University of Kentucky, the Impact of Incarceration on Food Insecurity Among Households With Children, 2012
Download original document:
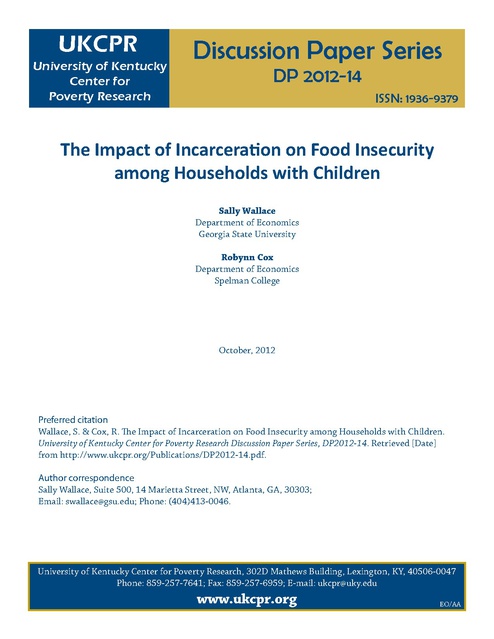
Document text
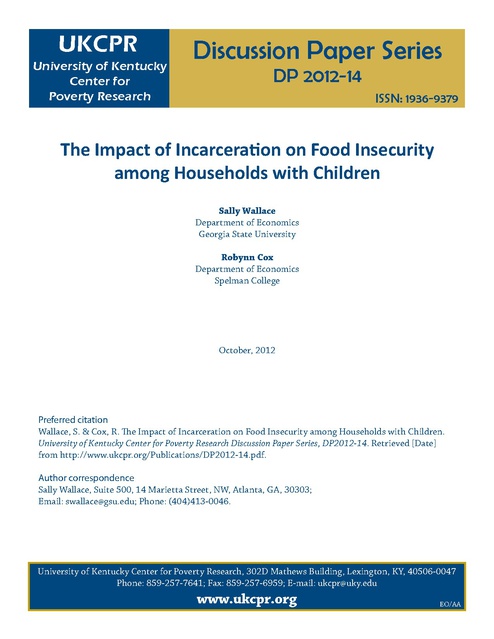
Document text
This text is machine-read, and may contain errors. Check the original document to verify accuracy.
UKCPR University of Kentucky Center for Poverty Research Discussion Paper Series DP 2012-14 ISSN: 1936-9379 The Impact of Incarceration on Food Insecurity among Households with Children Sally Wallace Department of Economics Georgia State University Robynn Cox Department of Economics Spelman College October, 2012 Preferred citation Wallace, S. & Cox, R. The Impact of Incarceration on Food Insecurity among Households with Children. University of Kentucky Center for Poverty Research Discussion Paper Series, DP2012-14. Retrieved [Date] from http://www.ukcpr.org/Publications/DP2012-14.pdf. Author correspondence Sally Wallace, Suite 500, 14 Marietta Street, NW, Atlanta, GA, 30303; Email: swallace@gsu.edu; Phone: (404)413-0046. University of Kentucky Center for Poverty Research, 302D Mathews Building, Lexington, KY, 40506-0047 Phone: 859-257-7641; Fax: 859-257-6959; E-mail: ukcpr@uky.edu www.ukcpr.org EO/AA The Impact of Incarceration on Food Insecurity among Households with Children Robynn Cox Department of Economics Spelman College rcox@spelman.edu and Sally Wallace Department of Economics Georgia State University swallace@gsu.edu 1 ACKNOWLEDGEMENT This project was supported with a grant from the University of Kentucky Center for Poverty Research through funding by the U.S. Department of Agriculture, Food and Nutrition Service, contract number AG-3198-B-10-0028. The opinions and conclusions expressed herein are solely those of the author(s) and should not be construed as representing opinions or policies of the UKCPR or any agency of the Federal Government. The authors wish to acknowledge the helpful comments and suggestions made by Jim Ziliak, Craig Gundersen, and participants of the Research Program on Childhood Hunger workshops in Lexington, KT and Champaign, IL and the excellent research assistance of Luciana Lopes. Finally, we are extremely grateful for the help provided by Mark Nord regarding the issue of missing values in the case of food security variables. 2 ABSTRACT This study seeks to determine the role that parental incarceration plays on the probability of food insecurity among families with children and very low food security of children using micro-level data from the Fragile Families and Child Well Being Study (FFCWS). The data set contains the 18-question food security module which allows us to explore the link between incarceration and food insecurity and very low food security among children, families, and adults. The incidence of very low food security in our data is somewhat higher than the national average, but the incidence of other levels of food security is similar to national aggregates. Since there is likely reverse causality in the relationship between parental incarceration and food insecurity, we employ a variety of program evaluation techniques to identify the causal relationship between food insecurity and parental incarceration. We employ imputation techniques to account for non-response among the food security variables and independent variables. Our ordinary least squares results suggest that having at least one parent that has ever been incarcerated has a small positive effect (1 to 4 percentage points) on the probability of very low food security among children, adults and households with children, but the results are not significant in various specification. Food insecurity for adults and households with children (a less dire level of food insecurity than very low food security) is affected by parental incarceration under most specifications with magnitudes of impact from 4 to 15 percentage points. This research provides some evidence that incarceration adversely affects children and families in terms of food insecurity. Policies to mitigate the impact could be addressed through the court system whereby children are provided with court-sanctioned support to address food needs. 3 EXECUTIVE SUMMARY An important step to reaching the goal of eliminating childhood hunger is a better understanding of the scope of the problem. Our research adds to that understanding by identifying the extent and determinants of very low food security and food insecurity among children and households that include incarcerated parents. This is an important group to understand because of the potential increase in their numbers associated in part with the increased incarceration rates in the U.S., and the potential to reach these children since one or both of their parents is “in the system.” Incarceration may impact food security of children by removing the incarcerated parent as a source of income for the child. Incarceration may also act as a shock to the household by placing an additional financial strain on the family (Grinstead et al., 2001) and limit upward mobility of families. In this paper, we use data from the Fragile Families and Child Well Being Study (FFCWS) to analyze the role parental incarceration plays on food insecurity and very low food security of children and households to attain the following goals: 1. Determine the magnitude and characteristics of this population. 2. Determine the role that parental incarceration plays in the likelihood of experiencing food insecurity and very low food security among children, adults, and households. 3. Determine whether incarceration exacerbates the likelihood of childhood food insecurity. 4. Determine how incarceration impacts the participation of nutrition assistance and non-food assistance programs. The policy implications of this research are two-fold. First, by identifying the magnitude of this at risk population, appropriate attention and resources may be offered to children of parents at risk of incarceration. Secondly, we can examine whether there is evidence of collateral damage associated with incarceration in terms of propensity of families to access public programs (focusing on TANF). The empirical analysis is described in detail in this report. We use a variety of techniques to identify the causal relationship between incarceration and food security. There is arguably an endogeneity to the relationship—parents facing food hardship may turn to crime, which may result in incarceration. Incarceration itself may limit family income and increase food insecurity. To help identify causal effects, we employ several empirical techniques to control for the endogeneity. We focus on two measures of the dependent variable: “very low food security” (VLFS) among children, adults, and households and also “food insecurity” (FS) among children, adults, and households. The Fragile Families and Child Wellbeing Study (FFCWS) is a longitudinal study that is currently following a set of 4,898 families that gave birth to a child in 20 large (population of 200,000 or larger) U.S. cities between the years 1998 and 2000. Each wave includes a “core” survey, which is a hospital based survey at birth, and a telephone survey of the mother and father at ages 1, 3, 5, and 9. At ages 3, 5, and 9 there is also an in-home assessment and various supplemental surveys. The survey collects information on parental history and behavior, parent 4 and child health, economic conditions, public program participation, demographic information, general health, and the environmental conditions in which the child is raised. Most importantly, FFCWS also contains food security questions in line with the Core Food Security Module (CFSM) in the third and fifth year in-home surveys, and detailed information on parental incarceration. Another major benefit of using FFCWS is that it surveys both the mother and the father, and the survey asks questions to the mother and the father about the other parent. We restrict our data to only those years that contain the Core Food Security Module: years 3 and 5. We employ a number of estimation strategies to account for endogeneity. We report results from many of the models so that we can assess the benefits of more complicated estimation strategies, some of which may entail a loss of power in estimation. The completeness of our data suffer from missing variables for incarceration, food security, and a number of covariates. Regarding the food security variables, we were able to recover responses for the vast majority of the food security questions. In the cases of missing values for other variables, we used multiple imputation by chained equations procedure to impute key variables. The imputations for the covariates do not have a markedly different effect on the results. In our data, the number of observations for very low food security for children is small, (50 observations), and this affects the precision of our results. We find that incarceration is almost universally positively correlated with measures of food insecurity (influencing the probability of very low food security among children by up to 4 percentage points), but our results are not significant at standard confidence levels. Incarceration of a parent is positively correlated with the “food insecurity” measure for adults and households with children. The magnitudes of significant impacts for these populations range from 4 to 15 percentage points. We have not identified the specific path of the incarcerationfood security interaction, but it is important to note that, based on our analysis, incarceration may marginally add to the propensity of families to be food insecure. One path of the incarceration-food security relationship could be via the loss of public benefits at the time of parental