Ui Crimes Averted by Incapacitation 2007
Download original document:
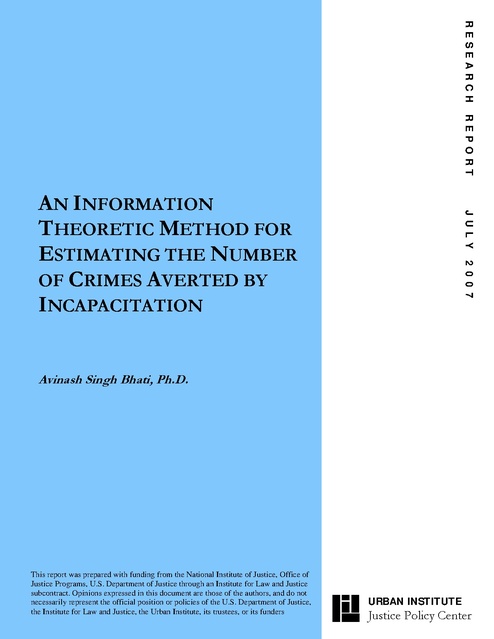
Document text
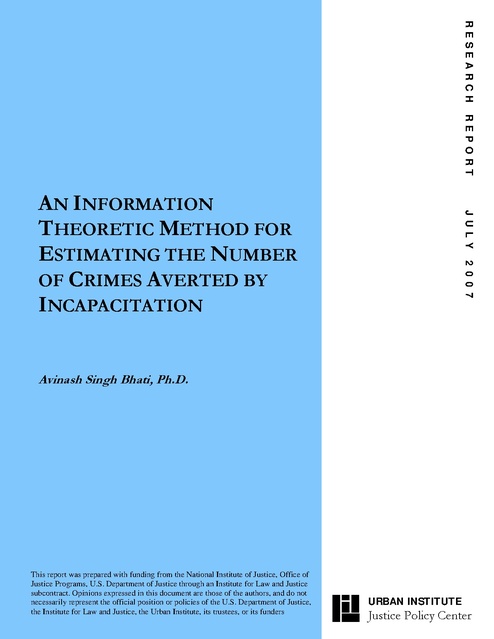
Document text
This text is machine-read, and may contain errors. Check the original document to verify accuracy.
RESEARCH REPORT JULY AN INFORMATION THEORETIC METHOD FOR ESTIMATING THE NUMBER OF CRIMES AVERTED BY INCAPACITATION 2007 Avinash Singh Bhati, Ph.D. This report was prepared with funding from the National Institute of Justice, Office of Justice Programs, U.S. Department of Justice through an Institute for Law and Justice subcontract. Opinions expressed in this document are those of the authors, and do not necessarily represent the official position or policies of the U.S. Department of Justice, the Institute for Law and Justice, the Urban Institute, its trustees, or its funders URBAN INSTITUTE Justice Policy Center URBAN INSTITUTE Justice Policy Center 2100 M Street NW Washington, DC 20037 www.urban.org © 2007 Urban Institute Opinions expressed in this document are those of the authors, and do not necessarily represent the official position or policies of the U.S. Department of Justice, the Institute for Law and Justice, the Urban Institute, its trustees, or its funders. AN INFORMATION THEORETIC METHOD FOR ESTIMATING THE NUMBER OF CRIMES AVERTED BY INCAPACITATION Avinash Singh Bhati abhati@ui.urban.org / (202) 261-5329 Justice Policy Center, The Urban Institute 2100 M Street, N.W., Washington, D.C. 20037 July 2007 Abstract This report describes an information theoretic approach for estimating the number of crimes averted by incapacitation. It first develops models of the criminal history accumulation process of a sample of prison releasees using their official recorded arrest histories prior to incarceration. The models yield individual offending trajectories that are then used to compute the number of crimes these releasees could reasonably have been expected to commit had they not been incarcerated—the counterfactual of interest. The modeled links between age, arrest number, time since last arrest, and the offending hazard afford the opportunity to conduct a limited set of policy simulations. Although a fair amount of heterogeneity is found, estimated incapacitation effects and simulated elasticities do not vary sufficiently by gender, race or ethnicity. Variations across states and offense types are more pronounced. Implications of the findings and promising avenues of future research are discussed. Contents Acknowledgments iv 1 Introduction 1.1 Overview . . . . . . . . . . . . . . . . . . . . . . . . . . . . . . . . . . . . 1.2 Background and Motivation . . . . . . . . . . . . . . . . . . . . . . . . 1 1 2 2 The Analytical Framework 2.1 Generating Plausible Counterfactuals . . . . . . . . . . . . . . . . . . 2.2 From Arrest Events to Offending Hazards . . . . . . . . . . . . . . 2.3 Policy Simulations . . . . . . . . . . . . . . . . . . . . . . . . . . . . . . 7 7 11 12 3 The Data 14 4 Findings 4.1 Crimes Averted by Incapacitation Estimates . . . . . . . . . . . . . 4.2 Incapacitation Elasticity Estimates . . . . . . . . . . . . . . . . . . . . 18 21 25 5 Conclusion 5.1 Summary . . . . . . . . . . . . . . . . . . . . . . . . . . . . . . . . . . . . 5.2 Future Research . . . . . . . . . . . . . . . . . . . . . . . . . . . . . . . . 29 29 31 References 34 A Mathematical Appendix A.1 Nonparametric Estimates . . . . . . . . . . . . . . . . . . . . . . . . . A.2 A Semiparametric Reformulation . . . . . . . . . . . . . . . . . . . . A.3 The Information-Theoretic Solution . . . . . . . . . . . . . . . . . . 41 41 44 46 i List of Figures 4.1 4.2 4.3 4.4 4.5 The composite and clock-specific offending micro-trajectories for a specific offender profile from California, all crimes. . . . . . . . Distribution of the estimated number of crimes against persons averted annually by incapacitation. . . . . . . . . . . . . . . . . . . . Distribution of the estimated number of property related crimes averted annually by incapacitation. . . . . . . . . . . . . . . . . . . . Distribution of the estimated elasticities of the incapacitation effect to enhanced sanctions, crimes against persons. . . . . . . . . . Distribution of the estimated elasticities of the incapacitation effect to enhanced sanctions, property related crimes . . . . . . . . . ii 20 23 23 26 26 List of Tables 4.1 4.2 4.3 Parameter estimates for simulating the criminal history accumulation process of releasees in California, all crime types. . . . . . . Annual number of crimes averted by incapacitation, distributed across states, crime types, and select demographic attributes. . . . Estimated elasticity of crimes averted by incapatitation to enhanced sanction, distributed across states, crime types, and select demographic attributes. . . . . . . . . . . . . . . . . . . . . . . . . . . . 19 24 27 A.1 An example of creating the y r mn and d r mn flags from arrest profiles. 43 iii Acknowledgments This research was supported by funding from the National Institute of Justice, Office of Justice Programs, U.S. Department of Justice through an Institute for Law and Justice subcontract agreement dated January 17, 2006. Points of view expressed here are those of the author and do not represent the official positions or policies of the U.S. Department of Justice, the Institute for Law and Justice, the Urban Institute, or its trustees and funders. A condensed version of this report is forthcoming in the Journal of Quantitative Criminlogy special issue on Incapacitation (Bhati 2007). Peter Reuter, Shawn Bushway, Christy Visher, Dan Mears, and Jennifer Yahner provided very thoughtful comments on earlier drafts of that paper, as did three anonymous reviewers. This research effort has benefitted greatly from their suggestions as well as feedback received from participants at the 2006 Criminology and Economic Summer Workshop (Queenstown, MD) and the 2006 American Society of Criminology annual meeting (Los Angeles, CA). The author is solely responsible for any remaining errors. iv Chapter 1 Introduction 1.1. OVERVIEW With an abandoning of the rehabilitative ideal in the 1970’s, incarceration has increasingly been justified as a means of removing individuals from society and incapacitating them, thereby preventing them from committing crimes they otherwise would have, had they been free to do so (Spelman 1994; Zimring and Hawkins 1995). Reliance on this strategy during the 1980’s and 1990’s resulted in a ballooning prison population with record number of offenders being incarcerated. The number of persons incarcerated in state or federal prisons grew from about 200,000 in 1973 to 1.4 million in 2003 (Pastore and Maguire 2005, 500). Before 1970, this number had remained fairly stable since the early 1930’s (Blumstein and Cohen 1973). Without a doubt, incapacitating criminals by imprisoning them does avert some crime (Spelman 2000). Knowing exactly how much crime is averted by incapacitation is, of course, impossible. Since incarcerated individuals cannot be in prison and in society at the same time, any claims about the number of crimes averted by incapacitation must, by definition, be based on counterfactual reasoning—reasoning that runs something like this: Had the individual not been incarcerated for z months, he or she would have committed x number of 1 Avi Bhati / Crimes Averted by Incapacitation 2 crimes over that period. Different ways of estimating the incapacitation effect can, therefore, be seen as alternate ways of generating this counterfactual. Despite the opposing views held by Blumstein and Piquero (2007), on the one hand, and Miles and Ludwig (2007), on the other, all would agree that there are several practical difficulties in generating a plausible counterfactual, especially if one believes that for policy purposes a single estimate is inadequate. This report aims to tackle this practical difficulty by generating the counterfactuals at the individual level. This is an important step forward that is needed to perform realistic policy simulations. The emphasis here is on linking the counterfactuals with important attributes like age and criminal history so that computations can take into account when in an offender’s life and criminal career this incapacitation occurs. Such details offer clearer insights not only into the anticipated crime reduction benefits associated with various incarceration strategies but also the distribution of the estimated incapacitation effect across offenders. Although incarceration can, and presumably does, have other effects on crime, for example through general and specific deterrence or deviance amplification, this report addresses only the incapacitation effect. Applying the framework developed here for recovering the deterrence or criminogenic effects of incarceration at the individual level is possible; those extensions are currently being developed. The report begins with a brief review of the literature, with an eye toward motivating this work. That is followed by a non-technical overview of the analytical framework. After describing the data used in this study, the report then presents the main findings with a discussion of their implications. It concludes with a discussion relating findings reported here with those reported elsewhere in the literature, and enumerates some promising directions for future research. 1.2. BACKGROUND AND MOTIVATION As noted above, all attempts at computing the number of crimes averted by incapacitation are really attempts at generating plausible counterfactuals. Early Avi Bhati / Crimes Averted by Incapacitation 3 attempts at generating this counterfactual relied on the mathematical model developed in Avi-Itzhak and Shinnar (1973) and Shinnar and Shinnar (1975). This model combines estimates of the annual offending rate (popularly denoted λ), the probabilities of arrest, conviction, and incarceration (given crime commission), and the expected incarceration term (conditional on being incarcerated). Combination of these quantities with estimates of the typical period for which offenders remain active can yield, under a host of assumptions, estimates of the number of crimes averted by incapacitation (Cohen 1978, 1983; Visher, 1987). One of the crucial inputs into this model is the offending rate while free— the λ. Researchers have used one of two approaches to estimate its value. They have either surveyed populations of offenders directly (Peterselia, Greenwood and Lavin 1978; Chaiken and Chaiken 1982; DiIulio 1990; Horney and Marshal 1991) or they have used official arrest records to estimate it indirectly (Greenburg 1975; Blumstein and Cohen 1979; Cohen 1986). Both approaches have their benefits and drawbacks (Blumstein, Cohen, Roth and Visher 1986). However, both approaches are concerned with estimating a value of a mean λ (or one that varies by offense categories) so that policy simulations can be conducted using the Avi-Itzhak and Shinar steady-state model. Despite its mathematical elegance, the steady-state model lacks two realistic feature—(i) the heterogeneity of offending rates among individuals and (ii) variations in the offending rate over the life course—that should be crucial for generating simulated impacts of policy choices. Offenders commit crimes at different rates (Nagin and Land 1993; Nagin and Paternoster 2000) and individuals’ offending rates evolve over their life course (Sampson and Laub 2005). Therefore, estimating how many crimes are averted (or can be averted under a simulated policy) must depend crucially on when in individuals’ lives and at what point in their criminal careers the incapacitation happens. To the extent that realistic models that link offending rates with offenders’ ages (i.e., how far along in their life they are) and criminal histories (i.e., how far along in their criminal career they are) can be generated, simulations from these models may provide more de- Avi Bhati / Crimes Averted by Incapacitation 4 tailed and more meaningful estimates of the number and distribution of crimes averted by incapacitation. In this report, I develop and apply one such model. I use a semiparametric approach to estimate the model using detailed dated arrest histories of a sample of prison releasees. Once estimated, I use the model to project a unique offending trajectory for each offender during the period he or she was incapacitated. These projections—the counterfactual micro-trajectories—form the basis for estimating the number of crimes averted by incapacitation. When normalized by their respective incarceration lengths, the model produces estimates of the annual number of crimes averted by incapacitation for each individual in the sample. Obtaining estimates for each individual in the sample has three direct benefits. First, the full distribution of the number of crimes averted by incapacitation can be generated using knowledge only of the way they were accumulating their criminal histories. This means incapacitation effects can be assessed across demographic and other subgroups and studied in detail. Any differences found among these groups can then be attributed to differences in their offending patterns since controversial demographic attributes like gender, race, and ethnicity are not included in developing the models. Second, an expanded array of policy simulations become possible. These include, for example, simulating the percent reduction in crime to be expected by increasing every releasee’s prison term by one percent. This quantity—the elasticity of the incapacitation effects to increases in prison term—is the appropriate quantity to assess when attempting to simulate the effects of increasing the punitiveness of current sanctioning policies. In a similar manner, one may simulate the effects of increasing sanction punitiveness by a fixed amount across offense and offender type. All the elasticities computed from these simulations are generated at the individual level and, therefore, allow an assessment of the full distribution across various offender subgroups. Third, the model provides an alternate criteria for assessing the viability of Avi Bhati / Crimes Averted by Incapacitation 5 selective incapacitation strategies. Since simulated elasticities vary across persons, rather than use the size of the expected incapacitation effect (akin to the λ) as the criterion for identifying individuals for selective incapacitation, one may use the efficiency of elongating incapacitation as the criterion on which to identify individuals for enhanced sanctions. Similarly, the strategy may be used to identify individuals for reduced sanctions based on the inefficiency of their current incarceration. In this report, I use the framework to produce estimates only of the distribution of crimes averted by incapacitation and the elasticities of this effect to altered prison terms.1 Chief among the limitations of this analytical strategy is that any estimates obtained from it are generalizable only to a population of prison releasees. If, for example, interest centers on assessing the effects of increasing the incarceration rate—e.g., by relying on incarceration more often than, say, community supervision—then this population is clearly inappropriate. Re-weighting the empirical distributions (reported in this report) to reflect other relevant populations is possible, but left for future work. If, on the other hand, interest centers on computing the number of crimes averted under current incarceration policies or on simulating the effects of altering current incarceration terms, then the population of releasees is an appropriate one. In this document, analysis, interpretations and discussion are restricted only to the population of releasees. The framework develop here can be extended to make inferences about the population of convicted individual who are not incarcerated by using inverse probability weighting techniques. In short, if estimates can be generated for each individual’s probability of incarceration (i.e., being selected in the sample), then these selection probabilities (of normalized versions thereof) can be used to inversely weigh sample member to make inferences about the population of 1 Identifying and predicting individuals for selective incapacitation strategies—be they for enhanced of reduced sanctions—is beyond the scope of this research effort. Indeed, here I go only so far as to suggest that the efficiency of incapacitation may serve as an alternate criteria. It is very possible that identifying such individuals a-priori may be as or more problematic as identifying the high λ offenders. Avi Bhati / Crimes Averted by Incapacitation 6 interest. Essentially, sample members that have a higher probability of being selected into the sample are given relatively lower weight than sample members who have a high probability of not being incarcerated—those sample members that “look more like” the population of interest. This additional analysis is currently under way and not reported here. Chapter 2 The Analytical Framework This chapter provides an intuitive, non-technical overview of the Information theoretic method used for generating the counterfactual offending trajectories for each individual in the sample. Detailed derivations of the model are provided in an appendix. 2.1. GENERATING PLAUSIBLE COUNTERFACTUALS In order to simulate counterfactuals at the individual level, what is needed is a dynamic model of the offending rate (or the λ) that is related to appropriate time-indexed variables as well as a set of offender-specific attributes. Links to the time-indexed variables will allow a simulation of the offending hazard as time passes. Links to offender-specific attributes will ensure that this process captures any population heterogeneity in the process. The analytical strategy described in this report utilizes information available in the criminal history accumulation process—i.e., how individuals were accumulating their respective criminal histories—to estimate the links between the offending hazard, the timeindexed variables, and offender attributes. Guidance on which time-indexed variables and which offender atributes to use in constructing the model can come either from formal theoretical reasoning 7 Avi Bhati / Crimes Averted by Incapacitation 8 or from exploratory empirical analysis. For example, it is a well established fact in criminology that the rate of offending increases as youthful offenders age but that, at some point, the rate begins to decline. This non-monotonic shape (first increasing then decreasing)—termed the “age-crime curve”—is a very predictable aspect of offending over the life course (Farrington 1986; Brame, Bushway, and Paternoster 2003; Bushway, Brame, and Paternoster 2004). Hence, the hazard model that we eventually develop must be consistent with this fact— i.e., it should exhibit a non-monotonic evolution with age. In a similar manner, it is often observed in recidivism studies that there exists duration dependence in criminal recidivism. That is, the hazard of re-offending may decrease (or increase) as time between events—the spell-length—increases (Maltz 1984; Allison 1995). Therefore, another fact that the model should be consistent with is this dependence of the hazard on time since the last arrest event. Other theoretical guidance or empirical regularities may exist that suggest how the hazard should evolve with time. The crucial question then is: How do we develop a hazard model that exhibits all of these dynamic features? To do so, the first task is to define all of the criterion variables (or outcomes) that the hazard model is being designed to predict. Assume there exists detailed dated information on the arrest sequence of individuals, along with their date of birth. This information allows us to construct a sequence of arrest ages. These sequences tell us exactly at what age the offender was arrested for the first, second, or subsequent time. Harding and Maller (1997) refer to these sequences as offenders’ arrest profiles. It is also straightforward to convert these sequences into a variable measuring elapsed time between successive arrest events. In a similar manner, we can develop measures of all the relevant “clocks” that may be needed to accurately describe the evolution of the hazard rate with time. The ultimate goal is to construct a model (for λ) that evolves along these multiple clocks. Such multiple-clock models allow researchers to capture several dimensions of time simultaneously when studying event histories (Yamaguchi 1991, Avi Bhati / Crimes Averted by Incapacitation 9 53; Lillard 1993). Next, we need some way to relate λ to the evidence we have in the sample. If we believe that λ increases or decreases with some variable x (e.g., age, spelllength, arrest number, etc.) then, at a minimum, λ should covary with x. But by how much? Provided that the sample is a random drawing from the population of interest, one may assume that the best estimate of this covariation is to be found in the sample itself. This principle, termed the analogy principle (Manski, 1988), suggests that the expected covariance between x and λ should be equal to the actual covariance between x and the timing of arrest events observed in the sample. Such reasoning allows us to derive a set of constraints that the hazards should satisfy, irrespective of their functional form. These constraints, however, are not sufficient to identify (yield a precise mathematical form for) the model. Typically, an infinite number of hazard paths will be consistent with the arrest patterns in the sample. We need a way to choose among them. Information theory, an inter-disciplinary field that uses entropy and entropyrelated measures to quantify uncertainty, provides the philosophical justification to make this choice. Edwin Jaynes, a physicist, argued in a series of influential papers that when faced with a problem that has an infinite number of solutions (the so-called ill-posed inversion problems) we should choose the solution that is least informative (or closest to our prior beliefs, if any) while satisfying what limited evidence we may have observed ( Jaynes 1957a,b). To operationalize such an agnostic approach, Jaynes needed some way to quantify the lack of information. Fortunately, within the context of a problem in communication theory, Shannon (1948) had, just a few years earlier, developed a precise definition of uncertainty and termed it Information Entropy. In what has come to be known as the Maximum Entropy formalism, Edwin Jaynes proposed to use Shannon’s Entropy as the criterion to maximize, subject to all available constraints, in order to derive conservative inferences from the evidence.1 1 The field of Information and Entropy Econometrics has grown exponentially over the two decades since econometricians were first introduced to this approach by Arnold Zellner and his 10 Avi Bhati / Crimes Averted by Incapacitation In our analysis, since there are an infinite number of hazard paths that could have generated the observed arrest histories, following Jaynes’ reasoning, the optimal choice among them should be the set of individual paths that are the least informative. Therefore, if we can quantify the uncertainty implied by the hazards then the conceptual solution suggested by Jaynes can be formulated as a constrained optimization problem. Solving this problem by variational methods yields a dynamic solution for the hazard rate that is the most conservative among all of the models consistent with observed arrest patterns. Full mathematical derivation of the solution is provided