefits (Number of Crimes Averted) All Non-Drug 99,072 100,868 102,665 66,782 Drug 14,639,353 15,078,106 15,516,858 18,853,277 Fraud 13,448 14,080 14,711 8,345 Burglary 7,313 7,990 8,667 9,617 Larceny 27,165 27,946 28,727 5,947 Robbery -112 -55 2 -420 Agg. Assaults -1,174 -1,095 -1,015 -1,286 Oth. Assaults 182 314 446 -424 Total Treatment Benefits ($ Averted - only dollarizable crime categories) Drug 483,098,660 497,577,492 512,056,326 622,158,155 Fraud 30,662,010 32,101,602 33,541,217 19,025,870 Burglary 30,954,659 33,821,077 36,687,538 40,707,364 Larceny 56,910,047 58,546,304 60,182,541 12,459,740 Robbery -4,033,261 -1,983,991 66,001 -15,145,195 Agg. Assaults -95,269,483 -88,819,870 -82,370,258 -104,316,462 Oth. Assaults 7,926,752 13,658,009 19,389,266 -18,444,859 Total (Benefits) 510,249,384 544,900,624 579,552,630 556,444,613 Number of clients treated under different modalities LT Residential 2,033 2,044 2,055 ST Inpatient 3,894 3,915 3,937 OP Methadone 5,655 5,686 5,717 OP DrugFree 20,050 20,160 20,269 Total (clients) 31,633 31,805 31,977 Total costs under different modalities LT Residential 41,688,034 41,914,973 42,141,912 ST Inpatient 28,610,670 28,766,419 28,922,168 OP Methadone71,646,586 72,036,612 72,426,637 OP DrugFree 152,723,862 153,555,252 154,386,642 Total (costs) 294,669,151 296,273,255 297,877,359 Clients at risk of dependence Low Mean High Table 4.4: Cost benefit analysis of treating eligible drug courts clients in available drug dourt treatment slots. Findings 55 56 To Treat or Not to Treat from drug court treatment. Notably, 34.4 million drug crimes are prevented annually. We count as a drug crime each drug transaction (so a drug deal would count as two crimes, one for the buyer and one for the seller). This reduction in drug offending leads to more than one billion dollars in savings. Notably, since the likelihood of arrest is trivial, this reduction in offending leads to little savings to the criminal justice system, but produces large benefits to private citizens. Reductions in other property crimes leads to significant savings, with almost $200 million in savings from reductions in fraud, burglary and larceny. However, drug court treatment does not reduce crimes in all categories. Notably, there are more than 400 additional robberies and 2,200 additional assaults, that would not have occurred in the absence of drug court. Increases in the crimes result in about $200 million dollars in negative benefits. Overall, we estimate that the current adult drug court treatment regime produces about $2.21 in benefit for every $1 in costs, for a net benefit to society of about $624 million. Interestingly, the benefit-cost ratio is higher for those at risk of abuse (2.71) as compared to those at risk of dependence (1.84), even though the abuse group is less prevalent in the drug court population. Much of this is due to much greater reductions in drug crimes and larceny. Our benefit-cost ratio is similar to other published estimates. For instance, Aos et al. (2005), using a meta analytic design, estimates $2.83 in benefit for every dollar in new cost. It turns out that the benefit-cost ratio is sensitive to which estimates of the price of victimization are used in the benefits calculations. If we rely on data from Miller et al. (1993) and Rajkumar and French (1997), our estimate of the benefit-cost ratio is 2.76, which is very close to the Aos et. al, estimate. Our decision to use the average of published costs to victims yields a lower—and therefore more conservative estimate. Next, we simulate the effects of expanding the number of treatment slots for eligible drug court clients so that all currently eligible drug court clients can be treated. Recall that we found that there were more than twice as many arrestees eligible for drug court (109,922) than there are currently available drug court 3,010 5,767 8,375 29,693 46,845 61,735,649 42,369,431 106,101,153 226,168,179 436,374,412 83,016,643 56,974,664 142,675,449 304,130,970 586,797,726 62,250,361 42,722,679 106,985,755 228,053,822 440,012,617 3,035 5,815 8,445 29,940 47,235 Net BC Ratio Net $ Saved 1.72 418,866,901 1.82 479,428,498 1.92 539,992,491 2.43 624,519,672 2.57 692,240,163 7,019 13,446 19,527 69,232 109,225 Low 2,182,362,842 105,930,715 153,705,775 144,863,112 -24,685,013 -368,778,972 2,228,556 2,195,627,015 342,345 66,132,207 46,461 36,311 69,147 -684 -4,546 51 144,863,521 99,420,431 248,967,765 530,706,637 1,023,958,354 7,064 13,532 19,652 69,674 109,922 Overall Mean 2.03 2.14 1,043,386,573 1,171,668,661 2,126,077,895 100,827,528 143,068,839 139,443,577 -31,838,704 -396,818,686 -19,905,623 2,060,854,826 1,245,972,802 45,955,042 95,550,874 31,772,917 -18,441,628 -180,956,615 -16,242,403 1,203,610,988 2.71 759,960,074 334,863 64,426,603 44,223 33,798 66,560 -883 -4,892 -458 149,191 37,756,752 20,156 22,573 15,166 -511 -2,231 -373 62,765,085 143,945,340 43,075,937 98,790,280 107,870,380 247,389,746 229,939,512 527,342,887 443,650,915 1,017,468,254 3,061 5,863 8,515 30,188 47,626 Clients at risk of abuse Mean High 4,048 7,755 11,262 39,928 62,993 Low Total Treatment Benefits (Number of Crimes Averted) All Non-Drug 194,032 197,334 200,636 140,831 145,011 Drug 28,495,085 29,288,073 30,081,060 35,931,518 36,844,135 Fraud 26,219 27,381 28,543 18,004 19,080 Burglary 13,802 15,026 16,251 19,997 21,285 Larceny 53,741 55,155 56,568 12,819 13,992 Robbery -187 -81 25 -695 -603 Agg. Assaults -2,255 -2,112 -1,970 -2,637 -2,434 Oth. Assaults 425 679 933 -883 -628 Total Treatment Benefits ($ Averted - only dollarizable crime categories) Drug 940,337,808 966,506,397 992,674,987 1,185,740,087 1,215,856,445 Fraud 59,778,408 62,428,634 65,078,906 41,049,120 43,502,081 Burglary 58,422,427 63,607,132 68,791,922 84,646,412 90,098,643 Larceny 112,588,149 115,548,929 118,509,709 26,855,428 29,314,183 Robbery -6,760,932 -2,924,953 911,388 -25,077,772 -21,760,060 Agg. Assaults -182,902,612 -171,343,439 -159,781,833 -213,916,074 -197,435,533 Oth. Assaults 18,497,495 29,551,534 40,605,138 -38,403,118 -27,322,978 Total (Benefits) 999,960,743 1,063,374,235 1,126,790,217 1,060,894,084 1,132,252,780 Number of clients treated under different modalities LT Residential 4,009 4,028 ST Inpatient 7,679 7,717 OP Methadone 11,152 11,207 OP DrugFree 39,540 39,734 Total (clients) 62,380 62,686 Total costs under different modalities LT Residential 82,209,691 82,613,160 ST Inpatient 56,420,850 56,697,752 OP Methadone141,288,593 141,982,010 OP DrugFree 301,174,708 302,652,815 Total (costs) 581,093,842 583,945,737 Clients at risk of dependence Low Mean High 2.26 1,299,952,564 2,238,647,788 111,033,948 164,342,796 150,282,625 -17,530,240 -340,738,448 24,362,735 2,330,401,205 349,827 67,837,812 48,699 38,824 71,734 -486 -4,201 560 145,781,728 100,050,601 250,545,829 534,070,483 1,030,448,641 7,109 13,618 19,776 70,116 110,618 High Table 4.5: Cost benefit analysis of treating all eligible drug court clients (retaining all eligibility restrictions). Findings 57 58 To Treat or Not to Treat treatment slots (55,365). In table 4.5, we simulate the effects of treating all who meet current eligibility standards (regardless of whether a treatment slot in drug court is available) in the four treatment modalities studied by DATOS. We find that the costs of treating these additional clients about doubles, to slightly more than $1 billion. We find that the expansion of drug treatment to this larger population remains cost-effective, although the benefit-cost ratio is fractionally reduced to 2.14 from 2.21. In total, this expansion of treatment yields a benefit to society of more than $1.17 billion dollars. 4.4. SIMULATED COST-EFFECTIVENESS OF EXPANDING CRIMINAL JUSTICE SYSTEM–BASED TREATMENT In the next five simulations, we incrementally relax existing drug court eligibility requirements to model the cost-effectiveness of expanding criminal justice system-based treatment to individuals who are currently excluded from drug courts. 