University of Toronto Mechoulan Research Paper Re the External Effects of Black Male Incarceration on Black Females 2007
Download original document:
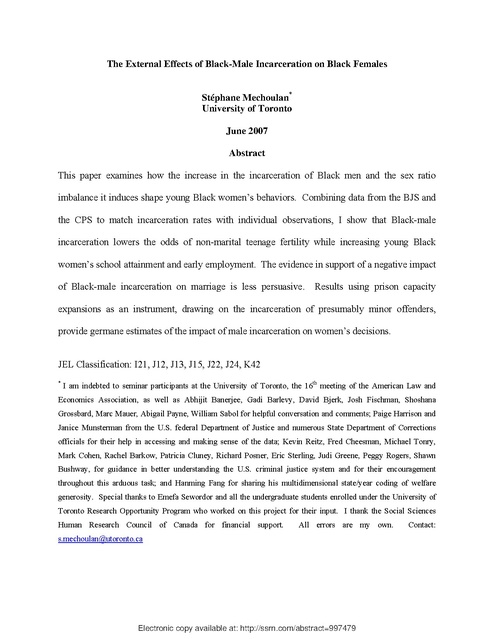
Document text
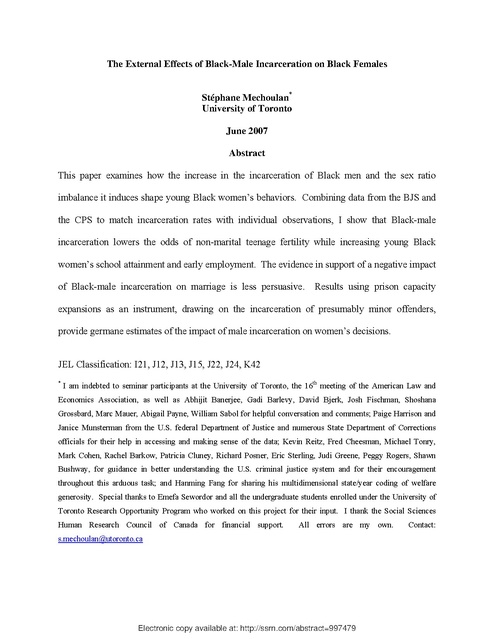
Document text
This text is machine-read, and may contain errors. Check the original document to verify accuracy.
The External Effects of Black-Male Incarceration on Black Females Stéphane Mechoulan* University of Toronto June 2007 Abstract This paper examines how the increase in the incarceration of Black men and the sex ratio imbalance it induces shape young Black women’s behaviors. Combining data from the BJS and the CPS to match incarceration rates with individual observations, I show that Black-male incarceration lowers the odds of non-marital teenage fertility while increasing young Black women’s school attainment and early employment. The evidence in support of a negative impact of Black-male incarceration on marriage is less persuasive. Results using prison capacity expansions as an instrument, drawing on the incarceration of presumably minor offenders, provide germane estimates of the impact of male incarceration on women’s decisions. JEL Classification: I21, J12, J13, J15, J22, J24, K42 * I am indebted to seminar participants at the University of Toronto, the 16th meeting of the American Law and Economics Association, as well as Abhijit Banerjee, Gadi Barlevy, David Bjerk, Josh Fischman, Shoshana Grossbard, Marc Mauer, Abigail Payne, William Sabol for helpful conversation and comments; Paige Harrison and Janice Munsterman from the U.S. federal Department of Justice and numerous State Department of Corrections officials for their help in accessing and making sense of the data; Kevin Reitz, Fred Cheesman, Michael Tonry, Mark Cohen, Rachel Barkow, Patricia Cluney, Richard Posner, Eric Sterling, Judi Greene, Peggy Rogers, Shawn Bushway, for guidance in better understanding the U.S. criminal justice system and for their encouragement throughout this arduous task; and Hanming Fang for sharing his multidimensional state/year coding of welfare generosity. Special thanks to Emefa Sewordor and all the undergraduate students enrolled under the University of Toronto Research Opportunity Program who worked on this project for their input. I thank the Social Sciences Human Research Council of Canada for financial support. All errors are my own. s.mechoulan@utoronto.ca Electronic copy available at: http://ssrn.com/abstract=997479 Contact: I. Introduction Over the last three decades the United States has experienced a dramatic surge in imprisonment that has specifically affected the Black community. Blacks are now incarcerated at nine times the rate of non-Hispanic Whites and comprise more than 40% of inmates. One in eight Black males age 25-29 was behind bars in 2004.1 Given current trends, one Black male child out of three will go to prison or jail at some point in his lifetime. High male-incarceration rates wreak havoc on the social structure of the Black community. In particular, as the prevalence of imprisonment is more than fifteen times higher for Black men than for Black women, Black women face a momentously unfavorable sex ratio.2 The analysis of Black women’s choices when facing a shortage in the supply of men provides a distinctive test of the standard model of market behavior in family economics. Yet, the collateral, unintended effects of Black-male incarceration on single Black females’ socio-economic outcomes are largely unexplored. Once quantified, the effects of incarceration may be fruitfully contrasted with the effects of explicit policies designed to improve those same outcomes. 1 Source: Bureau of Justice Statistics Prison and Jail Inmates at Midyear report, 2004. This is not counting those under bail, probation, parole, or hiding from the Justice system etc. In contrast, 1 in 28 Hispanic males and 1 in 59 White males were incarcerated in the same age group. 2 This imbalance is made even worse by the greater tendency of Black males to marry non Black females than the reverse, the greater enlistment in the military, the higher mortality rate among adult Black males, the higher rate of mental institutionalization, etc. (Tucker and Mitchel-Kernan, 1995). See for example the vivid excerpts from Black female interviewees in Lane et al. (2004). 1 Electronic copy available at: http://ssrn.com/abstract=997479 It is conceivable that a growing fraction of young Black women would decide to forego early motherhood, continue their studies or become financially independent through employment because of the mass confinement, hence shortage, of Black men. Indeed, the Black non-marital teen pregnancy rate, although higher in absolute terms, has decreased faster than its White counterpart in the last fifteen years (National Center for Health Statistics [NCHS], 2005). Further, Black women catch up in education (Allen et al., 2005) and in the labor market (Offner and Holzer, 2002; Western and Pettit, 2005) better than Black men relative to Whites. To examine how the rising levels of incarceration of Black men lead young Black women to change important lifetime decisions I compiled data on the number of male prisoners by race, gender, state and year from the Bureau of Justice Statistics (BJS). From there, I constructed male prison rates per adult male population using the U.S. Census. I then merged Census adjusted-BJS prison statistics with individual-level information on fertility, schooling, employment and marriage from the June and March supplements of the Current Population Survey (CPS) so that they match on a race, year and state basis. The benchmark Ordinary Least Squares (OLS) model allows for disentangling the impact of incarceration from year effects, state effects, secular trends in socio-economic changes within states, as well as from other identifiable variables that are expected to affect the outcomes of interest within states over time. I also implement an original Instrumental Variables (IV) strategy, predicting incarceration through the “suction effect” of prison capacity expansions uncovered in certain states. To summarize, I find that higher rates of Black-male incarceration have significantly lowered the odds of non-marital teenage fertility among young Black females, with the caveat that the average effect is driven by a small number of repressive states. I also find evidence of a positive effect of Black-male incarceration on Black women’s school attainment and early employment. 2 The evidence in support of a negative effect of Black male incarceration on marriage is weaker. The IV estimations suggest that the average effects encompass heterogeneity in the types of offenders that are being incarcerated and accordingly in their impact on women’s decisions. This work relates to several branches of a literature spanning different disciplines. Social scientists have long been intrigued by the consequences of sex ratio imbalances. In an influential book, Wilson (1987) expressed concern over the decline in acceptable marriage partners, or “quality men” in the Black community, usually defined as men with a stable job. Wilson’s ideas sparked a body of research on the impact of “quality men’s” scarcity (Horton and Burgess, 1992; Lichter et al., 1992; Kiecolt and Fossett, 1997; Neal, 2004). However, male (un)availability originates from multiple factors that were often left aggregated. Each of those factors, in turn, may have a different effect that needs to be separately estimated. As economic conditions improved in the 1990s, incarceration pursued an exponential trajectory.3 Legal scholars, psychologists and sociologists devoted more attention to the consequences massive incarceration may have on inner cities communities (Vera Institute of Justice, 1996; Hagan and Dinovitzer, 1999; Lynch and Sabol, 2003a and 2003b), yet little work has been done on the effects of imprisonment on family structure.4 Looking into the contribution of economics to crime and policy issues, most of the research has focused on the criminals themselves, whether on the determinants of criminal activity, the deterrence effectiveness of various policies, or the labor market consequences of incarceration 3 +360% in prisoners per inhabitant alone between 1978 and 2004 – see Mincy (2006). 4 Some research has investigated the impact of incarceration within the inmates’ families (Western and McLanahan, 2000; Thomas, 2006; Oliver et al., 2006), yet not on single women who are among the secondary victims of massscale male incarceration (Garland, 2001; Mauer and Chesney-Lind, 2002; Meares, 2004). 3 after release from prison. The role of aggregate male incarceration on single women’s fertility, human capital accumulation, employment decisions, etc. has been neglected. The present study is closest to that of Charles and Luoh (2006) which estimates the impact of male incarceration on selected female outcomes. They observe that women overwhelmingly marry slightly older men from the same race and state. Since the U.S. decennial Census tells us who is institutionalized, which is approximately the same as incarcerated (see Harcourt, 2006), they use the last three waves of that data set to match outcomes of women of different age groups, race, and state to the corresponding incarceration rates among slightly older men. Charles and Luoh (2006) find that rising levels of male incarceration have lowered the likelihood that women marry and have caused a shift in the gains from marriage away from women. In response to these changes, they also find that women have increased their schooling and labor supply. Building on the present findings, Kumdar (2007), also using the Census, argues that teen fertility is significantly negatively related to the incarceration rates of males likely to father the babies of teen mothers, and unrelated to the incarceration rates of males unlikely to father those babies. This study differs from Charles and Luoh (2006) and Kamdar (2007) on several counts, mainly: (1) I dispose of annual – as opposed to decennial – waves, therefore I can better pinpoint which period is driving the results – in particular, I can use male incarceration rates preceding the outcomes under investigation;5 (2) I tap into a more comprehensive set of controls; (3) more specifically, with respect to Charles and Luoh (2006), I apply an alternative set of IVs, which incidentally confirms that variables affecting incarceration are not color-blind; and (4) as a matter of exposition, I differentiate my results by racial groups – focusing on Black women – as opposed to leaving them averaged, which reveals additional insights. 5 More generally, I am not constrained to use those rates concomitant to the observations. 