in the appendix. The resulting model that emerges from the approach takes the functional form: λn (z) = exp xn θ0 + z xn θ1 + z log z xn θ2 + vn (z) xn θ3 ∀n ∈ N , (2.1) where xn is a vector of offender attributes, θ0 , . . . , θ3 are a set of Lagrange Multipliers (a bi-product of solving any constrained optimization problem) that reflect the value of each of the constraints on reducing uncertainty about the process; z captures the evolution of the hazard linearly with age; z log z captures the nonmonotonic shape of the hazard (provided that θ2 have the opposite sign of θ1 ); and vn (z) captures the dependence of the hazards on the time since last arrest (if θ3 are nonzero). The semiparametric nature of the approach stems from the fact that rather than make assumptions about the form of the hazard function, we recover the functional form from the imposed constraints directly. Therefore, any arbitrary set of constraints may be imposed. If they are irrelevant, then the corresponding Lagrange Multipliers will be close to zero. As with fully parametric models, asymptotic standard errors can be derived for these parameters and they can be subjected to standard statistical significance testing (Kullback 1959). It is important to note that this approach differs, both conceptually and empirically, from existing methods of modeling repeated events (Allison 1984; Blossfeld, Hamarele, and Mayer 1989; Mayer and Tuma 1990). Application of collegues (Zellner and Highfield 1988; Zellner 1991; Ryu 1993). Avi Bhati / Crimes Averted by Incapacitation 11 the information theoretic approach yields the form of the hazard trajectories as well as estimates for the parameters θ0 , . . . , θ3 . Moreover, under certain restrictive assumptions the information theoretic approach can yield functional forms and inferences identical to fully-parametric repeated event models. As such, the approach can yield models that encompass one or more fully-parametric models as special cases. The key conceptual distinction is that, in the information theoretic approach, the point of departure is the theoretical or empirical guidance regarding multiple clocks or moments. Once the θ parameters are recovered by solving the optimization problem, simulating the evolution of the hazard with age or time since last arrest, conditional on a given set of offender attributes, is simply a matter of plugging in the appropriate quantities into (2.1) and computing the hazard micro-trajectories for each individual. 2.2. FROM ARREST EVENTS TO OFFENDING HAZARDS Since the data typically available to analysts contains dated arrest sequences, the approach described above would yield, unless modified, estimates of the microtrajectories of arrest hazards. Our main interest, however, is in estimating the number of crimes averted by incapacitation. Therefore, we need some way to estimate offending hazard paths from observed arrest events. In order to do so, following Blumstein and Cohen (1979), a correction factor (denoted c) was first defined. With it, the analytical framework sketched above can be extended in a straightforward way to recover offense-specific trajectories of offending hazards rather than arrest hazards. It should be noted here that the strategy entails adjusting the event histories by this correction factor (at the micro-level) before estimating θ and not adjusting the crimes averted estimates after their computation. See the mathematical appendix for complete details. Data on number of charges (h) of crime category l (available for each arrest event), the crime clearance rate (b ) for various years during which arrest histories are observed (available annually by crime categories), the crime reporting 12 Avi Bhati / Crimes Averted by Incapacitation rate (e) by age of offender and crime categories, as well as crime category-specific co-offending rates (o) can be used to compute the correction factor as follows: c= h b ×e ×o . (2.2) Auxiliary data sources used to obtain each of these quantities for this study are provided in the next chapter. In the final models, what distinguishes each of the trajectories of different ˆ ,...,θ ˆ ∀l ∈ crime categories are the estimated parameters (now denoted θ 0l 3l L). Once these parameters are estimated, the total number of crimes of type l averted by incapacitating an individual (denoted ˆsnl ) between the ages of z n and z n can be estimated by integrating the hazard trajectory over that range. That is, ˆs l n = zn λˆl n (z) d z zn zn = zn ˆ +zx θ ˆ + z log z x θ ˆ + v (z) x θ ˆ dz exp xn θ 0l n n 1l n 2l n 3l ∀n, l . (2.3) Furthermore, dividing this number by the time spent in prison, i.e., z n − z n , yields an estimate of the annual number of crimes averted by incapacitation for each individual in the sample. 2.3. POLICY SIMULATIONS More interesting are the prospects of conducting detailed policy simulations. Simulating the effects of increasing (or decreasing) the prison term for each individual can be computed by altering the upper limit of integration by an appropriate amount. Given that the hazard’s solution is of an exponential form, increasing (decreasing) the range of integration is guaranteed to increase (decrease) Avi Bhati / Crimes Averted by Incapacitation 13 the crimes averted estimates by some amount. The question of interest is: by how much? The answer will depend on who the individual is (i.e., the set of attributes) and when during the career this increase takes place. If, as has been argued elsewhere (Blumstein and Piquero 2007), the enhanced incapacitation happens at a time when the offender would not have been active (or had a very low value of λ), then we should expect to see negligent increases in the number of crimes averted. To investigate this issue, the report presents the percent increase in the estimated number of crimes averted, had individuals served an additional 1 percent of their current incarceration term—the elasticity of the incapacitation effect to altered prison terms (ˆ η). This quantity, a unit-free measure of the responsiveness of the incapacitation effect to altered incarceration terms, is defined as z n +δn ˆ λ l n (z) d z zn ηˆl n = ∀l , n (2.4) zn ˆ λ (z) d z ln z n where δn represents one percent of the individual’s current incarceration term, i.e., (z n −z n )/100. Because individuals in a prison release cohort will have served varying lengths of prison terms prior to release, elasticities are a convenient way to standardize and compare the simulated effects of policy choices. Chapter 3 The Data The data used in this research effort are available to the public from the National Archives of Criminal Justice Data (NACJD), at the Inter-University Consortium for Political and Social Research (ICPSR), University of Michgan, Ann Arbor, MI. It is archived as study # 3355 (Recidivism of Prisoners Released in 1994 [United States] ) (BJS 2002). The data were collected by the Bureau of Justice Statistics (BJS). BJS tracked a sample of 38,624 prisoners released from 15 state prisons in 1994 for a period of 3 years. The vast majority of the archived database consists of information on each releasee’s entire officially recorded criminal history. This includes all recorded adult arrests through the end of the follow-up period. These data were obtained by BJS from state and FBI automated RAP sheets which include arrest, adjudication, and sentencing information. Each arrest event includes information on adjudication and sentencing related to that event if such action was taken. Unfortunately, however, the data do not contain detailed information on when these individuals were released from prison if they were imprisoned after a particular arrest event. This implies that the data are unable to calculate street time. This is a serious drawback of these data. In addition to the detailed dated event history data, this database also contains a limited amount of demographic and related information. Demographic 14 Avi Bhati / Crimes Averted by Incapacitation 15 measures available in the database include date of birth, race, ethnicity, and gender. Some detail is available about the type of release from prison (e.g., parole, mandatory release, etc.) and some about the type of admission into prison (e.g., new court commitment, new court commitment with a violation of conditions of release, etc.). However, this information is available only for the 1994 release and not for all prior (or future) arrest events. Before conducting the analysis, some diagnostic checks were run on the data to ensure they were compatible with the model requirements. Since the data are based on official records and possible disparate sources of date information (e.g., date of birth obtained from the state data and from the FBI data could differ), I first computed the ages for each of the arrests in the data. Then, I checked for the chronology of these dates and checked to see if the age variable was well defined. I created flags for any individual that had records that were not in proper chronological order or whose ages were incorrect/impossible (e.g., negative or below 15). In addition, I created flags that identified any individuals that were missing information on all ages or that had gaps in their age variable. For example, individuals that had appropriate ages for the first and second arrest events but were missing age on the third event and again had appropriate ages for all subsequent arrests were flagged as potentially problematic. After creating these flags, I performed a list wise deletion of persons—i.e., all records for individuals with any problem (as determined by the various flags) were dropped from the analysis set. This includes individuals excluded from BJS analysis for a variety of reasons (Langan and Levin 2002, 14). Because the California sample was very large (nearly 60,000 person-arrests before prison admission) I used a random subset of 2500 individuals (21,792 person events) from the California sample for estimating the criminal history accumulation process. For the simulation analysis, however, all individuals from California were included in the study. In addition, data for releasees from two states—Delaware and Maryland—were completely dropped from the analysis. Deleware’s sample was too small and convergence problem were encountered Avi Bhati / Crimes Averted by Incapacitation 16 in estimating some of the models. Maryland, on the other hand, lacked offense specific charge information. Since this information was crucial in estimating offense specific models, all records from Maryland were dropped. The final preincarceration sample used in the analysis consisted of 175,490 arrest events across 13 states. Arrest records for these persons were next re-structured into a hierarchical person-event level file. In addition to the key criterion variable—age at arrest, duration since last arrest, and number of charges for specific offense types at each arrest event—the data were also manipulated to create a set of individual level fixed covariates as well as covariates changing over time. The key independent variables used in estimating the criminal history accumulation process included the arrest number (EVENTNUM), the age at first arrest (AGE1ST), and age at last arrest (AGELAST). AGE1ST and AGELAST were set to 0 for the first arrest event. The same basic set of predictors were used to model all criterion variables and all states. In addition, to account for the lack of information to control for the street time issue, I include a flag indicating whether or not the last arrest event resulted in some confinement. Admission and release dates were used to construct the amount of time served in prison and age at admission. These variables were used in constructing the interval over which the hazard paths were integrated to compute the estimated crimes