incarceration—what the literature calls a collateral consequence or invisible punishment. We estimate the number of TANF eligible families and their reported usage of TANF (the “take-up” rate), and cross-tabulate this with parental incarceration. We expected to find that take-up is lower among families with incarcerations, but similar to Butcher and Lalonde (2006) we find no evidence of this impact. Based on our estimates, the take-up rate is low among all families in the data set, which alludes to another policy issue related to the education of the population eligible for government services. As a result of this analysis, we believe that there are a number of issues that need additional research. In our base data, weighted using national city weights, we find that the prevalence of very low food security among children is larger than what is reported nationally for one wave of the data but not for the second wave and the overall level of food insecurity is smaller than what might be expected. As noted below, these results may be artifacts of the small sample size of the FFCWS, but given this “evidence” more work should be done to understand the composition and dynamics of food insecurity among children within a household in urban versus rural areas. At the national level, rural areas report higher food insecurity (but we can find no statistics on the relative size of very low food security among children in rural versus urban areas). It may be 5 that in rural areas, children are at less risk of very low food security because of the working behavior of parents (one parent at home). In urban areas density would suggest more opportunity for food support programs but the low take-up of programs like TANF suggest that availability does not equal usage. There is also a need for larger samples of families like those studied in the FFCWS. The small number of observations for various categories of food insecurity makes it difficult to precisely estimate causal effects. With the backbone of the FFCWS in place, an expanded sample of new births (doubling in sample size) would be a substantial improvement in the ability to analyze food insecurity as well as other important policy related issues using this important data set. Furthermore, the FFCWS could be an important data set to analyze how food security changes within a family throughout childhood. Therefore, it would be beneficial for FFCWS to collect additional information on the core food security questions in all follow-up waves of the survey. 6 I. Introduction Household food insecurity is defined as “…limited or uncertain availability of nutritionally adequate and safe foods or limited or uncertain ability to acquire acceptable foods in socially acceptable ways” (Anderson, 1990). Food insecurity has been on the rise in the United States since 1999. In 2011, 14.9 percent of U.S. households experienced food insecurity, with 5.7 percent experiencing very low food security, an increase from 2010 (Coleman-Jenson, et al., 2012). Very low food security is the state in which there is a disruption in the normal quality and quantity of food consumed of at least one member in the household at some point during a given year (ERS, 2012). In 2011 20.6 percent of households with children were food insecure, and 10.0 percent of these households had children that experienced very low food security (Coleman-Jenson et al., 2012). Food insecurity can be very detrimental to a child’s current development, and future health and productivity (Nord, 2009). In trying to understand the causes of food insecurity, we believe that an understudied population is children of incarcerated parents. The number of children with an incarcerated parent has grown steadily since 1990. In 2007, 1.7 million children had an incarcerated parent--1 in 43 children in the U.S. (Sentencing Project, 2009). The number of incarcerated parents increased by 79 percent between 1991 and 2007 (Sentencing Project, 2009). In 2009, there were five states that experienced food insecurity above the U.S. average. Four out of these five states are among the top ten states for incarceration rates. Moreover, Black households and Hispanic households had higher rates of very low food security (United States Department of Agriculture, 2011) than the U.S. average. Given these statistics, it is interesting to note that both African-Americans and Hispanics are more than five times more likely and more than 2 times more likely, respectively, than their white counterparts to be incarcerated over their 7 lifetime (Bonczar, 2003). These facts provide cause to further investigate the link between food security and incarceration. While it is true that the majority of those who are incarcerated come from the type of homes that are more likely to experience food security (poor, single-parent, and minority), there is good reason to believe that the shock of incarceration may provide an explanation for why some lowincome households experience food insecurity while others do not. This is because incarceration could remove income from the household (including, in some cases, government transfer payments), incarceration imposes costs on family members (e.g., legal fees, visitation, monetarily aiding the inmate during confinement, etc.), and incarceration could have far reaching effects on parents even after their release (e.g., stigma of incarceration and interruptions in the development of skills and social networks which may lead to inferior employment outcomes upon release). Nonetheless, it is possible that incarceration could act as a life-changing event motivating the parent to improve their lifestyle. Moreover, many human capital development services (e.g., health services, skill development, and work programs) are offered behind-bars that may help to lessen the financial burden of incarceration and improve employment prospects upon release. Therefore, the above suggests that the theoretical effect of incarceration on food insecurity is ambiguous. Given the impact of incarceration on the life of the inmate and his or her family, it is clear that the role incarceration plays as a predictor of low or very low food security is an empirical question that must be explored further. The current statistics on food security suggest that food insecure families are most prevalent in single parent, black or Hispanic, low income households (USDA 2009). This profile is similar to that of incarcerated parents, which makes it more difficult to disentangle the marginal effect of incarceration on the food insecurity among 8 children. Nonetheless, it is important to do so in order to better focus current policies and reduce the probability of food insecurity. Therefore, this study seeks to provide a first attempt at identifying the causal mechanism of incarceration on food insecurity through the use of state of the art program evaluation techniques. Incarceration-Food Security Link Incarceration may impact the food security of children by removing the incarcerated parent as a source of income for the child. However, not only does incarceration hinder the imprisoned parent from financially providing for their children, incarceration may act as a shock to the household by placing an additional financial strain on the family (Grinstead et al., 2001). Incarceration may place a direct strain on the immediate family unit as well as a strain on other relatives. Children of incarcerated parents often go to live with a grandparent (Mumola, 2000).1 Ziliak et al. (2008) find that senior households living with a grandchild are at higher risk of hunger. Moreover, incarceration may limit the upward mobility of offenders and their families through three mechanisms: stigma, acquiring human capital, and obtaining social capital. Incarceration marks offenders as “untrustworthy” making it difficult for them to find employment. For example, offenders with felony records may be temporarily unable to find employment in licensed or professional positions, as well as public sector employment in some states. In addition, incarceration may weaken offenders’ job skills, hinder their attainment of job skills compared to those who are free, and lower their productivity through attrition of human capital (Cox, 2010). Empirical evidence suggests incarceration has little effect on employment, 1 13.3% of children with fathers incarcerated and 52.9% of children with mothers incarcerated live with a grandparent. 9 but has a significant negative effect on earnings (Grogger, 1995, Western et al., 2001, Kling, 2002, Holzer, 2007). Finally, there are collateral consequences to incarceration. Collateral consequences are statutory restrictions imposed on the offenders in addition to the convictions and sentences administered by the courts (Olivares et al., 1996). These limitations include prohibitions on voting, parenting, public employment, as well as debarment for certain federal benefits such as TANF, food stamps (SNAP), and public housing (American Bar Association, 2009, Sentencing Project 2009).2 Incarceration may place a ban on qualified low-income families’ participation in public assistance programs that would help to alleviate or lower the risk of food insecurity. Somewhat ironically, this is done at a time when these families are known to the corrections system and by extension to other public institutions. There is also some evidence to suggest that stricter child support enforcement during and after incarceration can also reduce work incentives for non-custodial fathers (Holzer, 2005). Nevertheless, incarceration could act as a life-altering event for the detained parent causing him or her to move away from a life of crime. Moreover, due to the availability of human capital investment programs behind bars, confined parents may leave prison or jail with an increased skill set. These events may allow the incarcerated parent to improve their life circumstances upon release, and the circumstance of their children. There is an additional argument that incarceration may benefit the family by taking the “bad apple” out of the 2 Nationally, jailed individuals do not receive SNAP benefits and convicted drug felons face a life-time ban from receiving SNAP benefits in 13 states: Alabama, Alaska, Arizona, Arkansas, Florida, Georgia, Indiana, Mississippi, Missouri, North Dakota, South Carolina, Texas and West Virginia (National Re-entry Resource Center, 2012, http://www.nationalreentryresourcecenter.org/documents/0000/1085/Reentry_Council_Mythbuster_SNAP.pdf). Children of incarcerated parents do not technically lose SNAP benefits. However, the household benefit is reduced by the amount allocated to the incarcerated adult (although their income is not included in the aid calculation for the remaining household members). In addition, based on interviews with advisors at Atlanta Legal Aid, if the incarcerated parent is the designated head of household, there may be a lapse in SNAP coverage for their children while the legal guardian gets “reconnected” to the SNAP benefit system. 