4 II. Data This work uniquely combines different data sets to assess the impact of Black-male incarceration rates on Black female outcomes. My statistics for incarceration come from the “Correctional Population in the United States” series (1985-2003) and “Prisoners in State and Federal Institutions on December 31st” series (1978-1984), both from the BJS. Prison statistics by race were first released in 1978. Coincidentally 1978 roughly corresponds to the beginning of the giant wave of incarcerations that has been sweeping the United States since. With a few exceptions, these data give the numbers of prisoners by gender and race for every year in every state.6 I focus on prison statistics because of the misleading and inconsistent nature of jail statistics.7 I cannot subtract the number of federal prisoners from total prisoners in each state and year, so the numbers collected represent both types; however, the overwhelming majority of prisoners are state prisoners (89% in 2000).8 Note that the proportion of state 6 For prisoners at the state level there was no specific category for Hispanics before 2000. States could include Hispanics under Whites, or could categorize them as Unknown Race but it appears that in some cases Hispanics were not counted altogether. Also, some states changed their labeling over time, making comparisons across years difficult. In such cases, with great caution, I retained as much information I could so that within each state, the White male prisoners’ series displays consistency (notably, this led to the deletion of the White prisoners series for California after 1994 and for Texas before 1986). These considerations only play a role for the estimations requiring data on the number of White male prisoners since the fraction of Black Hispanics is negligible (~ 2%). 7 It is difficult to separate jail and prison populations and to prevent double counting as more jails began to hold state and federal prisoners through the years. In a few small states, the prison figures used include both jail and prison inmates because jails and prisons are combined into one system in those jurisdictions. 8 Federal prisoners may be held in another state because of the relatively small number of federal prisons. 5 prisoners incarcerated in a state different from the one they lived in at the time of committing their offense is negligible and does not affect the assignment of prisoners by state.9 This is of great importance because it gives us confidence that the evolution of male incarceration in one particular state would directly affect females in that state.10 To transform the raw figures of inmates into percentages of the adult population in each year and state, I use the U.S. Census Estimates 1970-2000 provided by the Center for Disease Control (CDC) Wonder website which gives in each state and year the number of inhabitants by gender, race and 5-year age group. Unfortunately, the BJS, which releases data by gender, race, state, and year does not break them by age. Since roughly 95% of prisoners are between the age of 20 and 54 for each race, I use the number of males age 20-54 as the deflator. As incarceration is rising over time and feeds mainly from men in their 20s and 30s, such an approximation would tend to underestimate the most relevant incarceration rates to young women e.g., that of males slightly older than them. Thus, the effects found in this study may be viewed as a lower bound of the true effects I try to measure. The main explanatory variable of interest is therefore the incarceration rate of Black males per 20-54 Black male population. Table 1 presents descriptive statistics for all fifty states: note that min. and max. values correspond in all but a few cases to the two end points of the period, 1978 and 1999. I also looked at the same statistics with states grouped in regions by Circuit 9 Some states use other states’ facilities to hold some of their populations. Yet, even if state prisoners are held outside a state prison, they are in their jurisdiction counts, even if another State has actual custody. 10 It is of course possible that a criminal committed an offense in a state in which he does not live. Charles and Luoh (2006) present evidence that this is negligible. 6 Court. Apart from Circuit Court 1, average incarceration by Circuit Court is fairly uniform over the country. The main differences are not so much by region but by state: indeed, a few states play a disproportionate role in the empirical estimations. Graph 1 shows the dramatic evolution of the average male incarceration rate per 20-54 male population by race over time in the U.S. I use CPS data for the dependent variables and individual covariates, including indirectly CPS data for Black male unemployment rates, which have been compiled and released through the Bureau of Labor Statistics. I also use different measures of state level welfare generosity collected by Fang and Keane (2004) over the same period. For the estimation of teenage fertility, the information comes from the June CPS. I use the variable “number of babies” to construct an indicator for whether a woman has had a child.11 In Graph 2 I plot the total teenage pregnancy rate by race for women age 18-19 from 1964 to 2001 from the NCHS. Graph 2 shows a relative stability among White females over the entire period: more precisely, an increase in the mid 1980s to early 1990s, followed by a decrease later on. For Black females, the same movement occurs in the mid 1980s and early 1990s but the decline is more pronounced. The general decline over the 1990s led a columnist to write: “In the past decade, possibly no social program has been as dramatically effective as the effort to reduce teen pregnancy, and no results so uniformly celebrated. Between 1990 and 2000 the U.S. teen pregnancy rate plummeted by 28 percent (…) Births to teenagers are also down, as 11 Prior to 1990, fertility questions were only asked to married women only or women 18 and above. Years 1986- 1988 are excluded because the question on fertility was asked to married women only. Also, fertility was not part of the questionnaire in 1991, and the June CPS is not available for years 1993, 1996-1997 and 1999, which leaves us with 1979-85, 1990, 1992, 1994-1995, 1998, and 2000. 7 are teen abortion rates. It’s an achievement so profound and so heartening that left and right are eager to take credit for it, and both can probably do so.” (Mundy, 2006) Many hypotheses can be advanced to account for this phenomenon: liberal sex education, abstinence initiatives, welfare reforms, etc. Be that as it may, it is unclear from the graph alone whether male incarceration rates contributed to the decline in teenage motherhood. I also looked at education, labor-force participation and marriage. For those variables, I use the March CPS data since the collection contains fewer gaps. To summarize the patterns that characterize educational attainment of women by race, one may observe a convergence in attainment between Black and White females regarding high school completion, but the trend started well before the mass incarceration movement. The gap for college enrollment and completion slightly widened because the proportion of White females who go to college increases faster than its counterpart among Blacks. In particular, the 1980s are a lost decade for Black women regarding education. The proportion of Black women with a four-year degree or enrolled in college picks up again in the early 1990s, not long after an increase in the growth rate of male incarceration in the late 1980s. However, the evidence of a link between the two patterns is unclear. Overall, female employment increased at a declining rate from the late 1970s to the early 1990, after which it leveled off before picking up again in the mid 1990s (Fullerton, 1999). Regarding the evolution of full time employment for young women by race and different age brackets the most striking feature is the catch-up between Black and White women over the period, in particular a general decrease for Whites as well as a slight increase for Blacks, 8 especially in the 1990s. While it is not apparent that incarceration rates can explain the convergence, the absence of decline in young Black-women employment rates is puzzling. As for the proportion of unmarried women by race and age brackets, the essential feature is a slow and continuous evolution capturing the decline in the institution of marriage, for both Blacks and Whites, and for all ages. There is no graphical evidence to support the hypothesis that marriage and male incarceration are causally related since the growth in male incarceration is much faster than the growth in the proportion of never-married women (especially for Blacks). III. Methods and Estimation Strategies Let us consider a linear model estimating the impact of male incarceration on any single female outcome: Outcome = α + β Incarceration + ∑ λ j Characteristics j + ε . (1)12 j At the aggregate level, large male-incarceration rates should have some impact over a female individual’s lifetime, yet the main difficulty in assessing this relationship lies in the assignment of incarceration rates to individual observations. 12 A matching based on race and state is To keep things simple at this stage I do not consider the incarceration rate from a different racial group on the outcome of an individual of a given race. 9 intuitive.13 But at what age are these rates most relevant? Further, should one consider some average local male-incarceration rate over a certain number of years before the time when the individual is observed? The absence of definite, undisputable answers to such modeling questions must have played a role in the relative absence of quantitative studies on this issue. This is why the present work focuses on women in their late teens and early twenties. First, this narrow age range corresponds to a particularly critical point in a woman’s life-cycle; that is, when she is at risk of not completing high school, of becoming a single teenage mother and not joining the labor market. Second, if local incarceration plays a role in such decisions, it is likely that the most influential incarceration rate is that which immediately precedes those decisions. At the very least in this way one conservatively limits the chances of mismatch between relevant incarceration rates and outcomes of interest. In this paper, I present the results obtained with a one-year lag between incarceration rates and observed outcomes to capture the response of women to the latest incarceration rate they have experienced. In other words, for all the estimations, the male incarceration rate at the end of year t-1 is matched with observations in year t: Outcomeist = α + β Incarcerationst −1 + ∑ λ j Characteristicsistj + ε ist (2) j where i, s, t index the individual, state and year respectively. Note that the approach is flexible enough to accommodate alternative lag structures if one believes different outcomes respond to incarceration with different delays. 