averted numbers. To obtain estimates of offending trajectories from arrest events, additional data on the number of crime cleared by arrest (by year and crime type), the rate at which crimes are reported to police (by offender age and crime type), and co-offending rates (by crime type) were used. Year and offense specific clearance rates were obtained from Table 4.20 in Pastore and Maguire (2005, 377). Reporting rates are computed from data provided in Hart and Rennison (2003). Co-offending rates of 2 for property crimes and 1.5 for crimes against persons were used (Reiss 1988). The number of charges of offense type l that were associated with each arrest (available in the BJS data) Avi Bhati / Crimes Averted by Incapacitation 17 was used to model offense type specific processes. Based on this additional data, a correction factor was computed for each individual at each arrest event and for each of the offense types analyzed (see technical appendix for details). Chapter 4 Findings In this chapter, I discuss the results obtained by applying the framework described in this report to the data described in the previous chapter. Because states vary in their penal policies and practicies, separate models were estimated for each of the 13 states included in this study. Separate models were also developed for crimes against persons, property related crimes, and all crimes combined. Although the parameter point estimates from these models varied considerably (both across states and offense type) their signs were largely consistent across samples. Hence, detailed estimates and interpretation of parameter signs are provided for only one model (all crime types for the California sample). ˆ as well as their asymptotic standard errors. Table 4.1 provides estimates of θ Since the samples include multiple arrest events per individual, standard errors need to be corrected for this clustering. The modified sandwich variance estimator (Ezell, Land and Cohen 2003)—a modified version of sandwich estimators (Huber 1967; White 1980) that account for this clustering—is used here. To get an intuition for what the parameter signs mean, consider the effects of age at first arrest on the process. Starting the criminal career later in life implies a permanently lower hazard (a negative θˆ0 ), with a steeper rise in offending hazard with age (a positive θˆ ), but for a shorter career (a negative θˆ ) relative 1 2 18 19 Avi Bhati / Crimes Averted by Incapacitation Table 4.1: Parameter estimates for simulating the criminal history accumulation process of releasees in California, all crime types. θˆ Covariates a.s.e.a χ2 p-val θ0 : Fixed Intercept Arrest Number Age @ 1st arrest Age @ last arrest Confined @ last arrest 2.444 0.051 -0.797 0.292 -0.151 0.494 0.051 0.043 0.018 0.032 24.46 0.99 339.44 249.98 22.93 0.00 0.32 0.00 0.00 0.00 θ1 : Age (linear) Intercept Arrest Number Age @ 1st arrest Age @ last arrest 0.777 -0.010 0.070 -0.035 0.075 0.007 0.005 0.003 108.12 2.19 191.49 172.66 0.00 0.14 0.00 0.00 θ2 : Age (non-linear) Intercept Arrest Number Age @ 1st arrest Age @ last arrest -0.218 0.003 -0.014 0.008 0.019 0.002 0.001 0.001 132.76 2.89 167.13 162.39 0.00 0.09 0.00 0.00 θ3 : Time since last arrest (linear) Intercept Arrest Number Age @ 1st arrest Age @ last arrest Confined @ last arrest -0.462 -0.028 -0.007 0.019 0.047 0.038 0.004 0.003 0.003 0.019 146.21 59.54 4.55 45.27 6.04 0.00 0.00 0.03 0.00 0.01 a Modified sandwich estimates to someone who starts offending much earlier. Moreover, starting the criminal career later in life implies stronger negative duration dependence—i.e., hazard drops more rapidly as time since the last arrest increases—relative to someone who starts their career earlier (a negative θˆ ). 3 In a similar manner, being confined at the last arrest reduces the hazard permanently (a negative θˆ0 ) but also reduces the amount of negative duration dependence (a positive θˆ3 ). These signs are consistent with a process where individuals are not at risk of being rearrested for a period after an arrest event that resulted in some confinement. By setting this variable to zero for all individuals in the sample while simulating their micro-trajectories, we are able to account for, albeit in a very rudimentary way, the lack of precise information on prior incarceration spells. 20 Avi Bhati / Crimes Averted by Incapacitation 5 Offender Profile AGE1ST AGELAST EVENTNUM CONFINEDLAST 4 = 19 = 25 = 3 = 0 Annual Offending Rate AGE-BASED CLOCK 3 MULTIPLE CLOCKS 2 1 SPELL-BASED CLOCK 0 18 20 Age @ First arrest = 19 22 24 Age @ Last Arrest = 25 26 28 30 32 34 Age @ Prison Admission = 25 36 38 Age @ Release in 1994 = 35 Time Served in Prison Figure 4.1: The composite and clock-specific offending micro-trajectories for a specific offender profile from California, all crimes. To provide more clarity on what these model estimates imply, a graphical depiction of the counterfactual micro-trajectory for a hypothetical offender profile is presented in figure 4.1. Consider a man who was arrested for the first time at age 19, was rearrested at age 25 at which point he was incarcerated for 10 years. Had he not been incarcerated (between ages 25 to 35) what would his offending trajectory have looked like? Given the parameter estimates in table 4.1, and these attributes, one can plot the counterfactual micro-trajectory for this individual. This plot, along with its two clock components, appears in figure 4.1. The two individual clock components are defined by rewriting (2.1) as follows: spell-based clock age-based clock λn (z) = exp xn θ0 + vn (z) xn θ3 exp z xn θ1 + z log z xn θ2 ∀n ∈ N (4.1) The plots in figure 4.1 show that, had this person not been incarcerated for 10 years at the age of 25, his offending rate would have continued to drop from Avi Bhati / Crimes Averted by Incapacitation 21 about 3.5 to about 0.5 by age 35. This drop is expected due to two stochastic processes at work. First, there is the anticipated age-crime curve effect (as shown in figure 4.1 by the age-based clock component, displayed with hollow circles). This individual’s anticipated age-based offending trajectory had almost peaked when he was arrested and incarcerated. However, the stronger effect is with spell length (as shown by the spell-based clock in figure 4.1, depicted by the filled circles). If this individual had not been incarcerated at age 25, then as time elapsed, the two stochastic processes would have jointly applied a negative pressure. Initially, though, the offender was young enough so that the slight upward pressure of the age-based clock was competing with the downward pressure from the spell-length clock. When combined, however, the two components represent a fairly dramatic downward trend in the offending trajectory expected for this individual between the age of 25 and 35. Much of the 10 years he was actually incarcerated for may have been inefficient use of prison space: at least for the last five of those 10 years (age 30–35), his offending rate was expected to be negligible in any case. Integrating this individual’s multiple-clock hazard path between ages 25 and 35 suggests that a total of 23.9 crimes were averted by his incarceration. This translates into an annual crimes averted by incapacitation estimate of 2.39 since he was incarcerated for 10 years. Note that this number representes the number of crime averted (not the number of arrests) since a correction factor has allready been introduced into the modeling exercise. Based on model estimates and offenders’ attributes, it is possible to plot such trajectories and perform such computations for each individual in the sample. The results are discussed next. 4.1. CRIMES AVERTED BY INCAPACITATION ESTIMATES Based on the simulated micro-trajectories, the integrated counterfactual, normalized by the incarceration terms, were computed for each individual in the sample Avi Bhati / Crimes Averted by Incapacitation 22 for the 3 offense types (crimes against persons, property related crimes and all crimes). Since there were a few large outlier estimates that would have skewed sample statistics (like the mean) all estimated means reported in this chapter are computed from a distribution truncated at the ninety-ninth percentile. Figure 4.2 shows the distribution of the annual number of crimes against persons averted by incapacitating the offenders released from all 13 states. As is expected, there is a distinct skew in the distribution of crimes averted by incapacitation with a mean of 1.93 and a median of 1.41. Only about five percent of the releasees would have committed more than five crimes against persons annually. In a similar manner, figure 4.3 shows the distribution of property related crimes averted by incapacitation. The distribution is, as expected, on a higher level with a mean of 8.47 and a median of 5.75 but the skew is still prominent. Only about five percent of the releasees would have committed an estimated 30 or more property related crimes annually, while most (roughly 75 percent) of them were expected to commit less than 10 property related crimes annually. In each of the distributions it is interesting to see that there are small proportions of releasees that were not anticipated to commit any crime. Although the graphical presentation of the distribution of crimes averted by incapacitation provides some insights, it would be interesting to see if there are any systematic difference among various offender subgroups. Towards that end, table 4.2 presents detailed state- and demographic subgroup-specific estimates of the annual incapacitation effects. In general, there seems to be little systematic difference among the various groups. With few exceptions, the annual number of crimes averted by incapacitating males is slightly higher than females. Casual inspecition of the results reported in table 4.2 suggests that there are little or no discernible substantive differences between groups based on race and ethnicity. There is, however, a fair amount of variation across states. For example, incarceration helped avert a large number of property related crimes in North Carolina annually—the most among all states. On the other hand, the most 23 Avi Bhati / Crimes Averted by Incapacitation 100 6 90 5 80 Empirical PDF (Left Scale) 60 50 3 40 Percent of Sample Percent of Sample 70 Empirical CDF (Right Scale) 4 2 30 Mean = 1.93 Median = 1.41 1 20 10 0 0 0 1 2 3 4 5 6 7 8 9 10 Crimes Against Persons Averted by Incapacitation, Annual Estimates Figure 4.2: Distribution of the estimated number of crimes against persons averted annually by incapacitation. 1.8 100 1.6 90 80 1.4 70 Empirical PDF (Left Scale) Empirical CDF (Right Scale) 60 1 50 0.8 40 Percent of Sample Percent of Sample 1.2 0.6 30 Mean = 8.47 Median = 5.75 0.4 20 0.2 10 0 0 0 2 4 6 8 10 12 14 16 18 20 22 24 26 28 30 Property Related Crimes Averted by Incapacitation, Annual Estimates Figure 4.3: Distribution of the estimated number of property related crimes averted annually by incapacitation. 24 Avi Bhati / Crimes Averted by Incapacitation Table 4.2: Annual number of crimes averted by incapacitation, distributed across states, crime types, and select demographic attributes. AZ CA FL IL MI MN NJ NY NC OH OR TX VA All Mean Med Males Females Mean Med Mean Med 17.01 13.55 23.63 17.37 13.64 11.55 13.83 11.14 6.51 5.36 11.58 9.94 16.35 14.02 16.24 14.19 24.14 18.25 10.22 7.89 24.48 16.03 9.19 7.69 11.33 9.65 17.05 23.73 13.59 13.91 6.63 11.59 16.40 16.37 24.03 10.23 24.15 9.24 11.42 AZ CA FL IL MI MN NJ NY NC OH OR TX VA 1.81 2.61 0.80 1.60 0.94 1.56 1.34 2.14 1.68 2.16 1.07 0.95 1.29 1.45 1.96 0.71 1.37 0.59 1.29 1.14 1.53 1.21 1.43 0.78 0.79 1.09 AZ CA FL IL MI MN NJ NY NC OH OR TX VA 7.69 9.83 5.05 7.22 2.93 6.67 8.71 7.67 15.26 4.33 7.37 4.30 6.24 5.78 6.56 4.16 5.05 2.17 5.67 7.29 5.97 11.60 3.23 4.42 3.41 5.05 Blacks Mean Med Nonblacks Mean Med Hispanics Mean Med Number of all crimes averted annually by incapacitation 13.57 16.60 13.40 16.71 13.99 17.07 13.49 16.04 17.50 22.64 14.89 25.54 18.66 22.69 16.66 22.37 11.48 14.03 12.98 13.25 11.25 14.16 11.93 13.13 11.23 12.63 10.42 14.28 11.23 12.97 11.01 13.31 5.43 4.99 4.50 6.52 5.37 6.50 5.35 ... 