10 household, thereby reducing negative influences within the household. At the extreme, this could potentially afford those remaining in the household opportunities to access education, employment, and services to increase food security (Finlay and Neumark, 2010). The causal relationship between food security and incarceration is complex. Most of the preceding arguments suggest that food insecurity is caused, in part, by incarceration. In turn, it is plausible that incarceration is a function of food security as financially and emotionally stressed parents engage in criminal activity to feed their families. Given the discussion above, it is clear that the mechanisms through which incarceration influences the food security of children must be identified empirically. Therefore, the remaining portion of this report will seek to develop and test an empirical framework to better understand the causal role of parental confinement on food insecurity among families with children using micro-level data from the Fragile Families and Child Wellbeing Study. II. Data The Fragile Families and Child Wellbeing Study (FFCWS) is a longitudinal study that is currently following a set of 4,898 families that gave birth to a child in 20 sizeable (population of 200,000 or more) U.S. cities between the years 1998 and 2000. These families are classified as “fragile” because many of the parents are not married; and, as a result, are at greater risk of dissolving relative to traditional families. The study uses a survey interview methodology administered to both the mother and father at birth and again at ages one, three, five and nine (referred to as waves).3 Each wave includes a series of core surveys. The baseline core survey is a hospital based survey taken at birth of the child. Follow up core surveys are telephone surveys 3 In 2012, the nine-year follow up interview and in-home survey became available. This wave did not capture incarceration and food security variables that were consistent with the previous surveys. Therefore, we are not able to use that wave in our analysis. 11 of the mother and father at ages 1, 3, 5, and 9. At ages 3, 5, and 9 there is also an in-home assessment and various supplemental surveys (see Figure 1 for more information). The surveys collect information on parental history and behavior, health of parents and children, socio-economic conditions, public program participation, demographic information, and the environmental conditions in which the child is raised. Most importantly, FFCWS also contains food security questions in-line with the Core Food Security Module (CFSM) in the third and fifth year in-home assessment survey, and detailed information on parental incarceration. Another major benefit of using FFCWS is that it surveys both the mother and the father about the other parent. This allows for a degree of cross-checking of responses to various questions. In general, we restrict our data to only those years that contain the Core Food Security Module: years 3 and 5. As mentioned earlier, there are 4,898 families in the base data. Of these families, 3,288 families responded to the third-year core survey and the third year in-home survey and 3,001 responded to the fifth-year core survey and the fifth year in-home survey. There are 2,489 observations for which there are responses for the baseline core survey, and the third and fifth year surveys.4 The CFSM questions are critical to this research. There are eighteen food security questions in the module. The food security module is designed to allow administrators to implement two common screens (and a third less common screen) when it appears the food security questions may pose an unnecessary burden on the respondents.5 While it is not a requirement to employ 4 The observations lost moving from the third to the fifth wave are not significantly different in terms of the mean value of the following important characteristics: mothers’/fathers’ incarceration, mothers’/fathers’ income, low/very low food security among households with children, low/very low food security among adults, and very low food security among children. 5 The first screen is comprised of the first five questions of the FFCWS food security module (questions d1a-d1e listed in Appendix A-1). If a respondent answers in the affirmative to any of these five food security questions, then they continue to the second stage of the survey comprised of the next six questions (questions d3-d7 also listed in Appendix A-1). If the respondent answers “never true” to all 5 questions in the first stage, then they can skip the remaining questions of the survey. Note that we screened individuals out in the first stage if a respondent answered 12 these screens, it appears that all three were used in the FFCWS food security questions. Taking into account the three screens allowed us to recover roughly 96% of the total sample. Utilizing recognized patterns of non-response, we imputed responses for all but 57 (.9% of the total sample) observations for household food insecurity, food insecurity among children, and very low food security among children; and all but 172 observations (2.7% of the total sample) for food insecurity among adults, very low food security in the household, and very low food security among adults using the methodology detailed in Bickel et al 2000.6 An additional complication in our analysis is defining incarceration to capture the event of removal from the family. As in previous research on incarceration using the FFCWS, we used multiple means to capture the incarceration variable including comparing the mother’s response with the father’s response and information from the previous survey for those who responded to multiple surveys to clarify answers. There are missing data for a number of variables (including incarceration) and the percent missing ranges from a high of 49.2 percent for the parent earnings variable, 29 percent for adults and children in the father’s family variable, 28 percent for the variable parent’s employment (in weeks) to a low of less than 1 percent for the following variables: adults in mother’s family, children in mother’s family, mother’s age, mother’s race, father’s race, and parental cohabitation. We impute values for many variables using STATA’s multiple imputation (MI) by chained equations methodology (MICE). Following van Buuren and Oudshoorn (1999), let X = (X1,X2,…,Xk) be a set of k random variables where each variable may have some missing at least one of the first five questions in the negative with missing values for the remaining questions in the first level internal screen. Those who move on to the second stage are screened out (i.e., they do not have to answer questions d9-d13) if they respond negative to all questions in this second stage, otherwise, they move on to the remaining six questions in the third stage of the survey (questions d9-d13). A less common screen is implemented in FFCWS that allows the respondent to skip question d13 if they had a negative response to question d12. 6 We acknowledge the help of Mark Nord in implementing these adjustments. Any errors are ours. 13 observations. The dilemma for imputation is to pull from P(X), the unconditional multivariate distribution of X. Allowing t to represent the number of iterations, and assuming the data are missing at Random (MAR), one may replicate the subsequent series of Gibbs sampler iterations: For X1: draw imputations X1t+1 from P(X1|X2t,X3t,…,Xkt) For X2: draw imputations X2t+1 from P(X2|X1t,X3t,…,Xkt) . . . For Xk: draw imputations Xkt+1 from P(Xk|X1t,X2t,…,Xk-1t) In other words, the above iterations specify that one “…conditions each time on the most recently drawn values of all other variables” (p. 9,van Buuren and Oudshoorn, 1999). If we assume P(X) is multivariate normal, then linear regression models such as X1 = X2tβ12 + X3tβ13 + …+ Xktβ1k + ε1, with ε1 ~ N(0,σ12 ) for a continuous variable (and logistic regression for binary variables) can be used to obtain a random draw from the preferred distribution (van Buuren and Oudshoorn, 1999). For our analysis, X includes the following variables: mother’s and the father’s incarceration status, the number of adults in the mother’s and the fathers’ household, the number of children in the mother’s and the fathers’ household, fathers’ age, mother’s age, the log of the sum of the mother’s and the father’s earnings (parental income), the sum of the mother’s and the father’s education level (parental education), the sum of the total