4.4.1. Simulation 1: Adding Individuals with Other Pending Charges Beginning with the same group described in table 4.5 (everyone eligible for drug court regardless of whether there would be a treatment slot in the current regime), we first relax the assumption about current charges that currently restrict drug court eligibility. From table 3.1, about half of drug courts restrict eligibility to those arrestees who do not have a current charge pending in court. In table 4.6, we relax this restriction, and allow drug courts to enroll into treatment any arrestee, regardless of whether they have some other pending charge. This change in eligibility has little impact on the number of individuals entering drug court. Because the presence of any ineligible characteristic causes an arrestee to be excluded from drug court, few additional arrestees become eligible. We suspect that exclusions for active criminal justice cases is highly correlated with other grounds for exclusion, such as a past violence history. We 74,001,824 50,787,757 127,182,252 271,105,238 523,077,071 73,431,485 50,396,331 126,202,046 269,015,803 519,045,665 Net BC Ratio Net $ Saved 2.02 692,611,484 2.15 780,972,766 2.27 869,335,740 2.51 784,043,567 8,250 15,805 22,953 81,378 128,386 2,289,287,763 110,859,300 183,975,958 156,454,286 47,114,458 -272,990,341 338,917,596 2,853,619,020 409,082 69,372,356 48,623 43,462 74,680 1,306 -3,365 7,791 170,234,729 116,832,796 292,571,654 623,653,903 1,203,293,081 8,301 15,902 23,094 81,877 129,173 Overall Mean 2.81 2.23 2.37 954,661,827 1,476,655,051 1,650,325,940 2,228,193,866 105,315,457 172,058,920 150,181,164 32,732,059 -308,640,451 292,778,260 2,672,619,277 1,275,739,482 1,308,183,511 46,863,348 49,501,992 101,425,347 107,484,929 27,397,342 30,403,038 10,112,185 15,595,299 -175,461,749 -155,016,209 106,354,290 125,617,838 1,392,430,244 1,481,770,397 2.66 869,353,174 400,059 67,521,026 46,191 40,647 71,686 908 -3,805 6,730 74,572,177 169,197,886 51,179,192 116,121,206 128,162,480 290,789,698 273,194,720 619,855,436 527,108,570 1,195,964,226 3,636 6,966 10,116 35,866 56,585 Low 177,520 39,641,925 21,711 25,392 14,512 432 -1,911 2,888 172,690 38,658,772 20,554 23,961 13,077 280 -2,163 2,445 3,608 6,913 10,039 35,592 56,152 Clients at risk of abuse Mean High 3,581 6,859 9,961 35,318 55,719 Low Total Treatment Benefits (Number of Crimes Averted) All Non-Drug 232,199 236,392 240,585 167,860 Drug 29,845,406 30,713,584 31,581,762 37,675,620 Fraud 26,794 28,068 29,343 19,397 Burglary 18,118 19,502 20,885 22,529 Larceny 60,043 61,602 63,162 11,643 Robbery 779 1,026 1,273 128 Agg. Assaults -1,390 -1,202 -1,015 -2,415 Oth. Assaults 4,728 5,346 5,964 2,002 Total Treatment Benefits ($ Averted - only dollarizable crime categories) Drug 984,898,413 1,013,548,280 1,042,198,148 1,243,295,454 Fraud 61,090,776 63,995,952 66,901,060 44,224,681 Burglary 76,693,071 82,550,611 88,408,237 95,365,850 Larceny 125,789,540 129,056,944 132,324,327 24,391,624 Robbery 28,102,267 37,002,273 45,901,920 4,629,792 Agg. Assaults -112,731,540 -97,528,591 -82,324,021 -195,908,911 Oth. Assaults 205,687,518 232,563,306 259,439,529 87,090,742 Total (Benefits) 1,369,530,045 1,461,188,776 1,552,849,199 1,303,089,232 Number of clients treated under different modalities LT Residential 4,670 4,692 4,715 ST Inpatient 8,946 8,989 9,033 OP Methadone 12,991 13,055 13,118 OP DrugFree 46,060 46,284 46,509 Total (clients) 72,667 73,021 73,375 Total costs under different modalities LT Residential 95,766,401 96,232,904 96,699,408 ST Inpatient 65,724,876 66,045,039 66,365,202 OP Methadone 164,587,652 165,389,402 166,191,152 OP DrugFree 350,839,633 352,548,665 354,257,698 Total (costs) 676,918,561 680,216,010 683,513,459 Clients at risk of dependence Low Mean High 2.51 1,823,997,567 2,350,381,658 116,403,052 195,893,165 162,727,365 61,497,219 -237,340,230 385,057,367 3,034,619,596 418,105 71,223,687 51,054 46,278 77,674 1,705 -2,926 8,852 171,271,584 117,544,394 294,353,632 627,452,418 1,210,622,029 8,351 15,999 23,234 82,375 129,960 High Table 4.6: Cost benefit analysis of treating all eligible drug court clients without regard to current offense (retaining other eligibility restrictions). Findings 59 60 To Treat or Not to Treat find that the number of arrestees enrolled in drug court would increase by only a little more than 19,000, to a total of 129,173 arrestees if this exclusion criteria was removed. However, we find that allowing these arrestees to participate in treatment significantly enhances the cost-effectiveness of drug courts. Treating these additional clients increases the total cost of drug court and treatment to a little more than $1.2 billion. The benefits increase from about $2.196 billion to $2.854 billion, and the benefit-cost ratio increases from 2.14:1 to 2.37:1. Since there are only 19,000 new eligibles, these changes in overall mean disguise the effectiveness of treating this group. We estimate that the group of arrestees who are currently ineligible because of a pending court case are a particularly cost-effective population to serve, with a benefit-cost ratio of 3.67:1. While both groups of new eligibles (those at risk of drug dependence and those at risk of drug abuse) have high benefit-cost ratios, the benefit-cost ratio for the 10,000 individuals who are at-risk of drug dependence and previously had been excluded for a pending charge is the highest for any sub-group in any of the simulations (4.13:1). 4.4.2. Simulation 2: Adding Individuals with Prior Violence In table 4.7, we return again to the population in table 4.5 (all eligible arrestees) and relax the eligible exclusion for those with past violence. That is, we are now studying the population that would be eligible for drug court if there were enough slots, and allowing those with past violence to be eligible (we are once again not allowing those with a current charge to be eligible). Since 88% of drug courts exclude those with past violence, removing this exclusion leads to the size of eligibles more than doubling, from 109,922 to 213,460 (but we note that many who are excluded because of past violence are excluded on other grounds as well). Total costs of treating this group are almost $2 billion. Benefits increase substantially as well, to almost $4.3 billion. The overall benefit to cost ratio is 2.15, almost identical to the 2.14:1 ratio for the eligible group with the violence restriction. Net BC Ratio Net $ Saved 1.50 562,967,489 1.61 691,420,902 13,631 26,112 37,921 134,446 212,110 Low 13,717 26,278 38,162 135,302 213,460 Overall Mean 13,804 26,445 38,404 136,158 214,810 High 1.72 2.67 2.85 3.03 819,876,515 1,424,367,171 1,591,171,205 1,757,973,601 2,256,039,712 75,557,809 136,527,415 60,604,055 -29,920,714 -151,682,301 277,576,401 2,624,702,378 2,199,920,825 71,798,864 128,463,550 56,925,822 -45,812,115 -181,853,769 220,897,208 2,450,340,385 2.01 1,987,334,660 3,765,471,598 145,007,316 162,473,757 256,721,447 -104,060,148 -719,059,213 456,662,188 3,963,216,945 635,131 114,105,200 63,600 38,383 122,540 -2,885 -8,864 10,498 2.15 2,282,592,107 3,862,769,893 152,930,453 179,412,319 266,234,653 -75,835,257 -658,235,253 543,772,940 4,271,049,748 649,811 117,053,633 67,075 42,384 127,081 -2,103 -8,115 12,500 2.29 2,577,850,116 3,960,068,187 160,853,635 196,351,049 275,747,881 -47,610,005 -597,412,104 630,884,563 4,578,883,206 664,492 120,002,066 70,550 46,386 131,622 -1,320 -7,365 14,503 122,619,618 279,536,041 281,315,128 283,094,227 84,154,349 191,846,737 193,067,732 194,288,735 210,738,577 480,420,902 483,478,505 486,536,131 449,216,232 1,024,078,604 1,030,596,276 1,037,113,996 866,728,776 1,975,882,285 1,988,457,641 2,001,033,089 299,980 68,364,840 33,139 32,253 28,928 -830 -1,870 6,381 121,550,131 83,420,356 208,900,518 445,298,175 859,169,180 120,480,658 82,686,372 207,062,482 441,380,165 851,609,677 5,979 11,454 16,634 58,975 93,043 292,155 66,664,267 31,491 30,348 27,172 -1,270 -2,242 5,078 5,927 11,354 16,489 58,461 92,232 Clients at risk of abuse Mean High 5,875 11,254 16,344 57,947 91,420 Low Total Treatment Benefits (Number of Crimes Averted) All Non-Drug 350,800 357,656 364,512 284,331 Drug 49,141,505 