13 The fraction of Black women marrying non-Black men has been less than 5% on average in the last thirty years (U.S. Decennial Census 1980, 1990 and 2000) – see also Brien (1997). Similarly, the percentage of marriages where the bride and the groom are residents of different states is negligible. 10 I assume throughout that decisions made by young women do not cause the behaviors that result in men being incarcerated, such as drug possession or violent crimes, nor that they cause the policies that influence incarceration, such as legal changes, changes in law enforcement personnel per capita or prison constructions. A related concern is that women’s decisions are driven by males’ conduct. In that case, young women would change their behavior over time because men are becoming less suitable as husbands, not because they are locked up. However, there is evidence that changes in male-incarceration rates over time are not caused by changes in male behavior but rather by changes in policy.14 BJS statistics show that violent crime exhibited no clear direction from the early 1970s up to the early 1990s and has been rapidly going down since. For drug charges, according to the U.S. National Household Survey on Drug Abuse, an estimated 14.8 million Americans, about 6.7% of the household population age 12 and older, used illegal drugs on a current basis in 1999. Note that the proportion of Blacks is close to that of Whites (7.7% vs. 6.6%) even though Blacks are arrested on drug charges at several times the rate of Whites (the racial disparity in arrests on drug charges increases significantly over the period). This number of past-month drug users declined by more than 50% from the 1979 high of 25 million (14.1% of the population). This is precisely the beginning of the period covered here.15 The discrepancy between increase in incarceration and decline in criminal behavior is mainly the result of the so-called War on Drugs. Similar drug 14 This argument enables us to counter the hypothesis that Black-male incarceration is partly a consequence of Black female empowerment (precisely, through increased schooling, employment etc.). The possibility of reverse causality would have made the problem infinitely more complex to analyze. 15 Charles and Luoh (2006) concur based on juvenile statistics (a good predictor of future adult behavior). 11 offenses, notably possession – for which, again, prevalence does not increase – are prosecuted more aggressively, resulting in a higher likelihood of being brought to courts.16 This being said, the interpretation of male incarceration effects on women is in principle twofold: the removal of some men from the population (direct effect) and the tougher approach to crime inducing better behavior among those who are not arrested (indirect effect). Given that incarceration increases by many times more than the decrease in criminal behavior during the period, it is legitimate to consider that the direct effect dominates the indirect effect. Note however, that the indirect effect should bias the measurement of the direct effect towards zero. In the following discussion, I interpret the effect of incarceration as mostly the direct effect. Still, the identification of the causal impact of incarceration is not straightforward because of the numerous potential confounding factors associated with incarceration. It is well known that using a single cross section to tackle such a problem is inadequate. When pooling cross sections, year effects control for the evolving unobserved national attributes that affect the different outcomes of interest. Similarly, state fixed effects control for time invariant unobserved influences that vary across states. Yet, the factors that affect incarceration may also vary within a state over time: simply controlling for year and state effects could still bias the estimation of the incarceration coefficients.17 To cope with this problem, the specifications can be made more 16 According to Charles and Luoh (2006), the fraction of drug offenders increases because a higher fraction of cases brought to state courts are drug related. In contrast, for all drug charges, they do not detect a change in the probability of conviction, or a change in the probability of imprisonment conditional on conviction, or a change in the average sentence conditional on imprisonment. 17 This would happen if those changing factors within states are correlated with variations in incarceration and if such factors do not change at a national level uniformly and do not get picked up by the year effects. 12 flexible by adding interaction terms between state effects and a time trend and between state effects and the square of a time trend (see for example Friedberg, 1998). These terms, therefore, capture slow drifts in state-level characteristics that influence the outcomes of interest with the slopes of those trends allowed to vary smoothly within states. Such local changes can be of political, socio-economic or demographic nature.18 The benchmark model can be rewritten, with standard notations: Outcomeist = α + β Incarcerationst −1 + γ 1t + δ 1s + μ (Trendt × 1s ) +ν (Trendt2 × 1s ) + ∑ λ j Characteristicsistj + ε ist (3) j In theory, variations across time and within states translating into discrete jumps in incarceration rates would provide a good source of identification that enables disentangling incarceration paths from state effects, year effects and secular trends in socio-economic changes within states. In practice, however, it can be observed that in almost every state, incarceration increases every year over the period – although not always at the same rate, which is of course crucial. In other words, the causal effects of incarceration need to be identified against the monotonic trends that characterize incarceration paths: at the state level, there is just enough variability around a linear-quadratic trend for adequate identification.19 18 Note that they also include the possible social impacts of mass incarceration on disadvantaged minority communities through the increasing concentration of released prisoners over time. These agglomeration effects may affect social norms and formative institutions of social control and organizations, which in turn can exert an independent impact on female outcomes. For simplicity, I do not consider these possible effects in the present analysis. 19 I am thus “pushing the limits” of the identification strategy. To give an idea, an OLS regression of adult Black- male incarceration rates on a linear and a quadratic trend alone would yield an adjusted R2 over 0.9 in most states. 13 Notwithstanding the difficulty, I use linear probability models which successively control for year effects, state effects and state linear and quadratic time trends. Because of the “black box” nature of this approach, I further try to characterize which are the main variables potentially correlated with incarceration and the outcomes of interest that those state-level trends absorb. I also test the sensitivity of the results to adding some relevant variables that change within state over time, notably state-level Black male unemployment rates and a large list of variables measuring local, time-varying welfare generosity. Robust standard errors clustered by state account for the heteroskedasticity of the error terms and for serial correlation, as recommended by Bertrand, Duflo, and Mullainathan (2004). The use of individual observations on Black women largely self-weights the sample to emphasize the states with a large Black population. The interpretation of the coefficients becomes the impact of male incarceration on the average young Black woman in the U.S., as opposed to the average effect of Black male incarceration across states. Conceptually, the former is more important for policy analysis, and may also reveal more insights for at least two reasons. First, we may think that the effects should be better captured in the more heavily populated states because criminal activity (hence arrests) exhibits increasing returns with respect to population density, which is correlated with population size. Second, in states with a (relative) large Black population, interracial marriage is more atypical.20 Since the underlying assumption is that the incarceration of Black males affects the local relationships market within Blacks, the 20 This can be inferred from the Vital Statistics section of NCHS: between 1980 and 1988, a majority of states reported the number of marriages by race of bride and race of groom. It is readily seen that for Black women, intraracial marriage is positively correlated with the local proportion of Black males (relative to total males). 14 effects are expected to more accurately reflect the relevant sex ratio in states with a large Black population.21 To provide additional robustness to the results, I use two different strategies. First, I suspect a stronger impact of incarceration for Blacks than for Whites. This is because the Black teenage fertility rate is higher, while the female educational achievement, labor-force participation are lower than those of Whites – thus leaving more room for a detectable marginal response. Alternatively, even if there is no racial difference but the effect of male incarceration on females is nonlinear (e.g., threshold effects), given that Blacks are on average eight to nine times more likely to be incarcerated than Whites, an interaction term Black × Incarceration would specifically reflect this nonlinear impact. To that effect I assign the White-male incarceration rate to White females and the Black-male incarceration rate to Black females and run regressions over both groups. However, Whites are not a perfect control group for Blacks; rather I am evaluating treatment intensities in two groups that otherwise differ systematically. To account for such differences, I control for the race-specific incarceration rate and add all the other controls (year effects, state effects, etc.) interacted with the White/Black dummy. The interpretation of the interaction term coefficient is then the pure differential effect of incarceration between Blacks and Whites, and the race-specific coefficient becomes mechanically the incarceration coefficient for the White group only. With standard notations: 21 Note that results using non-weighted aggregated state-level data support most of the conclusions advanced in the paper and are available upon request. 