number of weeks the mother and the father were employed (parental employment), mother’s race, father’s race, and parents’ cohabitation status. If it is also assumed that the multivariate distribution exists, and that values pulled from it can be produced by iteratively selecting from the conditional distribution, the multivariate imputation puzzle can be separated into a chain of univariate problems. The imputed data for the multivariate case is then estimated using the regression switching, or variable-by-variable, 14 imputation method (van Buuren and Oudshoorn, 1999). This technique is then used to create a set of Y imputed data sets (often 10 to 100), with estimation being performed on each of the imputed data sets, and the final results averaged over the J data sets (Rubin 1987, Donders et al 2006, Little and Rubin 2002). We report results using the imputed data as well as those from the original data (with list-wise deletion of missing variables). In general, the results between imputed and original are quite similar. Table 1 provides a comparison of the incidence of food insecurity in the FFCWS data (weighted) and national statistics from USDA using wave 3 data. The comparisons are not straightforward for several reasons. First, when appropriately weighted, the FFCWS is representative of births in large U.S. cities.7 Secondly, the various waves of FFCWS data are established based on the birth year of the child (birth year from 1998 to 2000) and the follow up year (one year old, three years old, etc.) so that the waves are not coincident with one calendar year (the three year wave, which is the first follow up year with the core food security module, includes families in years 2001, 2002, and 2003). Also, the FFCWS is a longitudinal data set anchored by the birth of a child. As a result, the households are young by national standards. Finally, as noted above, missing food security responses were imputed for many observations and while this is consistent with USDA’s imputation methodology, there may be some minor measurement error in the FFCWS statistics presented in Table 1. Overall, the percent of households with children in the FFCWS weighted data that are food secure is similar to the percent reported nationwide (85.2 percent and 83.3 percent respectively). The percent of children who are food insecure is somewhat smaller in the FFCWS data versus 7 The FFCWS data reported in Table 1 are weighted by the national weights which are designed to make the data in the 16 randomly selected cities in the national sample to be representative of all births in large U.S. cities: http://www.fragilefamilies.princeton.edu/documentation/core/weights/using_ffwgts_rev0709.pdf. . 15 what is reported nationally (15.5 percent versus 18.2 percent respectively), but the percent of children with very low food security is larger in the FFCWS data than what is reported nationally (1.3 percent versus 0.6 percent respectively). However, in the wave 5 data, the prevalence of very low food security in the FFCWS data is smaller than reported in the national data. Given the structure of the FFCWS data, we might expect the incidence of food insecurity to be larger than reported in the national data at least for the unweighted data, but this is not the case. We have analyzed the data carefully and do not have a definitive answer to this potential anomaly. When we break down the comparison between the FFCWS and USDA food security data by income groups (relative to the poverty level), the FFCWS data set simply contains fewer observations at all income levels. It is possible that the sampling design did not lead to a representative sample along the food security spectrum. It is also possible that there were errors in administering the food security questions in the FFCWS. We find similar results for the prevalence of basic food insecurity using FFCWS’ wave 5 observations, but for VLFS among children, the wave 5 FFCWS rates are smaller than national levels reported by USDA (with fewer total observations than in wave 3). The higher incidence of very low food security in wave 3 may be accounted for in part by the 2000-01 recession encompassing wave 3 observations. We are wary of the significance of the difference in the percent of children with very low food security in the FFCWS versus the national results because of the small sample size and the observation that the same relationship is not seen in wave 5 data. There may be a different dynamic at work between low and very low food security in urban versus rural households, which deserves additional research. 16 Table 2 provides summary statistics (unweighted) for the variables used in this analysis.8 The table also reports the mean value of the imputed data for variables that were imputed using the MICE procedure. The mean values for the food security variables show that food insecurity is more common at the household level than among children in the household. The median education for the father and mother is a high school diploma or GED. 9 Thirty-four percent of families reported a father ever incarcerated and five percent of families report a mother incarcerated.10 The average age for the mothers in our sample is 29 and the average father’s age is 31. Using the mother’s information, most families are intact about half of the time (mtogether4). Finally, 22 percent of the mothers and 19 percent of the fathers are non-Hispanic white; 50 percent of the mothers and 52 percent of the fathers are non-Hispanic black; 25 percent of the mothers and 26 percent of the fathers are Hispanic; and 3 percent of the mothers and 3 percent of the fathers are classified as other. III. Research Methods Our research question is whether incarceration increases the probability of very low food security for children. We think it is important to understand the impact of incarceration on food security for the adults and households as well and so we estimate a series of food security measures as a function of incarceration and additional explanatory variables. 8 Table 2 reports statistics for wave 3 and 5 combined. The education variable for the baseline year of the survey for the mother and the father is defined as follows: no formal education =1, less than an 8th grade education=2, some high school=3, high school diploma or GED=4, some college or technical training=5, a BA or BS=6, and graduate school=7. In each follow up survey if the mother or father indicated they have completed a degree higher than the degree they had in the previous wave, then, the education level is updated. 10 The prevalence of incarceration in FFCWS is high, even after weighting our analysis. However, they are in line with other studies (Geller, Garfinkel, Western, 2011). The high levels of incarceration are most likely due to the sampling design (incarceration is correlated with lower rates of marriage) and the fact that urban areas have higher rates of incarceration (Charles and Luoh, 2010,Wildeman and Western, 2010,Western and Wildeman, 2008, Pettit and Western, 2004, Western and McLanahan, 2000). 9 17 Since many of those incarcerated come from households that are more likely to experience food insecurity, we cannot rely on simple OLS to determine if the shock of incarceration leads to increased food insecurity among households with children, although it is useful to start with a simple OLS/probit specification to explore basic correlations. We expand our empirical analysis to program evaluation techniques in order to identify as best possible, causal effect of incarceration on food security. In particular, we will think of incarceration as a “program” that parents choose to participate in (through their decision to partake in illegal behavior). In doing so, we will implement a two-step propensity score matching technique to identify the impact of incarceration on food insecurity among households with children. The first stage of the analysis will use propensity score matching to create our matched sample, and the second stage will model the relationship between food insecurity and incarceration in a regression framework in order to get more precise results. Since propensity score matching only solves the selection problem based on observable traits, our results could still be biased by unobservable characteristics. We therefore also employ a difference-in-difference estimation strategy that seeks to identify the causal relationship between food insecurity and incarceration. Finally we also estimate the impact of incarceration at birth on food security in wave 3 and wave 5. Unless food security is a chronic problem, in this treatment, incarceration is predetermined, and not endogenously determined. We begin with the ordinary least squares (OLS) regression model to estimate the following equation:11 , where: 11 We also estimate this equation using a probit specification. 