50,389,366 51,637,227 64,963,695 Fraud 33,758 35,584 37,410 29,842 Burglary 9,940 12,036 14,133 28,443 Larceny 97,124 99,909 102,694 25,417 Robbery -1,174 -832 -490 -1,711 Agg. Assaults -6,251 -5,873 -5,495 -2,614 Oth. Assaults 6,723 7,422 8,122 3,775 Total Treatment Benefits ($ Averted - only dollarizable crime categories) Drug 1,621,669,661 1,662,849,068 1,704,028,475 2,143,801,937 Fraud 76,967,328 81,131,588 85,295,826 68,039,988 Burglary 42,073,988 50,948,769 59,823,634 120,399,769 Larceny 203,473,858 209,308,831 215,143,825 53,247,588 Robbery -42,356,992 -30,023,142 -17,689,291 -61,703,155 Agg. Assaults -507,032,354 -476,381,484 -445,729,803 -212,026,859 Oth. Assaults 292,444,608 322,875,732 353,308,162 164,217,580 Total (Benefits) 1,687,240,097 1,820,709,363 1,954,180,828 2,275,976,848 Number of clients treated under different modalities LT Residential 7,756 7,790 7,825 ST Inpatient 14,858 14,924 14,990 OP Methadone 21,577 21,673 21,769 OP DrugFree 76,500 76,841 77,182 Total (clients) 120,690 121,229 121,767 Total costs under different modalities LT Residential 159,055,383 159,764,996 160,474,609 ST Inpatient 109,160,365 109,647,375 110,134,386 OP Methadone 273,358,421 274,577,987 275,797,554 OP DrugFree 582,698,439 585,298,102 587,897,764 Total (costs) 1,124,272,608 1,129,288,461 1,134,304,313 Clients at risk of dependence Low Mean High Table 4.7: Cost benefit analysis of treating all eligible drug court clients without regard to past violence (retaining other eligibility restrictions). Findings 61 62 To Treat or Not to Treat However, this similarity masks a critical difference among those with past violence. For those at risk of abuse, removing the past violence exclusion increases the eligible population from 47,235 to 92,232. Adding this population is very cost-effective. Overall, the benefit to cost ratio for the newly eligible group of at-risk with past violence (3.14) is higher than the at-risk group in general (2.85). Conversely, relaxing the exclusion for prior violence for the population at risk of drug dependence is much less cost-effective. For those at risk of drug dependence, removing the past violence exclusion increases the eligible population from 62,686 to 121,229. Overall, the benefit to cost ratio declines from 1.82:1 to 1.61:1. Focusing only on those who become newly eligible reveals that the benefit is much smaller than it appears in the overall means. New costs of treating this added group are about $545 million, and benefits are $757 million, for a benefit-cost ratio of 1.38:1. Put another way, even though the newly eligible population of those at risk of drug dependence is about 30% larger than the newly eligible population of those at risk of drug abuse, the total benefits are about 40% smaller. Adding those at risk of drug abuse with past violence is significantly more cost-effective than adding those at risk of drug dependence with past violence. 4.4.3. Simulation 3: Adding Individuals with Prior Failed Treatment Next, we return to the eligible population in table 4.5, and simulate the effect of dropping the exclusion on past treatment. Many drug courts exclude individuals who have a history of failed treatment, have failed drug court in the past, or have participated in drug court in the past. In table 4.8, we estimate the effect of allowing the arrestee group to enter into treatment, regardless of whether they had failed treatment (or drug court) in the past. By dropping the past treatment exclusion, about 38,000 additional arrestees become eligible for drug court managed treatment. There is little change in the cost-effectiveness of drug court as a result, which suggests it is cost-effective to treat this group. The benefit 3,705 7,098 10,308 36,547 57,659 75,987,963 52,150,852 130,595,702 278,381,444 537,115,962 118,636,627 81,420,806 203,893,262 434,624,567 838,575,261 Net BC Ratio Net $ Saved 1.72 595,134,514 1.82 683,050,071 1.92 770,964,948 2.38 740,609,401 2.52 823,846,564 1,526,825,621 48,373,369 94,609,667 35,125,357 -42,460,862 -267,162,084 -29,463,662 1,365,847,404 175,287 46,267,443 21,216 22,351 16,766 -1,177 -3,294 -677 76,679,046 52,625,146 131,783,424 280,913,224 542,000,840 3,739 7,163 10,402 36,880 58,184 460,726 86,524,250 57,806 39,968 93,939 -1,922 -6,879 -508 193,522,261 132,815,125 332,594,462 708,967,639 1,367,899,488 9,436 18,077 26,253 93,077 146,844 Low 2,930,596,869 138,625,847 182,003,719 204,290,531 -58,745,383 -520,745,183 7,550,469 2,883,576,869 470,736 88,805,966 60,801 42,996 97,513 -1,629 -6,420 174 194,764,509 133,667,685 334,729,435 713,518,605 1,376,680,233 9,497 18,194 26,421 93,674 147,786 Overall Mean 3,005,893,480 145,453,968 194,824,714 211,779,130 -48,159,290 -483,488,144 37,205,970 3,063,509,827 480,746 91,087,681 63,796 46,025 101,088 -1,335 -5,960 855 196,006,756 134,520,245 336,864,407 718,069,572 1,385,460,979 9,558 18,310 26,590 94,272 148,729 High 2.66 1.98 2.09 2.21 907,083,900 1,335,743,915 1,506,896,635 1,678,048,848 1,563,989,457 2,855,300,257 51,412,700 131,797,817 101,017,921 169,182,681 38,283,339 196,801,891 -37,040,143 -69,332,918 -246,878,778 -558,003,032 -16,814,877 -22,103,293 1,453,969,619 2,703,643,403 180,589 47,393,620 22,549 23,864 18,274 -1,027 -3,043 -387 77,370,130 53,099,439 132,971,145 283,445,005 546,885,719 3,773 7,227 10,496 37,212 58,708 Clients at risk of abuse Mean High 5,785 11,082 16,094 57,060 90,021 Low Total Treatment Benefits (Number of Crimes Averted) All Non-Drug 290,741 295,449 300,157 169,985 Drug 41,382,984 42,538,523 43,694,061 45,141,266 Fraud 37,923 39,584 41,246 19,883 Burglary 19,131 20,646 22,161 20,837 Larceny 78,680 80,747 82,814 15,259 Robbery -595 -452 -308 -1,328 Agg. Assaults -3,335 -3,126 -2,917 -3,544 Oth. Assaults 460 851 1,242 -968 Total Treatment Benefits ($ Averted - only dollarizable crime categories) Drug 1,365,638,472 1,403,771,248 1,441,904,024 1,489,661,785 Fraud 86,463,733 90,252,478 94,041,268 45,334,084 Burglary 80,981,269 87,394,052 93,806,793 88,201,412 Larceny 164,834,537 169,165,175 173,495,791 31,967,354 Robbery -21,450,614 -16,284,520 -11,119,148 -47,882,304 Agg. Assaults -270,557,642 -253,583,098 -236,609,366 -287,445,390 Oth. Assaults 20,008,285 37,014,131 54,020,847 -42,111,578 Total (Benefits) 1,425,918,040 1,517,729,464 1,609,540,208 1,277,725,363 Number of clients treated under different modalities LT Residential 5,731 5,758 ST Inpatient 10,979 11,031 OP Methadone 15,944 16,019 OP DrugFree 56,530 56,795 Total (clients) 89,184 89,603 Total costs under different modalities LT Residential 117,534,298 118,085,462 ST Inpatient 80,664,273 81,042,540 OP Methadone 201,998,760 202,946,011 OP DrugFree 430,586,194 432,605,381 Total (costs) 830,783,526 834,679,393 Clients at risk of dependence Low Mean High Table 4.8: Cost benefit analysis of treating all eligible drug court clients without regard to past treatment (retaining other eligibility restrictions). Findings 63 64 To Treat or Not to Treat to cost ratio decreases slightly, from 2.14:1 to 2.09:1. Again, the results vary by drug severity. That is, the newly added group at risk of drug dependence has a benefit to cost ratio of 1.81:1, while the newly added group at risk of drug abuse has a benefit to cost ratio of 2.29:1. Again, it is important to illustrate that the group that is most benefited from a change to one policy regime, may not be the group that benefits the most from a change to a different policy regime. 