15 Outcomeirst = α + β Incarcerationrst −1 + φ ((1 − 1r ) × Incarcerationrst −1 ) +γ 1t + δ 1s + μ (Trendt × 1s ) + ν (Trendt2 × 1s ) + ∑ λ j Characteristicsirstj (4) j +1r × {γ 1t + δ 1s + μ (Trendt × 1s ) + ν (Trend t2 × 1s ) + ∑ λ j Characteristicsirstj } + ε rist j I also take advantage of the White male prisoners series to run false experiments by regressing Black female outcomes on White male incarceration. Second, because incarceration rates might still pick-up effects not captured by the controls and that would simultaneously determine female outcomes, I instrument incarceration. Sentencing change are natural IV candidates: changes in sentencing policies occurred at the state level throughout the 1970s, 1980s and 1990s in most states. Given the political nature of the determinants of these laws (Dharmapala et al., 2006) it is unlikely that they are related to young women’s decisions regarding fertility, education, marriage or employment. Note that changes in state criminal codes do not appear to occur in response to an increase in crime either. I thus considered (1) determinate sentencing (i.e., the abolition of discretionary parole release), (2) alternative indicators of parole restriction (3) structured sentencing (recommended prison terms for offenses), either with presumptive sentencing (systems of single recommended sentences for each offense or offense class), or with presumptive or voluntary guidelines (systems of multiple sentence recommendations for each offense or offense class), (4) provisions enhancing sentences for second- and third- time offenders, violent offenders or drug offenders, (5) enactment by the states of the Federal Truth In Sentencing (TIS) program and (6) “three-strikes” laws. Another determinant of incarceration is prison capacity. Capacity is appealing as an instrument because decisions to build take years, sometimes more than a decade, before translating into operational facilities. Recall Levitt (1996) documents the lengthy course of 16 prison overcrowding litigation. In particular, he shows that states where overcrowding lawsuits are filed have higher than average incarceration growth rates before the filing and shorter ones afterwards. A common outcome for a state that has been sanctioned by courts for its prison overcrowding practice is to build new facilities – even though overcrowding litigation is not the only reason for the building of new prisons.22 If prison capacity expansion was simply the consequence of overcrowding in preexisting facilities so as to accommodate the excess number of prisoners already housed, it would have no predictive power on actual incarceration counts, and this seems to be the case for several states (e.g., South Carolina). However, the consequence of capacity expansion for incarceration can still be important: as long as prison overcrowding prevails, judges who are aware of the situation may be reluctant to send minor criminals to prison and may prefer to sentence them to probation. Similarly, at the margin, parole boards should be more generous in granting release and probation officers more hesitant to revoke paroles. Once new facilities are built, the capacity constraint is no longer binding. Empirically, this conjecture is validated in several states, especially for parole:23 there, I find that following a major stepping up of prison capacity the trend in the number of parolees decelerates or even gets 22 In general, prison capacity would be expanded for one of the following reasons: (1) a federal court concludes that the state’s current prison allocation of prisoners to cells is in violation of the 8th Amendment and orders fewer prisoners per cell, which requires expansion; (2) a jurisdiction changes its parole policies (typically meaning fewer offenders will be released); (3) the jurisdiction alters its policies on when someone is sent to jail versus a state prison facility (some states try to keep people in jails instead of prisons to save money and put the costs on localities; if the locality resists because the jails are full or for other reasons, prisons might need to be expanded). 23 Numbers of individuals on probation and parole come from a similar BJS series as for prisoners. 17 reversed. Most importantly for our purpose, the proportion of offenders sent behind bars increases accordingly. I provide the revealing examples of Texas and West Virginia in Graphs 3 and 4. They show the concomitant sudden increase in prison capacity and Black-male incarceration starting in the mid-1990s, as well as the abrupt decrease in the number of adult offenders on parole during that same period. Although I could not find a systematic study on this phenomenon,24 there is evidence in the data to support this causation mechanism, and it appears to be part of the culture of numerous state department of corrections officials I talked to.25 The opening of new facilities and the change in incarceration they induce should be, from the perspective of the young women I observe, largely exogenous. For sentencing changes, I used the compilation prepared by the Vera Institute of Legal Research (Stemen et al., 2005), the report on the influence of TIS reforms prepared by the Urban Institute (Sabol et al., 2002), and the BJS report on TIS in State Prisons (Ditton and Wilson, 1999). For measures of prison capacity, I used the yearly publication “Prisoners in Year X” published by the BJS. The BJS releases three distinct prison capacity statistics: rated capacity (defined as “the number of beds or inmates assigned by a rating official to institutions within the jurisdiction”), operational capacity (“the number of inmates that can be accommodated based on a facility’s staff, existing programs, and services”) and design capacity (“the number of inmates 24 25 This suction effect is reminiscent of the popular movie line “If you build it, they will come” (Field of Dreams). To give a recent example, a new prison was opened in Maine in early 2002, and the state prison population spiked by more than 11% – by far the highest growth rate in the U.S. that year (average: 2.6%). Previously the annual growth rate in Maine had been running below 2%. 18 that planners or architects intended for the facility”). Those last two statistics seem the most useful. However, the collection is imperfect, and data are missing for a large number of possible observations.26 Therefore, I created a dummy variable to capture the most dramatic increases in prison capacity (relative to a smooth trend) that occurred in those states where such a pattern can be found, and where the mechanism of capacity constraint described earlier appears to have been binding. In other words, in those states, the dummy variable takes value one if capacity increases sharply after a period of relative stability, or experiences a significant acceleration within a period of steady increase, and the number of offenders on parole initially decreases at the same time. This approach is conservative because some states do experience shifts in prison capacity trend that seem to parallel incarceration patterns but absent simultaneous changes for probation or parole, the causal mechanism is less compelling. When choosing those variables that will eventually be used in the Two-Stage Least Squares (2SLS) estimation, I run first-stage regressions from the different samples, including the source file (one observation per state/year), and select those variables, from the list of possible IV candidates, which are significant in most samples. To summarize, the sentencing change that appears to influence Black incarceration the most is presumptive sentencing , even though it is not significant when using the set of controls for state-level welfare generosity in the sample used to measure teen fertility decisions – other changes, including the much publicized “three- 26 Imputing the design capacity values with those for operational capacity (or the reverse) only makes sense when there is evidence that the series are equivalent or close enough when a state reports those two statistics at the same time. Unfortunately, this helps but in a few cases. 19 strike laws” have less impact on Black male incarceration.27 Table 2 provides dates following the year of enactment for each state: note that because presumptive sentencing started on or before 1979 in some of the highly populated states where it has been in use, the identification off state fixed effects is slim. The prison capacity change variable applied to those states that reverse their parole trend reveals itself as the most solid IV candidate. Since I have two different potential IVs, I run Sargan tests of overidentifying restrictions.28 None of the instruments is rejected according to those tests. For both the June and March CPS samples, the resulting F-test from the first stage, i.e., testing that both instrument coefficients are jointly equal to zero, is high (as expected this is driven by the second one): it strongly rejects the hypothesis that the instruments have no effect on incarceration. Table 3 shows the first stage for the teen fertility sample I focus on. However, the results from the IV strategy are not directly comparable to those which derive from the non-instrumented incarceration rates. This is because the IVs would affect the incarceration of some men more than others, and therefore affect women in different ways. This argument applies further when comparing the IVs against each other. In the present case, capacity expansion, being less perceptible to the offender population than changes in sentencing practices, should have more of a purely incapacitative, rather than deterrent, effect. It is also 27 The significance of either TIS or a synthetic indicator of parole restriction do not resist state-level standard errors clustering; this finding may be useful when evaluating other results on this theme by Greenberg and West (2001) and Jacobs and Carmichael (2001). 