18 FISit: equal to 1 if household i suffers from food insecurity (various measures including very low food security for children) at time t, Xit: a vector of covariates for household i at time t affecting food security such as household income, employment, public assistance program participation, mother’s education, father’s education, household size, household composition, race, age (Rose, 1999), PIncit: equal to 1 if either the mother or the father has been incarcerated by time t for household i, year5: a time fixed effect equal to 1 if the interview period for the survey is the fifth year follow up wave, dit: an indicator variable equaled to 1 if the family responded in year t of the survey, εit : error term. The above analysis will provide a reasonable estimate of δ1 given the gauss markov assumptions hold. Obviously, the homoskedasticity assumption does not hold when there is a binary dependent variable, so we correct for this by implementing the sandwich estimator for robust estimation of the variance. However, it is very possible that our model suffers from endogeneity due to omitted variable bias and reverse causality between parental incarceration and food insecurity causing the zero conditional mean assumption of the error term to fail. In order to control for omitted variable bias, we can exploit the longitudinal nature of our data and estimate the following equation using the feasible generalized least squares (FGLS) estimator known as the error components model (ECM): , where uit is the error term equal to ηi+εit. With this model, it is assumed that the individualspecific effects, ηi, are manifestations of iid random variables, and are uncorrelated with the independent variables (Cameron and Trivedi, 2005). There still remain two possible sources of endogeneity with this model: 1) reverse causality between parental incarceration and food 19 insecurity, and 2) unobserved heterogeneity caused by time-varying omitted variables. Moreover, this model will be inconsistent if ηi is correlated with the independent variables in the equation. Due to the concerns above, we implement three techniques to control for the potential correlation between the individual-specific effects and the independent variable, and the possible endogenous relationship between parental incarceration and food insecurity. The first strategy exploits the panel nature of our data by using a difference-in-difference (DID) estimator. In particular, our data include information on incarceration at various collection points (years three and five). Therefore, we restrict our model to only those observations that are not incarcerated in the third year interview of the panel and use the change in parental incarceration status between the third and fifth year (no incarceration to incarcerated) in order to control for time invariant observed and unobserved factors affecting food security for families in which at least one parent is incarcerated between the third year and fifth year follow up survey. This restricts the sample to approximately 1,500 observations (before imputation of the right hand side variables), which is an admittedly selected sample and limits our ability to generalize to the at risk population. However, we believe this to be an important modeling strategy to identify the causality. Our model takes the following form: (3)FJS,L = µ + fi1Plnci. + «1yaar5 + Y1Plnc:1 where Yi = (FlS i- -r- y.carS + XidJ + 'iL , l-'/S ~) - {f,'/S ~ - FlS ~) . The parameter γ1 gives us the average difference in the probability of food insecurity between the fifth year survey (time period 1) and the third year survey (time period 0) for those who are incarcerated between year three and year five (our treatment group) and those who are not confined between survey waves and were not previously incarcerated (our control group). The 20 benefit of this estimator is that it removes time invariant unobserved heterogeneity between the treatment and control group, as well as biases that could be due to time trends of the dependent variable. However, the key assumption that may fail with this technique is the parallel trend assumption. This assumption requires the food insecurity time trend to be the same between the treatment and the control group. While this is a relatively common problem with the DID estimator we are unable to investigate the validity of this assumption in our analysis due to the short time frame of the panel data. We also estimate a specification using a two-step approach that utilizes propensity score matching with regression analysis. The first step in this method seeks to match individuals who have been incarcerated (the treatment group) to those who have not (the control group) using propensity score matching. With this method, the propensity scores are estimated using the following probit model: . Where () is the standard normal cumulative distribution function, PInc is a binary response variable equal to 1 if the parent (individual) was ever incarcerated by time t, and x is a vector of covariates that may impact a parent’s (individual’s) likelihood of being incarcerated. These regressors include the number of adults in the mother's household, the number of adults in the father's household, the number of children in the mother’s household, the number of children in the father's household, a time dummy for wave 3, an indicator variable for whether or not the household is included in both in-home surveys, the mother’s age, the mother's age-squared, the father's age, the father's age squared, the race of the father and mother (white non-Hispanic, black non-Hispanic, other), an indicator variable equal to one if the mother is Hispanic, an indicator variable equal to one if the father is Hispanic, and an indicator variable equal to one if 21 the interview took place in the 3rd year follow-up. In addition, we also include two indicator variables for whether or not the family responded to the 3rd year and 5th year of the surveys. Matching then takes place, using the radius method, on the odds ratio since the predicted probabilities of being incarcerated are not consistently estimated in choice-based samples like the FFCWS (Heckman and Todd, 2009). By using propensity score matching, we have created a treatment group and a control group that can be used to investigate how incarceration impacts food insecurity. In the second stage of the analysis we will use our matched sample to reestimate the impact of parental confinement on food insecurity using the OLS (equation 1) and ECM (equation 2) models. In doing so we will be able to more precisely calculate the average treatment effect on the treated (the incarcerated), i.e., how incarceration has impacted the food security of children in households that have experienced parental incarceration.12 Our final analysis defines incarceration by the father’s incarceration status at the child’s birth and evaluates food insecurity in waves 3 and 5. This analysis potentially breaks the endogeneity of the food security-incarceration relationship and assumes that there is no chronic food securityincarceration link. Intuitively, if the father had ever been incarcerated by the child’s birth, but had no subsequent incarceration spells, incarceration would be predetermined to any food insecurity experienced in waves 3 and/or 5. We test this hypothesis by running the ECM models of food security indicators as a function of incarceration and other previously defined determinants for wave 3 and wave 5. For those for whom food security is chronic (before and after the birth of a child), this is a somewhat less convincing argument. IV. Results and Discussion 12 In addition, the balancing property was not achieved for the education, earnings, and employment variables demonstrating the need to continue to control for these variables in our analysis. 22 Our estimation categories are as follows:13 Non-imputed explanatory variables Incarceration Baseline incarceration √ √ marginal √ √ OLS14 Probit effect GLS √ Matching √ (propensity score, probit, marginal effect) Difference-in√ Difference √ Imputed explanatory variables Incarceration Baseline incarceration √ √ √ √ √ Incarceration refers to the models where incarceration is measured as an event that may have occurred at any time before or after birth (except in the DID case). Baseline incarceration refers to the models where incarceration is pre-determined as the value of incarceration at the birth of the child. The imputed results utilize the MICE imputed data, while the non-imputed results do not (means for the imputed variables are reported in italics in Table 2 for easy comparison to the non-imputed data). All models use data that have been adjusted for imputations and reclassifications of the food security variables. Where possible, each estimator is run for the following categories of food insecurity: Very low food security among Households with children Very low food security among Adults Very low food security among Children Food insecurity among Households with children Food insecurity among Adults Food insecurity among Children 13 We do not present every combination in this matrix as many of the results are quite similar. We provide an illustrative group of results from the models in Table 3. 14 The OLS, Probit and GLS models do not treat the endogeneity, we simply acknowledge that incarceration in these models may be endogenous. 23 Appendix Table A1 provides a copy of the food security questions as reported in the FFCWS. We have two types of dependent variables—very low food security and food insecurity (a lesser level of hardship). The dependent variables are 0/1 binary variables equal to one if there is very low food security (or food insecurity) and zero otherwise. The hypothesis we test is that parental incarceration has a nonzero effect on the probability of very low food security or food insecurity. Given our previous discussion, incarceration has a theoretically ambiguous relationship with food security status. If the direct and indirect costs of incarceration outweigh its potentially “positive” influences, our expectation is that incarceration will be positively correlated with the food insecurity dummy variables—if a parent has been incarcerated, there is an increased likelihood of food insecurity. We hypothesize that family size will also positively affect the likelihood of food insecurity. Alternatively, we hypothesize a negative relationship between food insecurity and the control variables that increase family opportunities to sustain food security: parents’ earnings, parents’ education, and cohabitating parents. We control for parents’ age, family size, and parents’ race but do not assign a hypothesized value to these variables. As discussed earlier in this report, some of the estimation techniques are chosen to explicitly account for the endogeneity of incarceration and food security. The OLS, Probit, and GLS specifications do not control for the endogeneity, while the propensity score, difference-indifference, and baseline incarceration models do control for the potential endogeneity of incarceration. We present results from many of these models to help us better understand the impact of the endogeneity and to test the sensitivity of our results to alternative specifications.15 15 In this text, we focus on the incarceration coefficient but the results for all of the covariates are available upon request. 