4.4.4. Simulation 4: Adding Individuals with Co-Occurring Alcohol Problems Next, we again return to the eligible population in table 4.5, and this time we simulate the effect of removing current exclusions on alcohol problems. About one-third of drug courts exclude individual with a history of alcohol abuse, and in table 4.9, we simulate the effect of including the eligible pool of treatment clients. By dropping the alcohol problem exclusion, about 70,000 additional arrestees become eligible for drug court managed treatment. The results are mixed. Overall, adding this group reduces the benefit to cost ratio from 2.14:1 to 1.73:1. However, again, these aggregate numbers mask important differences in the responsiveness of this new population to treatment. The newly added group with a history of alcohol abuse adds $654 million in new costs as well as $714 million in new benefits. Thus, the benefit to cost ratio for this group is only 1.09:1. For those at risk of dependence, the results are better, with the newly added group estimated to have a benefit to cost ratio of 1.43:1. However, adding those with a history of alcohol abuse and a risk of drug abuse may not be cost-effective. While the mean expected benefit to cost ratio is 0.70 to 1 for this group. Thus, adding those with co-occurring alcohol problems who are at risk of drug abuse is cost-effective. Adding those with co-occurring alcohol problems who are at risk of drug dependence is not cost-effective. 11,576 22,177 32,206 114,184 180,142 1.77 726,292,699 1.64 473,619,785 1.81 602,147,881 1.98 730,675,363 1.73 1,232,068,523 1.60 1,007,169,906 1.67 629,920,643 Net BC Ratio Net $ Saved 1.57 533,550,121 2,980,699,093 154,102,350 342,463,415 259,984,765 -35,369,566 -810,585,146 18,863,774 2,910,158,685 105,836,486 235,843,155 237,406,188 72,636,016 161,860,129 162,932,845 181,894,470 405,328,704 408,014,989 387,731,328 864,009,977 869,736,139 748,098,300 1,667,041,965 1,678,090,161 11,500 22,031 31,994 113,432 178,956 2,895,152,860 145,549,272 320,256,970 251,106,658 -43,875,010 -875,730,209 -18,248,670 2,674,211,871 104,888,811 71,985,623 180,265,761 384,259,527 741,399,722 5,161 9,886 14,357 50,903 80,308 Overall Mean 644,604 90,324,215 67,589 80,903 124,098 -981 -9,993 434 103,941,136 71,335,230 178,637,052 380,787,726 734,701,144 133,132,735 91,369,545 228,806,805 487,730,973 941,040,058 5,115 9,798 14,229 50,448 79,589 Low 631,935 87,731,905 63,837 75,657 119,860 -1,217 -10,796 -420 5,068 9,709 14,100 49,992 78,870 Clients at risk of abuse Mean High 6,492 12,436 18,060 64,032 101,020 Low Total Treatment Benefits (Number of Crimes Averted) All Non-Drug 354,779 360,237 365,695 277,155 284,367 291,578 Drug 40,492,375 41,711,729 42,931,083 47,239,530 48,612,486 49,985,442 Fraud 37,026 38,810 40,595 26,811 28,778 30,746 Burglary 31,379 33,665 35,951 44,278 47,238 50,199 Larceny 90,931 93,222 95,513 28,929 30,876 32,823 Robbery -125 -3 118 -1,092 -977 -863 Agg. Assaults -4,078 -3,801 -3,523 -6,718 -6,192 -5,667 Oth. Assaults 1,315 1,661 2,007 -1,734 -1,227 -720 Total Treatment Benefits ($ Averted - only dollarizable crime categories) Drug 1,336,248,381 1,376,487,064 1,416,725,747 1,558,904,478 1,604,212,029 1,649,519,581 Fraud 84,419,850 88,487,689 92,555,483 61,129,422 65,614,661 70,099,922 Burglary 132,826,714 142,503,014 152,179,271 187,430,256 199,960,401 212,490,589 Larceny 190,500,717 195,300,174 200,099,630 60,605,941 64,684,592 68,763,284 Robbery -4,505,004 -119,378 4,266,247 -39,370,006 -35,250,187 -31,131,089 Agg. Assaults -330,799,182 -308,290,026 -285,779,247 -544,931,027 -502,295,121 -459,659,215 Oth. Assaults 57,199,465 72,242,546 87,285,627 -75,448,134 -53,378,772 -31,309,410 Total (Benefits) 1,465,890,942 1,566,611,082 1,667,332,757 1,208,320,929 1,343,547,603 1,478,773,663 Number of clients treated under different modalities LT Residential 6,432 6,462 ST Inpatient 12,321 12,379 OP Methadone 17,893 17,977 OP DrugFree 63,440 63,736 Total (clients) 100,086 100,553 Total costs under different modalities LT Residential 131,902,019 132,517,377 ST Inpatient 90,524,899 90,947,222 OP Methadone 226,691,652 227,749,229 OP DrugFree 483,222,252 485,476,612 Total (costs) 932,340,821 936,690,439 Clients at risk of dependence Low Mean High 1.86 1,456,968,062 3,066,245,327 162,655,405 364,669,860 268,862,914 -26,864,842 -745,438,461 55,976,217 3,146,106,420 657,273 92,916,525 71,340 86,149 128,336 -745 -9,190 1,287 238,969,221 164,005,561 410,701,275 875,462,301 1,689,138,358 11,652 22,323 32,418 114,935 181,328 High Table 4.9: Cost benefit analysis of treating all eligible drug court clients without regard to co-occurring alcohol problems (retaining other eligibility restrictions). Findings 65 66 4.4.5. To Treat or Not to Treat Simulation 5: Expanding Eligibility to Include all Arrestees Finally, we simulate the effects of treating all arrestees at risk of drug dependence or at risk of drug abuse. That is, in table 4.10 we estimate the costs and benefits of treating all 1.47 million arrestees with a serious drug problem who are likely to be found guilty each year. The results suggest doing so would be very costeffective. For the entire group, the costs of treatment would exceed $13.7 billion. The benefits are estimated at more than $46 billion. Overall, the benefit to cost ratio is 3.36:1, meaning that for every dollar in costs, there are more than three dollars in benefits. The results suggest that treating those who are currently excluded will be substantially more cost-effective than current practice or any limited expansion of current eligibility. 4.24 9,796,861,814 4.01 9,029,179,797 3.77 8,261,498,407 Net BC Ratio Net $ Saved 3.31 24,811,224,952 5,549,430,293 213,026,807 653,233,470 251,457,508 342,175,454 295,442,715 5,515,396,088 12,820,162,334 5,393,134,402 199,245,871 619,071,043 236,589,125 279,344,514 131,920,577 5,172,398,098 12,031,703,631 3.18 23,330,768,029 1,557,254 168,164,554 93,433 154,319 120,027 9,487 3,642 126,788 2.12 11,950,310,365 94,151 180,367 261,934 928,671 1,465,123 Low 7,330,119 640,816,332 352,914 435,575 1,249,205 32,548 9,538 407,024 1,939,048,802 1,330,777,185 3,332,520,453 7,103,693,610 13,706,040,050 94,551 181,132 263,045 932,610 1,471,339 Overall Mean 7,477,027 656,122,556 378,639 461,914 1,281,587 37,892 15,972 426,526 1,947,240,011 1,336,398,845 3,346,598,165 7,133,702,054 13,763,939,075 94,950 181,897 264,156 936,550 1,477,554 High 2.48 20,211,808,772 3.36 32,359,947,826 3.51 34,608,086,766 10,741,833,561 21,146,938,948 21,652,044,336 745,989,803 804,643,145 863,296,555 1,732,300,644 1,843,790,330 1,955,279,972 2,549,244,101 2,617,084,119 2,684,924,116 981,170,841 1,173,891,676 1,366,611,429 251,782,301 773,671,233 1,295,560,166 16,857,628,453 17,705,968,424 18,554,309,266 33,859,949,704 46,065,987,876 48,372,025,840 7,183,211 325,510,108 327,189 409,237 1,216,823 27,205 3,104 387,523 427,718,526 1,930,857,580 293,544,988 1,325,155,516 735,092,761 3,318,442,718 1,566,944,246 7,073,685,118 3,023,300,520 13,648,140,932 1,520,967 163,428,315 87,389 146,249 112,930 7,745 1,626 118,903 424,779,165 291,527,691 730,041,066 1,556,175,912 3,002,523,834 20,856 39,954 58,023 205,717 324,550 High Total Treatment Benefits (Number of Crimes Averted) All Non-Drug 5,698,532 5,809,152 5,919,773 1,484,679 Drug 166,818,032 477,388,017 487,958,001 158,692,076 Fraud 245,844 265,525 285,206 81,344 Burglary 271,059 289,327 307,594 138,178 Larceny 1,110,990 1,136,274 1,161,559 105,833 Robbery 21,202 24,803 28,404 6,003 Agg. Assaults 3,494 7,911 12,329 -390 Oth. Assaults 276,505 288,121 299,738 111,018 Total Treatment Benefits ($ Averted - only dollarizable crime categories) Drug 5,504,995,050 15,753,804,547 16,102,614,043 5,236,838,510 Fraud 560,524,822 605,397,274 650,269,748 185,464,981 Burglary 1,147,392,070 1,224,719,286 1,302,046,503 584,908,575 Larceny 2,327,523,338 2,380,494,994 2,433,466,608 221,720,764 Robbery 764,657,627 894,547,162 1,024,435,976 216,513,215 Agg. Assaults 283,383,862 641,750,656 1,000,117,450 -31,601,561 Oth. Assaults 12,028,227,475 12,533,570,326 13,038,913,178 4,829,400,978 Total (Benefits) 22,616,704,243 34,034,284,245 35,551,863,506 11,243,245,461 421,839,790 289,510,386 724,989,348 1,545,407,530 2,981,747,054 1,519,521,484 1,042,853,858 2,611,505,404 5,566,757,808 10,740,638,554 20,713 39,680 57,624 204,303 322,320 Clients at risk of abuse Mean 20,570 39,405 57,225 202,889 320,090 Low 74,094 141,943 206,134 730,833 1,153,004 Clients at risk of dependence Mean High Number of clients treated under different modalities LT Residential 73,582 73,838 ST Inpatient 140,962 141,452 OP Methadone 204,709 205,421 OP DrugFree 725,781 728,307 Total (clients) 1,145,034 