28 The test is partially informative regarding the IVs’ hypothesized exogeneity, not their power. For a detail of the procedure, see Wooldridge (2006). The presence of two instruments of different nature reinforces the strength of the test. 20 plausible that prison capacity expansion would bring into prisons a wider range of offenders, including less violent offenders who would otherwise be on probation, or parole violators, and it can be used to keep people who would otherwise be paroled. On the other hand, presumptive sentencing rules would typically affect sentence lengths for people who are already going to prison, and therefore, at the margin, they are more likely to target more serious criminals. To that extent, the IVs capture more detailed features of the problem at stake. Yet, for that same reason, the instrumental strategy should not be interpreted as a simple sensitivity analysis. Finally, a noticeable by-product of this analysis lies in the differences of impact of these instruments between White and Black male incarceration. There is already a large literature on whether sentencing policies apply differently between Blacks and Whites (see Spohn, 2000). Here, results for presumptive sentencing point toward a significant effect for Blacks and an insignificant effect for Whites, although they are sensitive to the specifications chosen. However, for prison capacity expansion, while it is significant for the White sample, the magnitude of the effect is considerably lower than for Black male incarceration and statistically different at very high levels of confidence across specifications. This result is new. A comprehensive investigation of this puzzle would take us too far, but different hypotheses will need to be tested to understand why the adjustment margin of capacity expansion would target Black offenders in priority. 21 IV. Results IV. 1. Fertility The results in Table 5 support the hypothesis that Black-teenage fertility declined as a consequence of increased Black-male incarceration. I present different estimations of the model that provide insight into the identification of the parameter of interest. Columns (1)-(2) showing the results of specifications that do not include state fixed effects, are presented for completeness. In model (3), which includes year and state fixed effects, the coefficient on incarceration is negative but insignificant. Yet, we have seen earlier that because incarceration increases almost every year in each state, the concern is that it may be still be confounded with other variables that exhibit somewhat parallel growth patterns over the period within states. When adding state-level linear and linear-quadratic time trends, in columns (4) and (5), the coefficient on incarceration remains negative but now becomes more precisely estimated and significant. An F-test on all state linear trends rejects the null hypothesis in model (4), and so does an F-test on all state linear and quadratic trends in model (5). The interpretation of the coefficient is now easier since incarceration is purged of the local effects previously picked up which, to the extent that they change slowly over time, are now well captured by the trend terms.29 A sensitivity analysis yields the following results. When dropping one Circuit Court at a time from model (5), all results hold except when removing Circuit Court 5. Digging further, the 29 The increase in adjusted R2, although small, is noticeable given the large number of variables added. Yet, in model (5), the quasi stability in adjusted R2 compared to model (4) suggests that there is nothing to be gained by adding even higher-order terms. 22 5% significance level breaks down if Texas alone (5% of the sample) is removed – although removing Texas during the 1980s only would leave the results unaffected. This indirectly confirms the role of the dramatic and much publicized increase of incarceration in Texas: it suggests a steep marginal effect of incarceration beyond a certain threshold.30 To strengthen this intuition, I partitioned the sample to single out those states which, at the end of the 1990s, reached Texas-like rates of adult Black male incarceration (in particular, Connecticut, Delaware, Iowa, Oklahoma, Rhode Island, Wisconsin): keeping those states only, plus Texas and Washington D.C., yields a 1% level coefficient with only 15% of the sample. Moving to checking the sensitivity of the results to the period considered, keeping only 1992-2000 is enough to retain a 5% level (with only 31% of the sample left). On the other hand, eliminating the 1990s leaves the results insignificant. Adding variables for state-level Black male unemployment or corresponding to different measures of state welfare generosity (model 6) does not alter the results qualitatively. These variables, however, are jointly significant.31 The role of welfare reforms, especially in the second half of the 1990s could explain why including time varying effects within states is important hence the abrupt change in the coefficient and significance from model (3) to models (4) and (5): in particular, one may think of states’ discretion in their use of TANF funds. 32 At 30 I also tested the hypothesis that the changes driven by the inclusion of state trends reflect the rapid increase in Hispanic population in some states. However, when removing states other than Texas with a large Hispanic population (California, New York, Florida, Illinois, Arizona and New Jersey), the results are virtually unchanged. 31 This result accords with the findings of Offner (2003) and Kaestner, Korenman, and O'Neill (2003). 32 The stated purposes of TANF are to: (a) Provide assistance to needy families so children may be cared for (b) End the dependence of needy parents on government benefits by promoting job preparation, work, and marriage (c) 23 the same time, it is plausible that changes in state-level welfare generosity, while not necessarily causal in nature, would be correlated with state-level incarceration policy, both of which being bent by the same “tougher” ideology towards social issues and by an evolution of local norms regarding tolerance and work ethics. Looking at the preferred estimation (model 6), the magnitude of the effect is sizable: at the means of the data, a 1% increase in the adult Black-male incarceration rate (per adult Black-male population 20-54 y/o) decreases the probability of having a child by 0.05. Recall that the average proportion of mothers in this sample is about 30%. Given an average adult Black-male incarceration rate of close to 4% this corresponds to an elasticity of -0.7. The presentation of this result becomes perhaps more compelling if we compare the effect of incarceration with that of age: in absolute terms, the decline in teenage fertility associated with a 1% increase in adult Black male incarceration rate is equivalent to the expected average increase in teenage fertility associated with seven extra months of age at age 19. I suspect that the effect would be even greater if I could more specifically capture the incarceration rate of younger adults. I apply different methods to affirm the robustness of these results, all of which make use of the controls included in model (5). Looking into the Black/White comparison, the interaction coefficient Black × incarceration rate in model (7) shows that in response to male incarceration in their group, Black females reduce their fertility relative to White females, but not significantly. In the White population in the same age range, the effect measured here by the Prevent and reduce the incidence of out-of-wedlock pregnancies and (d) Encourage the formation and maintenance of two-parent families. 24 racial-specific incarceration coefficient, is positive, but insignificant.33 I also regressed Black teenage fertility on White male incarceration, which was found to be insignificant. Finally, I run a 2SLS estimation using sentencing and prison capacity changes as instruments. The IV analysis confirms the previous analysis and the coefficient is significant at the 5% or 1% level depending on the specifications.34 The result is also robust to the exclusion of the state trend terms. Although the point estimate is higher, the Davidson and McKinnon (1993) test cannot reject the hypothesis that the IV coefficient is equal to the OLS coefficient at the 5% level. Note that the average incarceration rate is higher in the states that contribute to the identification than in the others. This could explain part of the discrepancy in point estimates. Further, I already suggested that the coefficients are not comparable: the IVs influence the incarceration of certain men more than others. To the extent that the IVs more particularly affect marginal offenders, hence potential matches, it is logical that the magnitude of the IV coefficient would be actually higher. To be more specific, the driving IV here is prison capacity expansion: in other words, the results hold when keeping that IV alone, the reverse it not true for presumptive sentencing.35 33 I could not find plausible exogenous background characteristics leading to differential treatment within either group. Kamdar (2007) reports a small negative coefficient among low-income families White females. Selecting White women with below average grade for age yielded inconclusive results. However, the significant effects found for the overall Black sample is indeed more pronounced among below average grade for age young women. 34 The result is confirmed when using actual operational capacity data over the fraction of the sample where the information is available. 35 In this case, the result is consistent with a comparison of the separate F-tests, i.e., could come from the fact that the prison capacity IV is a stronger instrument than presumptive sentencing. Alternatively, or concurrently, I offered a 25 Overall, the results on fertility converge to the conclusion that the sheer magnitude of adult Black-male incarceration is enough to significantly reduce Black teenagers’ non-marital fertility, a result further supported by Kamdar (2007). This conclusion goes against the qualitative argument that the smaller number of men leads to more bargaining power on the male side and in turn, more extra marital relations and pregnancies (Courtwright, 1996). Quantitatively, my results run opposite to those of South and Lloyd (1992) who found that in 1980, male scarcity broadly defined had no significant effect on the non-marital fertility rate for any age range among Blacks.36 However, the study was conducted at a time when Black-male incarceration rates were much lower than the average in my sample. Further, the effect of the sex ratio needs not be linear. This would be consistent with finding a positive (non significant) effect among the White sample and a consistently negative effect in the Black sample. Presumably, small deviations from a unitary sex ratio could produce the kind of consequences Courtwright is describing. On the other hand, at some point, large shortages of men would inevitably lead to a decrease in fertility.37 According to Donohue and Levitt (2001), abortion availability, which should contribute to a reduction in teen births, led to a decline in crime with an 18 year lag. In simplistic terms, the argument presented here appears as the reverse, but this time with more immediate effects: in the hypothesis for why the marginal incarceration resulting from prison capacity expansion would affect those men responsible for impregnating teenage girls more than that resulting from presumptive sentencing. 