24 Table 3 presents the results of our specifications (the table contains six components). The first three components report results for very low food security and the remainder for the basic food insecurity measure. We begin with the basic OLS model (first entry of Table 3) for the column non-imputed incarceration. Although the dependent variable is binary, OLS can provide a reasonable estimate of the average partial effect of parental incarceration on food insecurity. The OLS point estimates are positive across specifications indicating that the negative effects of incarceration outweigh the positive influences. Nonetheless, in the case of very low food security for children, adults, and households, the level of significance is low. In the case of food insecurity the OLS results suggest a moderate and significant impact of incarceration on the probability of being food insecure on the order of 6 to 8 percentage points for adults and households with children. With these results, we believe that there is weak evidence of a small impact of parental incarceration on very low food security for children, adults and households, but stronger evidence of a moderate impact of food insecurity on the adults and at the household level. A larger sample might allow us to estimate these effects with more precision. The marginal effects of a standard probit model are reported in the second line of Table 3. The marginal effects are calculated at the mean value of the variables, marginal effect at the mean (MEM), using Stata’s mfx command. The marginal effects are positive, but significance is again quite weak in the cases of very low food security of children, adults, and households with children. In the case of overall food insecurity, the marginal effects are significant for adults and households and on the order of 6 to 7 percentage point impact on the likelihood of food insecurity if a parent is incarcerated. Since it is likely that we have unobserved heterogeneity in our model, we run our specification within an error components model in order to control for time invariant unobserved 25 heterogeneity (third entry in Table 3). This model is appropriate if the individual-specific effects are random, iid, and independent of the explanatory variables included in the model.16 The point estimate is not statistically significant for any of the very low food security models. In the case of basic food insecurity, controlling for invariant unobserved heterogeneity with the GLS model, we find slightly smaller significant impacts of incarceration on food security for adults and households with children than in the OLS case. Consistent with the probit model, these estimates show that parental incarceration causes the probability of food insecurity to increase by about 5 to 6 percentage points. Turning to the matching estimator (the fourth entry in Table 3), we examine the conditions of unconfoundedness (no unobserved heterogeneity) and common support. The common support assumption was first met by dropping those observations that had propensity scores lower than the minimum and greater than the maximum of the control group. We then dropped the 10 highest propensity score values and the 10 lowest propensity score values of the treatment and control groups. We restrict our common support criteria to families that have propensity scores that are greater than or equal to the highest minimum propensity score value and less than or equal to the lowest maximum propensity score value among this subgroup (Caliendo and Kopeinig, 2008). As can be seen from Figure 2, the common support criterion is now achieved: there is overlap among the propensity scores for each group, and both the treatment and the control groups have propensity scores that are less than 1. The balancing property was examined by using a t-test to determine if the matching process eliminated any statistically significant differences in the mean values of the control and treatment group. Matching eliminated all differences between the treatment and the control groups that are present prior to the 16 If the fixed-effects model is the true estimator, then the ECM model will be inconsistent. We conduct Hausman Tests that generally fail to reject the null that the coefficients are systematically different between the ECM and fixed effects model. 26 implementation of the matching procedure for all variables included in the first stage of the propensity score model. The balancing property is not met for variables such as parental employment, parental earnings, etc. Therefore, it is important to control for these variables in our main regressions. We use our matched sample to estimate a probit model of very low food security (and food insecurity) as a function of incarceration and the same set of independent variables used previously.17 In the very low food security regressions, the incarceration variable is positive but not significant for children and households. The coefficient is significant for adults, albeit quite small in magnitude (0.02). In the cases of basic food insecurity in the adult and household regressions, incarceration is positive and statistically significant and of similar magnitude to the previously reported OLS, probit, and GLS estimates at about 6 to 8 percentage points. Finally, we restrict our analysis to only those households that did not have at least one parent incarcerated by the third year follow-up survey and estimate our model using the DID approach. The DID estimator allows us to control for time trends in food insecurity as well as difference out time-invariant unobserved heterogeneity between the treatment and the control group. The generally positive coefficients are not significant in any case. Turning to the second column of results, we identify incarceration based on whether or not the father was ever incarcerated at birth (we do not have information on the mother’s incarceration status at birth). In this case, incarceration is predetermined and the issues associated with endogeneity are eliminated. We use OLS, probit, and GLS estimators with this “baseline” specification. For very low food security among children, adults, and households the coefficient on baseline incarceration is positive, but the OLS estimates are not significant. Nontheless, the OLS coefficients for all specifications are approximately .04 in magnitude. 17 We also used the matched data to estimate OLS and GLS models. The results are not qualitatively different. 27 For the basic food insecurity analyses, we see that in the baseline incarceration, the coefficient for incarceration is positive and significant for adults and that these seem to be driving the result for households. These coefficients are statistically significant and relatively large ranging in magnitude from 0.07 to 0.15. As reported above, we imputed a series of independent variables (including incarceration) using multiple imputation by chained equations. In general, the results from the various models using imputed data are similar to those of the original data but the magnitudes differ. When the coefficients are significant, we find the magnitude of the effect to be smaller using the imputed data. For example, the coefficient for incarceration in the food insecurity regressions for the household (OLS) was roughly 0.08 for the non-imputed data and 0.05 for the imputed data. Among the other covariates, the strongest performers in terms of consistent sign and significance are the education of the parents and parents’ income. The size of these coefficients is typically less than 3 percentage points. V. Conclusions Food insecurity has been on the rise in the United States. Households with children are at greater risk of experiencing food insecurity. In 2010 roughly 20 percent of households with children and almost 10 percent of children encountered food insecurity at some point during the previous year. Food insecurity among children is of concern because it not only leads to poor development in the present, but it can also impact a child’s ability to live a healthy and productive life as an adult. In this study, we used data from the Fragile Families and Child Well-Being Study (FFCWS) to estimate the impact of parental incarceration on very low food security and food insecurity of children, adults and families. The number of observations for very low food security for children 28 is small, (30 observations in wave 3 and 20 observations in wave 5), and this is a challenge for the estimation. We find that incarceration is almost universally positively correlated with very low food security among children but are not significant at standard confidence levels. Incarceration of a parent is positively correlated with food security measures for adults and households with children. The magnitudes of significant effects for these populations range from 4 to 15 percentage points. We have not identified the specific path of the incarceration-food insecurity interaction, but it is important to note that incarceration may significantly add to food