9.98 11.46 9.73 11.76 10.00 11.50 9.93 ... 14.05 15.76 13.63 16.63 14.45 15.78 13.61 15.96 14.30 14.57 12.08 16.65 14.82 15.74 13.46 15.69 18.29 24.87 18.13 24.00 18.47 24.37 17.91 ... 7.92 ... ... 9.47 7.37 10.90 8.44 ... 15.66 ... ... 28.68 20.43 23.70 15.53 18.52 7.72 8.61 7.28 9.28 7.63 9.09 7.79 8.77 9.76 10.35 8.71 11.32 9.72 11.34 9.62 ... Number of crimes against persons averted annually by incapacitation 1.80 1.45 1.91 1.35 1.61 1.27 1.85 1.47 1.76 2.62 1.97 2.52 1.84 2.70 1.99 2.57 1.94 2.47 0.81 0.71 0.75 0.68 0.77 0.68 0.85 0.76 0.85 1.62 1.37 1.36 1.23 1.59 1.34 1.63 1.39 1.62 0.95 0.60 0.70 0.48 1.01 0.60 0.84 0.58 ... 1.55 1.29 1.70 1.36 1.39 1.16 1.63 1.34 ... 1.35 1.14 1.22 1.09 1.34 1.15 1.36 1.09 1.34 2.19 1.56 1.49 1.21 2.29 1.62 1.96 1.42 1.93 1.66 1.21 1.84 1.18 1.66 1.22 1.73 1.17 0.94 2.17 1.43 ... ... 2.01 1.28 2.28 1.57 ... 1.06 0.77 ... ... 1.20 0.87 1.05 0.77 ... 0.96 0.79 0.82 0.78 0.96 0.78 0.95 0.79 0.89 1.30 1.10 1.11 0.95 1.30 1.09 1.26 1.09 ... 12.33 16.02 8.80 10.28 ... ... 13.91 13.46 ... ... 12.45 7.83 ... Non-Hisp Mean Med 17.44 14.14 24.22 17.98 13.66 11.61 13.88 11.15 6.51 5.36 11.62 9.98 16.43 14.11 16.52 14.66 24.16 18.29 10.27 7.92 25.12 16.75 9.30 7.68 11.34 9.72 1.40 1.82 0.70 1.37 ... ... 1.10 1.36 0.84 ... ... 0.79 ... 1.84 2.68 0.80 1.60 0.94 1.56 1.34 2.24 1.69 2.16 1.09 0.97 1.29 1.47 2.02 0.71 1.37 0.59 1.29 1.14 1.61 1.21 1.43 0.79 0.79 1.09 Number of all property related crimes averted annually by incapacitation 7.82 5.83 6.47 5.41 8.07 6.08 7.62 5.64 7.15 5.24 9.84 6.59 9.71 6.04 10.93 7.15 9.29 6.25 9.39 6.05 5.00 4.09 5.51 4.80 4.91 4.09 5.24 4.31 4.67 3.33 7.21 5.10 7.34 4.85 7.63 5.25 6.43 4.78 6.63 4.20 2.95 2.21 2.70 1.78 2.95 2.18 2.91 2.17 ... ... 6.70 5.74 6.16 4.68 7.18 6.06 6.44 5.47 ... ... 8.70 7.31 8.85 7.12 8.95 7.51 8.23 6.86 8.21 6.85 7.69 6.02 7.46 5.44 7.90 6.31 7.40 5.58 7.46 5.94 15.31 11.80 14.88 10.52 15.09 11.78 15.54 11.44 ... ... 4.31 3.28 ... ... 4.19 3.28 4.45 3.20 ... ... 7.30 4.37 ... ... 8.99 5.27 7.07 4.27 5.55 3.29 4.32 3.40 4.06 3.41 4.38 3.42 4.22 3.40 4.08 3.26 6.27 5.06 5.93 4.96 6.21 5.06 6.28 4.94 ... ... 7.94 10.03 5.06 7.27 2.93 6.69 8.82 7.78 15.27 4.35 7.57 4.36 6.24 6.00 6.85 4.17 5.10 2.17 5.69 7.38 5.99 11.60 3.28 4.60 3.42 5.06 ... Fewer than 100 obervations. Avi Bhati / Crimes Averted by Incapacitation 25 number of crimes against persons were averted in California. Examining the causes of the state variation uncovered here is left for future work as it would require careful modeling not only of state policy levers, but also variation in relevant offender attributes across states. The sample of offenders vary considerably across states with respect to attributes like age at release or age at first arrest that are included in the models. 4.2. INCAPACITATION ELASTICITY ESTIMATES The results discussed above were for estimated number of crime averted by the current incarceration periods. In what follows, the estimated model is used for simulating the effects of increasing prison terms for all individuals in the sample by one percent each and computing the elasticities as defined in (2.4).1 Figure 4.4 shows the distribution, across sample members, of the elasticities for crimes against persons and figure 4.5 shows the same for property related crimes. An increase of a prison term by one percent can be expected to bring about a roughly proportional increase in the number of crimes against persons averted. Although there are individuals on both tails of the distribution, it is interesting to note that the skew is in fact in the other direction—the mean is smaller than the median. However, the number of persons for whom the increased prison term would more than compensate for an increase in the crimes averted estimate is still small. Only 15 percent of the sample had an estimated elasticity greater than one (i.e., a one percent increase in their prison term would yield an increase in the estimated number of crimes averted by more than one percent). A very different story emerges when assessing the distribution of the incapacitation effect elasticities for property related crimes. Here, we find that nearly everyone (about 98 percent) had an elasticity of less then one. Moreover, among these the distribution shows a very fat tail. There is no clustering of individuals around the higher elasticity values. Instead, the empirical cumulative 1 The resulting estimates were also truncated at the ninety-ninth percentile prior to computing any sample statistics. 26 Avi Bhati / Crimes Averted by Incapacitation 9 100 8 90 80 7 Empirical CDF (Right Scale) 60 5 50 4 40 Percent of Sample Percent of Sample 70 Empirical PDF (Left Scale) 6 3 30 Mean = 0.93 Median = 0.95 2 20 1 10 0 0 0 0.2 0.4 0.6 0.8 1 1.2 1.4 1.6 1.8 2 Crimes Against Persons Averted by Incapacitation, Elasticity to Altered Prison Term Figure 4.4: Distribution of the estimated elasticities of the incapacitation effect to enhanced sanctions, crimes against persons. 3.5 100 90 3.0 80 Empirical PDF (Left Scale) 2.5 70 60 2.0 50 Mean = 0.69 Median = 0.76 1.5 40 Percent of Sample Percent of Sample Empirical CDF (Right Scale) 30 1.0 20 0.5 10 0.0 0 0 0.2 0.4 0.6 0.8 1 1.2 Property Related Crimes Averted by Incapacitation, Elasticity to Altered Prison Term Figure 4.5: Distribution of the estimated elasticities of the incapacitation effect to enhanced sanctions, property related crimes 27 Avi Bhati / Crimes Averted by Incapacitation Table 4.3: Estimated elasticity of crimes averted by incapatitation to enhanced sanction, distributed across states, crime types, and select demographic attributes. All Mean Med 0.91 0.89 0.74 0.82 0.95 0.87 0.88 0.63 0.97 0.92 0.70 0.92 0.83 Males Females Mean Med Mean Med Blacks Mean Med Nonblacks Mean Med Hispanics Mean Med Non-Hisp Mean Med Elasticity of all crimes averted by incapacitation to enhanced sanctions 0.84 0.91 0.89 0.91 0.79 0.86 0.85 0.92 0.84 0.91 0.85 0.89 0.87 0.92 0.84 0.88 0.86 0.90 0.85 0.88 0.64 0.72 0.70 0.82 0.61 0.66 0.70 0.80 0.77 0.86 0.76 0.81 0.85 0.90 0.76 0.81 0.80 0.84 0.79 0.81 0.92 0.94 0.96 0.97 0.93 0.94 0.92 0.95 ... ... 0.83 0.87 0.87 0.93 0.79 0.84 0.84 0.88 ... ... 0.83 0.88 0.86 0.90 0.83 0.87 0.85 0.89 0.87 0.90 0.58 0.61 0.74 0.79 0.55 0.58 0.65 0.72 0.66 0.72 0.92 0.96 0.96 0.98 0.93 0.96 0.93 0.97 ... ... 0.85 0.92 ... ... 0.86 0.91 0.86 0.94 ... ... 0.63 0.69 ... ... 0.68 0.78 0.63 0.69 0.67 0.72 0.88 0.92 0.93 0.95 0.89 0.93 0.87 0.92 0.88 0.92 0.76 0.82 0.83 0.88 0.76 0.83 0.76 0.82 ... ... AZ CA FL IL MI MN NJ NY NC OH OR TX VA 0.84 0.85 0.65 0.77 0.93 0.83 0.84 0.60 0.93 0.86 0.64 0.88 0.76 0.84 0.86 0.64 0.77 0.93 0.83 0.83 0.56 0.93 0.86 0.64 0.88 0.76 0.91 0.90 0.74 0.82 0.95 0.87 0.87 0.59 0.97 0.92 0.70 0.93 0.83 AZ CA FL IL MI MN NJ NY NC OH OR TX VA 0.87 0.90 0.79 0.90 1.20 0.92 0.93 1.00 0.97 1.11 0.76 0.99 0.88 Elasticity of crimes against persons averted by incapacitation to enhanced sanctions 0.93 0.87 0.93 0.89 0.92 0.84 0.90 0.88 0.94 0.87 0.93 0.94 0.90 0.94 0.90 0.95 0.89 0.93 0.91 0.94 0.90 0.93 0.88 0.79 0.87 0.81 0.91 0.76 0.84 0.83 0.91 0.91 0.95 0.93 0.90 0.93 0.93 0.96 0.89 0.92 0.93 0.94 0.91 0.93 1.06 1.21 1.06 1.11 1.03 1.22 1.06 1.17 1.06 ... ... 0.95 0.91 0.94 0.93 0.97 0.90 0.93 0.93 0.95 ... ... 0.94 0.94 0.94 0.93 0.95 0.93 0.94 0.95 0.95 0.97 0.96 0.95 1.00 0.95 0.99 0.97 0.99 0.93 1.02 0.97 1.02 0.96 0.98 0.97 0.98 0.99 0.99 0.97 0.98 0.97 0.99 ... ... 0.99 1.12 0.99 ... ... 1.19 1.01 1.03 0.97 ... ... 0.83 0.76 0.82 ... ... 0.79 0.87 0.76 0.82 0.79 0.85 0.99 0.99 0.99 1.00 0.99 0.99 0.99 0.98 0.98 0.96 0.99 0.93 0.88 0.92 0.89 0.95 0.88 0.93 0.87 0.91 ... ... 0.87 0.90 0.78 0.90 1.20 0.92 0.93 0.99 0.97 1.11 0.76 0.99 0.88 0.93 0.94 0.88 0.93 1.06 0.95 0.94 0.94 0.98 0.99 0.82 0.99 0.93 AZ CA FL IL MI MN NJ NY NC OH OR TX VA 0.83 0.74 0.62 0.63 0.70 0.72 0.69 0.39 0.87 0.72 0.61 0.77 0.59 Elasticity of property related crimes averted by incapacitation to enhanced sanctions 0.90 0.82 0.90 0.90 0.94 0.79 0.85 0.84 0.91 0.83 0.90 0.83 0.79 0.74 0.78 0.79 0.83 0.73 0.78 0.75 0.79 0.74 0.78 0.74 0.71 0.61 0.69 0.67 0.78 0.58 0.63 0.67 0.77 0.73 0.82 0.61 0.68 0.62 0.68 0.75 0.81 0.62 0.67 0.66 0.71 0.65 0.67 0.63 0.73 0.68 0.71 0.85 0.84 0.68 0.72 0.71 0.74 ... ... 0.70 0.77 0.72 0.77 0.79 0.87 0.69 0.75 0.73 0.79 ... ... 0.72 0.74 0.68 0.74 0.73 0.81 0.68 0.74 0.70 0.76 0.72 0.79 0.68 0.35 0.37 0.34 0.56 0.61 0.35 0.31 0.43 0.43 0.45 0.47 0.35 0.92 0.86 0.92 0.91 0.95 0.87 0.92 0.87 0.93 ... ... 0.87 0.81 0.70 0.78 ... ... 0.68 0.77 0.75 0.85 ... ... 0.72 0.67 0.61 0.66 ... ... 0.65 0.75 0.61 0.66 0.63 0.66 0.61 0.83 0.76 0.82 0.85 0.88 0.77 0.83 0.76 0.83 0.77 0.84 0.77 0.64 0.57 0.62 0.71 0.75 0.58 0.64 0.59 0.63 ... ... 0.59 0.90 0.79 0.70 0.68 0.73 0.77 0.74 0.31 0.93 0.81 0.67 0.83 0.64 ... Fewer than 100 obervations. Avi Bhati / Crimes Averted by Incapacitation 28 density function rises gradually from zero to one. Interestingly enough, almost 10 percent of the sample has an estimated elasticity of less than 0.2 with about one percent for whom enhancing sanctions would produce absolutely no benefits. This suggests that these individuals were released at a point in their life when their careers had allready terminated. Since the elasticities are normalized they can be compared across persons, states, and offense types. A quick comparison of the two offense types suggests that in a large portion of the sample, the point of diminishing returns has been reached with respect to crimes against persons. There still exists some unutilized potential reductions in person related crimes that can be accrued if individual are selectively incapacitated. On the other hand, the analysis of property related crimes suggests that further increases in sanction severity will not yield proportional increases in crimes averted by incapacitation. Table 4.3 shows the estimated elasticities by state and various offender demographic characteristics. As was found with the annual crimes averted by incapacitation estimates, there seem to be no major systematic patterns discernable here along demographic attributes. However, some states (e.g., Michigan, Ohio, and Texas) clearly have a relatively higher potential for reducing crimes against persons by pursuing selective incapacitation strategies. Increasing the prison terms of nearly half the releasees in these states by a percent would yield at least a percent increase in the number of crimes against persons averated. These findings are further summarized in the next chapter. Chapter 5 Conclusion 5.1. SUMMARY This researh effort developed an information theoretic approach for modeling the criminal history accumulation process of a sample of prison releasees. Separate models were estimated for two crime categories—crimes against persons and property related crimes. In addition, a model was estimated for all crimes combined. These sets of models were estimated for each of the 13 states included in the analysis. The estimated parameters were then combined with individual offender attributes to compute counterfactual offending micro-trajectories that one could reasonably expect the offender to have been on, had he or she not been incarcerated. These counterfactuals were then integrated over the actual incarceration period to obtain estimates of the annual number of crimes averted by incapacitation. Further, the models were used to simulate the anticipated effects of increasing prison terms for all individuals. The resulting elasticities