1,149,019 Total costs under different modalities LT Residential 1,509,017,790 1,514,269,637 ST Inpatient 1,035,645,129 1,039,249,493 OP Methadone2,593,453,370 2,602,479,387 OP DrugFree 5,528,277,588 5,547,517,698 Total (costs) 10,666,393,877 10,703,516,216 Low Table 4.10: Cost benefit analysis of treating all drug involved arrestees (with no eligibility restrictions). Findings 67 68 To Treat or Not to Treat Chapter 5 Discussion Our main motivation was to provide some answers to the question: What crime reducing benefits can we reasonably expect if the therapeutic jurisprudence model was extended to all offenders in need of treatment. Clearly information needed to answer this question cannot be found in one place. Therefore, we designed a synthetic data set—of 40,320 possible profiles—and using data from various sources interpolated values conditional on profile attributes. We computed the prevalence of the potential clients and computed the expected number of crimes avertable by treating them under various modalities for each of the profiles in our synthetic data. Moreover, for each of them, we computed precision estimates as well. Using combinations of several of the interpolated outcomes, we estimate that nearly 1.5 million arrestees nationwide who are probably guilty are either at risk of drug dependence or of abusing drugs. Moreover, several million crimes could be averted annually by treating these individuals. Although our current work was motivated by a limited question, we believe the creating of the synthetic data has several future benefits. Clearly, the prevalence estimates and the conditional crime reducing benefits provide some guidance to policy makers on the prospects of going to scale in therapeutic jurisprudence. A more thorough analysis, however, would require additional pieces of information. For example, not every profile in our data set and not every treatment 69 70 To Treat or Not to Treat modality costs the same. Hence, even if we could attach dollar estimates to the crimes avertable by treatment, an important quantity to add to this data set would the anticipated costs of treating individuals. Similarly, the counterfactual currently is based on the absence of treatment. This may be statistically a clean counterfactual, however from a policy perspective, the benefits of treating drug involved offender should be compared against other policy options—e.g., incarcerating them. Clearly, incarcerating these offenders will avert some crimes (if for no other reason than the mere fact that they are incapacitated). What is important for making informed policy choices is whether the addition reduction in crimes, over and above those averted by treatment, are worth the additional costs of incarcerating offenders. As we continue to refine these data, we can create estimates of the costs and benefits of treating varying offender profiles across available treatment modalities to estimate the optimal mix of treatment. In addition, the current drug court eligibility assumptions (that, for example, those with violence in their current charge should be excluded) are testable using this method. Estimates can also be generated about the treatment capacity that would have to become available to facilitate the additional demand for treatment. We believe the synthetic data set we have designed is ideally suited to address these future questions. Note that the additional variables of interest could be interpolated onto the current dataset from any other data source. In a similar manner, if in future researchers develop better estimates of the long term (post graduation) crime reducing benefits of treatment, they can be interpolated onto the synthetic data as well and built into the analysis. A final future benefit of the synthetic data approach is that local jurisdictions that may not have the resources to conduct expensive studies of the potential costs and benefits of its policy options (regarding offenders at risk of drug dependence or abuse) may be in a position to simply replace the prevalence estimates in the synthetic data with numbers that better reflect their jurisdiction. Thereafter, they can utilize all the knowledge that has been interpolated onto Discussion 71 the synthetic data from several data sources and, potentially, several scholars for making informed decision relevant to their population. These extensions are exciting and possible, at least in theory. Several are currently under way. We hope that this article will help trigger interest in the use of such detailed and large synthetic data, as a viable alternative to real microdata, for generating and assessing simulated evidence on the prospects of going to scale in other interventions. 72 To Treat or Not to Treat Chapter 6 References Anglin, M.D. & Maugh, T.H.I. (1992). Ensuring Success in Interventions with Drug Using Offenders. Annals, AAPSS, 521, 66-90. Anglin, M.D. and Perrochet, B. (1998). Drug Use and Crime: A Historical Review of Research Conducted by the UCLA Drug Abuse Research Center. Anglin MD, Brecht ML, Maddahian E. Pre-treatment Characteristics and Treatment Performance of Legally Coerced Versus Voluntary Methadone Maintenance Admissions. Criminology 1990; 27, 537-57. Anglin, M.D., Longshore, D. & Turner, S. (1999). Treatment Alternatives to Street Crime: An Evaluation of Five Programs. Criminal Justice and Behavior, 26, 2, 168-195. Aos, S., Phipps, P., and Barnoski, R. (2005). Washington’s drug offender sentencing alternative: An evaluation of benefits and costs, Olympia: Washington State Institute for Public Policy, Document No. 05-01-1901. Ball, J., Rosen, L., Flueck, J. & Nurco, D. (1982). Lifetime Criminality of Heroin Addicts in the United States. Journal of Drug Issues, 3, 225-239. Ball, J.C., Shaffer, J.W., & Nurco, D.N. (1983). Day to Day Criminality of Heroin Addicts in Baltimore: A Study in the Continuity of Offense Rates. Drug and Alcohol Dependence, 12, 119-142. 73 74 To Treat or Not to Treat Belenko, S. (1999). Research on Drug Courts: A Critical Review. National Drug Court Institute Review, 2, 2, 1-58. Blumstein, A. (2000). The Replacement of Drug Offenders to Diminish the Effects of Incarceration. Unpublished manuscript, Carnegie Mellon University. Blumstein, A., & Cork, D. (1996) Linking Gun Availability to Youth Gun Violence. Law and Contemporary Problems, 59, 5-24. Boyum, D. A., & Kleiman, M. A. (2002). Substance-abuse policy from a crimecontrol perspective. In J. Q. Wilson & J. Petersilia (Eds.), Crime: Public policies for crime control. Oakland, CA: Institute for Contemporary Studies, 331-382 Brownstein, H.H., Shiledar Baxi, H., Goldstein, P.J., & Ryan, P.J. (1992). The Relationship of Drugs, Drug Trafficking, and Drug Traffickers to Homicide. Journal of Crime and Justice, 15, 25-44. Caulkins, J.P. & Reuter, P. (1997). Setting Goals for Drug Policy: Harm Reduction or Use Reduction? Addiction, 92, 1143-1150. Chaiken, J.M. and Chaiken, M.R. (1990). Drugs and Predatory Crime. Crime and Justice, 13, 203-39. Collins J.J. & Allison M. (1983) Legal coercion and retention in drug abuse treatment. Hospital and Community Psychiatry, 34, 12, 1145-49. Condon, J. and Smith, N. (2003). Prevalence of Drug Use: Key Findings from the 2002/2003 British Crime Survey, United Kingdom Home Office. Cottler, L.B., Compton, W.M., Mager, D., Spitznagel, E., & Janca, A. (1992). Posttraumatic Stress Disorder Among Substance Users from the General Population. American Journal of Psychiatry, 149, 664-670. Dawkins, M.P. (1997). Drug Use and Violent Crime Among Adolescents. Adolescence, 32, 395-405. References 75 Degenhardt, L., Conroy, E., Gilmour, S. and Collins, L. (2005). The Effect of a Reduction in Heroin Supply in Australia upon Drug Distribution and Acquisitive Crime. The British Journal of Criminology, 45, 2-24. DeLeon, G. (1988a). Legal Pressure in Therapeutic Communities. Journal of Drug Issues,18, 625-640. DeLeon, G. (1988b). Legal Pressure in Therapeutic Communities. In C. G. Leukfield and F. M. Tims (Eds.), Compulsory Treatment of Drug Abuse: Research and Clinical Practice (NIDA Research Monograph 86, DHHS Publication No. ADM 88-1578, Rockville, MD: National Institute on Drug Abuse, 160-177. Finigan, M. (1998). An Outcome Program Evaluation of the Multnomah County S.T.O.P.Drug Diversion Program. West Linn, OR: Northwest Professional Consortium. Flynn, P. M., Kristiansen, P. L., Porto, J. V., & Hubbard, R. L. (1999). Costs and Benefits of Treatment for Cocaine Addiction in DATOS. Drug and Alcohol Dependence, 57, 167-174 Freeman, R.B. (1996). Why Do So Many Young American Men Commit Crimes and What Might We Do About It? Journal of Economic Perspectives, 10, 25-42. French, M. T., Zarkin, G. A., Hubbard, R. L., & Rachal, J. V. (1993). The Effects of Time in Drug Abuse Treatment and Employment on Posttreatment Drug Use and Criminal Activity. American Journal on Drug and Alcohol Abuse 19, 19-33. Goldkamp, J.S. & Weiland, D. (1993). Assessing the Impact of Dade County’s Felony Drug Court. Washington, DC: U.S. Department of Justice, Office of Justice Programs, National Institute of Justice. . Goldstein, P. (1985). The Drug/violence Nexus: A Tripartite Conceptual Framework. Journal of Drug Issues, 14, 493-506. 