insecurity in the population. We envision that this analysis will be the first of many that seek to identify the relationship between incarceration and food insecurity. Our future research goals are to conduct an in depth analysis into the causes of missing data for the food security questions that pertain to children, and the mechanism by which incarceration influences food insecurity. Incarceration can lead to loss of public services. If this were significant, incarceration could increase food insecurity if services including SNAP and TANF were at risk. To test the evidence of this relationship, we estimate the number of TANF eligible families and their reported usage of TANF (“take-up”), and cross-tabulate this with parental incarceration. The variables included in the FFCWS do not allow us to perfectly identify eligibility since it is a function of earnings and other income as well as location. We do not have these data and instead, we estimate the eligibility of families as if they lived in Milwaukee (a relatively generous TANF location) and Indianapolis (a less generous location). We then compare eligibility to reported use of TANF (any use greater than zero) and calculate take-up: number of observations that report receiving TANF/number of observations that are eligible. We tabulate this take-up for observations in which a parent was ever incarcerated and those for which there was no incarceration. The 29 incarcerated observations had a larger take-up rate than the non-incarcerated. Given the imputations needed to estimate eligibility we do not consider these results definitive, but they are certainly not in the direction we expected. Nonetheless, research by Butcher and Lalonde (2006) may lend support to this outcome. As a result of this analysis, we believe that there are a number of issues that need additional research. There is need for large samples of families like those studied in the FFCWS to better understand if the baseline levels of food security in the FFCWS are accurate. The small number of observations for various categories of food insecurity can make it difficult to analyze causal effects of food security. With the backbone of the FFCWS in place, an expanded sample of new births (doubling in size) would be a substantial improvement in the ability to analyze food insecurity as well as other important policy related issues. Furthermore, the FFCWS could be an important data set to analyze how food security changes within a family throughout childhood. Therefore, if possible, it would be beneficial for FFCWS to collect additional information on the core food security questions in all follow-up waves of the survey. 30 References American Bar Association (2009).“Internal Exile.” Chicago, IL. Bickel, Gary, Mark Nord, Cristofer Price, William Hamilton, and John Cook (2000). Guide to Measuring Household Food Security, Revised 2000. U.S. Department of Agriculture, Food and Nutrition Service, Alexandria VA. March, 2000. Retrieved from: http://www.fns.usda.gov/FSEC/FILES/FSGuide.pdf. Butcher, K., & LaLonde, R. (2006). Female Offenders' Use of Social Welfare Programs Before and After Jail and Prison: Does Prison Cause Welfare Dependency?. Harris School Working Paper Series 07.18, Chicago, IL. Retrieved from http://web.harrisschool.uchicago.edu/sites/default/files/working-papers/wp_07_18.pdf. The Center for Working Families (2012). Fragile Families and Child Wellbeing Study: http://www.fragilefamilies.princeton.edu/public.asp(accessed on May 21, 2012). Charles, K. K., & Luoh, M. C. (2010). Male incarceration, the marriage market, and female outcomes. The Review of Economics and Statistics, 92(3), 614-627. Coleman-Jensen, Alisha, Mark Nord, Margaret Andrews, and Steven Carlson (2012).Household Food Security in the United States in 2011.ERR-141, U.S. Department of Agriculture, Economic Research Service, September 2012. Cox, Robynn (2010).“Crime, Incarceration, and Employment in Light of the Great Recession.”The Review of Black Political Economy, 37 (3), 283-294. Dallaire, D.H. (2007). “Incarcerated Mothers and Fathers: A Comparison of Risks for Children and Families.” Family Relations, 56(5), 440-453. Donders, A. Rogier, Geert J.M.G. van der Heijden, Theo Stijnen, and Karel G.M. Moons (2006).“Review: A Gentle Introduction to Imputation of Missing Values,” Journal of Clinical Epidemiology, 59: 1087-1091. Feeding America (2011).Map the Meal Gap Child Food Insecurity 2011. Feeding America, Chicago, Illinois. Finlay, K., & Neumark, D. (2010). Is Marriage Always Good for Children? Evidence from Families Affected by Incarceration. Journal of Human Resources, 45(4), 1046-1088. Grinstead, Olga, Faigeles, Bonnie, Bancroft, Carrie, and Zack, Barry (2001). “The Financial Cost of Maintaining Relationships with Incarcerated African-American Men: A Survey of Women Prison Visitors.” Journal of African American Studies, 6(1), 59-69. 31 Grogger, J. (1995). “The Effect of Arrests on the Employment and Earnings of Young Men.”TheQuarterly Journal of Economics, 110(1), 51-71. Holzer, Harry J. (2007). “Collateral Costs: The Effects of Incarceration on the Employment and Earnings of Young Workers.” Institute for the Study of Labor (IZA) Discussion Paper 3318, Bonn, Germany. Holzer, Harry J., Offner, Paul, and Sorensen, Elaine (2005).“Declining Employment among Young Black Less-Educated Men: The Role of Incarceration and Child Support.”Journal of Policy Analysis and Management, 24(2), 329-350. Kling, J. R. (2002). “The Effect of Prison Sentence Length on the Subsequent Employment and Earnings of Criminal Defendants.”Princeton University, Woodrow Wilson School of InternationalAffairs. Little, R.J.A. and Rubin, D.B. (2002) Statistical Analysis with Missing Data, 2nd edition. New York: Wiley. Mumola, Christopher J. (2000). “Incarcerated Parents and Their Children.” U.S. Department of Justice, Bureau of Justice Statistics: Washington, D.C. National Re-entry Resource Center (2012). http://www.nationalreentryresourcecenter.org/documents/0000/1085/Reentry_Council_My thbuster_SNAP.pdf, accessed on October 14, 2012. Olivares, Kathleen M., Burton, Velmer S., and Cullen, Francis T. (1996). “Collateral Consequences of a Felony Conviction: A National Study of State Legal Codes 10 Years Later.” Federal Probation, 60(3), 10-17. Pettit, B., & Western, B. (2004). Mass imprisonment and the life course: Race and class inequality in US incarceration. American Sociological Review, 69(2), 151-169. Reichman, N. E., Teitler, J. O., Garfinkel, I., & McLanahan, S. S. (2001). Fragile families: Sample and design. Children and Youth Services Review, 23(4), 303-326. Royston, P. (2005). Multiple imputation of missing values: update. Stata Journal, 5(2), 188. Retrieved from: http://people.oregonstate.edu/~acock/growthcurves/royston_SJ_paper_nearly_final.pdf. Rubin, Donald B. (1987). Multiple Imputation for Non-response in Surveys. New York: Wiley. Sentencing Project (2009). “Incarcerated Parents and their Children: Trends 1991-2007.” Washington, D.C. 32 United States Department of Agriculture (2009). “Household Food Security in the United States 2008.”USDA Economic Research Service, Report #83, Washington, D.C. van Buuren, S., & Oudshoorn, K. (1999). Flexible multivariate imputation by MICE. Netherlands Organization for Applied Scientific Research (TNO) Report PG/VGZ/99.054. Retrieved from: http://www.stefvanbuuren.nl/publications/Flexible%20multivariate%20%20TNO99054%201999.pdf. Western, B., Kling, J., and Weiman, D.F. (2001).“The Labor Market Consequences of Incarceration.Crime and Delinquency, 47(3), 410-427. Western, B., & McLanahan, S. (2000). Fathers behind bars: The impact of incarceration on family formation. Contemporary Perspectives in Family Research, 2, 309-24. Western, B., & Wildeman, C. (2008). Punishment, inequality, and the future of mass incarceration. U. Kan. L. Rev., 57, 851. Wildeman, C., & Western, B. (2010). Incarceration in fragile families. The future of children, 20(2), 157-177. Ziliak, James P., Gunderson, Craig, and Haist, Margaret (2008). “The Causes, Consequences, and Future of Senior Hunger in America.” 1-88, http://216.235.203.153/Document.Doc?id=13 33 Table 1: Food Security 2003: National Data and Fragile Family Data Comparison Unit Households with children Data source USDA 40,286 FFCWS 814 USDA Children in Household Total (1,000s) FFCWS 72,969 1,847 Number Food secure (1,000s) Food Insecure (1,000s) (percent of total unit) 33,575 83.3% 694 85.18% 59,704 81.8% 1,560 84.5% (percent of total unit) 6,711 16.7% 121 14.82% 13,265 18.2% 286 15.5% Number Very Low Food Security (1,000s) (percent of total unit) 207 0.5% 13 1.65% 420 0.6% 24 1.3% Sources: USDA source is “Household Food Security in the United States, 2005” Tables 1B – year 2003; FFCWS source is the Fragile Families and Child Well Being Study data file. Notes: The FFCWS figures calculated above should be representative of data from households with children in U.S. large cities that had a child between 1998 and 2000 and the data are weighted by the third year weights for national representation. 