were assessed across offender subgroups and offense types. Although a fair amount of heterogeneity was found among offenders, there were few discernable differences across offender subgroups defined using demographic characteristics. With the exception of gender, where incarcerating males was found to avert slightly more crime than incarcerating females, the differ- 29 Avi Bhati / Crimes Averted by Incapacitation 30 ences were negligible and inconsistent across race and ethnicity groups. There was a fair amount of variation among the estimated annual crimes averted by incapacitation across various states and across the two offense categories analyzed. Across all 13 states, the average number of crimes against persons averted annually was 1.93 (with a median of 1.41). The average property related crimes averted annually across these states was 8.47 (with a median of 5.75). These numbers are comparable to estimates reported elsewhere using official arrest data. For example, Blumstein and Cohen (1979, 580) report the estimated individual crime rate for aggravated assault to be about 1.7 where as that for burglary, larceny, and auto theft to be between 2.8 to 10. Similarly, Marvel and Moody (1994, 118) summarize that between 16 and 25 index crimes are committed annually by incarcerated prisoners. In the present analysis, the estimated mean number of all crimes averted by incapacitation annually (across the 13 states) was 18.5 (with a median of 13.9). Despite the similarity in these estimates, I hasten to add a cautionary note here since estimates reported in Blumstein and Cohen (1979) and those summarized by Marvel and Moody (1994) were generated from data nearly two decades prior to those used in my analysis. It is unclear whether the less punitive systems of those times should produce similar, larger, or smaller crimes averted estimates than those reported here. What this analysis does suggest is that mean rates reported in earlier studies undoubtedly mask huge variations among individuals. Understanding the source of this variation is crucial to developing efficient policies that do not squander resources. Clearly, since states have different policies and practices, we can expect (and do find in this analysis) a fair amount of variation in the estimates across states. There is still a lot of heterogeneity among individuals that needs to be understood if any strategies are to be devised that will make the best use of limited prison space. The limited set of analyses simulating the elasticities of the incapacitation effect to an increased prison term suggest that the gains to be made by further increases in prison terms are disproportional. The elasticities of the crimes against Avi Bhati / Crimes Averted by Incapacitation 31 persons averted are larger than one for a few individuals, but are largely clustered around 0.9. For property related crimes, however, the point of diminishing marginal returns may have been crossed. Simulations suggest that, for most individuals, a percent increases in prison term will yield a less than one percent increase in the number of crimes averted. Based on these simulation, it is reasonable to conjecture that, at least for property related crimes, reducing the incarceration terms of a large number of inmates may result in little or no reductions in the number of crimes averted by incapacitation. Although the amount of reduction in prison term that may yield little or no reductions in public safety will vary tremendously, as expected, among individuals and will require more detailed analysis, the prospects of reducing incarceration expenses without reducing public safety is very appealing. The analytical framework developed here can provide helpful guidance on early release decisions, if such decisions are contemplated by policymakers when faced, for example, with prison overcrowding. 5.2. FUTURE RESEARCH The use of trajectory-based methods for studying offending over the life-course is not new. Nagin (2005) succintly summarizes what has been learned about this phenomenon to date by applying group-based semi-paramteric methods—first applied to this problem by Nagin and Land (1993). Although the purpose of developing offending trajectories for the present analysis was to project counterfactuals, an interesting avenue for future research would be to compare the substantive predictions made by the present model with those summarized in Nagin (2005). As was noted in the introductory chapter of this report, all inferences derived and discussed here pertain only to the population of releasees. However, incarceration policy may also be altered by increasing or decreasing the incarceration rate without altering the length of the imposed term. In order to empirically assess that set of policy options, it would be necessary to develop weights that Avi Bhati / Crimes Averted by Incapacitation 32 would allow the micro-simulations, developed in this report, to be re-weighted to reflect the unequal probabilities of selection into the current sample of releasees. If such selection weights could be developed satisfactorily, then it may be possible to use the empirical distributions discussed here to provide guidance for those policies choices. As suggested by a reviewer, it would be interesting to link the state variations uncovered in this study to state policy choices (e.g., the punitiveness of the justice system) since states vary considerably with respect to their penal policies and procedures. Moreover, given their policies, states could allready be selectively incapacitating high rate offenders at differential rates. A fruitful avenus for future research, thus, would be to attempt to empirically test competing hypotheses explaining these variations. Although the modeling exercise conducted here simulated counterfactual trajectories only for the period an individual was incapacitated, nothing precludes us from utilizing this model to simulate a post-release counterfactual. Since the data contain detailed information on the offending patterns of this sample of releasees for a period of three years after release, a comparison of the actual offending rate with the simulated counterfactual can be used to study whether, and to what extent, the incarceration has altered the offending patterns of each individual. This approach has been applied elsewhere (Bhati 2006; Bhati and Piquero mimeo) and preliminary results indicate that about 40 percent of the release cohort can be characterized as having been deterred from future offending (specific deterrence), about 56 percent returned back to offending patterns that were anticipated by their counterfactuals (were merely incapacitated), and about four percent actually experienced criminogenic effects. Similar analysis may be used to compute the number of future crimes averted or caused by incarceration, and what, if any, policy levers can be used to change those outcomes. Finally, since the data are at the individual level and since the analysis builds on developing the criminal history accumulation process, one can utilize time Avi Bhati / Crimes Averted by Incapacitation 33 varying macro covariates to incorporate general deterrence and replacement effects into the model. For example, to the extent that historic sentencing policies are found to affect the micro-trajectories estimated in this analysis, we will have uncovered general deterrence effects. Similarly, to the extent that historical incarceration rates or changes thereof are found to affect the micro-trajectories, we will have uncovered replacement effects. These extensions are possible, at least in theory. They have yet to be implemented and are promising avenues for future work. References Allison, P. D. (1984). Event History Analysis Beverly Hills, CA: Sage Publications. Allison, P. D. (1995). Survival Analysis Using SAS: A Practical Guide Cary, NC: SAS Institute Inc. Avi-Itzhak, B., and Shinnar, R. (1973). “Quantitative Models of Crime Control.” Journal of Criminal Justice 1:185–217. Bhati, A. S. (2006). Studying the Effects of Incarceration on Offending Trajectories: An Information-Theoretic Approach. Washington, DC: The Urban Institute. Bhati, A. S. (2007). “Estimating the Number of Crimes Averted by Incapacitation: An Information Theoretic Approach.” Journal of Quantitative Criminology. Forthcoming. Bhati, A. S., and Piquero, A. (mimeo). “On the Effects of Incarceration on Subsequent Individual Criminal Offending: Deterrent, Criminogenic, or Null Effects?” Blossfeld, F., Hamerele, A., and Mayer, K. U. (1989). Event History Analysis: Statistical Theory and Applications in the Social Sciences Hillsdale, NJ: Lawrence Erlbaum Association Publishers. Blumstein, A., and Cohen, J. (1973). “A Theory of the Stability of Punishment.” The Journal of Criminal Law and Criminology 64(2):198–207. 34 Avi Bhati / Crimes Averted by Incapacitation 35 Blumstein, A., and Cohen, J. (1979). “Estimating the Individual Crime Rates from Arrest Records.” The Journal of Criminal Law and Criminology 70(4):561–585. Blumstein, A., Cohen, J., Roth, J. A., and Visher, C. A. (1986). “Methodological Issues in Criminal Career Research.” In Blumstein, A., Cohen, J., Roth, J. A. and Visher, C. A. (eds.) Criminal Careers and “Career Criminals” Vol I. Washington, DC: National Academy Press. Blumstein, A., and Piquero, A. (2007). “Does Incapacitation Reduce Crime?” Journal of Quantitative Criminology. Forthcoming. Brame, R., Bushway, S., and Paternoster, R. (2003). “Examining the Prevalence of Criminal Desistance.” Criminology 41:423-448. Bureau of Justice Statistics (2002). Recidivism of Prisoners Released in 1994, Codebook for Dataset 3355. Downloaded from NACJD in August 2003. Bushway, S., Brame, R., and Paternoster, R. (2004). “Connecting Desistance and Recidivism: Measuring Changes in Criminality Over the Lifespan.” In Murana, S., and Immarigeon, R. (eds.) After Crime and Punishment: Pathways to Offender Reintegration. Portland, OR: Willian Publishing. Chaiken, J. M., and Chaiken, M. R. (1982). Variations in Criminal Behavior. Santa Monica, CA: Rand. Cohen, J. (1978). “The Incapacitation Effects of Imprisonment: A Critical Review of the Literure.” In Blumstein, A., Cohen, J., and Nagin, D. (eds.) Deterrence and Incapacitation: Estimating the Effects of Criminal Sanctions on Crime Rates. Washington, DC: National Academy of Sciences. Cohen, J. (1983). “Incapacitation as a Strategy for Crime Control: Possibilities and Pitfalls.” In Tonry, M., and Morris, N. (eds.) Crime and Justice: An Annual Review of Research, Volume 5. Chicago, IL: Chicago University Press. Cohen, J. (1986). “Research on Criminal Careers: Individual Frequency Rates Avi Bhati / Crimes Averted by Incapacitation 36 and Offense Seriousness.” In Blumstein, A., Cohen, J., Roth, J. A. and Visher, C. A. (eds.) Criminal Careers and “Career Criminals”, Vol I. Washington, DC: National Academy Press. Cressie, N., and Read, T. R. C. (1984). “Multimodel Goodness-of-Fit Tests.” Journal of the Royal Statistical Society, Ser. B 46(3):440-464. DiIulio, J. J. (1990). Crime and Punishment in Wisconsin. Wisconsin Policy Research Institute Report, Vol 3, no. 7. Milawukee, WI: Wisconsin Policy Research Institute. Ezell, M. E., Land, K. G., and Cohen, L. E. (2002). “Modeling Multiple Failure Time Data: A Survey of Variance-Corrected Proportional Hazard Models with Empirical Applications to Arrest Data.” Sociological Methodology 33:111-167. Farrington, D. (1986). “Age and Crime.” In Morris, N. and Tonry, M. (eds.) Crime and Justice. Chicago, IL: University of Chicago Press. Fomby, T. B. and Hill, R. C. (eds.) (1997). Advances in Econometrics: Applying Maximum Entropy to Econometric Problems Volume 12. Golan, A. (2002). “Information and Entropy Econometrics—Editor’s View.” Journal of Econometrics 107(1-2):1-357. Golan, A., Judge, G. G., and Miller, D. (1996). Maximum Entropy Econometrics: Robust Estimation with Limited Data. Chichester, England: John Wiley and Sons. Good, I. J. (1963). “Maximum Entropy for Hypothesis Formulation, Especially for Multidimensional Contingency Tables.” Annals of Mathematical Statistics 34:911-934. Greenburg, D. F. (1975). “The Incapacitation Effect of Imprisonment: Some Estimates.” Law and Society Review 9(4):541–580. Hart, T. C., and Rennison, C. (2003). Reporting Crime to the Police, 1992– 2000. Special Report. Washington, DC: Bureau of Justice Statistics (NCJ Avi Bhati / Crimes Averted by Incapacitation 37 195710). Harding, R. W., and Maller, R. A. (1997). “An Improved Methodology for Analyzing Age-Arrest Profiles: Application to a Estern Australian Offender Population.” Journal of Quantitative Criminology 13(4):349-372. Horney, J., and Marshall, I. (1991). “Measuring Lambda Through Self-Reports.” Criminology 29:401–425. Huber, P. J. (1967). “The Behavior of Maximum Likelihood Estimators under Non-Standard Conditions” Proceeding of the Fifth Symposium on Mathematical Statistics and Probability 1:221–233. Jaynes, E. T. (1957a). “Information Theory and Statistical Mechanics.” Physics Review 106:620–630. Jaynes, E. T. (1957b). “Information Theory and Statistical Mechanics II.” Physics Review 108:171–190. Jaynes, E. T. (1982). “On The Rationale of Maximum Entropy Methods.” Proceedings of the IEEE 70(9):939-952. Journal of Econometrics: Special Issues on Information and Entropy Econometrics (2002). ed. Amos Golan. 