76 To Treat or Not to Treat Golub, A., & Johnson, B.D. (1997). Crack’s Decline: Some Surprises Across U.S. Cities. Washington, DC: U.S. Department of Justice, National Institute of Justice, NCJ 165707. Gottfredson, D.C. & Exum M.L. (2002). The Baltimore City Drug Treatment Court: One-Year Results from a Randomized Study. Journal of Research in Crime and Delinquency, 39, 3, 337-356. Gropper, B. A. (1985). Probing the Links Between Drugs and Crime. National Institute of Justice Research in Brief. Washington, D.C.: U.S. Department of Justice, National Institute of Justice. Harrell, A. & Roman, J. (2001). Reducing Drug Use and Crime Among Offenders: The Impact of Graduated Sanctions. Journal of Drug Issues 31, 1, 207-232. Harrison, L., & Gfroerer, J. (1992). The Intersections of Drug Use and Criminal Behavior: Results from the National Household Survey on Drug Abuse. Crime and Delinquency, 38, 422-443. Holloway, K.R., Bennett, T.H. & Farrington, D.P. (2006). The Effectiveness of Drug Treatment Programs in Reducing Criminal Behavior: A MetaAnalysis. Psichothema 18, 3, 620-629 Hora, P.F., Schma, W.G. & Rosenthal., J. (1999). Therapeutic Jurisprudence and the Drug Treatment Court Movement: Revolutionizing the Criminal Justice System’s Response to Drug Abuse and Crime in America. Notre Dame Law Review, 74, 2, 439-527. Hubbard, R. L., Craddock, S. G., Flynn, P. M., Anderson, J., & Etheridge, R. M. (1997). Overview of 1-year Follow-up Outcomes in the Drug Abuse Treatment Outcome Study (DATOS). Psychology of Addictive Behaviors, 11, 261-278. Hubbard, R.L., Marsden, M.E., Rachal, J.V., Harwood, H.J., Cavanagh, E.R. & Ginzburg, H.M. (1989). Drug Abuse Treatment: A National Study of Effectiveness. Chapel Hill, NC: University of North Carolina Press. References 77 Inciardi, J. A. (1987). Heroin Use and Street Crime. In C. D. Chambers, A. Inciardi, D. M. Petersen, H. A. Siegel, & O. Z. White (Eds.), Chemical Dependencies: Patterns, Costs, and Consequences. Athens: Ohio University Press. Inciardi, J.A. (1992). The War on Drugs II: The Continuing Epic of Heroin, Cocaine, Crack, Crime, AIDS, and the Public Policy. Mountain View, CA: Mayfield Publishing Co. Inciardi, J.A., & Pottieger, A.E. (1994). Crack Cocaine Use and Street Crime. Journal of Drug Issues, 24, 273-292. Inciardi, J. A., Martin, S. S., Butzin, C. A., Hooper, R. M., & Harrison, L. D. (1996). An Effective Model of Prison-based Treatment for Drug-Involved Offenders. Journal of Drug Issues, 27, 2, 261-278. Johnson, B.D., Goldstein, P.J., Preble, E., Schmeidler, J., Lipton, D.S., Spunt, B., & Miller, T. (1985). Taking Care of Business: The Economics of Crime by Heroin Users. Lexington, MA: Lexington Books. Karberg, J., & James, J. (2005). Substance Dependence, Abuse, and Treatment of Jail Inmates, 2002. Washington, DC: U.S. Department of Justice, Office of Justice Programs, Bureau of Justice Statistics. Lawental, E., McClellan, A.T., Grissom, G.R., Brill, P. & O’Brien, C. (1996). Coerced Treatment for Substance Abuse Problems Detected Through Workplace Urine Surveillance: Is It Effective? Journal of Substance Abuse, 8, 1, 115-128. Lewis, B.F. & Ross, R. (1994). Retention in Therapeutic Communities: Challenges for the Nineties. In F. M. Tims, G. De Leon & N. Jainchill (Eds.) Therapeutic Community: Advances in Research and Application. Washington: Government Printing Office, 99-116. Lipsey, M.W. & Cullen, F.T. (2007) The Effectiveness of Correctional Rehabilitation: A Review of Systematic Reviews. Annual Review of Law and Social Science 3 (in press). 78 To Treat or Not to Treat Longshore, D., Urada, D., Evans, E., Hser, Y., Prendergast, M., Hawken, A., Bunch, T., and Ettner, S. (2004). Evaluation of the Substance Abuse and Crime Prevention Act 2003 Report. Department of Alcohol and Drug Programs California Health and Human Services Agency. MacCoun, R.J. & Reuter, P. (2001) . Drug War Heresies: Learning from Other Vices, Times, and Places. New York, NY: Cambridge University Press. MacCoun, R.J., Kilmer, B., & Reuter, P. (2003). Research on Drug-crime Linkages: The Next Generation. In: Toward a drugs and crime research agenda for the 21st century. National Institute of Justice Special Report. Mackenzie, Doris L. (2006) What Works in Corrections Reducing the Criminal Activities of Offenders and Delinquents. New York: Cambridge University Press. Marlowe, D. B., Festinger, D. S., & Lee, P. A. (2004). The Judge is a Key Component of Drug Court. National Drug Court Institute Review, 4, 2, 1-34. Miller, N.S., & Gold, M.S. (1994). Criminal Activity and Crack Addiction. The International Journal of Addictions, 29, 1069-1078. Mocan, H.N. and Tekin, E. (2004). Guns, Drugs and Juvenile Crime: Evidence From a Panel of Siblings and Twins. IZA Discussion Paper No. 932. Mumola, C., & Karberg, J. (2006). Drug Use and Dependence, State and Federal Prisoners, 2004. Washington, DC: U.S. Department of Justice, Office of Justice Programs, Bureau of Justice Statistics. Nolan, J.L. (2001). Reinventing Justice: The American Drug Court Movement. Princeton, NJ: Princeton University Press. Nurco, D. N., Kinlock, T. W., & Hanlon, T. E. (1990). The Drugs Crime Connection. In, J. A. Inciardi (Ed.), Handbook of Drug Control in the United States. Westport, CT: Greenwoood Press, 71-90. Peters, R.H. & Murrin, M.R. (2000). Effectiveness of Treatment-Based Drug Courts inReducing Criminal Recidivism. Criminal Justice and Behavior References 79 27, 1, 72-96. Petersilia, J., Turner, S. & Deschenes, E.P. (1992). The Costs and Effects of Intensive Supervision for Drug Offenders. Federal Probation, 35, 4, 1217. Reuter, P., MacCoun, R., & Murphy, P. (1990). Money from Crime. Santa Monica, CA: RAND. Rhodes, W., Layne, M., Johnston, P., & Hozik, L. (2000). What America’s Users Spend on Illegal Drugs: 1988-1998. Washington, DC: Office of National Drug Control Policy. Rossman, S.B., Zweig, J. & Roman, J. (2008). A Portrait of Adult Drug Courts. Washington, DC: The Urban Institute. Senjo, S, & Leip, L.A. (2001). Testing Therapeutic Jurisprudence Theory: An Empirical Assessment of the Drug Court Process. Western Criminology Review, 3, 1, . Siddall, J.W., & Conway, G.L. (1988). Interactional Variables Associated with Retention and Success in Residential Drug Treatment. International Journal of the Addictions, 23, 12, 1241-1254. Simpson, D.D., Joe, G.W., & Broome, K.M. (2002). A National 5-year Followup of Treatment Outcomes for Cocaine Dependence. Archives of General Psychiatry, 59, 538-544. Simpson, D.D., Joe, G.W., & Brown, B.S. (1997). Treatment Retention and Follow-up Outcomes in the Drug Abuse Treatment Outcome Study (DATOS). Psychology of Addictive Behaviors 11, 294-307. Simpson, D.D., Joe, G.W., Fletcher, B.W., Hubbard, R.L., & Anglin, M.D. (1999). A National Evaluation of Treatment Outcomes for Cocaine Dependence. Archives of General Psychiatry, 56, 507-514. Slobogin, C. (1995). Therapeutic Jurisprudence: Five Dilemmas to Ponder. Psychology, Public Policy and Law, 1, 193-196. 