36 Darity and Myers (1990) suggest that reducing the supply of marriageable mates would increase the proportion of Black families headed by females. Yet this is not inconsistent with the results presented here. 37 An analysis on completed fertility seems worthy of interest but falls beyond the scope of the present study. 26 Black community, the marginal impact of more men behind bars is now a decrease in early fertility. More research is necessary to identify whether this comes from an increase in the use of abortion, birth control methods or fewer sexual relations altogether. In particular it would be challenging to determine if those women forego early motherhood because of a simple shortage of partners or because they anticipate that the father of a potential child may not stay around in case he becomes incarcerated, or leaves all the more easily since there is an excess supply of women on the market. It goes without saying that finding a new man to support a single mother should be increasingly difficult in an environment where (free) men benefit from a rent. IV. 2. Other Outcomes IV. 2. 1. Education I followed the same methodology to study the impact of male incarceration rates on education for single Black women. Yet, a methodological challenge arises: the coding of education changes between the pre-1992 and post-1991 periods in the CPS. Unfortunately, there is no satisfactory recode that would make the series perfectly consistent over the two periods without too much loss in information (that proposed by Jaeger (1997) still has problems). I therefore investigated the two periods 1979-1991 and 1992-2000 separately. I focused on educational attainment for the age bracket 19-21, because it is likely that the relevant margin would be whether to complete high school or pursue some education beyond high school. Heuristically, the results point toward an effect of male incarceration concentrated at age 20; recall this measures incarceration when these women were 19, which for most of them, corresponds to the end of high school. 27 To summarize the results for 1979-1991, when adding year and state fixed effects, the coefficient on male incarceration becomes positive and significant, and the inclusion of statespecific time trends strengthens this finding. Adding the extra controls (state unemployment rates, welfare measures) leaves the conclusion unchanged.38 While the White female educational response to White male incarceration is negative and insignificant, the Black/White difference is significant at the 10% level. Note that for 1979-1991, the capacity expansion-based IV relies on a small number of observations from Rhode Island thus the IV results, although supportive of a significant effect, should be taken with caution given the unexpected magnitude of the coefficient in column (9). On the other hand, the corresponding results from the 1992-2000 period are not as persuasive, but this appears to stem from the smaller number of observations (about a thousand). When enlarging the age bracket considered, the regressions for 1992-2000 exhibit coefficients similar to those for 1979-1991 and, in particular, the results from the IV estimations become highly significant across specifications. The evidence is thus in favor of an effect of Black male incarceration on Black women’s education. Finally, education is not the only way through which young women can gain financial independence and self-reliance in response to aggregate male incarceration. Male incarceration could spur women to join the labor-force, become full-time employed or augment their hours worked. In the following section, I explore those hypotheses. IV. 2. 3. Employment Studying the impact of Black-male incarceration on Black-female employment presents more difficulties of interpretation. White and Black females compete for the same jobs more than they compete for the same men. Also, the local level of aggregate Black male incarceration could be 38 The false experiment of regressing Black education on White incarceration rates produces insignificant results. 28 likely to be correlated with employers’ attitudes (and perhaps bias) towards Blacks in general. Another problem is that employment is a flow. The previous two outcomes were the product of irreversible or quasi-irreversible decisions: a woman is a mother by age twenty or she is not, she either graduates from high school or she does not; cases of going back to school in adult life are rare. In contrast, work status is adjustable: one can move in and out of the labor force, partly in response to current labor conditions. Incarceration rates could therefore influence employment at any age. In the following, I concentrate on early employment. Several margins may be considered. Empirically, the results yield significant and sizeable coefficients for women in their early 20s, especially with regard to full-time employment. Those are presented in Table 7. The IV estimation produces the same kind of confirmation as earlier, with the driving IV still being prison capacity expansion. The conclusion is that Black male incarceration leads more young Black women to work full time.39 The Black/White differential analysis points to a reverse movement for young White women, which coincides with the previously documented convergence between Black female and White female early employment.40 That some Black women increase their employment in response to Black-male incarceration is intuitive given the above. Absent exogenous background characteristics, one may still refine these results. I find that they are driven by those women who are in the bottom half of the education distribution, and by married women. The first observation, i.e., women with lower 39 On the other hand, I did not find an effect on full time employment conditional on labor force participation. 40 More generally, the participation rate for women 16 to 24 years old has been a major source in the deceleration of female employment in general (Hayghe, 1997). Given that Whites outnumber Blacks roughly 8:1, one may therefore view rising male incarceration as a significant factor underlying this trend. 29 education should be most affected at the margin, makes sense. The second observation is less intuitive. However, married women in that age bracket are on average less educated than single women, so one reason why married women contribute to the increase in employment is because of the overlap with lower education. However, when selecting married women in the top half of the education distribution, the results still hold. I believe an interpretation for this phenomenon is the increasing uncertainty of the returns to marriage for these young women. First, there is a higher chance that their husbands, who are typically slightly older, thus in the most dangerous age range for incarceration, will become incapacitated. Second, the increased bargaining power of men should spur women to seek counter measures, and the most obvious way to achieve that goal is to become financially independent (or less dependent): according to Seitz (2007), a decrease in the ratio of men to women translates into decreased intra-household transfers to wives, which implies that black women are predicted to work more because they receive lower intra-household transfers. Third, an explanation could be the consequence of the effect of incarceration on education: those women who decided to increase their education as an insurance against an unfavorable marriage market – but still managed to get married – are in a better position to get a job. Given that the effect on education is concentrated at the high-school completion stage more so than at the college level, it is therefore logical to see an effect as early as at age 20-22. Finally, the June CPS confirms that married Black women respond to incarceration more than single ones, but also reveals that young Black married mothers significantly contribute to the increase in full-time employment among young Black women in general. This result is consistent with the observation that much of the recent increase in women’s labor force 30 participation can be attributed to the rising participation rates of women with young children (Cohen and Bianchi, 1999). IV. 2. 4. Marriage It sounds like a legitimate conjecture that by sending men to prison and thereby removing them from the marriage market, the marriage rate should mechanically fall. For Seitz (2007), the options of Black women outside marriage, combined with the poor labor market opportunities of Black males, provide an explanation for the low marriage rates in the Black population: the increased prospect of incarceration for a spouse would thus only contribute to the lower expected returns to marriage. Incarceration could have even larger effects by inflicting the stigma of prison on those who come back to the marriage market after their release. Yet, such a hypothesis would only hold if, absent incarceration, those men were indeed to get married. Incarcerated Black men are only about half as likely to be married as non-institutionalized men of the same age (Western, 2004) but one may not infer causality from that fact: prisoners may simply be barred from the marriage market through incapacitation; alternatively, many of them may not be “marriage material” in the first place. Further, some young women may prefer to marry earlier and secure a mate rather than face a stiff competition in the future. The removal of some men from the marriage market pool can generate another offsetting, indirect effect: in a perverted way, the judicial system is performing some of the screening process by filtering the good matches from the bad ones, hence eliminating search frictions. This compounds the already mentioned effect of incarceration on non-incarcerated men in terms of deterrence and improved behavior. Overall, the resulting outcome is ambiguous. 31 Using the same methodology as in the previous sections, I was not able to find a clear, consistent and significant effect of Black-male incarceration on the probability of having been married for young Black women. Even if some of the OLS-based evidence supports the hypothesis that Black male incarceration decreases the probability of marriage, it was not confirmed using IV.41 There is no apparent reason to think that neither of the IVs would affect the incarceration of men who would otherwise have gotten married. In fact, Wood (1990) already argued that the lack of “quality men” is only marginally responsible for the decline in marriage rates in the Black community. Similarly, Myers (2000) found “little support for the theoretically plausible hypothesis that there are strong unintended impacts of imprisonment policies on family structure.” My conclusion would therefore concur. On the other hand, it contrasts with the findings presented by Charles and Luoh (2006). One hypothesis to account for the discrepancy is the result I obtained from a regression using years 1980, 1990 and 2000 only, that is mimicking Charles and Luoh’s sample: in that case, I did find a highly significant negative impact on the likelihood to ever be married using both OLS and IV estimations. This suggests a conclusion to be tested more generally that the benefit of IVs may not overcome the loss of information that results from exploiting sample waves too far apart. 