107(1-2): 1–357. Kullback, J. (1959). Information Theory and Statistics. New York, NY: John Wiley. Langan, P. A., and Levin, D. J. (2002). Recidivism of Prisoners Released in 1994. Special Report. Washington, DC: Bureau of Justice Statistics. Levine, R. D. (1980). “An Information Theoretic Approach to Inversion Problems.” Journal of Physics A 13:91-108. Lillard, L. A. (1993). “Simultaneous Equations for Hazards: Marriage Duration and Firtility Timing.” Journal of Econometrics 56:189-217. Maasoumi, E. (1993). “A Compendium of Information Theory in Economics and Econometrics.” Econometric Reviews 12(2):137–181. Avi Bhati / Crimes Averted by Incapacitation 38 Maltz, M. D. (1984). Recidivism. Orlando, FL: Academic Press. Manski, C. (1988). Analog Estimation Methods in Econometrics. London: Chapman and Hall. Marvel, T. B., and Moody, C. E. (1994). “Prison Population Growth and Crime Reduction.” Journal Of Quantitative Criminology 10(2):109–140. Mayer, K. U., and Tuma, N. B. (eds.) (1990). Event History Analysis in Life Course Research Madison, EI: The University of Wisconsin Press. Miles, T., and Ludwig, J. (2007). “Silence of the Lambdas: Deterring Incapacitation Research.” Journal of Quantitative Criminology. Forthcoming. Mittelhammer, R. C., Judge, G. G., and Miller, D. J. (2000). Econometric Foundations. Cambridge, UK: Cambridge University Press. Nagin, D. S. (2005). Group Based Models of Development. Boston, MA: Harvard University Press. Nagin, D. S., and Land, K. G. (1993). “Age, Criminal Careers, and Population Heterogeneity: Specification and Estimation of a Non-Parametric, Mixed Poisson Model,” Criminology 31:327–362. Nagin, D. S., and Paternoster, R. (2000). “Population Heterogeneity and State Dependence: State of the Evidence and Directions for Future Research.” Journal of Quantitative Criminology 16:117–144. Peterselia, J., Greenwood, P. W., and Lavin, M. (1978). Criminal Careers of Habitual Felons. Santa Monica, CA: Rand. Pastore, A. L., and Maguire, K. (eds.) (2005). Sourcebook of Criminal Justice Statistics 2003. Washington, DC: Bureau of Justice Statistics, U. S. Department of Justice. Reiss, A. J. (1988). “Co-offending and Criminal Careers.” In Tonry, M., and Morris, N. (eds.) Crime and Justice: An Annual Review of Research Volume 10. Chicago, IL: Chicago University Press. Avi Bhati / Crimes Averted by Incapacitation 39 Ryu, H. K. (1993). “Maximum Entropy Estimation of Density and Regression Functions.” Journal of Econometrics 56:397-440. Sampson, R. J., and Laub, J. H. (2005). “A Life-course View of the Development of Crime.” The Annals of the American Academy of Political and Social Science 602:6–45. Shannon, C. E. (1948). “A Mathematical Theory of Communication.” Bell System Technical Journal 27:379-423. Shinnar, S., and Shinnar, R. (1975). “The Effects of the Criminal Justice System on the Control of Crime: A Quantitative Approach.” Law and Society Review 9:581–611. Soofi, E. S. (1994). “Capturing the Intangible Concept of Information.” Journal of the American Statistical Association 89(428):1243–1254. Spelman, W. (1994). Criminal Incapacitation. New York: Plenum. Spelman, W. (2000). “What Recent Studies Do (and Don’t) Tell Us about Imprisonment and Crime.” Crime and Justice 27:419-494. Visher, C. A. (1987). “Incapacitation and Crime Control: Does a “Lock ’Em Up” Strategy Reduce Crime?” Justice Quarterly 4(4):513–543. White, H. (1980). “A Heteroskedasticity-Consistent Covariance Matrix Estimator and a Direct Test for Heteroskedasticity.” Econometrica 48:817–830. Yamaguchi, K. (1991). Event History Analysis. Newbury Park, CA: Sage Publishing, Inc. Zellner, A. (1991). “Bayesian Methods and Entropy in Economics and Econometrics.” In Grady, W. T. and Schick, L. H. (eds.) Maximum Entropy and Bayesian Methods. Netherlands: Kulwer. Zellner, A., and Highfield, R. A. (1988). “Calculation of Maximum Entropy Distributions and Approximation of Marginal Posterior Distributions.” Journal of Econometrics 37:195-209. Avi Bhati / Crimes Averted by Incapacitation 40 Zimring, F. and Hawkins, G. (1995). Incapacitation: Penal Confinement and the Restraint of Crime. New York, NY: Oxford University Press. Appendix A Mathematical Appendix In this appendix, I provide detailed derivation of the information theoretic approach to modeling the criminal history accumulation process. A.1. NONPARAMETRIC ESTIMATES Consider, as a point of departure, that we have access to detailed information on dated arrest histories for a group of individuals recently released from prison. Detailed information pertaining to each arrest need to include, at a minimum, the date of the arrest and its order in the sequence (i.e., arrest number 1, 2, 3, etc.). Detailed information pertaining to the individuals need to include, at a minimum, the date of birth of the individual, the data of prison release and the date of prison admission. This minimal amount of information is needed in order to construct sequences of ages at each successive arrest events. Harding and Maller (1997) refer to this sequencing as individuals’ arrest profiles. Assume that such profiles exist for the period before incarceration. In what follows, let a r n denote the age of the nth individual when he or she was arrested for the r th time. The subscript n = 1, . . . , N is used to index individuals and r = 1, . . . , Rn is used to index arrest events. Next, let us artificially discretize the continuous “age at arrest” variable. 41 42 Avi Bhati / Crimes Averted by Incapacitation That is, for M mutually exclusive and exhaustive artificially defined intervals (say monthly, quarterly, etc.), let us define the following dummy variables y r mn = 1 if a r n ∈ (z m−1 , z m ) 0 otherwise ∀n ∈ N ; r ∈ Rn ; m ∈ M . (A.1) where z m is a grid of equidistant points descretizing the support of the outcome (a r n ). In effect, we are creating a set of M binary dummy variables for each arrest event for each individual at each age. Consider, next a positive quantity, denoted λ r mn , that we believe this set of dummy variables represent. We can think of the actual outcomes as a noisy (imperfect) manifestation of some underlying stochastic process that we wish to recover. Given the assumption of imperfection, we can only link these unknown quantities (λ r mn ) to their observed counterparts (y r mn ) as approximations. Therefore, let y r mn ≈ λ r mn ∀r, m, n. (A.2) Next, to build in the order of the events we need to define a corresponding set of dummy variables that flag whether or not a particular arrest was possible at a particular age. To do so, let d r mn = 1 if a(r −1)n < z m ≤ a r n 0 otherwise ∀n ∈ N ; r ∈ Rn ; m ∈ M . (A.3) Here, unlike (A.1), we are creating a set of dummy variables flagging the possibility of each arrest event for each individual at each age. An example of what these two sets of dummy variables would look like for an arrest profile is given in table A.1. The hypothetical individual was arrested for the first time at age 17, for the second time at age 23, and the third time at age 37 after which this individual 43 Avi Bhati / Crimes Averted by Incapacitation Table A.1: An example of creating the y r mn and d r mn flags from arrest profiles. z1 0 z2 5 z3 10 z4 15 z5 z6 z7 z8 z9 20 25 30 35 40 r ar n y1 y2 y3 y4 y5 y6 y7 y8 y9 1 2 3 17 23 37 0 0 0 0 0 0 0 0 0 0 0 0 1 0 0 0 1 0 0 0 0 0 0 0 0 0 1 r ar n d1 d2 d3 d4 d5 d6 d7 d8 d9 1 2 3 17 23 37 1 0 0 1 0 0 1 0 0 1 0 0 1 1 0 0 1 1 0 0 1 0 0 1 0 0 1 entered prison and was released as part of the release cohort under study. As is shown in table A.1, the y r mn flags identify when an arrest occurs and the d r mn flags identify when an individual is at risk of a particular arrest. Having defined the two interrelated sets of dummy variables, let us combine them. To do so, let us (i) pre-multiply both sides of (A.2) by the d r mn flags, (ii) sum across all individuals with the same r and m, and (iii) assume that this aggregation washes out all the imperfections. This allows us to convert the inequalities into the following equalities: Finally, if we assume that λ r mn = λ r m ∀r, m, i.e., that this quantity is fixed within each r and m pairs, then we can solve explicitly for each of these unknown quantities to get λr m = n d r mn y r mn n d r mn ∀r, m. (A.4) Since an event occurs (i.e., y r mn = 1) only when an individual is at risk of that event occurring (i.e., d r mn = 1), we see that the numerator of this ratio is merely the number of individuals being arrested for the r th time within the mth age interval. The denominator, on the other hand, is merely the number of persons 44 Avi Bhati / Crimes Averted by Incapacitation that were at risk of being arrested for the r th time during the mth age interval. This quantity is, of course, a familiar one—it represents the hazard rate. The derivation in (A.4) is in fact a nonparametric estimate of the hazard of the r th arrest occurring during age interval m. d r mn y r mn = n d r mn λ r mn ∀r, m. (A.5) n The point of this derivation was simply to demonstrate that, when combined with the set of dummy variables d r mn , any manipulation of the left and right hand sides of (A.2) will yield constraints on the values the hazard can take. In the example provided in table A.1, I used 5-year intervals. In fact, one can use as small an interval as one desires. For example, when studying age profiles as measured in year, one can define intervals as small as a quarter or a month. However, nonparametric computation of the hazard rate becomes more unstable because we end up with many cells with small counts in the denominator. This suggests moving towards a semiparametric formulation of the problem which allows a flexible functional form linking the hazards across persons, ages, and event numbers. I turn to that formulation next. A.2. A SEMIPARAMETRIC REFORMULATION Instead of making the assumptions needed to go from (A.2) to (A.4), as was done for the nonparametric derivation, we can develop moment conditions that we expect to see in the data. Formal theoretical reasoning or casual past experience may suggest various transformations of the left and right hand sides of (A.2) that we anticipate. If so, we can use this knowledge to convert the inequalities of (A.2) into a set of moment constraints that will replace the equalities (A.4). To be concrete, suppose we believe that λ increases (or decreases) with each subsequent arrest. This suggests, at a minimum, that λ r mn should covary with rn . But by how much? Using the analogy principle, one may assume, under a host of regularity conditions, that the best estimate of this covariance is found 45 Avi Bhati / Crimes Averted by Incapacitation in the sample itself (Manski 1988). In other words, the expected covariation between rn and λ r mn should mimic the observed covariation between rn and y r mn . This suggests the following equality constraint: rn d r mn y r mn = r mn rn d r mn λ r mn (A.6) r mn In general though, we can think of other factors that ought to affect the hazards. This could include individual attributes (e.g., fixed for an individual over the life), attributes that change within an individual over time, as well as various transformations of the age grid (z m ) itself. The last of these—the transformation of the age grid—is crucial in the models used in the latter part of this report so I provide some more explanation here. Suppose we pre-multiply d r mn y r mn by z m and sum across m. This will yield a crude approximation of the original age variable. If we do the same on the right hand side of (A.2) we obtain the quantity m z m d r mn λ r mn . In other words, if we use the age grid as an attribute, we can create equality constraints that equate moments involving the hazards to moments involving the observed age at arrest (i.e., a r n ). Similarly, we can transform the age grid in particular ways to obtains other constraints involving higher moments of age. For example, if we multiply both sides of (A.2) by d r mn z m log z m , then we obtain constraints involving the hazard and a r n log a r n . Another transformation that is particularly relevant is the spell length. Suppose we multiply both sides with d r mn (z m − a(r −1)n ). Then we obtain equality constraints involving the arrest hazards with moments involving the spell length (i.e., a r n −a(r −1)n ). As should be clear by now, the approach places no restriction on the number and kinds of constraints that can be imposed. The approach is fairly flexible and allows one to incorporate as many constraints as are either suggested by theory and/or past experience. Moreover, each of these transformations may be affected by the included attributes (e.g., fixed or time varying covariates, etc.). 46 Avi Bhati / Crimes Averted by Incapacitation In order to ease exposition, therefore, I introduce an abstract representation of the constraints. Let φ j (z m ) represent the j th transformation of the age grid and let xk r n represent a set of attributes that can include fixed and time varying covariates. We may generically write the set of constraints as: xk r n φ j (a r n ) = rn xk r n rn d r mn φ j (z m )λ r mn ∀k ∈ K; j ∈ J (A.7) m where the j transformation φ j include multiple moments as well as multiple clocks (Lillard 1993). Multiple clock models allow researchers to capture “additional dimensions of time” that may be relevant to the process when studying repeatable events (Yamaguchi 1991, 53). We now have, what is termed, an ill-posed inversion problem—more unknowns than equations linking them (Levine 1980). As such, an infinite number of solutions for λ r mn can satisfy the constraints given in (A.7). How do we solve this ill-posed problem? A.3. THE INFORMATION-THEORETIC SOLUTION Edwin Jaynes (1957a,b), in a series of influential papers in statistical mechanics proposed a solution to such a problem provided that the unknown quantities are in the form of proper probabilities. He proposed that when faced with a problem that has possibly an infinite number of solutions, we should choose the one solution that implies maximum uncertainty while ensuring that the constraints (evidence) are satisfied. That way, we will be making the most conservative (safe) use of the evidence. Jaynes (1982) provides an axiomatic derivation of the rationale underlying this approach. Of course, for the approach to be operationalized, Jaynes needed some quantification of uncertainty. Within the context of a problem in communication theory, Shannon (1948) had, a few years earlier, defined the uncertainty contained in a message with J mutually exclusive and exhaustive outcomes as H = − j p j log p j and termed it Information Entropy. Here p j is the probability Avi Bhati / Crimes Averted by Incapacitation 47 that event j will be observed from the set of J possible events. In what came to be known as the Maximum Entropy formalism, Edwin Jaynes proposed to use Shannon’s Entropy as the criterion to maximize, subject to all available constraints, in order to derive conservative inferences from the evidence. In addition, if there exists some non-sample prior information about the probabilities { p 0j }, then an equivalent problem is to minimize the KullbackLeibler directed divergence, or Cross Entropy, between the prior and the posterior probabilities (Kullback 1959; Good 1963). The Cross Entropy is defined as C E = j p j log( p j / p 0j ) if p 0j are the priors. If the prior probabilities p 0j are assumed to be uniform, then the Cross Entropy formalism reduces to the Maximum Entropy formalism. Not surprisingly, both the C E and the H objectives are related and really special cases of the family of Cressie Read power divergence measures (Cressie and Read 1984). Notwithstanding the diverse types of constraints that theory may suggest (e.g., geometric moment, higher order moment, inequality constraints, etc.) and whether or not we believe their sample analogs are measured with noise, this method of using information in a sample (evidence) to recover information about social, economic, or behavioral phenomenon falls within the growing field of Information and Entropy Econometrics.1 The key requirement of this formulation is that the unknowns be proper probabilities (i.e., non-negative quantities that sum to one). This is because Shannon’s entropy, as well as the Kullback-Leibler directed divergence measures, are defined in terms of proper probabilities. Zellner (1991) and Zellner and Highfield (1988) have developed this approach extensively in the econometrics field to derive a general class of distributions that satisfy various side conditions that may be suggested/provided by economic theory. 1 For recent theoretic and applied work in this field, see the 2002 special issue of the Journal of Econometrics (Vol 107, Issues 1&2), Chapter 13 of Mittelhammer, Judge and Miller (2000), the 1997 Volume (12) of Advances in Econometrics titled “Applying Maximum Entropy to Econometric Problems,” and the Golan, Judge, and Miller (1996) monograph. See also Maasoumi (1993), Soofi (1994), and Golan (2002) for historical discussions and general surveys. Avi Bhati / Crimes Averted by Incapacitation 48 In an important extension of their work, Ryu (1993) used this same principle to derive regression functions rather than probability distributions. Ryu (1993) showed that if the unknown quantities can be assumed to be non-negative, then the application of the Maximum Entropy (or Minimum Cross Entropy) principle can, under suitable side conditions, yield a large number of functional forms. Using the example of a production function with 2 inputs (Capital and Labor), Ryu (1993) derived the Exponential polynomial, the Cobb-Douglas, the Translog, the Generalized Cobb-Douglas, the Generalized Leontiff, the Fourier flexible form, and the Minflex-Laurent Translog production functions simply by manipulating the side conditions. This brings us back to the problem at hand. The evidence we have is in the form of the constraints (A.7) and our unknowns (λ r mn ) are in the form of non-negative hazards—precisely the kind of problem for which the Maximum or Cross Entropy formalism could be applied very profitably. However, unlike Ryu (1993), where each of the unknowns are completely unrestricted (other than being non-negative), in our case, some of the hazards are not possible. Hence, following Ryu (1993), we can define a generic Cross Entropy problem but, additionally, introduce the d r mn flags into the objective function. This ensures that hazards corresponding to periods when individuals are not at risk of a progression will in no way influence the objective being optimized. This modified information recovery problem can be written as: min {λ r mn } CE = r mn d r mn λ r mn log(λ r mn /λ0r mn ) (A.8) subject to the constraints of (A.7). Here λ0r mn is an arbitrary non-negative quantity representing our prior state of knowledge. This constrained optimization problem can be solved using the method of Lagrange and an optimum solution can be derived. The solution for the generic set of constraints (A.7) turn out to 49 Avi Bhati / Crimes Averted by Incapacitation be of the form: λ r mn = λ0r mn exp φ j (z m ) j xk r n θk j ∀k ∈ K; j ∈ J (A.9) k where θk j are a set of Lagrange multipliers from the optimization problem corresponding to constraints (A.7). In general, there is no analytical solution to this optimization problem and numeric solutions have to be obtained. Despite the complex nature of the optimization problem, it can be simplified considerably by inserting the solutions for λ r mn into the primal problem and deriving a dual objective function. The dual is an unconstrained optimization problem in the unknown θ and, as such, can be estimated in a variety of software. Once the values of the θk j are obtained they can be plugged into the solution and the hazard path can be traced for different values of φ j (z m ). In other words, armed with the set of attributes xk r n and θk j , analysts can use (A.9) to trace the expected trajectory of the hazard for any individual at any age. Computing the number of arrests averted by incapacitating an individual for some time is now simply a matter of integrating the hazard over that life period. In fact, once the parameters are estimated, we may now revert to a continuous time notation—i.e., assuming that we have an infinitely fine grained support space z1 , . . . , zM . The number of arrests averted by incapacitating an individuals between the ages of z n and z n can be computed as ˆsn = zn zn λn (z) d z = zn zn λ0n exp φ j (z) j xkn θk j dz ∀n ∈ N . k (A.10) The computation provided in previous section yield estimates of the number of arrest averted by incapacitation. Our main interest, however, is in estimating the number of crimes averted by incapacitation. Therefore, we need some way to account for the fact that each arrest represents several offenses. In order to do so, in the current framework, one needs to define a correction factor, preferably 50 Avi Bhati / Crimes Averted by Incapacitation at the person-event level. Let c l r mn be such a factor. In the analysis conducted in this study, data on number of charges of crime category l (h l r n ), the crime clearance rate for various years (b l r n ), the crime reporting rate by age of offender and crime category l (e l n ), as well as aggregate co-offending rates (o l ) were used to compute the corrections factor as follows: cl r n = hl r n bl r n × el n × ol ∀l , r, m, n. (A.11) These correction factors multiply each of the y r mn flags defined in Table 4. In other words, each arrest y r mn = 1 represents c l r n crimes of type l . The remaining derivations remains identical. The final set of constraints that emerge from this modification take the form: xk r n c l r n φ j (a r n ) = rn xk r n rn d r mn φ j (z m )λ l r mn ∀k ∈ K; j ∈ J ; l ∈ L, m (A.12) so that the constraints are upward adjusted and the parameters reflect this adjustment. As such, simulations do not need to be further adjusted. The λ estimates produced by imposing the adjusted constraints already are measured in the correct metric—the hazard rate of offense type l .