80 To Treat or Not to Treat Speckert, G.R., & Anglin, M.D. (1986). Narcotics and Crime: A Causal Modeling Approach. Journal of Quantitative Criminology, 2, 3-28. Stewart, D., Gossop, M., Marsden, J. & Rolfe, A. (2000). Drug Misuse and Acquisitive Crime Among Clients Recruited to the National Treatment Outcome Research Study (NTORS). Criminal Behaviour and Mental Health, 10, 10-20. Tonry, M. (1990). Stated and Latent Functions of ISP. Crime and Delinquency, 36, 1, 174-191. Trone, J., & Young, D. (1996). Bridging Drug Treatment and Criminal Justice.Vera Institute Program Brief. New York: Vera Institute of Justice. Turner, S., Petersilia, J. & Deschenes, E.P. (1992). Evaluating Intensive Supervision Probation/Parole (ISP) for Drug Offenders. Crime and Delinquency, 38, 4, 539-556. Vito, G. F. (1989). The Kentucky Substance Abuse Program: A Private Program to Treat Probationers and Parolees. Federal Probation, 65-72. Wexler, D.B. & Winick, B.J. (1991). Therapeutic Jurisprudence as a New Approach to Mental Health Law Policy Analysis and Research. University of Miami Law Review 979. Wilson, D.B., Mitchell, O. & MacKenzie, D.L. (2006). A Systematic Review of Drug Court effects on Recidivism. Journal of Experimental Criminology, 2, 4, 459-487. Appendix A Mathematical Appendix A.1. INTERPOLATION TECHNIQUE For all of the modeling work performed in this paper, we use a semi-parametric interpolation technique. Since we are interested in generating a synthetic data that resembles the evidence in several different sources as closely as possible, it would be desirable to allow non-linear, flexible links among the attributes and the estimated quantities. To do so, we utilize an approach which, like nonparametric regressions, computes expectations about an outcome as a weighted average of observed outcomes. The weights reflect the empirical similarity of a profile and an actual case in the data being used. The intuition behind this strategy is similar to the theory of case based reasoning among humans (Gilboa and Schmeidler 1995, 1997, 2001). When we encounter a new situation, a heuristic approach to forming expectations is to think back on our experience and assess the empirical similarity of this new situation to our experience. Gilboa, Lieberman, and Schmeidler (2006) provide several examples of this form of reasoning in everyday decision-making, and lay out its axiomatic foundation. For our analysis, we use the approach to merely interpolate data onto the synthetic dataset. One major advantage of the strategy is that we are able to uncover and use 81 82 To Treat or Not to Treat non-linear relationships without explicitly specifying complicated functional forms. The learning strategy is a localized one that uncovers such non-linear links, if they exist, automatically. It is similar, in spirit, to non-parametric regression and kernel based smoothing techniques (Silvernam 1986; Loader 1999; Pagan and Ullah 1999; Fan and Yao 2003). A brief explication of our approach follows. Let xkn and xk m represent the values of the kth attribute of any two sample members (indexed by n and m where both n, m = 1, . . . , N ). The empirical similarity weights between units n and all other units can be defined as: p mn = exp − m exp k − θk (xk n − xk m )2 k θk (xk n − xk m )2 (A.1) where θk are shrinkage parameters that must be estimated with the evidence to reflect the average amount of similarity in terms of the kth attribute. Our estimation procedure is similar to, and builds on, developments in the field of artificial intelligence (Huang, Kecman and Kopriva 2006). It falls under the general class of learning models dubbed unsupervised learning algorithms. It is designed to learn from the evidence (observed samples) to predict what can reasonably be expected to happen in hypothetical or unobserved instances. In order to make these predictions, the algorithm computes a similarity score for the hypothetical scenario to all scenarios already observed and makes the best guess based on that. The choice of θk parameters is crucial in the analysis. Appendix IV explain how these parameters are estimated and how the asymptotic standard errors for θk , as well as related quantities, are estimated. Similar to human learning, they are estimated by appropriately balancing the algorithm’s need to learn from the evidence, not memorize it. Once we have model estimates, i.e., once we have recovered the θˆk , we can compute a host of interesting quantities from the available evidence. For example, we can simulate the expected outcome (say y) for a profile as follows. Let the kth attribute for profiles j ∈ J be denoted by xk j . Armed with θˆk , we can 83 Mathematical Appendix compute the empirical similarity of this profile to all observations in the real data as exp − k θk (xk j − xk m )2 pj m = (A.2) 2 m exp − k θk (xk j − xk m ) and use the empirical similarity between the profile and the observed sample members to compute the expected outcome as y m ˆp j m yˆj = ∀ j ∈ J (profiles) (A.3) m Note, however, that the parameters are estimated just once and used to compute the empirical similarity of each and every profile to all observations in the sample. To see this, recall from (A.1) that the similarity between any two observations n or m is based only on xn and x m , and not any outcomes. Therefore, once the algorithm has been trained (i.e., θˆk estimated) it can be used to interpolate any number of outcomes onto the synthetic dataset. A.2. PREVALENCE ESTIMATION For each of the j = 1, . . . , J profiles, we are interested in estimating the annual number of arrestees nationwide (a j ). If we had this information directly in the re-weighted ADAM we could interpolate this variable directly onto the synthetic data. However, ADAM provides information on the number of times individuals were arrested in the year prior to the current booking ( bi where bi g t 1 ∀i ∈ N ). Using the empirical similarity between synthetic profiles and ADAM sample members, we compute the expected number of arrests for particular profiles as bˆ j = i bi ˆpi j . Note that individuals who are arrested more frequently ought to have a larger share of all arrests nationwide. Therefore, we next re-scale b j to mimic census numbers. This ensures that the total number of arrests across all profiles sums to the total number of adult arrests nationwide. 84 To Treat or Not to Treat Next, suppose that we can estimate a profile specific annual recapture rate (r j )—the proportion of all arrests in a year that are re-arrests. This would allow us to convert the number of arrests into the number of arrestees as a j = b j ∗ (1 − r j ) ∀j ∈ J (A.4) The intuition behind this equation is straight forward. If the re-capture rate is 0 then it must be the case that the number of arrestees are exactly equal to the number of arrests (since the probability of recapture is null). As the re-capture rate increase, the number of arrestees would be an appropriately scaled down version of the number of arrests. At the limit, the number of re-arrests will be equal to b j − 1 (i.e., the number of arrests less 1) so that, with a re-capture rate of r j = b j −1 bj , the number of arrestees a j is exactly 1 (i.e., all arrests are generated by just 1 arrestee being recaptured several times). We compute the expected re-capture rate also from the re-weighted ADAM data using bi . Note that the number of recaptures (re-arrests) is bi − 1. If we denote the hazard of a re-capture event at time t as hi (t ), then, by definition, the probability of recapture within the 1 year period can be computed as 1 hi (t ) d t 1 − exp − 0 1 since the survival probability is defined as exp − 0 hi (t ) d t . Moreover, since the hazard is an intensity function, the integrated hazard yields the number of events within a year. Since bi is the total number of arrests within the year, we 1 have 0 hi (t ) d t = bi − 1 and, therefore, we can define ri = 1 − exp(1 − bi ). Using the empirical similarity