41 More specifically, the IV specification produces significant negative effects for the period 1979-1994 when the identification from the capacity expansion IV comes from a small number of observations in Rhode Island. After 1994, the identification comes from a larger number of observations from different states and the effect disappears. 32 V. Conclusion This work shows that the massive incarceration of Black males in the U.S. has perceptible effects on Black women in their teen years and early to mid twenties. In particular, Black-male incarceration decreases early Black non-marital fertility and increases Black-female education and early employment. The evidence linking male incarceration and marriage is less persuasive. The conclusion of this work might be construed as running against the traditional wisdom that an unfavorable gender ratio results in adverse consequences for females. Yet, the deduction that Black women’s welfare has increased because of Black male incarceration would certainly misrepresents the message conveyed here. In a basic marriage-market model assuming rational agents, a shock in the supply of men such as that produced by massive incarceration should make women worse off at the margin.42 The study of the effects of massive male incarceration on women’s outcomes, a case of “tectonic economics” (Krueger, 2006), is in its infancy. For example, Johnson and Raphael (2005) advance that the higher prevalence of HIV among Black women is connected to Black male incarceration rates. Additionally, Cunningham (2007) finds that skewed sex ratios measured by the relative incarceration of men vs. women cause men to have more female 42 Certainly, this simple argument could be qualified. For instance, the large-scale confinement of working men could become a positive externality on labor market-oriented women. Alternatively, if women’s choices were previously constrained by men, for example if we believe that some women were bullied into demeaning roles, a decrease in early fertility, and an increase in education and job-market attachment could be viewed as beneficial. Still, these indirect effects would have to be extremely large to compensate for the direct decrease in welfare from choices made in the context of a crisis of gigantic proportions in the Black community. 33 partners in the Black community. Further exploration will give us a more comprehensive view of the different channels through which aggregate male incarceration affects women. Bibliography Allen, W. R., U. M. Jayakumar, K. A. Griffin, W. Korn and S. Hurtado (2005) “Black Undergraduates From Bakke to Grutter,” Higher Education Research Institute, UCLA Graduate School of Education & Information Studies publication. Bertrand, M., E. Duflo and S. Mullainathan (2004) Quarterly Journal of Economics 119(1): 249-75 Bureau of Justice Statistics “Correctional Population in the United States” series (19852001); “Prisoners in State and Federal Institutions on December 31st” series (1978-1984); and “Prisoners in year X” series (1983-2005) and “Adults on parole, Federal and State-by-State” (1975-2004) series Brien, M. (1997) “Racial Differences in Marriage and the Role of Marriage Markets,” Journal of Human Resources 32: 741-778 Charles, K. K. and M. C. Luoh (2006) “Male Incarceration, the Marriage Market and Female Outcomes, mimeo,” Department of Economics, University of Michigan Courtwright D. T. (1996) “The Drug War's Perverse Toll,” Issues in Science and Technology 13(2). CPS June 1979-2000 (not all inclusive). [machine readable data files] / conducted by the Bureau of the Census for the Bureau of labor Statistics. Washington: Bureau of the Census [producer and distributor] 1971-2004. Santa Monica, CA: Unicon Research Corporation [production and distributor of CPS utilities] 2005. CPS March 1979-2000. [machine readable data files] / conducted by the Bureau of the Census for the Bureau of labor Statistics. Washington: Bureau of the Census [producer and distributor] 1962-2004. Santa Monica, CA: Unicon Research Corporation [production and distributor of CPS utilities] 2005. 34 Cunningham S. A. (2006) Sex Ratios and Risky Sexual Behavior, Working paper, Department of Economics, University of Georgia, Darity, W. A., and S. L. Myers (1990) “Impacts of Violent Crime on Black Family Structure,” Contemporary Economic Policy 8(4): 15-29. Davidson, R., and J. G. MacKinnon (1993) Estimation and Inference in Econometrics, New York: Oxford University Press Dharmapala, D., N. Garoupa, J. Shepherd (2006) “Sentencing Guidelines, TIS Legislation, and Bargaining Power,” mimeo, Emory University School of Law Ditton, P. M., and D. J. Wilson (1999) “Truth in Sentencing in State Prisons,” Bureau of Justice Statistics Special Report NCJ 170032 Donohue, J. J. III and S. D. Levitt (2001) “The Impact of Legalized Abortion on Crime,” Quarterly Journal of Economics 116(2): 379-420 Garland, D., 2001. Mass Imprisonment, Social Causes and Consequences, Sage Publications Ltd Greenberg, D. F., and V. West (2001) “State Prison Populations and their Growth, 19711991,” Criminology 39(3): 615–53. Hagan, J. and R. Dinovitzer (1999) “Collateral Consequences of Imprisonment for Children, Communities and Prisoners,” Crime and Justice 26: 121-162. Harcourt, B. E. (2006) “From the Asylum to the Prison: Rethinking the Incarceration Revolution,” U Chicago Law & Economics, Olin Working Paper No. 277 Horton H. D., and N. Burgess (1992) “Where are the Black Men, Regional Differences in the pool of marriageable Black males in the U.S.,” National Journal of Sociology 6: 3-19. Jacobs, D., and J. T. Carmichael (2001) “The Politics of Punishment across Time and Space: A Pooled Time-Series Analysis of Imprisonment Rates.” Social Forces 80(1): 61–91. Jaeger D. A., (1997) “Reconciling Educational Attainment Questions in the CPS and the Census,” Monthly Labor Review 120: 36-40. Johnson, R. C., and S. Raphael (2005) “The Effects of Male Incarceration Dynamics on AIDS Infection Rates among African-American Women and Men,” Working Paper, Goldman School of Public Policy, U.C. Berkeley Kaestner, R., S. D. Korenman and J. E. O’Neill (2003) “Has Welfare Reform Changed Teenage Behaviors?,” Journal of Policy Analysis and Management 22(2): 225-248 35 Kamdar, Amee (2007) Male Incarceration and Teen Fertility, Working paper, University of Chicago Graduate School of Business Fang, H., and M. P. Keane (2004) “Assessing the Impact of Welfare Reform on Single Mothers,” Brookings Papers on Economic Activity 1: 1-95 Kiecolt, J. K., and M. A. Fossett (1997) “Mate Availability, Economic Opportunity, and Marriage among Black Americans,” pp. 63-78 in Family Life in Black America, edited by R. J. Taylor. Newbury Park, CA: Sage Publications Krueger, A. (2006) quoted on economicprincipals.com, January 15th 2006 Lane, S. D., R. H. Keefe, R. A. Rubinstein, B. A. Levandowski, M. Freedman, A. Rosenthal, D. A. Cibula, M. Czerwinski (2004) “Marriage Promotion and Missing Men,” Medical Anthropology Quarterly 18(4): 405-28. Levitt, S. D. (1996) “The Effect of Prison Population on Crime Rates: Evidence from Prison Overcrowding Litigation,” Quarterly Journal of Economics 111: 319-352 Lichter, D. T., D. K. McLaughlin, G. Kephart and D. J. Landry (1992) “Race and the retreat from marriage: A shortage of marriageable men?,” American Sociological Review 57: 781-799. Lynch, J. P. and W. J. Sabol (2003a) “Effects of incarceration on informal social control in communities,” in B. Western, D. F. Weiman and M. Pattillo (eds.) The Impacts of Incarceration on Families and Communities, New York: Russell Sage Foundation. Lynch, J. P. and W. J. Sabol (2003b) “Assessing the Longer-run Consequences of Incarceration: Effects on Families and Employment,” in Crime Control and Social Justice: the Delicate Balance, edited by D. Hawkins, S. L. Myers, and R. Stone: Greenwood Press. Mauer, M. and M. Chesney-Lind (eds), 2002. Invisible Punishment: The Collateral Consequences of Mass Imprisonment New Press. Meares, T. L. (2004) “Mass Incarceration: Who Pays the Price for Criminal Offending?,” Criminology & Public Policy 3(2): 295-302. Mincy R. B. (2006) Black Males Left Behind, Urban Institute Press Mundy, L. (2006) “What’s Really behind the Plunge in Teen Pregnancy? It’s time to look at boys’ contributions,” Slate Magazine, Wednesday, May 3rd, 2006 Myers, S. L. (2000) “The Unintended Impacts of Sentencing on Family Structure,” report presented at the Washington D.C.,” ASA Annual Meeting 36 Neal, D. (2004) “The Relationship between Marriage Market Prospects and Never-Married Motherhood,” Journal of Human Resources 39(4): 938-957. Offner, P., and H. Holzer (2002) “Left Behind in the Labor Market: Recent Employment Trends Among Young Black Men,” Center on Urban and Metropolitan Policy, The Brookings Institution, Survey Series (April). Offner, P. (2003) “Teenagers and Welfare Reform,” Washington, DC: Urban Institute. Oliver, P. E., G. Sandefur, J. Jacubowski and J. E. Yocom (2006) “Have High Black Imprisonment Rates contributed to African American Child Poverty?” mimeo, Department of Sociology, University of Wisconsin Madison, Sabol, W. J., K. Rosich, K. Mallik Kane, D. P. Kirk, and G. Dubin (2002) “The influence of TIS reforms on changes in state's prison sentencing practices and prison populations,” Urban Institute research report to the National Institute of Justice, Seitz, S. (2007) “Accounting for Racial Differences in Marriage and Employment,” working paper, Department of Economics, Boston College Spohn, C. C. (2000) “Thirty Years of Sentencing Reform: The Quest for a Racially Neutral Sentencing Process,” in Policies, Processes, and Decisions of the Criminal Justice System, volume 3 in Criminal Justice 2000, National Institute of Justice, Washington, DC South, S. J. and K. M. Lloyd (1992) “Marriage Markets and Nonmarital Fertility in the United States,” Demography 29(2): 247-264. Stemen, D., A. Rengifo and J. Wilson (2005) “Of Fragmentation and Ferment: The Impact of State Sentencing Policies on Incarceration Rates, 1975-2002, Mimeo, Vera Institute of Justice Thomas, A. (2006) “The Old Ball and Chain: Unlocking the Correlation between Incarceration and Marriage,” mimeo, Kennedy