34 Table 2: Descriptive Statistics Waves 3 and 5 (value for imputed data) Variable Description N Mean Min pedu pemp Max Total parents’ education (years) 6075 8.56 (8.56 ) 3 14 Parents’ employment (weeks) 4577 71.0 (70.2 ) 0 104 msizea Adults in mother's family 6260 2.00 ( 2.0 ) 1 9 msizek Children in mother's family 6260 2.43 (2.42 ) 0 11 fsizea Adults in father's family 4515 2.18 (2.20 ) 1 14 fsizek Children in father's family 4515 1.67 ( 1.52 ) 0 10 lpearn Parent’s earnings (log) 3277 10.4 (10.22 ) 4.6 12.8 mage Mother's age 6286 29.1 (29.1) 16 50 fage Father’s age 4754 31.8 (31.6 ) 17 71 mrace1 Mother’s race = White/Non-Hispanic 6274 0.22 ( 0.21) 0 1 mrace2 Mother's race = Black/Non-Hispanic 6274 0.50 (0.50 ) 0 1 mrace3 Mother's race = Hispanic 6274 0.25 (0.25 ) 0 1 mrace4 Mother's race = other 6274 0.03 (0.03) 0 1 frace1 Father’s race = White/Non-Hispanic 6260 0.19 (0.19) 0 1 frace2 Father's race = Black/Non-Hispanic 6260 0.52 (0.52) 0 1 frace3 Father's race = Hispanic 6260 0.26 (0.25 ) 0 1 frace4 Father's race = other 6260 0.03 (0.03) 0 1 mtogether1 Parents never live together = 1 6281 0.51 (0.51) 0 1 mtogether2 Parents rarely live together = 1 6281 0.005 (0.005 ) 0 1 mtogether3 Parents sometimes live together = 1 6281 0.02 (0.02 ) 0 1 mtogether4 Parents always live together = 1 6281 0.46 (0.46) 0 1 bothinhome Responded to both in-home surveys 6289 0.79 (.079 ) 0 1 finc Father ever incarcerated by/in wave 3 5910 0.34 (0.34) 0 1 minc Mother ever incarcerated by/in wave 3 6092 0.05 (0.05) 0 1 foodinsec Food insecurity among HH with children 6232 0.16 0 1 foodinsec_ac Food insecurity among children 6232 0.08 0 1 foodinsec_ad Food insecurity among adults 6117 0.12 0 1 vlowfdsec Very low food security among HH with children 6117 0.04 0 1 vlowfdsec_ac Very low food security among children 6232 0.01 0 1 vlowfdsec_ad Very low food security among adults 6117 0.04 0 1 Source: Authors tabulations of FFCWS data for waves 3 and 5 and in parentheses and italics are the means of the imputed data. 35 Table 3: Estimation Results by Level of Food Insecurity: Coefficient of Parental Incarceration (standard error, number of observations, R-square) Very Low Food Security among Children Non-imputed explanatory variables Imputed explanatory variables Incarceration Baseline Incarceration Baseline incarceration Incarceration OLS 0.0050 0.0431 0.0012 .012 (0.0041, 2947, (0.0270, 2264, (0.0031, 6232) (.011,3367) 0.016) 0.0326) Probit (marginal effect) 0.0019 0.0163 (0.0017, 2680) (0.0128, 2063) GLS 0.0044 0.0410 0.0007 .013 (0.0038, 2947) (0.0322, 2264) (0.003, 6232) (.013, 3367) Matching (propensity 0.002 score, probit, marginal (0.0019, 2472) effect) Difference-in-Difference Insufficient -0.0039 observations (0.015,2642) ------------- ------------- ~~~ ------------- -------------- OLS Probit (marginal effect) GLS Matching (propensity score, probit, marginal effect) Difference-in-Difference OLS Probit (marginal effect) GLS Matching (propensity score, probit, marginal effect) Difference-in-Difference Very Low Food Security among Adults Non-imputed explanatory variables Imputed explanatory variables Incarceration Baseline Incarceration Baseline incarceration Incarceration 0.0186 0.0383 0.0128 0.019 (0.010, 2894, 0.021) (0.036, 2233, 0.0354) (0.007, 6117) (0.011, 3367) 0.0155 0.0174 (0.0082, 2763) (0.0183, 2081) 0.0178 0.0390 0.0099 0.0126 (0.010, 2894) (0.041,2233) (0.0066, 6117) (0.013, 3367) 0.0197* (0.0092, 2488) 0.046 (0.0384, 1513, 0.0235) -0.013 (0.0325, 2642) Very Low Food Security among Households with Children Non-imputed explanatory variables Imputed explanatory variables Incarceration Baseline Incarceration Baseline incarceration incarceration 0.0125 0.0365 0.0060 0.027 (0.0094, 2894, 0.024) (0.0362, 2233, 0.029) (0.007, 6117) (0.020,3309 ) 0.0096 0.0158 (0.0069, 2763) (0.0172, 2081) 0.0110 0.0375 0.0033 0.031 (0.0097, 2894) (0.041, 2233) (0.0067, 6117) (0.021, 3309) 0.0109 (0.0075, 2556) 0.0158 (0.045, 1513, 0.0255) -0.0202 (0.032, 2642) Table 3: continued 36 OLS Probit (marginal effect) GLS Matching (propensity score, probit, marginal effect) Difference-in-Difference OLS Probit (marginal effect) GLS Matching (propensity score, probit, marginal effect) Difference-in-Difference OLS Probit (marginal effect) GLS Matching (propensity score, probit, marginal effect) Difference-in-Difference Food insecurity among Children Non-imputed explanatory variables Incarceration Baseline incarceration 0.0082 0.0263 (0.0133, 2947, 0.038) (0.0428, 2264, 0.047) 0.0078 0.0088 (0.0102, 2947) (0.0228, 2264) 0.0068 0.0265 (0.0133, 2947) (0.045, 2264) 0.0098 (0.011, 2731) 0.0432 (0.0594, 1538, 0.045) Food insecurity among Adults Non-imputed explanatory variables Incarceration Baseline incarceration 0.0632*** 0.1253* (0.0167, 2894, 0.055) (0.057, 2233, 0.058) 0.0557*** 0.075 (0.015,2894) (0.042, 2233) 0.0522** 0.1363* (0.016, 2894) (0.060, 2233) 0.0636*** (0.0159, 2677) 0.0415 (0.0712, 1513, 0.047) Imputed explanatory variables Incarceration Baseline incarceration 0.0135 0.0253 (0.0096, 6232) (0.010,3367) 0.0117 (0.0096, 6232) 0.0310 (0.0276,3367) 0.0214 (0.0415, 2689) Imputed explanatory variables Incarceration Baseline incarceration 0.0411*** 0.082** (0.0114, 6117) (0.031,3309) 0.0326*** (0.011, 6117) 0.096** (0.035,3309) 0.0076 (0.047, 2642) Food insecurity among Households with Children Non-imputed explanatory variables Imputed explanatory variables Incarceration Baseline Incarceration Baseline incarceration incarceration 0.0772*** 0.1265* 0.0485*** 0.085** (0.0186, 2947, 0.069) (0.059, 2264, 0.065) (0.0127, 6232) (0.033,3367) 0.068*** 0.077 (0.0169, 2947) (0.045, 2264) 0.0574*** 0.146* 0.0384** 0.098** (0.0172, 2947) (0.062, 2264) (0.0122, 6232) (0.036,3367) 0.0776*** (0.0178, 2714) -0.0209 0.0143 (0.0809, 1538, 0.062) (0.054, 2689) Notes: Baseline results are for combined wave 3 and wave 5. “*” significant at the 5% level, “**” at the 1% level and “***” at the .1% level 37 Figure 1: Fragile Families Survey Waves Baseline Age 1 Age 3 Age5 Age 9 core mother core mother core mother core mother core mother survey /in hosoital\ survey /teleohonel survey /teleohonel survey /telephone) survey (telephone) core father survey /teleohonel core father survey /teleohonel core father survey (telephone) core father survey /telephone) primary caregiver survey; in-home assessment primary caregiver survey; in-home assessment primary caregiver survey; in-home assessment child care provider survey and assessment ( 10 cities) kindergarten survey (10 cities) teacher survey (20 cities) core father survey (in hospital or by teleohonel mecical records extraction child survey (in-home) Source: The Center for Child Well-Being (2012) http://www.fragilefamilies.princeton.edu/study_design.asp 38 Figure 2: Common Support Assumption (All Cases) Household Food Insecurity Untreated -3 -2 -1 Treated 0 1 -3 -2 -1 0 psmatch2: Propensity Score Graphs by psmatch2: Treatment assignment Child Food Insecurity 39 Untreated -3 -2 -1 Treated 0 1 -3 -2 -1 0 psmatch2: Propensity Score Graphs by psmatch2: Treatment assignment Adult Food Insecurity Untreated Treated ~ ·1n C: Q) 0 -3 -2 -1 0 -3 -2 -1 0 psmatch2: Propensity Score Graphs by psmatch2: Treatment assignment Household Very Low Food Security 40 Untreated -3 -2 -1 Treated 0 1 -3 -2 -1 0 psmatch2: Propensity Score Graphs by psmatch2: Treatment assignment Child Very Low Food Security Untreated Treated ~ ·1n C: Q) 0 -3 -2 -1 0 -3 -2 -1 0 psmatch2: Propensity Score Graphs by psmatch2: Treatment assignment Adult Very Low Food Security 41 Untreated -3 -2 -1 Treated 0 1 -3 -2 -1 0 psmatch2: Propensity Score Graphs by psmatch2: Treatment assignment 42 Appendix A-1: The Fragile Families and Child Well Being Study Core Food Security Questions Variable name Question First Stage d1a We worried whether food would run out before we got more money d1b The food that we bought didn’t last and we didn’t have money for more d1c We couldn’t afford to eat balanced meals Responses 1 = often true 2 = sometimes true 3 = never true 1 = often true 2 = sometimes true 3 = never true 1 = often true 2 = sometimes true 3 = never true d1d We relied on a few low-cost 1 = often true foods to feed the children 2 = sometimes true 3 = never true d1e We couldn’t afford to feed 1 = often true the children a balanced meal 2 = sometimes true 3 = never true 1st Level Internal Screen Second Stage d3 The children were not eating 1 = often true enough because we just 2 = sometimes true couldn’t afford enough food 3 = never true d4 In the past 12 months did 0 = no you cut the size of 1 = yes meals/skip meals because of not enough money for food? d4a In the past 12 months, how 1=almost every often did adults cut size month of/skipped meals because 2=some months but not enough money? not every month 3=only 1 or two months d5 In the past 12 months did 0=no you ever eat less than 1=yes desired because there wasn’t money for food? d6 In the past 12 months were 0=no you ever hungry, but not eat 1=yes because you couldn’t afford enough food? d7 In the past 12 months have 0=no Classification as affirmative response 1 or 2 1 or 2 1 or 2 1 or 2 1 or 2 1 or 2 1 1 or 2 1 1 1 43 you lost weight because 1=yes there wasn’t enough food? 2nd Level Internal Screen Third Stage d9 In the past 12 months did 0=no you/any adults ever not eat 1=yes for a whole day because of a lack of money? d9a How often did adults not eat 1=almost every for a whole day because not month enough money? 2=some months but not every month 3=only 1 or two months d10 In the past 12 months did 0=no you ever cut the size of 1=yes children’s meals because of a lack of money d11 In the past 12 months did 0=no children ever skip a meal 1=yes because of a lack of money? d11a How often did children skip 1=almost every meals because lack of month money? 2=some months but not every month 3=only 1 or two months d12 In the past 12 months were 0=no children ever hungry, but 1=yes you couldn’t afford more food? 3rd Level Internal Screen (Uncommon) d13 In the past 12 months did 0=no children ever not eat for a 1=yes whole day because there was not enough money? 1 1 or 2 1 1 1 or 2 1 1 Source: Fragile Families and Child Wellbeing Study and Bickel et al. (2000) Households with food insecurity among children are defined by affirmative answers to two or more of the following questions: d1d, d1e, d3, d10, d11, d11a, d12, d13 Households with very low food security among children are defined by affirmative answers to five or more of the following questions: d1d, d1e, d3, d10, d11, d11a, d12, d13 44