between synthetic profiles and ADAM sample members, we can now compute the expected re-capture rate for a particular profile as ˆr j = i 1 − exp(1 − bi ) ˆpi j . The expected number of arrestees of a particular profile can now be computed by plugging in estimates of b j and r j into (A.4). Furthermore, since ADAM has classifications for arrestees being at risk of 85 Mathematical Appendix drug dependence or abuse, we can convert these into two binary outcomes. Now, using the empirical similarity weights, we can generate estimates of the ˆ j (d )) or probability of an arrestee profile being at risk of drug dependence (π ˆ j (a)). When combined with aˆj we obtained estimates of the numabuse (π ˆ )) or abuse ber of potential clients at risk of drug dependence (ˆ ad j = aˆj π(d ˆ (ˆ aa j = aˆj π(a)). A.3. COMPUTING VARIANCE Formulas for computing the variance of the sum of independent random variables or scaled random variables are available in introductory statistics texts. For the product of a set of independent random variables, denoting xˆ the expectation and x˜ the variance of a random variable, we use the following formula provided in Goodman (1962). If y = k xk , then yˆ = k xˆk and y˜ = ˜k + xˆk2 − k xˆk2 . k x A.4. EMPIRICAL SIMILARITY WEIGHTS Let pnm reflect the empirical similarity between unit m and n for all n, m = 1, . . . , N units in the training sample. Then we wish to link these weights to a set of K attributes recorded, say, as xn = (xn1 , . . . , xnK ) for each unit. The Euclidean distance between any to units, along the K different dimensions, may be computed as φk n m = (xk n − xk m )2 so that we can compute the sum of p-weighted dis-similarity among the training sample units as: φk n m pn m n m If we wish these weights to be a root-N consistent estimates of the diversity among sample members, then a good sample analog of this quantity is σk2 / N 86 To Treat or Not to Treat where σk2 = 2 1 xk n − x k N n is the observed sample variance of the kth attribute and x k its mean. In order to ensure that the estimated weights provide this consistency, we therefore convert this requirement into a set of constraints that the weights must satisfy (in the sample). In other words, we require that σk2 / N = φk n m p mn n ∀k ∈ K (A.5) m If in addition, we wish to use the weights to compute expectations, we want them to be normalized–i.e., sum to 1. This results in another set of constraints 1= p mn ∀n ∈ N (A.6) m What we now have is an ill-posed inversion problem: many more unknowns (N ) than equations linking them (N + K). Typically, an infinite number of solutions will be consistent with the constraints. We need a way to choose among them. Information theory, an inter-disciplinary field that uses entropy and entropyrelated measures to quantify uncertainty, provides the philosophical justification to make this choice. Edwin Jaynes, a physicist, argued in a series of influential paper that when faced with a problem that has an infinite number of solutions we should choose the solution that is least informative while satisfying the constraints ( Jaynes, 1957a; Jaynes, 1957b). To operationalize such an agnostic approach, Jaynes needed some way to quantify the lack of information. Fortunately, within the context of a problem in communication theory, Shannon (1948) had, just a few years earlier, developed a precise definition of uncertainty and termed it Information Entropy. Given a set of J proper probabilities, Shannon defined the uncertainty captured in them as H (w) = − j w j log w j . In 2 87 Mathematical Appendix what has come to be known as the Maximum Entropy formalism, Edwin Jaynes proposed to use Shannon’s Entropy as the criterion to maximize, subject to all available constraints, in order to derive conservative inferences from the data. The problem of assigning values to our similarity weights then can be formulated as a constrained maximization problem and solved by the method of Lagrange. The primal Lagrange functions is: =− pnm log pnm + ηn (1 − nm n m and solving the first order conditions the solution: p mn = exp(− k ∂ ∂ p mn φk mn θk ) k φk mn θk ) m exp(− θk (σk2 / N − p mn ) + k = 0 and ∂ ∂ ηn φk mn p mn ) nm (A.7) = 0 simultaneously yields = Ω−1 exp(− n φk mn θk ) (A.8) k where θ1 , . . . , θK are the Lagrange Multipliers to be estimated and Ωn , termed the partition function, ensures that the weights are properly normalized. Note that this optimal solution can be inserted back into the primal to derive the corresponding dual objective functions: θk σk2 / N + = k log Ωn (A.9) n This is an unconstrained minimization problem in the unknown Lagrange Multipliers that can be solved in a variety of software fairly easily. Note that once the Lagrange Multipliers are estimated, they can be ported to the validations samples and similarity weights can readily be computed for each sample member or can be used to assess the similarity/dissimilarity between a new observations (or a profile) and the entire training data. Suppose we denote a new observations with the index j , i.e., this observations has attribute profile x j . Then we can compute how similar this individual 88 To Treat or Not to Treat is to the training sample by plugging his/her attribute set into (A.8) to get: ˆp j m = Ω−1 exp − j θk (xk j − xk m )2 ∀m ∈ N (A.10) k Since they are normalized, they will yield weights that can be applied to compute expectations using past outcomes for the training sample (i.e., ∀m ∈ N ). Therefore, a data-driven expectations for an outcome for this individual can be computed as: ym p j m (A.11) yˆj = m The dual objective function (A.9) is an unconstrained optimization problem in the parameter vector θ1 , . . . , θk . It falls under the general class of extremum estimators. The consistency and asymptotic normality of these estimators can be established under fairly general regularity conditions (Mittelhammer, Judge, and Miller 2000,132–139). One way to assess the sampling variability of the Lagrange Multipliers is to compute the negative inverted Hessian of the dual objective function. That is, ΣΘ = − ∂2 ∂ Θ∂ Θ −1 (A.12) where Θ = (θ1 , . . . , θK ) . ΣΘ can be used to assess the sampling variability of the Θ. But, more importantly, it can be used to construct asymptotic standard errors around the various expectations derived in this paper. Consider, for example, the quantity yˆj defined in (A.11). We have defined it as the expected outcome for profile j . Since the algorithm has used a single data set, we need a way to assess the sampling variability of this quantity. To do so, we use the Delta-method (Greene 2000,357). Given an estimate of the asymptotic covariance of Θ, we can approximate the covariance of any non-linear function 89 Mathematical Appendix of it, e.g., yˆj , as follows: y˜j = ∂ yˆj ∂Θ ΣΘ ∂ yˆj (A.13) ∂Θ Using the definitions of yˆj from (A.11) and the definition of ˆp j m from (A.10), we can derive ∂ yˆj ∂Θ m [x j − x m ]2 ˆp j m y m ˆp j m y m [x j − x m ]2 ˆp j m − =− m (A.14) m and use that in (A.13) to approximate the asymptotic variance of each quantity interpolated onto the synthetic data. To show that the approach does successfully uncover hidden signals, even from a small training data set (N = 200) with sufficient noise, we performed a small simulation exercise. We generated data from the following process: y = sin(2 × π × (1 − x)2 ) + ε (A.15) where x ∼ UNIFORM(0, 1) is some profile variable, y is some outcome variable, and ε ∼ NORMAL(0, 1). After estimating the lagrange multiplier using only the information in the x variable, we recovered the underlying signal. The true signal, the noisy data, and the recovered signals are displayed in the figure A.1. As is evident, the algorithm provides a very robust method for uncovering hidden relationships between the variables—if such relationships truly exist. 90 Some Outcome Variable To Treat or Not to Treat True Signal Recovered Signal Noisy Data Some Profile Variable Figure A.1: Successfully recovering hiddens signals using empirical similarity weights: An example.