School, Harvard University Tucker, M. B. and C. Mitchell-Kernan (editors.) (1995) The decline in marriage among African Americans: Causes, consequences, and policy implications. New York: Russell Sage Foundation. Vera Institute of Justice (1996) “The unintended Consequences of Incarceration,” Vera Institute of Justice Conference Proceedings Western B., and S. McLanahan (2000) “Fathers Behind Bars: The Impact of Incarceration on Family Formation,” Contemporary Perspectives in Family Research 2: 307-322 37 Western, B. (2004) “Incarceration, Marriage, and Family Life,” Mimeo, Department of Sociology, Princeton University Western, B., and B. Pettit (2005) “Black-White Wage Inequality, Employment Rates, and Incarceration,” American Journal of Sociology 111(2): 553-78 Wilson, W. J. (1987) The Truly Disadvantaged: The Inner City, the Underclass, and Public Policy. Chicago: The University of Chicago Press Wood, R. (1990) “Marriage Rates and Marriageable Men: A Test of the Wilson Hypothesis,” The Journal of Human Resources 30: 163-193 38 Graph 1: Male Incarceration rate per 20-54 y/o Male Population, by race 7.00 6.00 Unweighted BMPRate 20-54 5.00 Weighted BMPRate 20-54 4.00 3.00 Unweighted WMPRate 20-54 2.00 Weighted WMPRate 20-54 1.00 99 97 19 95 19 93 19 91 19 89 19 87 19 85 19 83 19 81 19 19 19 79 0.00 Graph 2: Birth Rates for Teenagers age 18-19 by race, 1964-2001 Source: NCHS Reports 1979-2002 300.0 250.0 200.0 White Black 150.0 100.0 50.0 39 00 98 20 96 19 94 19 92 19 90 19 88 19 86 19 84 19 82 19 19 80 19 78 19 76 19 74 19 72 19 70 19 68 19 66 19 19 64 0.0 Graph 3: Texas Prison Capacity, Black Male incarceration and Total Adults on Parole 180000 160000 140000 120000 Prison Capacity 100000 Number of Black Men Incarcerated 80000 Adults on Parole 60000 40000 20000 98 96 19 94 19 92 19 19 90 88 19 86 19 19 84 19 82 80 19 19 19 78 0 Graph 4: West Virginia Prison Capacity, Black Male Incarceration, and Total Adults on Parole 3000 2500 Prison Capacity 2000 Number of Black Male Incarcerated 1500 Adults on Parole 1000 500 40 98 19 96 19 94 19 92 19 90 19 88 19 86 19 84 19 82 19 80 19 19 78 0 Table 1: Black-Male Incarceration Rate per Black Male Population Age 20-54, by State, 1978-1999 (%) State Average Std Dev Min Max 1.346 5.92 1.656 Alabama 3.932 Alaska 4.067 1.84 1.653 7.595 Arizona 6.257 1.477 3.852 8.158 Arkansas 4.369 1.534 2.091 6.389 California 4.411 1.904 1.651 7.094 Colorado 3.829 1.884 1.865 7.006 Connecticut 6.413 2.493 3.093 11.016 Delaware 7.204 2.015 3.788 11.119 Florida 5.274 1.387 3.4 7.339 Georgia 3.368 0.933 2.247 4.893 Hawaii 1.014 0.432 0.191 1.624 Idaho 2.402 0.424 1.624 3.095 Illinois 3.999 1.606 1.942 6.58 Indiana 4.407 1.594 1.721 6.841 Iowa 7.003 2.503 3.809 11.431 Kansas 5.057 1.595 2.631 7.455 Kentucky 4.264 2.166 1.794 8.048 Louisiana 4.652 1.719 2.325 7.674 Maine* 1.373 0.663 0.69 2.829 Maryland 3.902 0.737 2.6199 4.762 Massachusetts 2.865 0.603 2.009 3.752 Michigan 5.384 1.862 2.997 7.674 Minnesota 3.576 1.115 2.45 5.317 Mississippi 3.091 1.276 1.181 5.764 Missouri 4.848 1.971 2.541 8.098 Montana 2.895 1.066 1.403 5.269 Nebraska 5.062 0.742 3.728 6.12 Nevada 6.276 1.388 3.483 7.75 New Hampshire 2.146 1.608 0.35 4.815 New Jersey 4.171 1.695 1.832 6.689 New Mexico 3.386 0.580 2.424 4.297 New York 3.417 0.999 2.09 4.826 North Carolina 3.480 0.882 2.697 5.068 North Dakota 2.735 1.119 1.105 4.903 Ohio 2.851 0.984 1.809 5.452 Oklahoma 6.453 2.894 2.85 10.69 Oregon 5.188 1.231 2.745 7.164 Pennsylvania 4.502 1.944 2.003 7.463 Rhode Island 5.750 2.28 2.333 9.352 South Carolina 3.852 1.206 2.284 5.409 South Dakota 2.735 1.119 1.105 4.903 Tennessee 2.851 0.984 1.809 5.452 Texas 5.338 2.719 2.99 10.45 Utah 4.617 1.121 2.892 6.536 Vermont** 2.552 1.788 0.303 6 Virginia 3.587 1.251 2.182 5.454 Washington 3.738 0.817 2.711 5.158 Washington D.C. 7.698 3.449 2.591 12.190 West Virginia 2.208 0.756 1.528 3.888 Wisconsin*** 6.256 3.014 3.520 13.689 Wyoming 4.136 1.608 1.563 6.678 * Year 1996 missing Years 1982-1993 missing *** Year1978 missing ** 41 Table 2: Selected Sentencing* and Capacity Expansion Changes,** 1978-1999 Presumptive State Major Capacity Sentencing Expansion Change Alabama Alaska 1982-1999 Arizona 1979-1999 Arkansas California 1978-1999 Colorado 1980-1999 Connecticut Delaware Florida Georgia Hawaii Idaho Illinois Indiana 1978-1999 Iowa 1995-1999 Kansas Kentucky Louisiana Maine Maryland Massachusetts Michigan Minnesota Mississippi Missouri Montana Nebraska Nevada New Hampshire New Jersey 1978-1999 New Mexico 1978-1999 New York North Carolina North Dakota Ohio 1997-1999 Oklahoma Oregon Pennsylvania Rhode Island 1982-1999 1988-1999 South Carolina South Dakota Tennessee Texas 1994-1999 Utah Vermont Virginia Washington Washington D.C. West Virginia 1995-1999 Wisconsin 1996-1999 Wyoming * Source: Stemen et al. (2005). Relative to a linear-quadratic state-level time trend concurrent with a shift in trend of parolees– see text for details. Source: BJS “Prisoners in Year X” series and “Adults on parole, Federal and State-by-State, 1975-2004”. ** 42 Table 3: First Stage Linear Regressions with robust standard errors clustered by state Dependent Variable: “State/Year Black Male Prisoners per 20-54 Black Men (%)” (1) (2) Presumptive Sentencing (Dummy variable) -0.542 (0.288)* -0.683 (0.682) Major Capacity Expansion (Dummy variable) 1.925 (0.125)*** 1.849 (0.093)*** Adjusted R2 0.977 0.985 F-test (both IV =0) 158*** 199*** 1,779*** F-test (all extra variables =0) # Observations 5,369 5,133 Sample used in Table 5; Models (1)-(2) contain year, state, state×trend and state×trend2 effects; Model (2) contains the following extra variables: State-level Black men unemployment rate and different measures of state-level welfare generosity as coded in Fang and Keane (2004), namely: child support (existence and amount of pass-through program, existence, amount and rate for disregard from pass through), flat income disregard, AFDC/TANF payment standards for one person with two and with three children, child support enforcement expenditures, existence of work requirement and the degree in which the work requirement is binding for the year, the length of time in months allowed on welfare before work requirement hits, the age in months of the youngest child that the states allow the women to be exempt from work requirement, exemption if the woman is disabled or ill; exemption if a family member of the woman is disabled, exemption if the child care is not available for a kid less than 6, if the state has full (vs. partial) sanction, age of the child for exemption, and state EITC rules. * 10% significance; ** 5% significance; *** 1% significance Table 4: Summary Statistics of Dependent Variables in Tables 5, 6 and 7 # Observations Average Std Dev Min Max “Whether a Mother,” (Black) 5,369 0.3 - 0 1 “Whether a Mother,” (White) “Educational attainment” (Black) “Educational attainment” (White) “Full Time Employed” (Black) “Full Time Employed” (White) 8,987 0.063 - 0 1 1,793 12.55 1.72 0 18 9,850 13.13 1.727 0 18 8,324 0.29 - 0 1 56,567 0.392 - 0 1 43 Black Prison rate 20-54 y/o (1) Table 5 Linear Regressions with robust standard errors clustered by state Sample: June CPS unmarried Black women age 18-20 (1979-85, 1990, 1992, 1994-1995, 1998, 2000) Dependent Variable: “whether a mother” (8) (IV) (2) (3) (4) (5) (6) (7)† 0.001 0.001 -0.002 -0.039 -0.043 -0.056 -0.1 -0.1 -0.129 -0.148 (0.004) (0.009) (0.011) (0.016)** (0.021)** (0.024)** (0.044)** (0.05)** (0.019)*** (0.022)*** Prison rate (9) (IV) (10) (IV2)‡ (11) (IV2)‡ 0.052 (0.052) Prison rate×Black -0.095 (0.058) Year Yes State Yes Yes Yes Yes Yes Yes Yes Yes Yes Yes Yes Yes Yes Yes Yes Yes Yes Yes Yes Yes Yes Yes Yes Yes Yes Yes Yes Yes Yes Yes Yes Yes Yes State×Trend 2 State×Trend Extra Controls• Yes Yes Yes Adjusted R2 0.017 0.019 0.032 0.036 0.036 0.034 0.108 0.034 0.033 0.032 0.031 # Observations 5,369 5,369 5,369 5,369 5,369 5,133 34,356 5,369 5,133 5,369 5,133 All models control for age, age2 † In model (7) using both Blacks and Whites, the prison rate is race-specific and all the controls interacted with the white dummy are added – see Equation (4). ‡ IV2 refers to IV estimation with prison capacity expansion as sole IV. • State-level Black Men Unemployment rate and different measures of state-level welfare generosity– see text for details. * 10% significance; ** 5% significance; *** 1% significance 45 Black Prison rate 20-54 y/o (1) Table 6 Linear Regressions with robust standard errors clustered by state Sample: March CPS unmarried Black women age 20 (1979-1991) Dependent Variable: “Last attended grade”○ (2) (3) (4) (5) (6) (7)† (8) (IV) (9) (IV) -0.028 -0.018 2.22 (0.048) (0.06) 0.119 (0.064) 0.368 0.614 0.6 0.678 (0.163)** (0.242)** (0.271)** Prison rate (0.147)*** (0.915)** -0.833 (0.754) Prison rate×Black 1.447 (0.772)* Year Yes State Yes Yes Yes Yes Yes Yes Yes Yes Yes Yes Yes Yes Yes Yes Yes Yes Yes Yes Yes Yes Yes Yes Yes Yes Yes Yes State×Trend State×Trend2 • Yes Extra Controls Adjusted R2 ≈0 0.001 0.013 0.02 0.018 0.022 0.319 0.018 0.001 # Observations 1,793 1,793 1,793 1,793 1,793 1,711 11,643 1,793 1,711 † In model (7) using both Blacks and Whites, the prison rate is race-specific and all the controls interacted with the white dummy are added – see Equation (4). State-level Black Men Unemployment rate and different measures of state-level welfare generosity– – see text for details. * 10% significance; ** 5% significance; *** 1% significance • 47 Black Prison rate 20-54 y/o (1) Table 7 Linear Regressions with robust standard errors clustered by state Sample: March CPS Black women age 20-22 (1979-93 and 1996-2000) Dependent Variable: “employed full-time” (8) (IV) (2) (3) (4) (5) (6) (7)† (9) (IV) 0.01 0.008 0.16 0.081 0.166 (0.043)*** (0.013)*** (0.047)*** -0.007 0.035 (0.04)** (0.008) (0.008) (0.01)*** 0.046 0.06 0.082 (0.019)** (0.022)** Prison rate (0.012)*** (10) (IV2) (11) (IV2)‡ -0.113 (0.06)* Prison rate×Black 0.159 (0.06)** Year Yes State Yes Yes Yes Yes Yes Yes Yes Yes Yes Yes Yes Yes Yes Yes Yes Yes Yes Yes Yes Yes Yes Yes Yes Yes Yes Yes Yes Yes Yes Yes Yes Yes Yes State×Trend 2 State×Trend Extra Controls• Yes Yes Yes Adjusted R2 0.016 0.022 0.038 0.044 0.045 0.044 0.03 0.044 0.043 0.044 0.04 # Observations 8,324 8,324 8,324 8,324 8,324 8,324 64,891 8,324 7,919 8,324 7,919 Years 1994-95 missing. All models control for age, age2 † In model (7) using both Blacks and Whites, the prison rate is race-specific and all the controls interacted with the white dummy are added – see Equation (4). ‡ IV2 refers to IV estimation with prison capacity expansion as sole IV. • State-level Black Men Unemployment rate and different measures of state-level welfare generosity– see text for details. * 10% significance; ** 5% significance; *** 1% significance 49